Latest YouTube Video
Saturday, March 26, 2016
Allow registered users and anonymous users to add node with Password
from Google Alert - anonymous http://ift.tt/1RGyZPJ
via IFTTT
Ravens: Close to 50 coaches, executives and players attend Tray Walker's funeral; John Harbaugh offers \"touching sermon\" (ESPN)
via IFTTT
I have a new follower on Twitter
esta nole
USA
Following: 730 - Followers: 0
March 26, 2016 at 03:51PM via Twitter http://twitter.com/estaifp3445
Ravens: John Harbaugh is demanding on the field but unique in the way he's \"loyal and loving\" off of it - Van Valkenburg (ESPN)
via IFTTT
I have a new follower on Twitter
Jeff
pizza
Following: 4098 - Followers: 1970
March 26, 2016 at 08:08AM via Twitter http://twitter.com/Jeff65248334
Facebook's latest feature Alerts You if Someone Impersonates Your Profile
from The Hacker News http://ift.tt/1UjImFy
via IFTTT
How do I add anonymous users to view content of a groep?
from Google Alert - anonymous http://ift.tt/1MrhxMY
via IFTTT
Microsoft says It's Deeply Sorry for Racist and Offensive Tweets by Tay AI Chatbot
from The Hacker News http://ift.tt/1ocQ461
via IFTTT
New Bill targets Anonymous Prepaid 'Burner' phones by requiring Registration
from The Hacker News http://ift.tt/1LOAXeJ
via IFTTT
Global Temperature Anomalies from January 2016
from NASA's Scientific Visualization Studio: Most Recent Items http://ift.tt/22LZQf6
via IFTTT
Arctic Sea Ice Maximum - 2016
from NASA's Scientific Visualization Studio: Most Recent Items http://ift.tt/22LZQeY
via IFTTT
Close Comet and the Milky Way
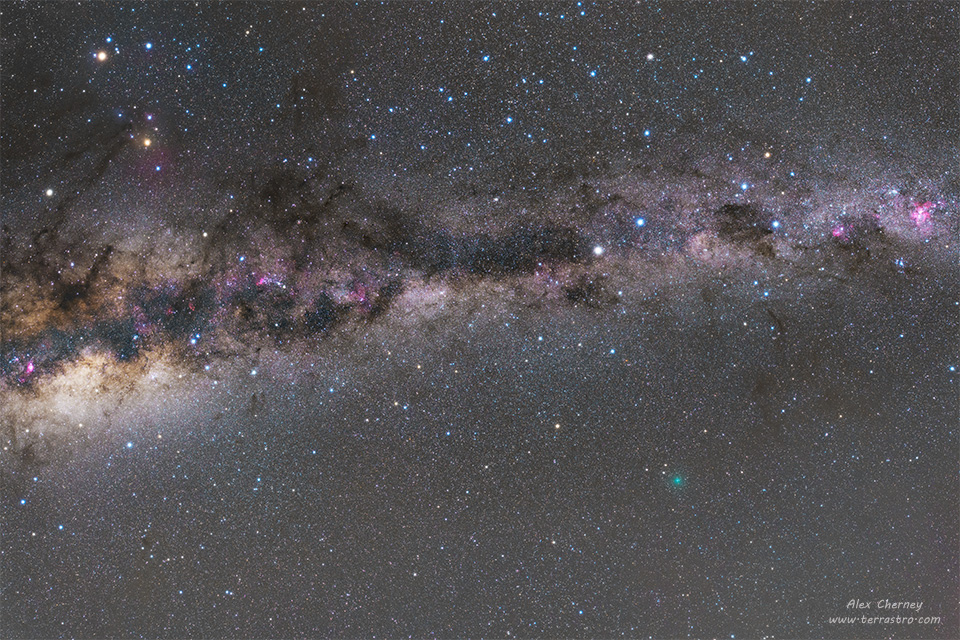
Friday, March 25, 2016
Woman marries anonymous sperm donor, the father of her daughter
from Google Alert - anonymous http://ift.tt/1RsvSYu
via IFTTT
Orioles Video: Team is \"hopeful\" P Kevin Gausman (shoulder) will be ready shortly after Opening Day, says Buster Olney (ESPN)
via IFTTT
ISS Daily Summary Report – 03/25/16
from ISS On-Orbit Status Report http://ift.tt/21KW5nE
via IFTTT
Google Calendar Events get GMT Timezones for Anonymous Users
from Google Alert - anonymous http://ift.tt/1VNpH4u
via IFTTT
Woman Tracks Down Her Anonymous Sperm Donor...And Marries Him
from Google Alert - anonymous http://ift.tt/1RpSir0
via IFTTT
I have a new follower on Twitter
David
140 characters of me and you'll wish you didn't know me.
