Latest YouTube Video
Saturday, August 20, 2016
Orioles: C Matt Wieters placed on paternity leave list; P Odrisamer Despaigne, C Francisco Pena recalled from Triple-A (ESPN)
via IFTTT
I have a new follower on Twitter
Java Next Generation
Sourcing Java Talent for exclusive roles in Dublin, Ireland the Tech Hub of Europe. Positions Recruited by @NextGenRecruit
Dublin City, Ireland
https://t.co/xmhgEvXNej
Following: 1815 - Followers: 2861
August 20, 2016 at 12:49PM via Twitter http://twitter.com/java_ng
Does your WebCam Crash after Windows 10 Anniversary Update? Here’s How to Fix It
from The Hacker News http://ift.tt/2btw9Pm
via IFTTT
Emotions Anonymous
from Google Alert - anonymous http://ift.tt/2b67eCg
via IFTTT
Leaked Exploits are Legit and Belong to NSA: Cisco, Fortinet and Snowden Docs Confirm
from The Hacker News http://ift.tt/2bt1jX6
via IFTTT
[FD] Path traversal vulnerability in WordPress Core Ajax handlers
Source: Gmail -> IFTTT-> Blogger
Lambda (anonymous/first class procedures) and custom reporters
from Google Alert - anonymous http://ift.tt/2bEG81u
via IFTTT
Perseid Fireball at Sunset Crater
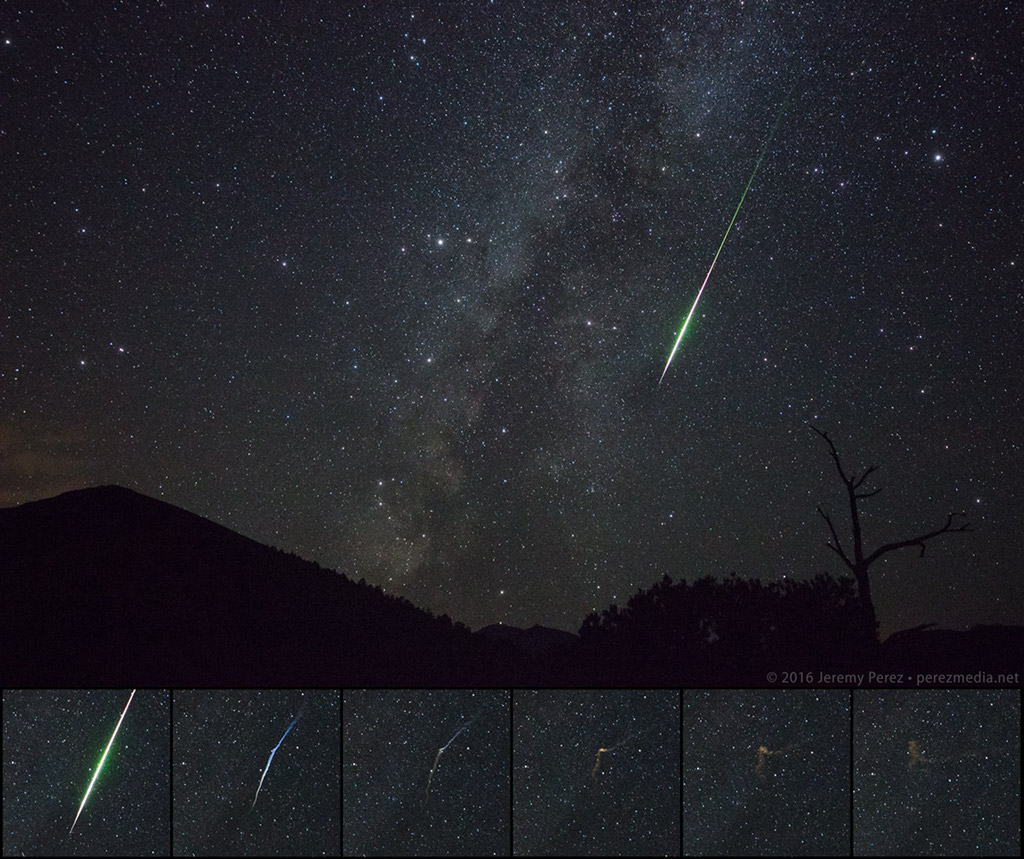
Study Domain for the Arctic-Boreal Vulnerability Experiment
from NASA's Scientific Visualization Studio: Most Recent Items http://ift.tt/2boh0N9
via IFTTT
Arctic Sea Ice from March to August 2016
from NASA's Scientific Visualization Studio: Most Recent Items http://ift.tt/2b4CAYS
via IFTTT
Friday, August 19, 2016
Guest checkout card is saved and exposed to any other anonymous users
from Google Alert - anonymous http://ift.tt/2bAvd86
via IFTTT
[FD] Onapsis Security Advisory ONAPSIS-2016-038: SAP HANA Information disclosure in EXPORT
Source: Gmail -> IFTTT-> Blogger
[FD] Onapsis Security Advisory ONAPSIS-2016-040: SAP HANA potential wrong encryption
Source: Gmail -> IFTTT-> Blogger
[FD] Onapsis Security Advisory ONAPSIS-2016-037: SAP HANA Potential Remote Code Execution
Source: Gmail -> IFTTT-> Blogger
[FD] Onapsis Security Advisory ONAPSIS-2016-034: SAP TREX remote command execution
Source: Gmail -> IFTTT-> Blogger
[FD] Onapsis Security Advisory ONAPSIS-2016-033: SAP TREX TNS Information Disclosure in NameServer
Source: Gmail -> IFTTT-> Blogger
[FD] Onapsis Security Advisory ONAPSIS-2016-027: SAP HANA User information disclosure
Source: Gmail -> IFTTT-> Blogger
Ravens: Terrell Suggs cut out fried chicken, pizza, gefilte fish to get into best shape of his career - Jamison Hensley (ESPN)
via IFTTT
[FD] Onapsis Security Advisory ONAPSIS-2016-026: SAP HANA SYSTEM user brute force attack
Source: Gmail -> IFTTT-> Blogger
[FD] Onapsis Security Advisory ONAPSIS-2016-024: SAP HANA arbitrary audit injection via HTTP requests
Source: Gmail -> IFTTT-> Blogger
[FD] Onapsis Security Advisory ONAPSIS-2016-025: SAP HANA arbitrary audit injection via SQL protocol
Source: Gmail -> IFTTT-> Blogger
[FD] Onapsis Security Advisory ONAPSIS-2016-022: SAP TREX Arbitrary file write
Source: Gmail -> IFTTT-> Blogger
[FD] Onapsis Security Advisory ONAPSIS-2016-021: SAP TREX Remote file read
Source: Gmail -> IFTTT-> Blogger
[FD] Onapsis Security Advisory ONAPSIS-2016-020: SAP TREX Remote Directory Traversal
Source: Gmail -> IFTTT-> Blogger
[FD] Onapsis Security Advisory ONAPSIS-2016-019: SAP TREX Remote Command Execution
Source: Gmail -> IFTTT-> Blogger
[FD] Onapsis Security Advisory ONAPSIS-2016-007: SAP HANA Password Disclosure
Source: Gmail -> IFTTT-> Blogger
ISS Daily Summary Report – 08/18/2016
from ISS On-Orbit Status Report http://ift.tt/2blKdrH
via IFTTT
Warning — Bitcoin Users Could Be Targeted by State-Sponsored Hackers
from The Hacker News http://ift.tt/2bn55g9
via IFTTT
Omegle, the Popular 'Chat with Strangers' Service Leaks Your Dirty Chats and Personal Info
from The Hacker News http://ift.tt/2b1RmiJ
via IFTTT
Perseid Night at Yosemite
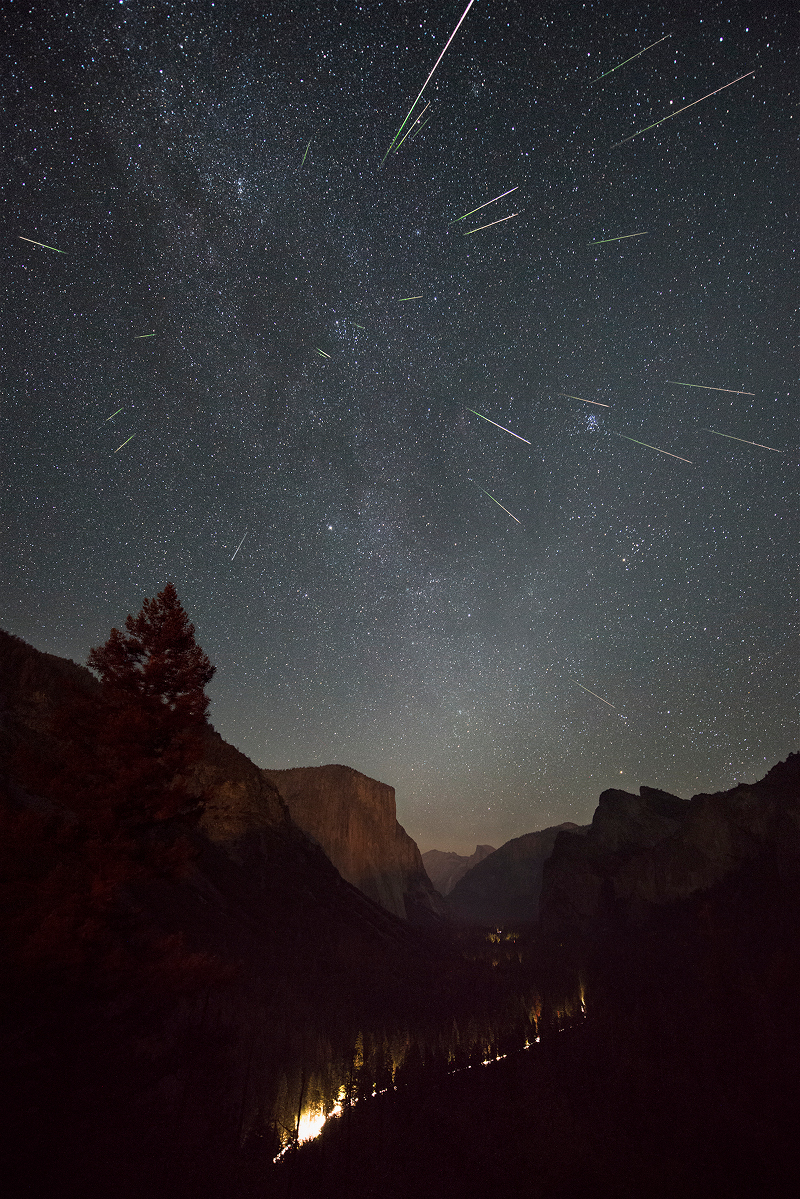
How to hack Online Head Ball with latest cheat tool in ipad mini
from Google Alert - anonymous http://ift.tt/2bxHpq9
via IFTTT
Prompt Electron Acceleration in the Radiation Belts
from NASA's Scientific Visualization Studio: Most Recent Items http://ift.tt/2bkfA68
via IFTTT
Thursday, August 18, 2016
Orioles Video: Manny Machado and Chris Davis slug back-to-back home runs in the 6th inning of 13-5 blowout vs. Astros (ESPN)
via IFTTT
I have a new follower on Twitter
Benny V
Going to save this world & take us to another, watch. |#Engineer |#Developer |#Science, #Tech, #Culture, & #Data lover | Web & App Developer at BluePrint
Atlanta, GA
https://t.co/Dmj83J7nvl
Following: 1734 - Followers: 12511
August 18, 2016 at 09:39PM via Twitter http://twitter.com/OyeBenny
Anonymous donor gives money to library in honor of late Harlan Co. teen
from Google Alert - anonymous http://ift.tt/2b5rw9h
via IFTTT
Effective Multi-step Temporal-Difference Learning for Non-Linear Function Approximation. (arXiv:1608.05151v1 [cs.AI])
Multi-step temporal-difference (TD) learning, where the update targets contain information from multiple time steps ahead, is one of the most popular forms of TD learning for linear function approximation. The reason is that multi-step methods often yield substantially better performance than their single-step counter-parts, due to a lower bias of the update targets. For non-linear function approximation, however, single-step methods appear to be the norm. Part of the reason could be that on many domains the popular multi-step methods TD($\lambda$) and Sarsa($\lambda$) do not perform well when combined with non-linear function approximation. In particular, they are very susceptible to divergence of value estimates. In this paper, we identify the reason behind this. Furthermore, based on our analysis, we propose a new multi-step TD method for non-linear function approximation that addresses this issue. We confirm the effectiveness of our method using two benchmark tasks with neural networks as function approximation.
