Latest YouTube Video
Saturday, March 18, 2017
Ravens: Free agent King Dunlap might end up being good, cheap fit at RT despite struggles in pass protection, age - Jamison Hensley (ESPN)
via IFTTT
Anonymous (Original Mix)
from Google Alert - anonymous http://ift.tt/2nCZ6uy
via IFTTT
Museo Vigili del Fuoco Mantova
from Google Alert - anonymous http://ift.tt/2n8Rm5o
via IFTTT
WikiLeaks Won't Disclose CIA Exploits To Companies Until Certain Demands Are Met
from The Hacker News http://ift.tt/2mCOhqw
via IFTTT
[FD] TS Session Hijacking / Privilege escalation all windows versions
Source: Gmail -> IFTTT-> Blogger
[FD] [CVE-2017-6878]:MetInfo5.3.15 Stored Cross Site Scripting
Source: Gmail -> IFTTT-> Blogger
Gamblers Anonymous
from Google Alert - anonymous http://ift.tt/2mZOEyK
via IFTTT
Phases of Venus
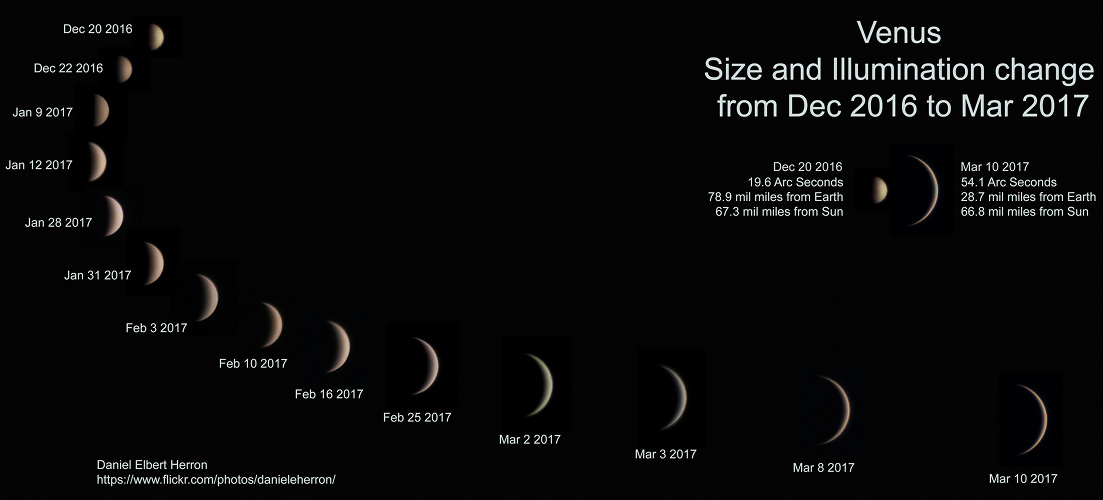
Friday, March 17, 2017
Ravens: Brandon Carr signing \"stabilizes a cornerback position that has been in flux for years\" - Jamison Hensley (ESPN)
via IFTTT
Prevent anonymous callers on OpenVoice conferences
from Google Alert - anonymous http://ift.tt/2nhT4SK
via IFTTT
I have a new follower on Twitter
Randolph Paper
Free Delivery, No Minimum Order, Next Day Delivery in 70% of the U.S.A. Carbonless, Specialty Papers, and 20yrs Experience for You, the Customer. 800-752-2339
USA
https://t.co/iOUMfWDp3L
Following: 1362 - Followers: 1466
March 17, 2017 at 01:12PM via Twitter http://twitter.com/RandolphNext
Orioles release P Logan Ondrusek, who was due to have his elbow examined next week by Dr. James Andrews (ESPN)
via IFTTT
ISS Daily Summary Report – 3/16/2017
from ISS On-Orbit Status Report http://ift.tt/2mzIvGg
via IFTTT
[FD] HumHub 0.20.1 / 1.0.0-beta.3: Code Execution
Source: Gmail -> IFTTT-> Blogger
[FD] HumHub 1.0.1: XSS
Source: Gmail -> IFTTT-> Blogger
[FD] phplist 3.2.6: XSS
Source: Gmail -> IFTTT-> Blogger
[FD] phplist 3.2.6: SQL Injection
Source: Gmail -> IFTTT-> Blogger
NCIS: Hidden Crimes [hack]
from Google Alert - anonymous http://ift.tt/2ngzGp5
via IFTTT
Museo Vigili del Fuoco Mantova
from Google Alert - anonymous http://ift.tt/2mz95za
via IFTTT
I have a new follower on Twitter
4dable Advertising
Over @4dabledotcom some say we're business experts who support, others say we're #MicroInfluencers promoting the best business. #worktogethergrowtogether✌🏻️
Somewhere around the Milky Way
https://t.co/ybCWCraPaR
Following: 8061 - Followers: 8182
March 17, 2017 at 04:32AM via Twitter http://twitter.com/4dable
Sony Is Working On Mobile-to-Mobile Wireless Charging Technology
from The Hacker News http://ift.tt/2mB45uI
via IFTTT
The Simpson Tapped Out (cheat)
from Google Alert - anonymous http://ift.tt/2m8HREk
via IFTTT
I have a new follower on Twitter
Ramesh Padala
Founder @flexheroapp, @yogatailor, ex @Saavn, Meditator,Yogi,Chai Maker, Stanford, Angel , Advisor, Snowboarder, HuffPost Writer & Poop Picker / @akirathedoggie
Palo Alto, CA
https://t.co/9RF920cuOE
Following: 10456 - Followers: 9520
March 17, 2017 at 03:42AM via Twitter http://twitter.com/rameshdot0
I have a new follower on Twitter
Freedym
Freedym: Netflix for Online Entrepreneurs. 1,000+ hours of training for both startups & business owners, private mastermind group & more. Get Instant Access!