Following: 6320 - Followers: 8135
March 25, 2016 at 09:30AM via Twitter http://twitter.com/David64227789
ISS Daily Summary Report – 03/24/16
from ISS On-Orbit Status Report http://ift.tt/1UNkJ89
via IFTTT
Anonymous has declared war on Isis (again)
from Google Alert - anonymous http://ift.tt/1oaXiaE
via IFTTT
The 7 Most Wanted Iranian Hackers By the FBI
from The Hacker News http://ift.tt/1MFZVYq
via IFTTT
Mac OS X Zero-Day Exploit Can Bypass Apple's Latest Protection Feature
from The Hacker News http://ift.tt/1Zz7Iie
via IFTTT
Anonymous targets Denver's Tattered Cover Book Store over city's handling of homeless
from Google Alert - anonymous http://ift.tt/1PuyuRk
via IFTTT
GMM-3 Mars Gravity Map
from NASA's Scientific Visualization Studio: Most Popular
via IFTTT
Hickson 91 in Piscis Austrinus
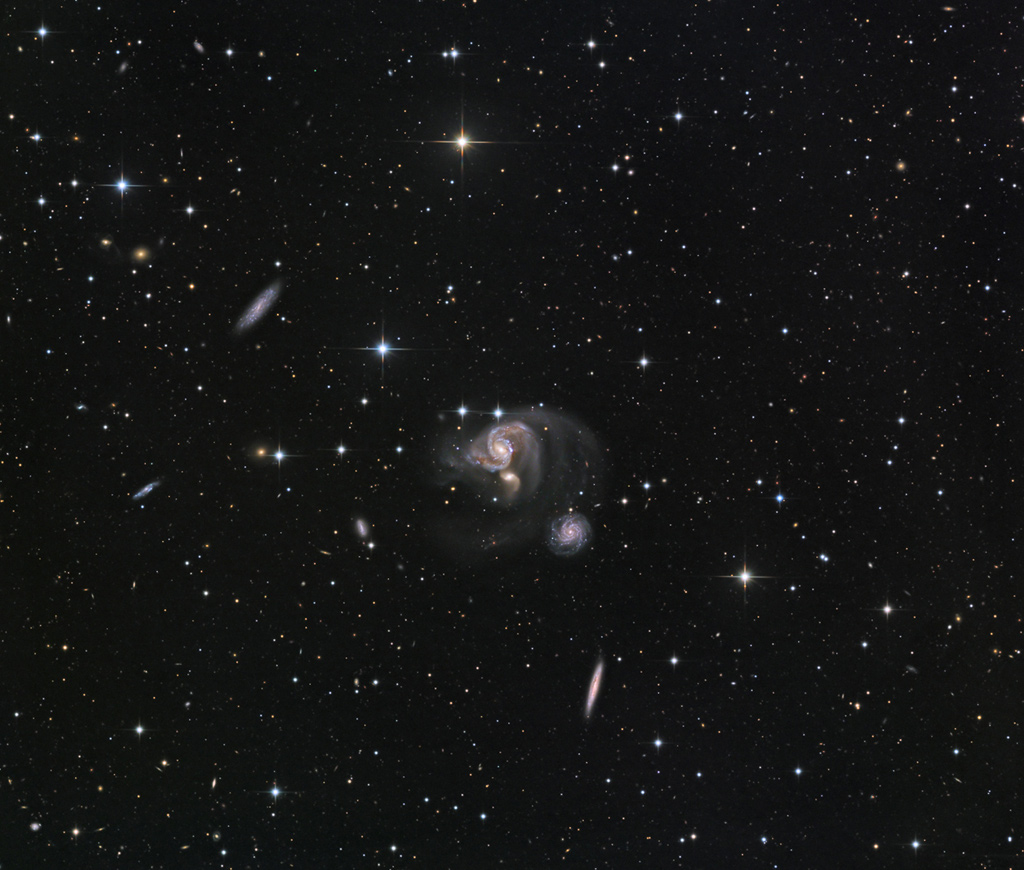
Thursday, March 24, 2016
I have a new follower on Twitter
SÔNIA MOSSRI
Brazilian journalist who left the mainstream media, writer, political economic risks analyst Against dictators, corporations, censhorship corruption.Pacifist.
New York City
https://t.co/GYh277Dn7S
Following: 8948 - Followers: 39224
March 24, 2016 at 10:04PM via Twitter http://twitter.com/soniamossri
A Diagram Is Worth A Dozen Images. (arXiv:1603.07396v1 [cs.CV])
Diagrams are common tools for representing complex concepts, relationships and events, often when it would be difficult to portray the same information with natural images. Understanding natural images has been extensively studied in computer vision, while diagram understanding has received little attention. In this paper, we study the problem of diagram interpretation and reasoning, the challenging task of identifying the structure of a diagram and the semantics of its constituents and their relationships. We introduce Diagram Parse Graphs (DPG) as our representation to model the structure of diagrams. We define syntactic parsing of diagrams as learning to infer DPGs for diagrams and study semantic interpretation and reasoning of diagrams in the context of diagram question answering. We devise an LSTM-based method for syntactic parsing of diagrams and introduce a DPG-based attention model for diagram question answering. We compile a new dataset of diagrams with exhaustive annotations of constituents and relationships for over 5,000 diagrams and 15,000 questions and answers. Our results show the significance of our models for syntactic parsing and question answering in diagrams using DPGs.
from cs.AI updates on arXiv.org http://ift.tt/1UPn4hN
via IFTTT
Load Disaggregation Based on Aided Linear Integer Programming. (arXiv:1603.07417v1 [cs.AI])
Load disaggregation based on aided linear integer programming (ALIP) is proposed. We start with a conventional linear integer programming (IP) based disaggregation and enhance it in several ways. The enhancements include additional constraints, correction based on a state diagram, median filtering, and linear programming-based refinement. With the aid of these enhancements, the performance of IP-based disaggregation is significantly improved. The proposed ALIP system relies only on the instantaneous load samples instead of waveform signatures, and hence does not crucially depend on high sampling frequency. Experimental results show that the proposed ALIP system performs better than the conventional IP-based load disaggregation system.
from cs.AI updates on arXiv.org http://ift.tt/1PudUQN
via IFTTT
Pixel-Level Domain Transfer. (arXiv:1603.07442v1 [cs.CV])
We present an image-conditional image generation model. The model transfers an input domain to a target domain in semantic level, and generates the target image in pixel level. To generate realistic target images, we employ the real/fake-discriminator in Generative Adversarial Nets, but also introduce a novel domain-discriminator to make the generated image relevant to the input image. We verify our model through a challenging task of generating a piece of clothing from an input image of a dressed person. We present a high quality clothing dataset containing the two domains, and succeed in demonstrating decent results.
from cs.AI updates on arXiv.org http://ift.tt/1UPn1CB
via IFTTT
An Expressive Probabilistic Temporal Logic. (arXiv:1603.07453v1 [cs.LO])
This paper argues that a combined treatment of probabilities, time and actions is essential for an appropriate logical account of the notion of probability; and, based on this intuition, describes an expressive probabilistic temporal logic for reasoning about actions with uncertain outcomes. The logic is modal and higher-order: modalities annotated by actions are used to express possibility and necessity of propositions in the next states resulting from the actions, and a higher-order function is needed to express the probability operator. The proposed logic is shown to be an adequate extension of classical mathematical probability theory, and its expressiveness is illustrated through the formalization of the Monty Hall problem.
from cs.AI updates on arXiv.org http://ift.tt/1PudREI
via IFTTT
Probabilistic Reasoning via Deep Learning: Neural Association Models. (arXiv:1603.07704v1 [cs.AI])
In this paper, we propose a new deep learning approach, called neural association model (NAM), for probabilistic reasoning in artificial intelligence. We propose to use neural networks to model association between any two events in a domain. Neural networks take one event as input and compute a conditional probability of the other event to model how likely these two events are associated. The actual meaning of the conditional probabilities varies between applications and depends on how the models are trained. In this work, as two case studies, we have investigated two NAM structures, namely deep neural networks (DNNs) and relation modulated neural nets (RMNNs), on several probabilistic reasoning tasks in AI, including recognizing textual entailment, triple classification in multirelational knowledge bases and common-sense reasoning. Experimental results on several popular data sets derived from WordNet, FreeBase and ConceptNet have all demonstrated that both DNNs and RMNNs perform equally well and they can significantly outperform the conventional methods available for these reasoning tasks. Moreover, comparing with DNNs, RMNNs are superior in knowledge transfer, where a pre-trained model can be quickly extended to an unseen relation after observing only a few training samples.