from cs.AI updates on arXiv.org http://ift.tt/2bjNhEW
via IFTTT
Accelerating Exact and Approximate Inference for (Distributed) Discrete Optimization with GPUs. (arXiv:1608.05288v1 [cs.AI])
Discrete optimization is a central problem in artificial intelligence. The optimization of the aggregated cost of a network of cost functions arises in a variety of problems including (W)CSP, DCOP, as well as optimization in stochastic variants such as Bayesian networks. Inference-based algorithms are powerful techniques for solving discrete optimization problems, which can be used independently or in combination with other techniques. However, their applicability is often limited by their compute intensive nature and their space requirements. This paper proposes the design and implementation of a novel inference-based technique, which exploits modern massively parallel architectures, such as those found in Graphical Processing Units (GPUs), to speed up the resolution of exact and approximated inference-based algorithms for discrete optimization. The paper studies the proposed algorithm in both centralized and distributed optimization contexts. The paper demonstrates that the use of GPUs provides significant advantages in terms of runtime and scalability, achieving up to two orders of magnitude in speedups and showing a considerable reduction in execution time (up to 345 times faster) with respect to a sequential version.
from cs.AI updates on arXiv.org http://ift.tt/2bN5yh6
via IFTTT
Probabilistic Data Analysis with Probabilistic Programming. (arXiv:1608.05347v1 [cs.AI])
Probabilistic techniques are central to data analysis, but different approaches can be difficult to apply, combine, and compare. This paper introduces composable generative population models (CGPMs), a computational abstraction that extends directed graphical models and can be used to describe and compose a broad class of probabilistic data analysis techniques. Examples include hierarchical Bayesian models, multivariate kernel methods, discriminative machine learning, clustering algorithms, dimensionality reduction, and arbitrary probabilistic programs. We also demonstrate the integration of CGPMs into BayesDB, a probabilistic programming platform that can express data analysis tasks using a modeling language and a structured query language. The practical value is illustrated in two ways. First, CGPMs are used in an analysis that identifies satellite data records which probably violate Kepler's Third Law, by composing causal probabilistic programs with non-parametric Bayes in under 50 lines of probabilistic code. Second, for several representative data analysis tasks, we report on lines of code and accuracy measurements of various CGPMs, plus comparisons with standard baseline solutions from Python and MATLAB libraries.
from cs.AI updates on arXiv.org http://ift.tt/2bjNbNF
via IFTTT
On the expressive power of deep neural networks. (arXiv:1606.05336v3 [stat.ML] UPDATED)
We study the effects of the depth and width of a neural network on its expressive power. Precise theoretical and experimental results are derived in the generic setting of neural networks after random initialization. We find that three different measures of functional expressivity: number of transitions (a measure of non-linearity/complexity), network activation patterns (a new definition with an intrinsic link to hyperplane arrangements in input space) and number of dichotomies, show an exponential dependence on depth but not width. These three measures are related to each other, and, are also directly proportional to a fourth quantity, trajectory length. Most crucially, we show, both theoretically and experimentally, that trajectory length grows exponentially with depth, which is why all three measures display an exponential dependence on depth.
These results also suggest that parameters earlier in the network have greater influence over the expressive power of the network. So for any layer, its influence on expressivity is determined by the remaining depth of the network after that layer, which is supported by experiments on fully connected and convolutional networks on MNIST and CIFAR-10.
from cs.AI updates on arXiv.org http://ift.tt/1tznj7m
via IFTTT
A Convolutional Autoencoder for Multi-Subject fMRI Data Aggregation. (arXiv:1608.04846v1 [stat.ML])
Finding the most effective way to aggregate multi-subject fMRI data is a long-standing and challenging problem. It is of increasing interest in contemporary fMRI studies of human cognition due to the scarcity of data per subject and the variability of brain anatomy and functional response across subjects. Recent work on latent factor models shows promising results in this task but this approach does not preserve spatial locality in the brain. We examine two ways to combine the ideas of a factor model and a searchlight based analysis to aggregate multi-subject fMRI data while preserving spatial locality. We first do this directly by combining a recent factor method known as a shared response model with searchlight analysis. Then we design a multi-view convolutional autoencoder for the same task. Both approaches preserve spatial locality and have competitive or better performance compared with standard searchlight analysis and the shared response model applied across the whole brain. We also report a system design to handle the computational challenge of training the convolutional autoencoder.
from cs.AI updates on arXiv.org http://ift.tt/2b2i1L3
via IFTTT
[FD] Onapsis Security Advisory ONAPSIS-2016-006: SAP HANA Get Topology Information
Source: Gmail -> IFTTT-> Blogger
Microsoft Open Sources PowerShell; Now Available for Linux and Mac OS X
from The Hacker News http://ift.tt/2b262Q6
via IFTTT
I have a new follower on Twitter
AthelstanSearch
Analytics Talent in FinTech. #Analytics #FinTech #Athelstan
London
https://t.co/zMFXpNe9Qp
Following: 2456 - Followers: 827
August 18, 2016 at 09:25AM via Twitter http://twitter.com/AthelstanSearch
ISS Daily Summary Report – 08/17/2016
from ISS On-Orbit Status Report http://ift.tt/2b3nSzD
via IFTTT
Meet the Lawyer Who Defends Anonymous
from Google Alert - anonymous http://ift.tt/2b6Aip8
via IFTTT
de la soul and the anonymous nobody
from Google Alert - anonymous http://ift.tt/2beYqHY
via IFTTT
network-anonymous-tor
from Google Alert - anonymous http://ift.tt/2b0T6Uz
via IFTTT
Wednesday, August 17, 2016
I have a new follower on Twitter
VCCC
Musician / Producer
Manchester, England
https://t.co/2WKA5MLVQG
Following: 350 - Followers: 293
August 17, 2016 at 11:55PM via Twitter http://twitter.com/VCCC_album
Dynamic Collaborative Filtering with Compound Poisson Factorization. (arXiv:1608.04839v1 [cs.LG])
Model-based collaborative filtering analyzes user-item interactions to infer latent factors that represent user preferences and item characteristics in order to predict future interactions. Most collaborative filtering algorithms assume that these latent factors are static, although it has been shown that user preferences and item perceptions drift over time. In this paper, we propose a conjugate and numerically stable dynamic matrix factorization (DCPF) based on compound Poisson matrix factorization that models the smoothly drifting latent factors using Gamma-Markov chains. We propose a numerically stable Gamma chain construction, and then present a stochastic variational inference approach to estimate the parameters of our model. We apply our model to time-stamped ratings data sets: Netflix, Yelp, and Last.fm, where DCPF achieves a higher predictive accuracy than state-of-the-art static and dynamic factorization models.
from cs.AI updates on arXiv.org http://ift.tt/2b2hb0A
via IFTTT
Towards Music Captioning: Generating Music Playlist Descriptions. (arXiv:1608.04868v1 [cs.MM])
Descriptions are often provided along with recommendations to help users' discovery. Recommending automatically generated music playlists (e.g. personalised playlists) introduces the problem of generating descriptions. In this paper, we propose a method for generating music playlist descriptions, which is called as music captioning. In the proposed method, audio content analysis and natural language processing are adopted to utilise the information of each track.
from cs.AI updates on arXiv.org http://ift.tt/2boDSNF
via IFTTT
Open Problem: Approximate Planning of POMDPs in the class of Memoryless Policies. (arXiv:1608.04996v1 [cs.AI])
Planning plays an important role in the broad class of decision theory. Planning has drawn much attention in recent work in the robotics and sequential decision making areas. Recently, Reinforcement Learning (RL), as an agent-environment interaction problem, has brought further attention to planning methods. Generally in RL, one can assume a generative model, e.g. graphical models, for the environment, and then the task for the RL agent is to learn the model parameters and find the optimal strategy based on these learnt parameters. Based on environment behavior, the agent can assume various types of generative models, e.g. Multi Armed Bandit for a static environment, or Markov Decision Process (MDP) for a dynamic environment. The advantage of these popular models is their simplicity, which results in tractable methods of learning the parameters and finding the optimal policy. The drawback of these models is again their simplicity: these models usually underfit and underestimate the actual environment behavior. For example, in robotics, the agent usually has noisy observations of the environment inner state and MDP is not a suitable model.
More complex models like Partially Observable Markov Decision Process (POMDP) can compensate for this drawback. Fitting this model to the environment, where the partial observation is given to the agent, generally gives dramatic performance improvement, sometimes unbounded improvement, compared to MDP. In general, finding the optimal policy for the POMDP model is computationally intractable and fully non convex, even for the class of memoryless policies. The open problem is to come up with a method to find an exact or an approximate optimal stochastic memoryless policy for POMDP models.
from cs.AI updates on arXiv.org http://ift.tt/2bfQIwm
via IFTTT
Practical optimal experiment design with probabilistic programs. (arXiv:1608.05046v1 [cs.AI])
Scientists often run experiments to distinguish competing theories. This requires patience, rigor, and ingenuity - there is often a large space of possible experiments one could run. But we need not comb this space by hand - if we represent our theories as formal models and explicitly declare the space of experiments, we can automate the search for good experiments, looking for those with high expected information gain. Here, we present a general and principled approach to experiment design based on probabilistic programming languages (PPLs). PPLs offer a clean separation between declaring problems and solving them, which means that the scientist can automate experiment design by simply declaring her model and experiment spaces in the PPL without having to worry about the details of calculating information gain. We demonstrate our system in two case studies drawn from cognitive psychology, where we use it to design optimal experiments in the domains of sequence prediction and categorization. We find strong empirical validation that our automatically designed experiments were indeed optimal. We conclude by discussing a number of interesting questions for future research.
from cs.AI updates on arXiv.org http://ift.tt/2boE4wh
via IFTTT
Variational Information Maximizing Exploration. (arXiv:1605.09674v2 [cs.LG] UPDATED)
Scalable and effective exploration remains a key challenge in reinforcement learning (RL). While there are methods with optimality guarantees in the setting of discrete state and action spaces, these methods cannot be applied in high-dimensional deep RL scenarios. As such, most contemporary RL relies on simple heuristics such as epsilon-greedy exploration or adding Gaussian noise to the controls. This paper introduces Variational Information Maximizing Exploration (VIME), an exploration strategy based on maximization of information gain about the agent's belief of environment dynamics. We propose a practical implementation, using variational inference in Bayesian neural networks which efficiently handles continuous state and action spaces. VIME modifies the MDP reward function, and can be applied with several different underlying RL algorithms. We demonstrate that VIME achieves significantly better performance compared to heuristic exploration methods across a variety of continuous control tasks and algorithms, including tasks with very sparse rewards.
from cs.AI updates on arXiv.org http://ift.tt/1RKQdoC
via IFTTT
Bayesian Optimization with Dimension Scheduling: Application to Biological Systems. (arXiv:1511.05385v1 [stat.ML] CROSS LISTED)
Bayesian Optimization (BO) is a data-efficient method for global black-box optimization of an expensive-to-evaluate fitness function. BO typically assumes that computation cost of BO is cheap, but experiments are time consuming or costly. In practice, this allows us to optimize ten or fewer critical parameters in up to 1,000 experiments. But experiments may be less expensive than BO methods assume: In some simulation models, we may be able to conduct multiple thousands of experiments in a few hours, and the computational burden of BO is no longer negligible compared to experimentation time. To address this challenge we introduce a new Dimension Scheduling Algorithm (DSA), which reduces the computational burden of BO for many experiments. The key idea is that DSA optimizes the fitness function only along a small set of dimensions at each iteration. This DSA strategy (1) reduces the necessary computation time, (2) finds good solutions faster than the traditional BO method, and (3) can be parallelized straightforwardly. We evaluate the DSA in the context of optimizing parameters of dynamic models of microalgae metabolism and show faster convergence than traditional BO.
from cs.AI updates on arXiv.org http://ift.tt/1SWYNUt
via IFTTT
MLB: Orioles (66-52) host Red Sox (66-52) with both teams 1.5 games back in AL East race; watch live in the ESPN App (ESPN)
via IFTTT
Anonymous notes left on bistro bike by mystery 'busybody'
from Google Alert - anonymous http://ift.tt/2b1EswK
via IFTTT
Ravens: WR Steve Smith Sr. passes physical, activated off PUP list - multiple reports; tore Achilles in 2015 Week 8 (ESPN)
via IFTTT
The NSA Hack — What, When, Where, How, Who & Why? Explained Here...
from The Hacker News http://ift.tt/2aZrPbn
via IFTTT
The NSA Hack — What, When, Where, How, Who & Why? Explained Here...
from The Hacker News http://ift.tt/2bdFE2S
via IFTTT
ISS Daily Summary Report – 08/16/2016
from ISS On-Orbit Status Report http://ift.tt/2b0NHAs
via IFTTT
Migrate losing anonymous user record
from Google Alert - anonymous http://ift.tt/2bxJOT1
via IFTTT
I have a new follower on Twitter
Joan Carbonell
Several lifetimes of Projects + Learning + Sci-Fi + Family & Friends = obsession to inspire an amazing future. All opinions are my own.
Palma de Mallorca, Spain
http://t.co/sBlmpxRZuU
Following: 12573 - Followers: 14384
August 17, 2016 at 02:06AM via Twitter http://twitter.com/joancarbonell
I have a new follower on Twitter
Data Society
We envision a society where data science ignites conversations and collaboration across fields to solve problems we experience every day. #DataScienceEducation
Washington, DC
http://t.co/PvmdZct6VR
Following: 6940 - Followers: 9649
August 17, 2016 at 12:53AM via Twitter http://twitter.com/datasocietyco
Global Fires 2015-2016 B-Roll
from NASA's Scientific Visualization Studio: Most Recent Items http://ift.tt/2b376PH
via IFTTT
Five Planets and the Moon over Australia
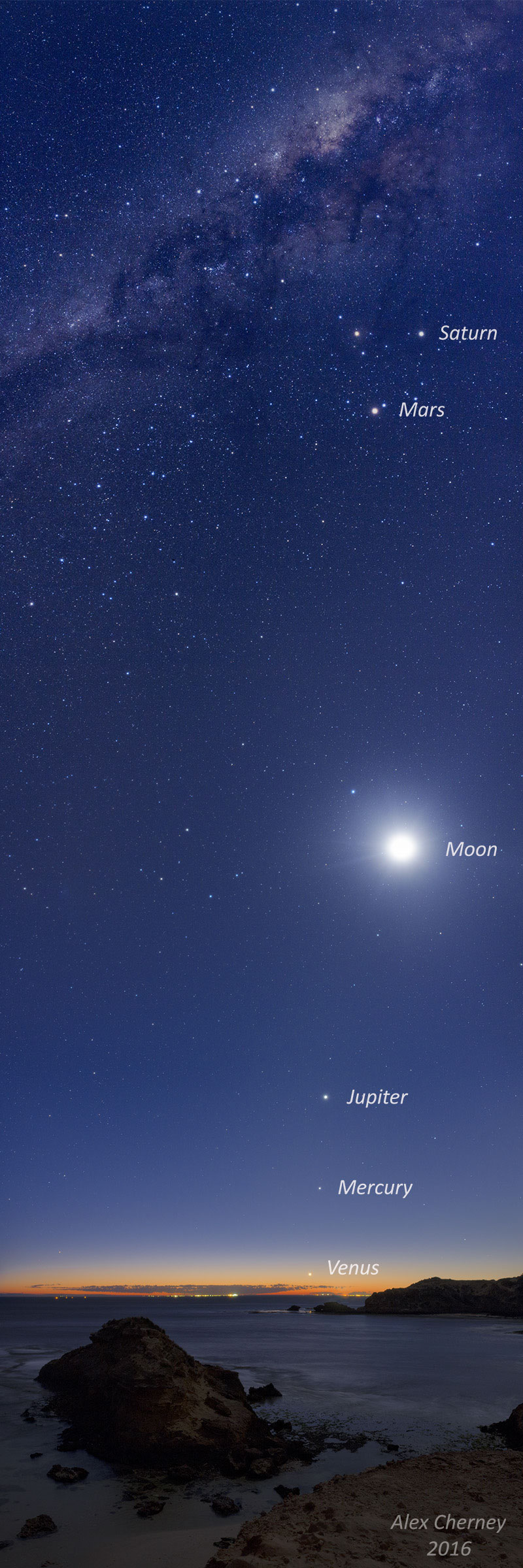
Tuesday, August 16, 2016
I have a new follower on Twitter
Fiona Green
28 years in #sportsbiz now focusing on the use of #CRM and BI with sports rights holders to drive #fanengagement, participation, insight and revenue
http://t.co/rdzvQqzGha
Following: 3091 - Followers: 6396
August 16, 2016 at 10:51PM via Twitter http://twitter.com/fionagreen66
I have a new follower on Twitter
Robert Osborne
Husband, Father, and Water Resources Engineer with Black & Veatch.
South Carolina
http://t.co/vjx9j8NZep
Following: 1891 - Followers: 3375
August 16, 2016 at 10:22PM via Twitter http://twitter.com/watercrunch
I have a new follower on Twitter
Samiran Ghosh
APAC Tech Leader @DnBUS. Accidental Technologist. #CIO. #Digital Evangelist. Movie Buff. Love Comics & #SocialMedia. Guest Speaker. Blogger. All views personal
Global Citizen
https://t.co/47APw6b8Yy
Following: 4066 - Followers: 4662
August 16, 2016 at 09:51PM via Twitter http://twitter.com/samiranghosh
No-Hitter Watch: Orioles' Steve Pearce singles to break up Red Sox's Eduardo Rodriguez, Matt Barnes combine no-hitter (ESPN)
via IFTTT
No-Hitter Watch: Red Sox's Eduardo Rodriguez and Matt Barnes have not allowed a hit through 6 innings vs. the Orioles (ESPN)
via IFTTT
TerpreT: A Probabilistic Programming Language for Program Induction. (arXiv:1608.04428v1 [cs.LG])
We study machine learning formulations of inductive program synthesis; given input-output examples, we try to synthesize source code that maps inputs to corresponding outputs. Our aims are to develop new machine learning approaches based on neural networks and graphical models, and to understand the capabilities of machine learning techniques relative to traditional alternatives, such as those based on constraint solving from the programming languages community.