from cs.AI updates on arXiv.org http://ift.tt/1UPn4hD
via IFTTT
Low-Autocorrelation Binary Sequences: On Improved Merit Factors and Runtime Predictions to Achieve Them. (arXiv:1406.5301v5 [cs.DS] UPDATED)
The search for binary sequences with a high figure of merit, known as the low autocorrelation binary sequence ($labs$}) problem, represents a formidable computational challenge. To mitigate the computational constraints of the problem, we consider solvers that accept odd values of sequence length $L$ and return solutions for skew-symmetric binary sequences only -- with the consequence that not all best solutions under this constraint will be optimal for each $L$. In order to improve both, the search for best merit factor $and$ the asymptotic runtime performance, we instrumented three stochastic solvers, the first two are state-of-the-art solvers that rely on variants of memetic and tabu search ($lssMAts$ and $lssRRts$), the third solver ($lssOrel$) organizes the search as a sequence of independent contiguous self-avoiding walk segments. By adapting a rigorous statistical methodology to performance testing of all three combinatorial solvers, experiments show that the solver with the best asymptotic average-case performance, $lssOrel\_8 = 0.000032*1.1504^L$, has the best chance of finding solutions that improve, as $L$ increases, figures of merit reported to date. The same methodology can be applied to engineering new $labs$ solvers that may return merit factors even closer to the conjectured asymptotic value of 12.3248.
from cs.AI updates on arXiv.org http://ift.tt/1p7LbdX
via IFTTT
2 Composiciones para instrumento de teclado (Anonymous)
from Google Alert - anonymous http://ift.tt/1RBWObv
via IFTTT
Baby Lambs in UK Receive Knitted Jumpers from Anonymous Donor
from Google Alert - anonymous http://ift.tt/22ypkjl
via IFTTT
Microsoft's Artificial Intelligence Tay Became a 'Racist Nazi' in less than 24 Hours
from The Hacker News http://ift.tt/1WLWQvx
via IFTTT
McShay's Mock Draft 4.0: Ravens pick Oregon DE DeForest Buckner No. 6 overall; E. Kentucky DE Noah Spence in 2nd round (ESPN)
via IFTTT
I have a new follower on Twitter
Honest Hillary
Wall Street's Favorite Candidate™. I've been running for the White House for 30 damn years and it's my goddamn turn. NOT the real Hillary, because I'm honest.
Washington, DC
https://t.co/sPTs73MSz5
Following: 6835 - Followers: 6928
March 24, 2016 at 01:01PM via Twitter http://twitter.com/Honest_Hillary
I have a new follower on Twitter
App Discovery
All things #apps! Our featured app lets you create a profile that shares all of your favorite apps with your friends: https://t.co/yqxcNpiJTT
Los Angeles
https://t.co/yqxcNpiJTT
Following: 1539 - Followers: 571
March 24, 2016 at 01:01PM via Twitter http://twitter.com/AppDiscoveryNow
What is SMTP STS? How It improves Email Security for StartTLS?
from The Hacker News http://ift.tt/1RnRfKq
via IFTTT
ISS Daily Summary Report – 03/23/16
from ISS On-Orbit Status Report http://ift.tt/1pA5anh
via IFTTT
network-anonymous-i2p
from Google Alert - anonymous http://ift.tt/1VIdHkK
via IFTTT
Why Were You Anonymous? Why Did You Go Public?
from Google Alert - anonymous http://ift.tt/1MnhQYU
via IFTTT
The Great Nebula in Carina
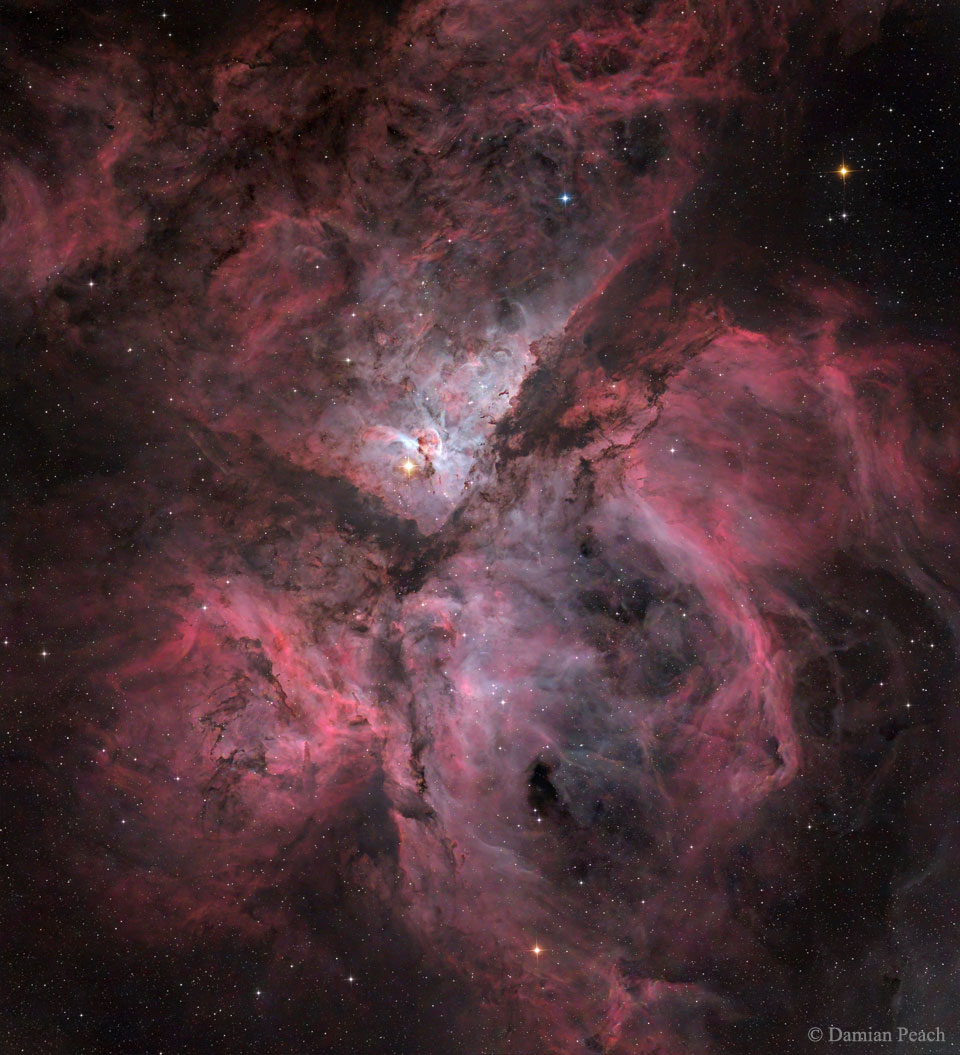
Wednesday, March 23, 2016
I have a new follower on Twitter
Natalie Lisowska
Teacher & Data Nerd with a passion for dogs, tomatoes and social justice. I'm an infographic enthusiast also.