Our key contribution is the proposal of TerpreT, a domain-specific language for expressing program synthesis problems. TerpreT is similar to a probabilistic programming language: a model is composed of a specification of a program representation (declarations of random variables) and an interpreter describing how programs map inputs to outputs (a model connecting unknowns to observations). The inference task is to observe a set of input-output examples and infer the underlying program. TerpreT has two main benefits. First, it enables rapid exploration of a range of domains, program representations, and interpreter models. Second, it separates the model specification from the inference algorithm, allowing like-to-like comparisons between different approaches to inference. From a single TerpreT specification we automatically perform inference using four different back-ends. These are based on gradient descent, linear program (LP) relaxations for graphical models, discrete satisfiability solving, and the Sketch program synthesis system.
We illustrate the value of TerpreT by developing several interpreter models and performing an empirical comparison between alternative inference algorithms. Our key empirical finding is that constraint solvers dominate the gradient descent and LP-based formulations. We conclude with suggestions for the machine learning community to make progress on program synthesis.
from cs.AI updates on arXiv.org http://ift.tt/2blJAQd
via IFTTT
Free Lunch for Optimisation under the Universal Distribution. (arXiv:1608.04544v1 [math.OC])
Function optimisation is a major challenge in computer science. The No Free Lunch theorems state that if all functions with the same histogram are assumed to be equally probable then no algorithm outperforms any other in expectation. We argue against the uniform assumption and suggest a universal prior exists for which there is a free lunch, but where no particular class of functions is favoured over another. We also prove upper and lower bounds on the size of the free lunch.
from cs.AI updates on arXiv.org http://ift.tt/2bcuRWs
via IFTTT
Informal Physical Reasoning Processes. (arXiv:1608.04672v1 [cs.AI])
A fundamental question is whether Turing machines can model all reasoning processes. We introduce an existence principle stating that the perception of the physical existence of any Turing program can serve as a physical causation for the application of any Turing-computable function to this Turing program. The existence principle overcomes the limitation of the outputs of Turing machines to lists, that is, recursively enumerable sets. The principle is illustrated by productive partial functions for productive sets such as the set of the Goedel numbers of the Turing-computable total functions. The existence principle and productive functions imply the existence of physical systems whose reasoning processes cannot be modeled by Turing machines. These systems are called creative. Creative systems can prove the undecidable formula in Goedel's theorem in another formal system which is constructed at a later point in time. A hypothesis about creative systems, which is based on computer experiments, is introduced.
from cs.AI updates on arXiv.org http://ift.tt/2blJmsp
via IFTTT
A Shallow High-Order Parametric Approach to Data Visualization and Compression. (arXiv:1608.04689v1 [cs.AI])
Explicit high-order feature interactions efficiently capture essential structural knowledge about the data of interest and have been used for constructing generative models. We present a supervised discriminative High-Order Parametric Embedding (HOPE) approach to data visualization and compression. Compared to deep embedding models with complicated deep architectures, HOPE generates more effective high-order feature mapping through an embarrassingly simple shallow model. Furthermore, two approaches to generating a small number of exemplars conveying high-order interactions to represent large-scale data sets are proposed. These exemplars in combination with the feature mapping learned by HOPE effectively capture essential data variations. Moreover, through HOPE, these exemplars are employed to increase the computational efficiency of kNN classification for fast information retrieval by thousands of times. For classification in two-dimensional embedding space on MNIST and USPS datasets, our shallow method HOPE with simple Sigmoid transformations significantly outperforms state-of-the-art supervised deep embedding models based on deep neural networks, and even achieved historically low test error rate of 0.65% in two-dimensional space on MNIST, which demonstrates the representational efficiency and power of supervised shallow models with high-order feature interactions.
from cs.AI updates on arXiv.org http://ift.tt/2bctBme
via IFTTT
Evaluating Causal Models by Comparing Interventional Distributions. (arXiv:1608.04698v1 [cs.AI])
The predominant method for evaluating the quality of causal models is to measure the graphical accuracy of the learned model structure. We present an alternative method for evaluating causal models that directly measures the accuracy of estimated interventional distributions. We contrast such distributional measures with structural measures, such as structural Hamming distance and structural intervention distance, showing that structural measures often correspond poorly to the accuracy of estimated interventional distributions. We use a number of real and synthetic datasets to illustrate various scenarios in which structural measures provide misleading results with respect to algorithm selection and parameter tuning, and we recommend that distributional measures become the new standard for evaluating causal models.
from cs.AI updates on arXiv.org http://ift.tt/2blIQuD
via IFTTT
Learning values across many orders of magnitude. (arXiv:1602.07714v2 [cs.LG] UPDATED)
Most learning algorithms are not invariant to the scale of the function that is being approximated. We propose to adaptively normalize the targets used in learning. This is useful in value-based reinforcement learning, where the magnitude of appropriate value approximations can change over time when we update the policy of behavior. Our main motivation is prior work on learning to play Atari games, where the rewards were all clipped to a predetermined range. This clipping facilitates learning across many different games with a single learning algorithm, but a clipped reward function can result in qualitatively different behavior. Using the adaptive normalization we can remove this domain-specific heuristic without diminishing overall performance.
from cs.AI updates on arXiv.org http://ift.tt/1Qit6Cj
via IFTTT
Learning to Track at 100 FPS with Deep Regression Networks. (arXiv:1604.01802v2 [cs.CV] UPDATED)
Machine learning techniques are often used in computer vision due to their ability to leverage large amounts of training data to improve performance. Unfortunately, most generic object trackers are still trained from scratch online and do not benefit from the large number of videos that are readily available for offline training. We propose a method for offline training of neural networks that can track novel objects at test-time at 100 fps. Our tracker is significantly faster than previous methods that use neural networks for tracking, which are typically very slow to run and not practical for real-time applications. Our tracker uses a simple feed-forward network with no online training required. The tracker learns a generic relationship between object motion and appearance and can be used to track novel objects that do not appear in the training set. We test our network on a standard tracking benchmark to demonstrate our tracker's state-of-the-art performance. Further, our performance improves as we add more videos to our offline training set. To the best of our knowledge, our tracker is the first neural-network tracker that learns to track generic objects at 100 fps.
from cs.AI updates on arXiv.org http://ift.tt/1XkoPmu
via IFTTT
Multi-way Monte Carlo Method for Linear Systems. (arXiv:1608.04361v1 [cs.NA])
We study the Monte Carlo method for solving a linear system of the form $x = H x + b$. A sufficient condition for the method to work is $\| H \| < 1$, which greatly limits the usability of this method. We improve this condition by proposing a new multi-way Markov random walk, which is a generalization of the standard Markov random walk. Under our new framework we prove that the necessary and sufficient condition for our method to work is the spectral radius $\rho(H^{+}) < 1$, which is a weaker requirement than $\| H \| < 1$. In addition to solving more problems, our new method can work faster than the standard algorithm. In numerical experiments on both synthetic and real world matrices, we demonstrate the effectiveness of our new method.
from cs.AI updates on arXiv.org http://ift.tt/2biWRsM
via IFTTT
I have a new follower on Twitter
SPBMC PC
Sullivan Papain Block McGrath & Cannavo P.C. - New York, Long Island and New Jersey Personal Injury Lawyers.
New York, NY
http://t.co/Fq9LgMx6UG
Following: 53 - Followers: 25
August 16, 2016 at 04:44PM via Twitter http://twitter.com/SPBMCPC
Can Anonymous functions contain unknown variables?
from Google Alert - anonymous http://ift.tt/2aYp8RB
via IFTTT
Someone is Spying on Researchers Behind VeraCrypt Security Audit
from The Hacker News http://ift.tt/2bfou2F
via IFTTT
Internet Traffic Hijacking Linux Flaw Affects 80% of Android Devices
from The Hacker News http://ift.tt/2b9b76V
via IFTTT
ISS Daily Summary Report – 08/15/2016
from ISS On-Orbit Status Report http://ift.tt/2aQYRco
via IFTTT
Re: [FD] Zabbix 2.2.x, 3.0.x SQL Injection Vulnerability
Timestamp | Value |
---|---|
No data found. |
- Error in query [INSERT INTO profiles (profileid, userid, idx, value_int, type, idx2) VALUES (39, 1, 'web.item.graph.period', '3600', 2, 2'3297)] [You have an error in your SQL syntax; check the manual that corresponds to your MySQL server version for the right syntax to use near ''3297)' at line 1]
- Error in query [INSERT INTO profiles (profileid, userid, idx, value_str, type, idx2) VALUES (40, 1, 'web.item.graph.stime', '20160813041028', 3, 2'3297)] [You have an error in your SQL syntax; check the manual that corresponds to your MySQL server version for the right syntax to use near ''3297)' at line 1]
- Error in query [INSERT INTO profiles (profileid, userid, idx, value_int, type, idx2) VALUES (41, 1, 'web.item.graph.isnow', '1', 2, 2'3297)] [You have an error in your SQL syntax; check the manual that corresponds to your MySQL server version for the right syntax to use near ''3297)' at line 1]
Source: Gmail -> IFTTT-> Blogger
[FD] German Cable Provider Router (In)Security
Source: Gmail -> IFTTT-> Blogger
[FD] Actiontec T2200H (Telus Modem) Root Reverse Shell
Source: Gmail -> IFTTT-> Blogger
An Anonymous User Authentication and Key Agreement Scheme Based on a Symmetric ...
from Google Alert - anonymous http://ift.tt/2bmyFD9
via IFTTT
China Launches World's 1st 'Hack-Proof' Quantum Communication Satellite
from The Hacker News http://ift.tt/2bjLBwb
via IFTTT
I have a new follower on Twitter
Carl-G Schimmelmann
Denmark
http://t.co/1OZlLz5z6O
Following: 7520 - Followers: 8195
August 16, 2016 at 12:54AM via Twitter http://twitter.com/TimeXtenderDWA
Human as Spaceship
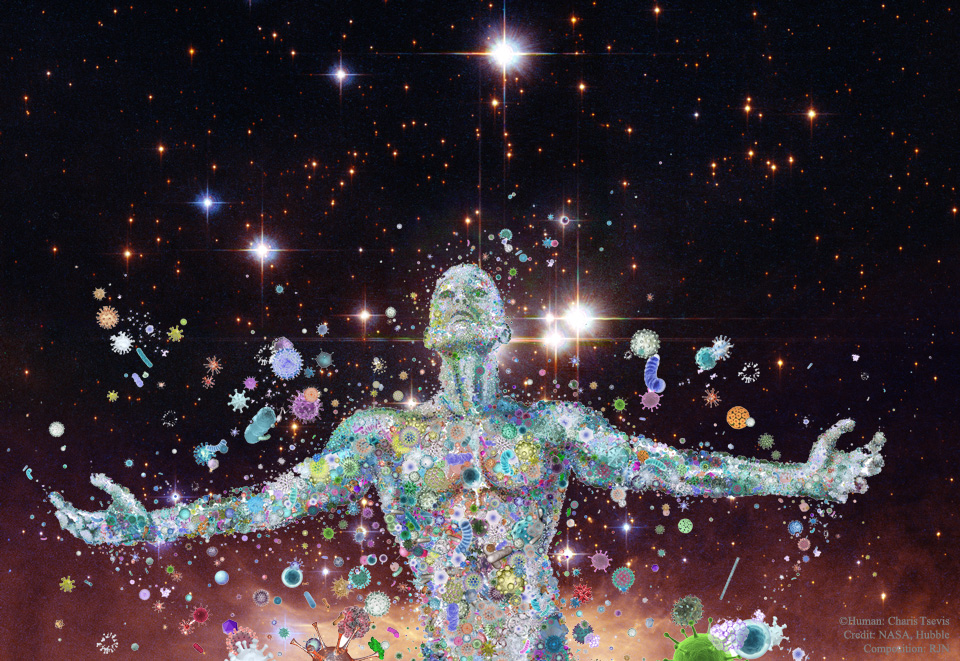
Monday, August 15, 2016
I have a new follower on Twitter
Kate Blanchard
Biotech co-founder @ORIG3N_Inc, technology enthusiast, driven to extend lives with regenerative medicine. #boston #ohiostate @kenanflagler
Boston, MA
https://t.co/z9WCZJqJyH
Following: 8988 - Followers: 9872
August 15, 2016 at 10:09PM via Twitter http://twitter.com/KateSBlanchard
I have a new follower on Twitter
Mark Crone
Passionate about #Travel & #Tourism, #TravelTips & #TravelReviews; @UniglobeBizTrvl, @CdnSkiPatrol, contributor @Liftopia, @thehipmunk; #Blogger on my blog:
Toronto, Canada
https://t.co/HmHV6EKbtH
Following: 11355 - Followers: 13901
August 15, 2016 at 10:09PM via Twitter http://twitter.com/MarkTravel
Determining Health Utilities through Data Mining of Social Media. (arXiv:1608.03938v1 [cs.CL])
'Health utilities' measure patient preferences for perfect health compared to specific unhealthy states, such as asthma, a fractured hip, or colon cancer. When integrated over time, these estimations are called quality adjusted life years (QALYs). Until now, characterizing health utilities (HUs) required detailed patient interviews or written surveys. While reliable and specific, this data remained costly due to efforts to locate, enlist and coordinate participants. Thus the scope, context and temporality of diseases examined has remained limited.
Now that more than a billion people use social media, we propose a novel strategy: use natural language processing to analyze public online conversations for signals of the severity of medical conditions and correlate these to known HUs using machine learning. In this work, we filter a dataset that originally contained 2 billion tweets for relevant content on 60 diseases. Using this data, our algorithm successfully distinguished mild from severe diseases, which had previously been categorized only by traditional techniques. This represents progress towards two related applications: first, predicting HUs where such information is nonexistent; and second, (where rich HU data already exists) estimating temporal or geographic patterns of disease severity through data mining.
from cs.AI updates on arXiv.org http://ift.tt/2aXlAlu
via IFTTT
Can Peripheral Representations Improve Clutter Metrics on Complex Scenes?. (arXiv:1608.04042v1 [cs.CV])
Previous studies have proposed image-based clutter measures that correlate with human search times and/or eye movements. However, most models do not take into account the fact that the effects of clutter interact with the foveated nature of the human visual system: visual clutter further from the fovea has an increasing detrimental influence on perception. Here, we introduce a new foveated clutter model to predict the detrimental effects in target search utilizing a forced fixation search task. We use Feature Congestion (Rosenholtz et al.) as our non foveated clutter model, and we stack a peripheral architecture on top of Feature Congestion for our foveated model. We introduce the Peripheral Integration Feature Congestion (PIFC) coefficient, as a fundamental ingredient of our model that modulates clutter as a non-linear gain contingent on eccentricity. We finally show that Foveated Feature Congestion (FFC) clutter scores r(44) = -0.82 correlate better with target detection (hit rate) than regular Feature Congestion r(44) = -0.19 in forced fixation search. Thus, our model allows us to enrich clutter perception research by computing fixation specific clutter maps. A toolbox for creating peripheral architectures: Piranhas: Peripheral Architectures for Natural, Hybrid and Artificial Systems will be made available.
from cs.AI updates on arXiv.org http://ift.tt/2aVsbcP
via IFTTT
A Geometric Framework for Convolutional Neural Networks. (arXiv:1608.04374v1 [stat.ML])
In this paper, a geometric framework for neural networks is proposed. This framework uses the inner product space structure underlying the parameter set to perform gradient descent not in a component-based form, but in a coordinate-free manner. Convolutional neural networks are described in this framework in a compact form, with the gradients of standard --- and higher-order --- loss functions calculated for each layer of the network. This approach can be applied to other network structures and provides a basis on which to create new networks.
from cs.AI updates on arXiv.org http://ift.tt/2aVrn7O
via IFTTT
A Parallel Algorithm for Exact Bayesian Structure Discovery in Bayesian Networks. (arXiv:1408.1664v3 [cs.AI] UPDATED)
Exact Bayesian structure discovery in Bayesian networks requires exponential time and space. Using dynamic programming (DP), the fastest known sequential algorithm computes the exact posterior probabilities of structural features in $O(2(d+1)n2^n)$ time and space, if the number of nodes (variables) in the Bayesian network is $n$ and the in-degree (the number of parents) per node is bounded by a constant $d$. Here we present a parallel algorithm capable of computing the exact posterior probabilities for all $n(n-1)$ edges with optimal parallel space efficiency and nearly optimal parallel time efficiency. That is, if $p=2^k$ processors are used, the run-time reduces to $O(5(d+1)n2^{n-k}+k(n-k)^d)$ and the space usage becomes $O(n2^{n-k})$ per processor. Our algorithm is based the observation that the subproblems in the sequential DP algorithm constitute a $n$-$D$ hypercube. We take a delicate way to coordinate the computation of correlated DP procedures such that large amount of data exchange is suppressed. Further, we develop parallel techniques for two variants of the well-known \emph{zeta transform}, which have applications outside the context of Bayesian networks. We demonstrate the capability of our algorithm on datasets with up to 33 variables and its scalability on up to 2048 processors. We apply our algorithm to a biological data set for discovering the yeast pheromone response pathways.
from cs.AI updates on arXiv.org http://ift.tt/1nxBLRX
via IFTTT
Natural Language Generation enhances human decision-making with uncertain information. (arXiv:1606.03254v2 [cs.CL] UPDATED)
Decision-making is often dependent on uncertain data, e.g. data associated with confidence scores or probabilities. We present a comparison of different information presentations for uncertain data and, for the first time, measure their effects on human decision-making. We show that the use of Natural Language Generation (NLG) improves decision-making under uncertainty, compared to state-of-the-art graphical-based representation methods. In a task-based study with 442 adults, we found that presentations using NLG lead to 24% better decision-making on average than the graphical presentations, and to 44% better decision-making when NLG is combined with graphics. We also show that women achieve significantly better results when presented with NLG output (an 87% increase on average compared to graphical presentations).
from cs.AI updates on arXiv.org http://ift.tt/1UuC2Gc
via IFTTT
Fully DNN-based Multi-label regression for audio tagging. (arXiv:1606.07695v2 [cs.CV] UPDATED)
Acoustic event detection for content analysis in most cases relies on lots of labeled data. However, manually annotating data is a time-consuming task, which thus makes few annotated resources available so far. Unlike audio event detection, automatic audio tagging, a multi-label acoustic event classification task, only relies on weakly labeled data. This is highly desirable to some practical applications using audio analysis. In this paper we propose to use a fully deep neural network (DNN) framework to handle the multi-label classification task in a regression way. Considering that only chunk-level rather than frame-level labels are available, the whole or almost whole frames of the chunk were fed into the DNN to perform a multi-label regression for the expected tags. The fully DNN, which is regarded as an encoding function, can well map the audio features sequence to a multi-tag vector. A deep pyramid structure was also designed to extract more robust high-level features related to the target tags. Further improved methods were adopted, such as the Dropout and background noise aware training, to enhance its generalization capability for new audio recordings in mismatched environments. Compared with the conventional Gaussian Mixture Model (GMM) and support vector machine (SVM) methods, the proposed fully DNN-based method could well utilize the long-term temporal information with the whole chunk as the input. The results show that our approach obtained a 15% relative improvement compared with the official GMM-based method of DCASE 2016 challenge.
from cs.AI updates on arXiv.org http://ift.tt/28X80gY
via IFTTT
CaR-FOREST: Joint Classification-Regression Decision Forests for Overlapping Audio Event Detection. (arXiv:1607.02306v2 [cs.SD] UPDATED)
This report describes our submissions to Task2 and Task3 of the DCASE 2016 challenge. The systems aim at dealing with the detection of overlapping audio events in continuous streams, where the detectors are based on random decision forests. The proposed forests are jointly trained for classification and regression simultaneously. Initially, the training is classification-oriented to encourage the trees to select discriminative features from overlapping mixtures to separate positive audio segments from the negative ones. The regression phase is then carried out to let the positive audio segments vote for the event onsets and offsets, and therefore model the temporal structure of audio events. One random decision forest is specifically trained for each event category of interest. Experimental results on the development data show that our systems significantly outperform the baseline on the Task2 evaluation while they are inferior to the baseline in the Task3 evaluation.