Washington, DC
Following: 4513 - Followers: 5079
March 23, 2016 at 11:09PM via Twitter http://twitter.com/datamatos
Comparing Human and Automated Evaluation of Open-Ended Student Responses to Questions of Evolution. (arXiv:1603.07029v1 [cs.AI])
Written responses can provide a wealth of data in understanding student reasoning on a topic. Yet they are time- and labor-intensive to score, requiring many instructors to forego them except as limited parts of summative assessments at the end of a unit or course. Recent developments in Machine Learning (ML) have produced computational methods of scoring written responses for the presence or absence of specific concepts. Here, we compare the scores from one particular ML program -- EvoGrader -- to human scoring of responses to structurally- and content-similar questions that are distinct from the ones the program was trained on. We find that there is substantial inter-rater reliability between the human and ML scoring. However, sufficient systematic differences remain between the human and ML scoring that we advise only using the ML scoring for formative, rather than summative, assessment of student reasoning.
from cs.AI updates on arXiv.org http://ift.tt/22wS38d
via IFTTT
Cosolver2B: An Efficient Local Search Heuristic for the Travelling Thief Problem. (arXiv:1603.07051v1 [cs.AI])
Real-world problems are very difficult to optimize. However, many researchers have been solving benchmark problems that have been extensively investigated for the last decades even if they have very few direct applications. The Traveling Thief Problem (TTP) is a NP-hard optimization problem that aims to provide a more realistic model. TTP targets particularly routing problem under packing/loading constraints which can be found in supply chain management and transportation. In this paper, TTP is presented and formulated mathematically. A combined local search algorithm is proposed and compared with Random Local Search (RLS) and Evolutionary Algorithm (EA). The obtained results are quite promising since new better solutions were found.
from cs.AI updates on arXiv.org http://ift.tt/1XOsk4q
via IFTTT
Debugging Machine Learning Tasks. (arXiv:1603.07292v1 [cs.LG])
Unlike traditional programs (such as operating systems or word processors) which have large amounts of code, machine learning tasks use programs with relatively small amounts of code (written in machine learning libraries), but voluminous amounts of data. Just like developers of traditional programs debug errors in their code, developers of machine learning tasks debug and fix errors in their data. However, algorithms and tools for debugging and fixing errors in data are less common, when compared to their counterparts for detecting and fixing errors in code. In this paper, we consider classification tasks where errors in training data lead to misclassifications in test points, and propose an automated method to find the root causes of such misclassifications. Our root cause analysis is based on Pearl's theory of causation, and uses Pearl's PS (Probability of Sufficiency) as a scoring metric. Our implementation, Psi, encodes the computation of PS as a probabilistic program, and uses recent work on probabilistic programs and transformations on probabilistic programs (along with gray-box models of machine learning algorithms) to efficiently compute PS. Psi is able to identify root causes of data errors in interesting data sets.
from cs.AI updates on arXiv.org http://ift.tt/1UNxcHJ
via IFTTT
On the Theory and Practice of Privacy-Preserving Bayesian Data Analysis. (arXiv:1603.07294v1 [cs.LG])
Bayesian inference has great promise for the privacy-preserving analysis of sensitive data, as posterior sampling automatically preserves differential privacy, an algorithmic notion of data privacy, under certain conditions (Wang et al., 2015). While Wang et al. (2015)'s one posterior sample (OPS) approach elegantly provides privacy "for free," it is data inefficient in the sense of asymptotic relative efficiency (ARE). We show that a simple alternative based on the Laplace mechanism, the workhorse technique of differential privacy, is as asymptotically efficient as non-private posterior inference, under general assumptions. The Laplace mechanism has additional practical advantages including efficient use of the privacy budget for MCMC. We demonstrate the practicality of our approach on a time-series analysis of sensitive military records from the Afghanistan and Iraq wars disclosed by the Wikileaks organization.
from cs.AI updates on arXiv.org http://ift.tt/1SinXNv
via IFTTT
Mutual Information and Diverse Decoding Improve Neural Machine Translation. (arXiv:1601.00372v2 [cs.CL] UPDATED)
Sequence-to-sequence neural translation models learn semantic and syntactic relations between sentence pairs by optimizing the likelihood of the target given the source, i.e., $p(y|x)$, an objective that ignores other potentially useful sources of information. We introduce an alternative objective function for neural MT that maximizes the mutual information between the source and target sentences, modeling the bi-directional dependency of sources and targets. We implement the model with a simple re-ranking method, and also introduce a decoding algorithm that increases diversity in the N-best list produced by the first pass. Applied to the WMT German/English and French/English tasks, the proposed models offers a consistent performance boost on both standard LSTM and attention-based neural MT architectures.
from cs.AI updates on arXiv.org http://ift.tt/1mBTy1w
via IFTTT
[FD] APPLE-SA-2016-03-21-7 OS X Server 5.1
Source: Gmail -> IFTTT-> Blogger
[FD] APPLE-SA-2016-03-21-6 Safari 9.1
Source: Gmail -> IFTTT-> Blogger
[FD] APPLE-SA-2016-03-21-5 OS X El Capitan 10.11.4 and Security Update 2016-002
Source: Gmail -> IFTTT-> Blogger
[FD] APPLE-SA-2016-03-21-4 Xcode 7.3
Source: Gmail -> IFTTT-> Blogger
[FD] APPLE-SA-2016-03-21-2 watchOS 2.2
Source: Gmail -> IFTTT-> Blogger
Beautiful/Anonymous - The Illustrator
from Google Alert - anonymous http://ift.tt/1S6ZqZa
via IFTTT
I have a new follower on Twitter
Zesty Orioles News
Twitter's #1 #Orioles account! We constantly comb the web for the best and freshest Orioles articles so we can share them with our followers.
Baltimore, MD
https://t.co/wxinr5ui5N
Following: 461 - Followers: 16
March 23, 2016 at 02:50PM via Twitter http://twitter.com/zesty_orioles
Doesn't work for anonymous users
from Google Alert - anonymous http://ift.tt/1RlyDbW
via IFTTT
I have a new follower on Twitter
Joe and Jenelle
Joe & Jenelle Iuliucci - Keller Williams Realty - 888-980-9820 Southern Nevada & Southern California - Las Vegas, Henderson, Mission Viejo, Corona, Yorba Linda
So.Nevada & So.California
http://t.co/8CVmHDP5tz
Following: 5569 - Followers: 7702
March 23, 2016 at 02:44PM via Twitter http://twitter.com/vegasreo
Israeli Forensic Firm 'Cellebrite' is Helping FBI to Unlock Terrorist's iPhone
from The Hacker News http://ift.tt/1pyW5uT
via IFTTT
Orioles: 3B Manny Machado No. 18 in Baseball Tonight's ranking of MLB's top 100 players; jumped from last year's No. 59 (ESPN)
via IFTTT
Warning! Think Twice Before Using USB Drives
from The Hacker News http://ift.tt/1VF77eA
via IFTTT
Badlock — Unpatched Windows-Samba Vulnerability Affects All Versions of Windows
from The Hacker News http://ift.tt/1UkEwfA
via IFTTT
Orioles: RP Zach Phillips agrees to 1-year deal after declining re-assignment by White Sox last week; 19 career games (ESPN)
via IFTTT
[FD] Facebook Messenger (iOS) Certificate Validation Vulnerability
Source: Gmail -> IFTTT-> Blogger
[FD] Remote Code Execution in DVR affecting over 70 different vendors
Source: Gmail -> IFTTT-> Blogger
[FD] HTTPS Only 3.1 (Detailed Analysis, Browser Security, Open Source, Python)
Source: Gmail -> IFTTT-> Blogger
FBI Most Wanted — Three 'Syrian Electronic Army' Hackers Charged for Cyber Crime
from The Hacker News http://ift.tt/1Rjz48E
via IFTTT
The Mars Fleet
from NASA's Scientific Visualization Studio: Most Recent Items http://ift.tt/1RfmkBo
via IFTTT
GMM-3 Mars Gravity Map
from NASA's Scientific Visualization Studio: Most Recent Items http://ift.tt/1WHcRCT
via IFTTT
Rainbow Airglow over the Azores
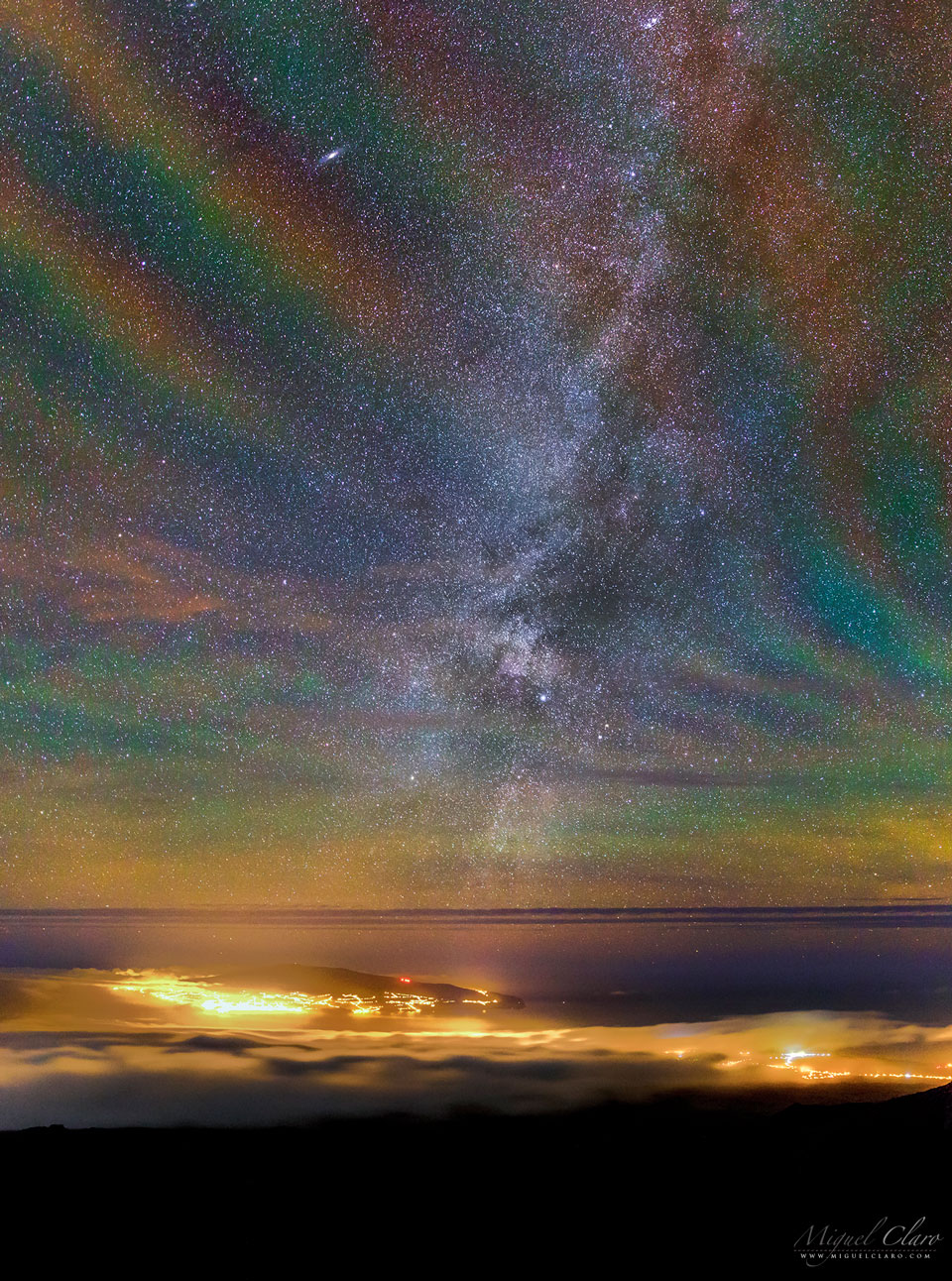
Tuesday, March 22, 2016
Anonymous user 83967f
from Google Alert - anonymous http://ift.tt/1U7a5Jg
via IFTTT
I have a new follower on Twitter
Luca Sadurny
Managing Partner at @mosalingua / Polyglot / Growth Hacker. I tweet about #languagelearning, #apps #webmarketing #mobilemarketing, #SEO #growthhacking...