from cs.AI updates on arXiv.org http://ift.tt/29wGQ3s
via IFTTT
Towards Analytics Aware Ontology Based Access to Static and Streaming Data (Extended Version). (arXiv:1607.05351v2 [cs.AI] UPDATED)
Real-time analytics that requires integration and aggregation of heterogeneous and distributed streaming and static data is a typical task in many industrial scenarios such as diagnostics of turbines in Siemens. OBDA approach has a great potential to facilitate such tasks; however, it has a number of limitations in dealing with analytics that restrict its use in important industrial applications. Based on our experience with Siemens, we argue that in order to overcome those limitations OBDA should be extended and become analytics, source, and cost aware. In this work we propose such an extension. In particular, we propose an ontology, mapping, and query language for OBDA, where aggregate and other analytical functions are first class citizens. Moreover, we develop query optimisation techniques that allow to efficiently process analytical tasks over static and streaming data. We implement our approach in a system and evaluate our system with Siemens turbine data.
from cs.AI updates on arXiv.org http://ift.tt/29TO2Et
via IFTTT
Anonymous donor gives trike to disabled man
from Google Alert - anonymous http://ift.tt/2btUx0I
via IFTTT
NSA's Hacking Group Hacked! Bunch of Private Hacking Tools Leaked Online
from The Hacker News http://ift.tt/2aW5gV7
via IFTTT
[FD] Persistent Cross-Site Scripting in Magic Fields 1 WordPress Plugin
Source: Gmail -> IFTTT-> Blogger
[FD] Persistent Cross-Site Scripting in Magic Fields 2 WordPress Plugin
Source: Gmail -> IFTTT-> Blogger
[FD] Cross-Site Scripting in Link Library WordPress Plugin
Source: Gmail -> IFTTT-> Blogger
[FD] Ajax Load More Local File Inclusion vulnerability
Source: Gmail -> IFTTT-> Blogger
[FD] Cross-Site Scripting/Cross-Site Request Forgery in Peter's Login Redirect WordPress Plugin
Source: Gmail -> IFTTT-> Blogger
[FD] Cross-Site Request Forgery vulnerability in Email Users WordPress Plugin
Source: Gmail -> IFTTT-> Blogger
[FD] Cross-Site Scripting vulnerability in Google Maps WordPress Plugin
Source: Gmail -> IFTTT-> Blogger
[FD] Stored Cross-Site Scripting vulnerability in Photo Gallery WordPress Plugin
Source: Gmail -> IFTTT-> Blogger
[FD] Cross-Site Request Forgery in Photo Gallery WordPress Plugin allows deleting of images
Source: Gmail -> IFTTT-> Blogger
[FD] Cross-Site Request Forgery in Photo Gallery WordPress Plugin allows deleting of galleries
Source: Gmail -> IFTTT-> Blogger
[FD] Cross-Site Request Forgery in Photo Gallery WordPress Plugin allows adding of images
Source: Gmail -> IFTTT-> Blogger
Ravens: LB Terrell Suggs releases statement after his first practice in 11 months, says "Darth Sizzle is back" (ESPN)
via IFTTT
I have a new follower on Twitter
Python Eggs
#python addict
Paris, Ile-de-France
Following: 2141 - Followers: 2655
August 15, 2016 at 12:12PM via Twitter http://twitter.com/PythonEggs
This basically anonymous fund manager oversees $800bn
from Google Alert - anonymous http://ift.tt/2bykFuw
via IFTTT
D8: Anonymous users can see unpublished content
from Google Alert - anonymous http://ift.tt/2bsGnw5
via IFTTT
How to tune hyperparameters with Python and scikit-learn
In last week’s post, I introduced the k-NN machine learning algorithm which we then applied to the task of image classification.
Using the k-NN algorithm, we obtained 57.58% classification accuracy on the Kaggle Dogs vs. Cats dataset challenge:
Figure 1: Classifying an image as whether it contains a dog or a cat.
The question is: “Can we do better?”
Of course we can! Obtaining higher accuracy for nearly any machine learning algorithm boils down to tweaking various knobs and levels.
In the case of k-NN, we can tune k, the number of nearest neighbors. We can also tune our distance metric/similarity function as well.
Of course, hyperparameter tuning has implications outside of the k-NN algorithm as well. In the context of Deep Learning and Convolutional Neural Networks, we can easily have hundreds of various hyperparameters to tune and play with (although in practice we try to limit the number of variables to tune to a small handful), each affecting our overall classification to some (potentially unknown) degree.
Because of this, it’s important to understand the concept of hyperparameter tuning and how your choice in hyperparameters can dramatically impact your classification accuracy.
Looking for the source code to this post?
Jump right to the downloads section.
How to tune hyperparameters with Python and scikit-learn
In the remainder of today’s tutorial, I’ll be demonstrating how to tune k-NN hyperparameters for the Dogs vs. Cats dataset. We’ll start with a discussion on what hyperparameters are, followed by viewing a concrete example on tuning k-NN hyperparameters.
We’ll then explore how to tune k-NN hyperparameters using two search methods: Grid Search and Randomized Search.
As our results will demonstrate, we can improve our classification accuracy from 57.58% to over 64%!
What are hyperparameters?
Hyperparameters are simply the knobs and levels you pull and turn when building a machine learning classifier. The process of tuning hyperparameters is more formally called hyperparameter optimization.
So what’s the difference between a normal “model parameter” and a “hyperparameter”?
Well, a standard “model parameter” is normally an internal variable that is optimized in some fashion. In the context of Linear Regression, Logistic Regression, and Support Vector Machines, we would think of parameters as the weight vector coefficients found by the learning algorithm.
On the other hand, “hyperparameters” are normally set by a human designer or tuned via algorithmic approaches. Examples of hyperparameters include the number of neighbors k in the k-Nearest Neighbor algorithm, the learning rate alpha of a Neural Network, or the number of filters learned in a given convolutional layer in a CNN.
In general, model parameters are optimized according to some loss function, while hyperparameters are instead searched for by exploring various settings to see which values provided the highest level of accuracy.
Because of this, it tends to be easier to tune model parameters (since we’re optimizing some objective function based on our training data) whereas hyperparameters can require a nearly blind search to find optimal ones.
k-NN hyperparameters
As a concrete example of tuning hyperparameters, let’s consider the k-Nearest Neighbor classification algorithm. For your standard k-NN implementation, there are two primary hyperparameters that you’ll want to tune:
- The number of neighbors k.
- The distance metric/similarity function.
Both of these values can dramatically affect the accuracy of your k-NN classifier. To demonstrate this in the context of image classification, let’s apply hyperparameter tuning to our Kaggle Dogs vs. Cats dataset from last week.
Open up a new file, name it
knn_tune.py, and insert the following code:
# import the necessary packages from sklearn.neighbors import KNeighborsClassifier from sklearn.grid_search import RandomizedSearchCV from sklearn.grid_search import GridSearchCV from sklearn.cross_validation import train_test_split from imutils import paths import numpy as np import argparse import imutils import time import cv2 import os
Lines 2-12 start by importing our required Python packages. We’ll be making heavy use of the scikit-learn library, so if you do not have it installed, make sure you follow these instructions.
We’ll also be using my personal imutils library, so make sure you have it installed as well:
$ pip install imutils
Next, we’ll define our
extract_color_histogramfunction:
# import the necessary packages from sklearn.neighbors import KNeighborsClassifier from sklearn.grid_search import RandomizedSearchCV from sklearn.grid_search import GridSearchCV from sklearn.cross_validation import train_test_split from imutils import paths import numpy as np import argparse import imutils import time import cv2 import os def extract_color_histogram(image, bins=(8, 8, 8)): # extract a 3D color histogram from the HSV color space using # the supplied number of `bins` per channel hsv = cv2.cvtColor(image, cv2.COLOR_BGR2HSV) hist = cv2.calcHist([hsv], [0, 1, 2], None, bins, [0, 180, 0, 256, 0, 256]) # handle normalizing the histogram if we are using OpenCV 2.4.X if imutils.is_cv2(): hist = cv2.normalize(hist) # otherwise, perform "in place" normalization in OpenCV 3 (I # personally hate the way this is done else: cv2.normalize(hist, hist) # return the flattened histogram as the feature vector return hist.flatten()
This function accepts an input
imagealong with a number of
binsfor each channel of the image.
We convert the image to the HSV color space and compute a 3D color histogram to characterize the color distribution of the image (Lines 17-19).
This histogram is then flattened into a single 8 x 8 x 8 = 512-d feature vector that is returned to the calling function.
For a more detailed review of this method, please refer to last week’s blog post.
# import the necessary packages from sklearn.neighbors import KNeighborsClassifier from sklearn.grid_search import RandomizedSearchCV from sklearn.grid_search import GridSearchCV from sklearn.cross_validation import train_test_split from imutils import paths import numpy as np import argparse import imutils import time import cv2 import os def extract_color_histogram(image, bins=(8, 8, 8)): # extract a 3D color histogram from the HSV color space using # the supplied number of `bins` per channel hsv = cv2.cvtColor(image, cv2.COLOR_BGR2HSV) hist = cv2.calcHist([hsv], [0, 1, 2], None, bins, [0, 180, 0, 256, 0, 256]) # handle normalizing the histogram if we are using OpenCV 2.4.X if imutils.is_cv2(): hist = cv2.normalize(hist) # otherwise, perform "in place" normalization in OpenCV 3 (I # personally hate the way this is done else: cv2.normalize(hist, hist) # return the flattened histogram as the feature vector return hist.flatten() # construct the argument parse and parse the arguments ap = argparse.ArgumentParser() ap.add_argument("-d", "--dataset", required=True, help="path to input dataset") ap.add_argument("-j", "--jobs", type=int, default=-1, help="# of jobs for k-NN distance (-1 uses all available cores)") args = vars(ap.parse_args()) # grab the list of images that we'll be describing print("[INFO] describing images...") imagePaths = list(paths.list_images(args["dataset"])) # initialize the data matrix and labels list data = [] labels = []
Lines 34-39 handle parsing our command line arguments. We only need two switches here:
-
--dataset
: The path to our input Dogs vs. Cats dataset from the Kaggle challenge. -
--jobs
: The number of processors/cores to utilize when computing the nearest neighbors for a particular data point. Setting this value to-1
indicates all available processors/cores should be used. Again, for a more detailed review of these arguments, please refer to last week’s tutorial.