Lyon, Francia
https://t.co/eZ00WIq2nX
Following: 2881 - Followers: 3214
March 22, 2016 at 10:48PM via Twitter http://twitter.com/lucasadurny
ISS Daily Summary Report – 03/22/16
from ISS On-Orbit Status Report http://ift.tt/1SgBFjQ
via IFTTT
http://www.workaholics-anonymous.org/http://ift.tt/1WGTbPw ...
from Google Alert - anonymous http://ift.tt/1WGT9Y1
via IFTTT
Learning Executable Semantic Parsers for Natural Language Understanding. (arXiv:1603.06677v1 [cs.CL])
For building question answering systems and natural language interfaces, semantic parsing has emerged as an important and powerful paradigm. Semantic parsers map natural language into logical forms, the classic representation for many important linguistic phenomena. The modern twist is that we are interested in learning semantic parsers from data, which introduces a new layer of statistical and computational issues. This article lays out the components of a statistical semantic parser, highlighting the key challenges. We will see that semantic parsing is a rich fusion of the logical and the statistical world, and that this fusion will play an integral role in the future of natural language understanding systems.
from cs.AI updates on arXiv.org http://ift.tt/1Sgzrku
via IFTTT
Generating Factoid Questions With Recurrent Neural Networks: The 30M Factoid Question-Answer Corpus. (arXiv:1603.06807v1 [cs.CL])
Over the past decade, large-scale supervised learning corpora have enabled machine learning researchers to make substantial advances. However, to this date, there are no large-scale question-answer corpora available. In this paper we present the 30M Factoid Question-Answer Corpus, an enormous question-answer pair corpus produced by applying a novel neural network architecture on the knowledge base Freebase to transduce facts into natural language questions. The produced question-answer pairs are evaluated both by human evaluators and using automatic evaluation metrics, including well-established machine translation and sentence similarity metrics. Across all evaluation criteria the question-generation model outperforms the competing template-based baseline. Furthermore, when presented to human evaluators, the generated questions appear to be indistinguishable from real human-generated questions.
from cs.AI updates on arXiv.org http://ift.tt/1Ujqzi0
via IFTTT
Feeling the Bern: Adaptive Estimators for Bernoulli Probabilities of Pairwise Comparisons. (arXiv:1603.06881v1 [cs.LG])
We study methods for aggregating pairwise comparison data in order to estimate outcome probabilities for future comparisons among a collection of n items. Working within a flexible framework that imposes only a form of strong stochastic transitivity (SST), we introduce an adaptivity index defined by the indifference sets of the pairwise comparison probabilities. In addition to measuring the usual worst-case risk of an estimator, this adaptivity index also captures the extent to which the estimator adapts to instance-specific difficulty relative to an oracle estimator. We prove three main results that involve this adaptivity index and different algorithms. First, we propose a three-step estimator termed Count-Randomize-Least squares (CRL), and show that it has adaptivity index upper bounded as $\sqrt{n}$ up to logarithmic factors. We then show that that conditional on the hardness of planted clique, no computationally efficient estimator can achieve an adaptivity index smaller than $\sqrt{n}$. Second, we show that a regularized least squares estimator can achieve a poly-logarithmic adaptivity index, thereby demonstrating a $\sqrt{n}$-gap between optimal and computationally achievable adaptivity. Finally, we prove that the standard least squares estimator, which is known to be optimally adaptive in several closely related problems, fails to adapt in the context of estimating pairwise probabilities.
from cs.AI updates on arXiv.org http://ift.tt/1Sgztcf
via IFTTT
Ravens: Owner Steve Bisciotti to charter plane from Baltimore to Florida so team can attend CB Tray Walker's funeral (ESPN)
via IFTTT
How to set a different frontpage to user/login for anonymous users
from Google Alert - anonymous http://ift.tt/1Uiclhg
via IFTTT
NFL: Ravens coach John Harbaugh says team has \"unofficial agreement\" with RB Trent Richardson; No. 3 overall pick 2012 (ESPN)
via IFTTT
ISS Daily Summary Report – 03/21/16
from ISS On-Orbit Status Report http://ift.tt/1S37N86
via IFTTT
FBI may have found a New Way to Unlock Shooter's iPhone without Apple
from The Hacker News http://ift.tt/1Poyged
via IFTTT
Anonymous user 32e954
from Google Alert - anonymous http://ift.tt/1T4ZtcQ
via IFTTT
[FD] [RT-SA-2016-002] Cross-site Scripting in Securimage 3.6.2
Source: Gmail -> IFTTT-> Blogger
Who Viewed Your Profile on Instagram? Obviously, Hackers!
from The Hacker News http://ift.tt/1ZncTS8
via IFTTT
Alaskan Moondogs
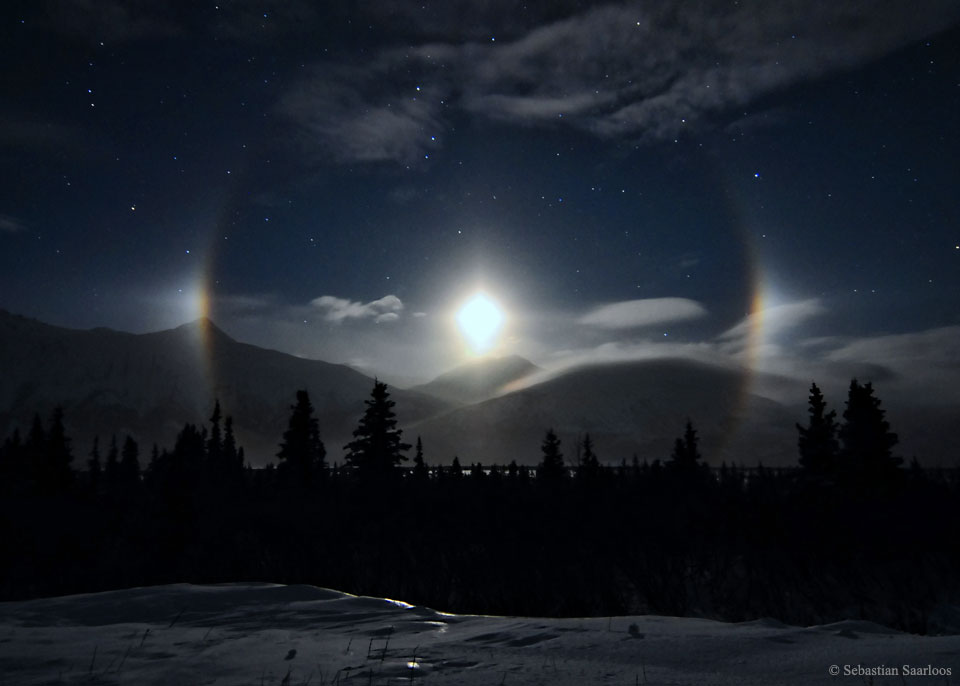
Monday, March 21, 2016
How to get more items in game Disney Magic Kingdoms
from Google Alert - anonymous http://ift.tt/1RcbKek
via IFTTT
I have a new follower on Twitter
Saasabi
SaaS BI & analytics solutions. OLAP engine on Microsoft Azure. We enable organizations to make better, data-driven decisions.
Sydney, Australia
http://t.co/8AMg9vQEef
Following: 1139 - Followers: 1352
March 21, 2016 at 10:27PM via Twitter http://twitter.com/Saasabi
I have a new follower on Twitter
Phil Kowalski
Excel Lover, Father, Husband, Teacher - not in this order maybe
bayern
https://t.co/bLbfzkqDtp
Following: 2257 - Followers: 2273
March 21, 2016 at 10:19PM via Twitter http://twitter.com/ExcelBlogDOTNet
A Comprehensive Performance Evaluation of Deformable Face Tracking "In-the-Wild". (arXiv:1603.06015v1 [cs.CV])
Recently, technologies such as face detection, facial landmark localisation and face recognition and verification have matured enough to provide effective and efficient solutions for imagery captured under arbitrary conditions (referred to as "in-the-wild"). This is partially attributed to the fact that comprehensive "in-the-wild" benchmarks have been developed for face detection, landmark localisation and recognition/verification. A very important technology that has not been thoroughly evaluated yet is deformable face tracking "in-the-wild". Until now, the performance has mainly been assessed qualitatively by visually assessing the result of a deformable face tracking technology on short videos. In this paper, we perform the first, to the best of our knowledge, thorough evaluation of state-of-the-art deformable face tracking pipelines using the recently introduced 300VW benchmark. We evaluate many different architectures focusing mainly on the task of on-line deformable face tracking. In particular, we compare the following general strategies: (a) generic face detection plus generic facial landmark localisation, (b) generic model free tracking plus generic facial landmark localisation, as well as (c) hybrid approaches using state-of-the-art face detection, model free tracking and facial landmark localisation technologies. Our evaluation reveals future avenues for further research on the topic.
from cs.AI updates on arXiv.org http://ift.tt/1XIClju
via IFTTT
The Computational Power of Dynamic Bayesian Networks. (arXiv:1603.06125v1 [cs.AI])
This paper considers the computational power of constant size, dynamic Bayesian networks. Although discrete dynamic Bayesian networks are no more powerful than hidden Markov models, dynamic Bayesian networks with continuous random variables and discrete children of continuous parents are capable of performing Turing-complete computation. With modified versions of existing algorithms for belief propagation, such a simulation can be carried out in real time. This result suggests that dynamic Bayesian networks may be more powerful than previously considered. Relationships to causal models and recurrent neural networks are also discussed.
from cs.AI updates on arXiv.org http://ift.tt/1Rgxjac
via IFTTT
Sentence Pair Scoring: Towards Unified Framework for Text Comprehension. (arXiv:1603.06127v1 [cs.CL])
We review the task of Sentence Pair Scoring, popular in the literature in various forms --- slanted as Answer Sentence Selection, Paraphrasing, Semantic Text Scoring, Next Utterance Ranking, Recognizing Textual Entailment or e.g. a component of Memory Networks.
We argue that such tasks are similar from the model perspective (especially in the context of high-capacity deep neural models) and propose new baselines by comparing the performance of popular convolutional, recurrent and attention-based neural models across many Sentence Pair Scoring tasks and datasets. We discuss the problem of evaluating randomized models, propose a statistically grounded methodology, and attempt to improve comparisons by releasing new datasets that are much harder than some of the currently used well explored benchmarks.
To address the current research fragmentation in a future-proof way, we introduce a unified open source software framework with easily pluggable models, allowing easy evaluation on a wide range of semantic natural language tasks. This allows us to outline a path towards a universal machine learned semantic model for machine reading tasks. We support this plan by experiments that demonstrate reusability of models trained on different tasks, even across corpora of very different nature.
from cs.AI updates on arXiv.org http://ift.tt/1S1WM78
via IFTTT
Automated Correction for Syntax Errors in Programming Assignments using Recurrent Neural Networks. (arXiv:1603.06129v1 [cs.PL])
We present a method for automatically generating repair feedback for syntax errors for introductory programming problems. Syntax errors constitute one of the largest classes of errors (34%) in our dataset of student submissions obtained from a MOOC course on edX. The previous techniques for generating automated feed- back on programming assignments have focused on functional correctness and style considerations of student programs. These techniques analyze the program AST of the program and then perform some dynamic and symbolic analyses to compute repair feedback. Unfortunately, it is not possible to generate ASTs for student pro- grams with syntax errors and therefore the previous feedback techniques are not applicable in repairing syntax errors.
We present a technique for providing feedback on syntax errors that uses Recurrent neural networks (RNNs) to model syntactically valid token sequences. Our approach is inspired from the recent work on learning language models from Big Code (large code corpus). For a given programming assignment, we first learn an RNN to model all valid token sequences using the set of syntactically correct student submissions. Then, for a student submission with syntax errors, we query the learnt RNN model with the prefix to- ken sequence to predict token sequences that can fix the error by either replacing or inserting the predicted token sequence at the error location. We evaluate our technique on over 14, 000 student submissions with syntax errors. Our technique can completely re- pair 31.69% (4501/14203) of submissions with syntax errors and in addition partially correct 6.39% (908/14203) of the submissions.
from cs.AI updates on arXiv.org http://ift.tt/1pwfsEW
via IFTTT
Evolving Shepherding Behavior with Genetic Programming Algorithms. (arXiv:1603.06141v1 [cs.AI])
We apply genetic programming techniques to the `shepherding' problem, in which a group of one type of animal (sheep dogs) attempts to control the movements of a second group of animals (sheep) obeying flocking behavior. Our genetic programming algorithm evolves an expression tree that governs the movements of each dog. The operands of the tree are hand-selected features of the simulation environment that may allow the dogs to herd the sheep effectively. The algorithm uses tournament-style selection, crossover reproduction, and a point mutation. We find that the evolved solutions generalize well and outperform a (naive) human-designed algorithm.
from cs.AI updates on arXiv.org http://ift.tt/1pGutop
via IFTTT
Neurally-Guided Procedural Models: Learning to Guide Procedural Models with Deep Neural Networks. (arXiv:1603.06143v1 [cs.GR])
We present a deep learning approach for speeding up constrained procedural modeling. Probabilistic inference algorithms such as Sequential Monte Carlo (SMC) provide powerful tools for constraining procedural models, but they require many samples to produce desirable results. In this paper, we show how to create procedural models which learn how to satisfy constraints. We augment procedural models with neural networks: these networks control how the model makes random choices based on what output it has generated thus far. We call such a model a neurally-guided procedural model. As a pre-computation, we train these models on constraint-satisfying example outputs generated via SMC. They are then used as efficient importance samplers for SMC, generating high-quality results with very few samples. We evaluate our method on L-system-like models with image-based constraints. Given a desired quality threshold, neurally-guided models can generate satisfactory results up to 10x faster than unguided models.