Line 43 grabs the paths to our 25,000 input images while Lines 46 and 47 initializes the
datalist (where we’ll store the color histogram extracted from each image) and
labelslist (either “dog” or “cat” for each input image), respectively.
Next, we can loop over our
imagePathsand describe them:
# import the necessary packages from sklearn.neighbors import KNeighborsClassifier from sklearn.grid_search import RandomizedSearchCV from sklearn.grid_search import GridSearchCV from sklearn.cross_validation import train_test_split from imutils import paths import numpy as np import argparse import imutils import time import cv2 import os def extract_color_histogram(image, bins=(8, 8, 8)): # extract a 3D color histogram from the HSV color space using # the supplied number of `bins` per channel hsv = cv2.cvtColor(image, cv2.COLOR_BGR2HSV) hist = cv2.calcHist([hsv], [0, 1, 2], None, bins, [0, 180, 0, 256, 0, 256]) # handle normalizing the histogram if we are using OpenCV 2.4.X if imutils.is_cv2(): hist = cv2.normalize(hist) # otherwise, perform "in place" normalization in OpenCV 3 (I # personally hate the way this is done else: cv2.normalize(hist, hist) # return the flattened histogram as the feature vector return hist.flatten() # construct the argument parse and parse the arguments ap = argparse.ArgumentParser() ap.add_argument("-d", "--dataset", required=True, help="path to input dataset") ap.add_argument("-j", "--jobs", type=int, default=-1, help="# of jobs for k-NN distance (-1 uses all available cores)") args = vars(ap.parse_args()) # grab the list of images that we'll be describing print("[INFO] describing images...") imagePaths = list(paths.list_images(args["dataset"])) # initialize the data matrix and labels list data = [] labels = [] # loop over the input images for (i, imagePath) in enumerate(imagePaths): # load the image and extract the class label (assuming that our # path as the format: /path/to/dataset/{class}.{image_num}.jpg image = cv2.imread(imagePath) label = imagePath.split(os.path.sep)[-1].split(".")[0] # extract a color histogram from the image, then update the # data matrix and labels list hist = extract_color_histogram(image) data.append(hist) labels.append(label) # show an update every 1,000 images if i > 0 and i % 1000 == 0: print("[INFO] processed {}/{}".format(i, len(imagePaths)))
Line 50 starts looping over each of the
imagePaths. For each
imagePath, we load it from disk and extract the
label(Lines 53 and 54).
Now that we have our
image, we compute a color histogram (Line 58), followed by updating the
dataand
labelslists (Lines 59 and 60).
Finally, Lines 63 and 64 display the feature extraction progress to our screen.
In order to train and evaluate our k-NN classifier, we’ll need to partition our
datainto two splits: a training split and a testing split:
# import the necessary packages from sklearn.neighbors import KNeighborsClassifier from sklearn.grid_search import RandomizedSearchCV from sklearn.grid_search import GridSearchCV from sklearn.cross_validation import train_test_split from imutils import paths import numpy as np import argparse import imutils import time import cv2 import os def extract_color_histogram(image, bins=(8, 8, 8)): # extract a 3D color histogram from the HSV color space using # the supplied number of `bins` per channel hsv = cv2.cvtColor(image, cv2.COLOR_BGR2HSV) hist = cv2.calcHist([hsv], [0, 1, 2], None, bins, [0, 180, 0, 256, 0, 256]) # handle normalizing the histogram if we are using OpenCV 2.4.X if imutils.is_cv2(): hist = cv2.normalize(hist) # otherwise, perform "in place" normalization in OpenCV 3 (I # personally hate the way this is done else: cv2.normalize(hist, hist) # return the flattened histogram as the feature vector return hist.flatten() # construct the argument parse and parse the arguments ap = argparse.ArgumentParser() ap.add_argument("-d", "--dataset", required=True, help="path to input dataset") ap.add_argument("-j", "--jobs", type=int, default=-1, help="# of jobs for k-NN distance (-1 uses all available cores)") args = vars(ap.parse_args()) # grab the list of images that we'll be describing print("[INFO] describing images...") imagePaths = list(paths.list_images(args["dataset"])) # initialize the data matrix and labels list data = [] labels = [] # loop over the input images for (i, imagePath) in enumerate(imagePaths): # load the image and extract the class label (assuming that our # path as the format: /path/to/dataset/{class}.{image_num}.jpg image = cv2.imread(imagePath) label = imagePath.split(os.path.sep)[-1].split(".")[0] # extract a color histogram from the image, then update the # data matrix and labels list hist = extract_color_histogram(image) data.append(hist) labels.append(label) # show an update every 1,000 images if i > 0 and i % 1000 == 0: print("[INFO] processed {}/{}".format(i, len(imagePaths))) # partition the data into training and testing splits, using 75% # of the data for training and the remaining 25% for testing print("[INFO] constructing training/testing split...") (trainData, testData, trainLabels, testLabels) = train_test_split( data, labels, test_size=0.25, random_state=42)
Here we’ll be using 75% of our data for training and the remaining 25% for evaluation.
Finally, let’s define the set of hyperparameters we are going to optimize over:
# import the necessary packages from sklearn.neighbors import KNeighborsClassifier from sklearn.grid_search import RandomizedSearchCV from sklearn.grid_search import GridSearchCV from sklearn.cross_validation import train_test_split from imutils import paths import numpy as np import argparse import imutils import time import cv2 import os def extract_color_histogram(image, bins=(8, 8, 8)): # extract a 3D color histogram from the HSV color space using # the supplied number of `bins` per channel hsv = cv2.cvtColor(image, cv2.COLOR_BGR2HSV) hist = cv2.calcHist([hsv], [0, 1, 2], None, bins, [0, 180, 0, 256, 0, 256]) # handle normalizing the histogram if we are using OpenCV 2.4.X if imutils.is_cv2(): hist = cv2.normalize(hist) # otherwise, perform "in place" normalization in OpenCV 3 (I # personally hate the way this is done else: cv2.normalize(hist, hist) # return the flattened histogram as the feature vector return hist.flatten() # construct the argument parse and parse the arguments ap = argparse.ArgumentParser() ap.add_argument("-d", "--dataset", required=True, help="path to input dataset") ap.add_argument("-j", "--jobs", type=int, default=-1, help="# of jobs for k-NN distance (-1 uses all available cores)") args = vars(ap.parse_args()) # grab the list of images that we'll be describing print("[INFO] describing images...") imagePaths = list(paths.list_images(args["dataset"])) # initialize the data matrix and labels list data = [] labels = [] # loop over the input images for (i, imagePath) in enumerate(imagePaths): # load the image and extract the class label (assuming that our # path as the format: /path/to/dataset/{class}.{image_num}.jpg image = cv2.imread(imagePath) label = imagePath.split(os.path.sep)[-1].split(".")[0] # extract a color histogram from the image, then update the # data matrix and labels list hist = extract_color_histogram(image) data.append(hist) labels.append(label) # show an update every 1,000 images if i > 0 and i % 1000 == 0: print("[INFO] processed {}/{}".format(i, len(imagePaths))) # partition the data into training and testing splits, using 75% # of the data for training and the remaining 25% for testing print("[INFO] constructing training/testing split...") (trainData, testData, trainLabels, testLabels) = train_test_split( data, labels, test_size=0.25, random_state=42) # construct the set of hyperparameters to tune params = {"n_neighbors": np.arange(1, 31, 2), "metric": ["euclidean", "cityblock"]}
The above code block defines a
paramsdictionary which contains two keys:
-
n_neighbors
: The number of nearest neighbors k in the k-NN algorithm. Here we’ll search over the odd integers in the range [0, 29] (keep in mind that thenp.arange
function is exclusive). -
metric
: This is the distance function/similarity metric for k-NN. Normally this defaults to the Euclidean distance, but we could also use any function that returns a single floating point value representing how “similar” two images are. In this case, we’ll search over both the Euclidean distance and Manhattan/City block distance.
Now that we have defined the hyperparameters we want to search over, we need a method that actually applies the search. Luckily, the scikit-learn library already has two methods that can perform hyperparameter search for us: Grid Search and Randomized Search.
As we’ll find out, it’s normally preferable to used Randomized Search over Grid Search in nearly all circumstances.
Grid Search hyperparameters
The Grid Search tuning algorithm will methodically (and exhaustively) train and evaluate a machine learning classifier for each and every combination of hyperparameter values.
In this case, given 16 unique values of k and 2 unique values for our distance metric, a Grid Search will apply 30 different experiments to determine the optimal value.
You can see how a Grid Search is performed in the following code segment:
# import the necessary packages from sklearn.neighbors import KNeighborsClassifier from sklearn.grid_search import RandomizedSearchCV from sklearn.grid_search import GridSearchCV from sklearn.cross_validation import train_test_split from imutils import paths import numpy as np import argparse import imutils import time import cv2 import os def extract_color_histogram(image, bins=(8, 8, 8)): # extract a 3D color histogram from the HSV color space using # the supplied number of `bins` per channel hsv = cv2.cvtColor(image, cv2.COLOR_BGR2HSV) hist = cv2.calcHist([hsv], [0, 1, 2], None, bins, [0, 180, 0, 256, 0, 256]) # handle normalizing the histogram if we are using OpenCV 2.4.X if imutils.is_cv2(): hist = cv2.normalize(hist) # otherwise, perform "in place" normalization in OpenCV 3 (I # personally hate the way this is done else: cv2.normalize(hist, hist) # return the flattened histogram as the feature vector return hist.flatten() # construct the argument parse and parse the arguments ap = argparse.ArgumentParser() ap.add_argument("-d", "--dataset", required=True, help="path to input dataset") ap.add_argument("-j", "--jobs", type=int, default=-1, help="# of jobs for k-NN distance (-1 uses all available cores)") args = vars(ap.parse_args()) # grab the list of images that we'll be describing print("[INFO] describing images...") imagePaths = list(paths.list_images(args["dataset"])) # initialize the data matrix and labels list data = [] labels = [] # loop over the input images for (i, imagePath) in enumerate(imagePaths): # load the image and extract the class label (assuming that our # path as the format: /path/to/dataset/{class}.{image_num}.jpg image = cv2.imread(imagePath) label = imagePath.split(os.path.sep)[-1].split(".")[0] # extract a color histogram from the image, then update the # data matrix and labels list hist = extract_color_histogram(image) data.append(hist) labels.append(label) # show an update every 1,000 images if i > 0 and i % 1000 == 0: print("[INFO] processed {}/{}".format(i, len(imagePaths))) # partition the data into training and testing splits, using 75% # of the data for training and the remaining 25% for testing print("[INFO] constructing training/testing split...") (trainData, testData, trainLabels, testLabels) = train_test_split( data, labels, test_size=0.25, random_state=42) # construct the set of hyperparameters to tune params = {"n_neighbors": np.arange(1, 31, 2), "metric": ["euclidean", "cityblock"]} # tune the hyperparameters via a cross-validated grid search print("[INFO] tuning hyperparameters via grid search") model = KNeighborsClassifier(n_jobs=args["jobs"]) grid = GridSearchCV(model, params) start = time.time() grid.fit(trainData, trainLabels) # evaluate the best grid searched model on the testing data print("[INFO] grid search took {:.2f} seconds".format( time.time() - start)) acc = grid.score(testData, testLabels) print("[INFO] grid search accuracy: {:.2f}%".format(acc * 100)) print("[INFO] grid search best parameters: {}".format( grid.best_params_))
The primary benefit of the Grid Search algorithm is also it’s major drawback: as an exhaustive search your number of possible parameter values explodes as both the number of hyperparameters and hyperparameter values increases.
Sure, you get to evaluate each and every combination of hyperparameter — but you pay a cost — it’s a very time consuming cost. And in most cases, it’s hardly worth it.
As I explain in the “Use Randomized Search for hyperparameter tuning (in most situations)” section below, there are rarely just one set of hyperparameters that obtain the highest accuracy.
Instead, there are “hot zones” of hyperparameters that all obtain near identical accuracy. The goal is to explore as many of these “zones” of hyperparameters a quickly as possible and locate one of these “hot zones”. It turns out that a random search is a great way to do this.
Randomized Search hyperparameters
The Random Search approach to hyperparameter tuning will sample hyperparameters from our
paramsdictionary via a random, uniform distribution. Given a set of randomly sampled parameters, a model is then trained and evaluated.
We perform this set of random hyperparameter sampling and model construction/evaluation for a preset number of times. You set the number of evaluations to be as long as you’re willing to wait. If you’re impatient and in a hurry, make this value low. And if you have the time to spend on a longer experiment, increase the number of iterations.
In either case, the goal of a Randomized Search is to explore a large set of possible hyperparameter spaces quickly — and the best way to accomplish this is via simple random sampling. And in practice, it works quite well!
You can find the code to perform a Randomized Search of hyperparameters for the k-NN algorithm below:
# import the necessary packages from sklearn.neighbors import KNeighborsClassifier from sklearn.grid_search import RandomizedSearchCV from sklearn.grid_search import GridSearchCV from sklearn.cross_validation import train_test_split from imutils import paths import numpy as np import argparse import imutils import time import cv2 import os def extract_color_histogram(image, bins=(8, 8, 8)): # extract a 3D color histogram from the HSV color space using # the supplied number of `bins` per channel hsv = cv2.cvtColor(image, cv2.COLOR_BGR2HSV) hist = cv2.calcHist([hsv], [0, 1, 2], None, bins, [0, 180, 0, 256, 0, 256]) # handle normalizing the histogram if we are using OpenCV 2.4.X if imutils.is_cv2(): hist = cv2.normalize(hist) # otherwise, perform "in place" normalization in OpenCV 3 (I # personally hate the way this is done else: cv2.normalize(hist, hist) # return the flattened histogram as the feature vector return hist.flatten() # construct the argument parse and parse the arguments ap = argparse.ArgumentParser() ap.add_argument("-d", "--dataset", required=True, help="path to input dataset") ap.add_argument("-j", "--jobs", type=int, default=-1, help="# of jobs for k-NN distance (-1 uses all available cores)") args = vars(ap.parse_args()) # grab the list of images that we'll be describing print("[INFO] describing images...") imagePaths = list(paths.list_images(args["dataset"])) # initialize the data matrix and labels list data = [] labels = [] # loop over the input images for (i, imagePath) in enumerate(imagePaths): # load the image and extract the class label (assuming that our # path as the format: /path/to/dataset/{class}.{image_num}.jpg image = cv2.imread(imagePath) label = imagePath.split(os.path.sep)[-1].split(".")[0] # extract a color histogram from the image, then update the # data matrix and labels list hist = extract_color_histogram(image) data.append(hist) labels.append(label) # show an update every 1,000 images if i > 0 and i % 1000 == 0: print("[INFO] processed {}/{}".format(i, len(imagePaths))) # partition the data into training and testing splits, using 75% # of the data for training and the remaining 25% for testing print("[INFO] constructing training/testing split...") (trainData, testData, trainLabels, testLabels) = train_test_split( data, labels, test_size=0.25, random_state=42) # construct the set of hyperparameters to tune params = {"n_neighbors": np.arange(1, 31, 2), "metric": ["euclidean", "cityblock"]} # tune the hyperparameters via a cross-validated grid search print("[INFO] tuning hyperparameters via grid search") model = KNeighborsClassifier(n_jobs=args["jobs"]) grid = GridSearchCV(model, params) start = time.time() grid.fit(trainData, trainLabels) # evaluate the best grid searched model on the testing data print("[INFO] grid search took {:.2f} seconds".format( time.time() - start)) acc = grid.score(testData, testLabels) print("[INFO] grid search accuracy: {:.2f}%".format(acc * 100)) print("[INFO] grid search best parameters: {}".format( grid.best_params_)) # tune the hyperparameters via a randomized search grid = RandomizedSearchCV(model, params) start = time.time() grid.fit(trainData, trainLabels) # evaluate the best randomized searched model on the testing # data print("[INFO] randomized search took {:.2f} seconds".format( time.time() - start)) acc = grid.score(testData, testLabels) print("[INFO] grid search accuracy: {:.2f}%".format(acc * 100)) print("[INFO] randomized search best parameters: {}".format( grid.best_params_))
Hyperparameter tuning with Python and scikit-learn results
To tune the hyperparameters of our k-NN algorithm, make sure you:
- Download the source code to this tutorial using the “Downloads” form at the bottom of this post.
- Head over to the Kaggle Dogs vs. Cats competition page and download the dataset.
From there, you can execute the following command to tune the hyperparameters:
$ python knn_tune.py --dataset kaggle_dogs_vs_cats
You’ll probably want to go for a nice walk and stretch your legs will the
knn_tune.pyscript executes.
On my machine, it took 19m 26s to complete, with over 86% of this time spent Grid Searching:
Figure 2: Applying a Grid Search and Randomized to tune machine learning hyperparameters using Python and scikit-learn.
As you can see from the output screenshot, the Grid Search method found that k=25 and metric=’cityblock’ obtained the highest accuracy of 64.03%. However, this Grid Search took 13 minutes.
On the other hand, the Randomized Search obtained an identical accuracy of 64.03% — and it completed in under 5 minutes.
Both of these hyperparameter tuning methods improved our classification accuracy (64.03% accuracy, up from 57.58% from last week’s post) — but the Randomized Search was much more efficient.
Use Randomized Search for hyperparameter tuning (in most situations)
Unless your search space is small and can easily be enumerated, a Randomized Search will tend to be more efficient and yield better results faster.
As our experiments demonstrated, Randomized Search was able to obtain 64.03% accuracy in < 5 minutes while an exhaustive Grid Search took a much longer 13 minutes to obtain an identical 64.03% accuracy — that’s a 202% increase in evaluation time for identical accuracy!
In general, there isn’t just one set of hyperparameters that obtains optimal results — instead, there are usually a set of them that exist towards the bottom of a concave bowl (i.e., the optimization surface).
As long as you hit just one of these parameters towards the bottom of the bowl, you’ll still obtain the same accuracy as if you enumerated all possibilities along the bowl. Furthermore, you’ll be able to explore various regions of this bowl faster by applying a Randomized Search.
Overall, this will lead to faster, more efficient hyperparameter tunings in most situations.
Summary
In today’s blog post, I demonstrated how to tune hyperparameters to machine learning algorithms using the Python programming language and the scikit-learn library.
First, I defined, the difference between standard “model parameters” and the “hyperparameters” that need to be tuned.
From there, we applied two methods to tune hyperparameters:
- An exhaustive Grid Search
- A Randomized Search
Both of these hyperparameter tuning routines were then applied to the k-NN algorithm and the Kaggle Dogs vs. Cats dataset.
Each respective tuning algorithm obtained identical accuracy — but the Randomized Search was able to obtain this increase of accuracy in a fraction of the time!
In general, I highly encourage you to use Randomized Search when tuning hyperparameters. You’ll often find that there is rarely just one set of hyperparameters that obtains optimal accuracy. Instead, there are “hot zones” of hyperparameters that will obtain near-identical accuracy — the goal is to explore as many zones and try to land on one of these zones as fast as possible.
Given no a priori knowledge of good hyperparameter choices, a Randomized Search to hyperparameter tuning is the most optimal way to find reasonable hyperparameter values in a short amount of time as it allows you to explore many areas of the optimization surface.
Anyway, I hope you enjoyed this blog post! I’ll be back next week to discuss the basics of linear classification (and the role it plays in Neural Networks and image classification).
But before you go, be sure to signup for the PyImageSearch Newsletter using the form below to be notified when future blog posts are published!
Downloads:
The post How to tune hyperparameters with Python and scikit-learn appeared first on PyImageSearch.
from PyImageSearch http://ift.tt/2aNY1gk
via IFTTT