from cs.AI updates on arXiv.org http://ift.tt/25h2bkt
via IFTTT
Evaluation of a Tree-based Pipeline Optimization Tool for Automating Data Science. (arXiv:1603.06212v1 [cs.NE])
As the field of data science continues to grow, there will be an ever-increasing demand for tools that make machine learning accessible to non-experts. In this paper, we introduce the concept of tree-based pipeline optimization for automating one of the most tedious parts of machine learning---pipeline design. We implement an open source Tree-based Pipeline Optimization Tool (TPOT) in Python and demonstrate its effectiveness on a series of simulated and real-world benchmark data sets. In particular, we show that TPOT can design machine learning pipelines that provide a significant improvement over a basic machine learning analysis while requiring little to no input nor prior knowledge from the user. We also address the tendency for TPOT to design overly complex pipelines by integrating Pareto optimization, which produces compact pipelines without sacrificing classification accuracy. As such, this work represents an important step toward fully automating machine learning pipeline design.
from cs.AI updates on arXiv.org http://ift.tt/1pGutoj
via IFTTT
An Approximation Approach for Solving the Subpath Planning Problem. (arXiv:1603.06217v1 [cs.RO])
The subpath planning problem is a branch of the path planning problem, which has widespread applications in automated manufacturing process as well as vehicle and robot navigation. This problem is to find the shortest path or tour subject for travelling a set of given subpaths. The current approaches for dealing with the subpath planning problem are all based on meta-heuristic approaches. It is well-known that meta-heuristic based approaches have several deficiencies. To address them, we propose a novel approximation algorithm in the O(n^3) time complexity class, which guarantees to solve any subpath planning problem instance with the fixed ratio bound of 2. Also, the formal proofs of the claims, our empirical evaluation shows that our approximation method acts much better than a state-of-the-art method, both in result and execution time.
from cs.AI updates on arXiv.org http://ift.tt/1UzVVjP
via IFTTT
Multi-fidelity Gaussian Process Bandit Optimisation. (arXiv:1603.06288v1 [stat.ML])
In many scientific and engineering applications, we are tasked with the optimisation of an expensive to evaluate black box function $f$. Traditional methods for this problem assume just the availability of this single function. However, in many cases, cheap approximations to $f$ may be obtainable. For example, the expensive real world behaviour of a robot can be approximated by a cheap computer simulation. We can use these approximations to eliminate low function value regions and use the expensive evaluations to $f$ in a small promising region and speedily identify the optimum. We formalise this task as a \emph{multi-fidelity} bandit problem where the target function and its approximations are sampled from a Gaussian process. We develop a method based on upper confidence bound techniques and prove that it exhibits precisely the above behaviour, hence achieving better regret than strategies which ignore multi-fidelity information. Our method outperforms such naive strategies on several synthetic and real experiments.
from cs.AI updates on arXiv.org http://ift.tt/1VxnLgg
via IFTTT
Harnessing Deep Neural Networks with Logic Rules. (arXiv:1603.06318v1 [cs.LG])
Combining deep neural networks with structured logic rules is desirable to harness flexibility and reduce unpredictability of the neural models. We propose a general framework capable of enhancing various types of neural networks (e.g., CNNs and RNNs) with declarative first-order logic rules. Specifically, we develop an iterative distillation method that transfers the structured information of logic rules into the weights of neural networks. We deploy the framework on a CNN for sentiment analysis, and an RNN for named entity recognition. With a few highly intuitive rules, we obtain substantial improvements and achieve state-of-the-art or comparable results to previous best-performing systems.
from cs.AI updates on arXiv.org http://ift.tt/1pwfpJk
via IFTTT
Incorporating Copying Mechanism in Sequence-to-Sequence Learning. (arXiv:1603.06393v1 [cs.CL])
We address an important problem in sequence-to-sequence (Seq2Seq) learning referred to as copying, in which certain segments in the input sequence are selectively replicated in the output sequence. A similar phenomenon is observable in human language communication. For example, humans tend to repeat entity names or even long phrases in conversation. The challenge with regard to copying in Seq2Seq is that new machinery is needed to decide when to perform the operation. In this paper, we incorporate copying into neural network-based Seq2Seq learning and propose a new model called CopyNet with encoder-decoder structure. CopyNet can nicely integrate the regular way of word generation in the decoder with the new copying mechanism which can choose sub-sequences in the input sequence and put them at proper places in the output sequence. Our empirical study on both synthetic data sets and real world data sets demonstrates the efficacy of CopyNet. For example, CopyNet can outperform regular RNN-based model with remarkable margins on text summarization tasks.
from cs.AI updates on arXiv.org http://ift.tt/1S1WM6Q
via IFTTT
Characterization of neighborhood behaviours in a multi-neighborhood local search algorithm. (arXiv:1603.06459v1 [cs.AI])
We consider a multi-neighborhood local search algorithm with a large number of possible neighborhoods. Each neighborhood is accompanied by a weight value which represents the probability of being chosen at each iteration. These weights are fixed before the algorithm runs, and are considered as parameters of the algorithm. Given a set of instances, off-line tuning of the algorithm's parameters can be done by automated algorithm configuration tools (e.g., SMAC). However, the large number of neighborhoods can make the tuning expensive and difficult even when the number of parameters has been reduced by some intuition. In this work, we propose a systematic method to characterize each neighborhood's behaviours, representing them as a feature vector, and using cluster analysis to form similar groups of neighborhoods. The novelty of our characterization method is the ability of reflecting changes of behaviours according to hardness of different solution quality regions. We show that using neighborhood clusters instead of individual neighborhoods helps to reduce the parameter configuration space without misleading the search of the tuning procedure. Moreover, this method is problem-independent and potentially can be applied in similar contexts.
from cs.AI updates on arXiv.org http://ift.tt/1Rgxh2b
via IFTTT
A System for Probabilistic Linking of Thesauri and Classification Systems. (arXiv:1603.06485v1 [cs.AI])
This paper presents a system which creates and visualizes probabilistic semantic links between concepts in a thesaurus and classes in a classification system. For creating the links, we build on the Polylingual Labeled Topic Model (PLL-TM). PLL-TM identifies probable thesaurus descriptors for each class in the classification system by using information from the natural language text of documents, their assigned thesaurus descriptors and their designated classes. The links are then presented to users of the system in an interactive visualization, providing them with an automatically generated overview of the relations between the thesaurus and the classification system.
from cs.AI updates on arXiv.org http://ift.tt/25h2cow
via IFTTT