https://t.co/27Djbas3RN
Following: 5493 - Followers: 6357
March 17, 2017 at 03:42AM via Twitter http://twitter.com/FreedymFan
Mimas in Saturnlight
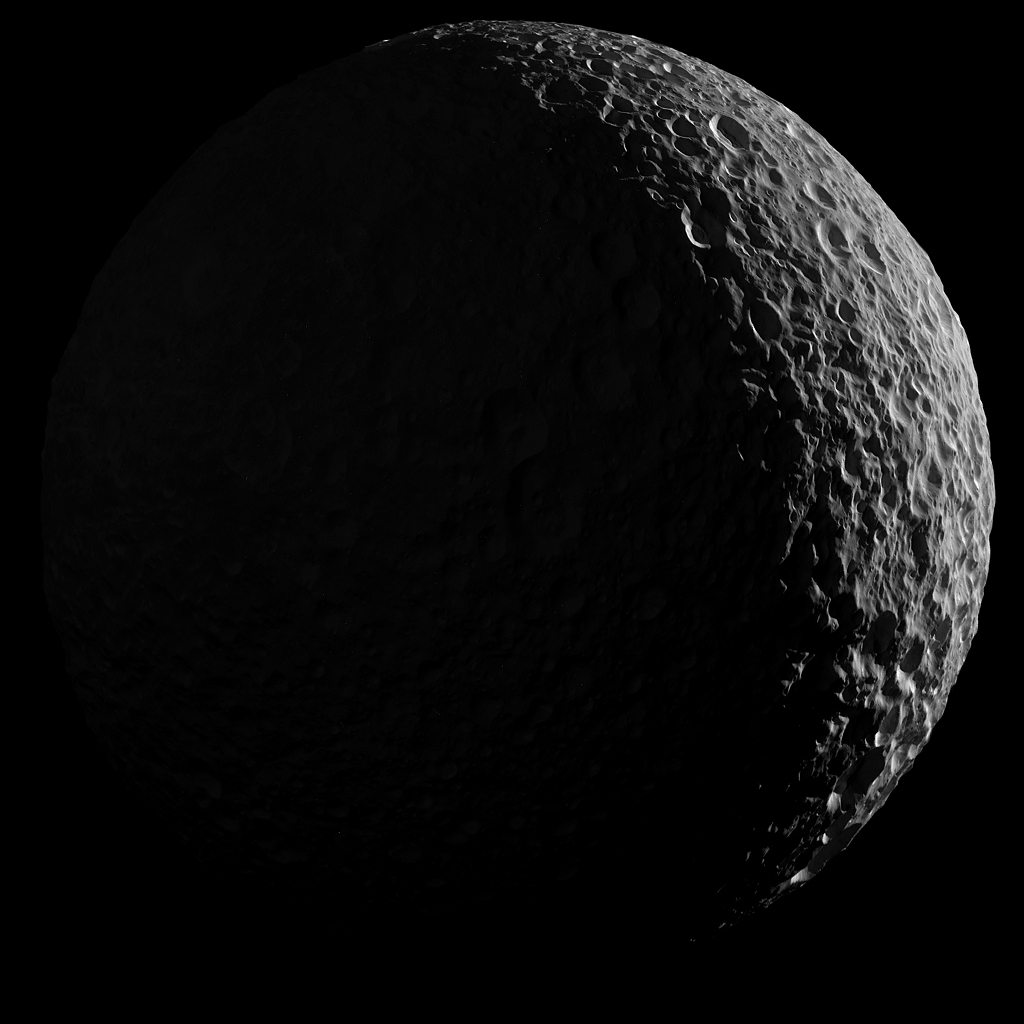
Rumors: Anonymous Warriors player says the team has problems
from Google Alert - anonymous http://ift.tt/2mATDn7
via IFTTT
Our Retail Brands
from Google Alert - anonymous http://ift.tt/2m8EQUl
via IFTTT
Museo Vigili del Fuoco Mantova
from Google Alert - anonymous http://ift.tt/2mB1GQH
via IFTTT
Book Review: The Lean Startup
from Google Alert - anonymous http://ift.tt/2m8CLYn
via IFTTT
Thursday, March 16, 2017
Parameterize function without anonymous or nested functions
from Google Alert - anonymous http://ift.tt/2m7OlDj
via IFTTT
Legal Question Answering using Ranking SVM and Deep Convolutional Neural Network. (arXiv:1703.05320v1 [cs.CL])
This paper presents a study of employing Ranking SVM and Convolutional Neural Network for two missions: legal information retrieval and question answering in the Competition on Legal Information Extraction/Entailment. For the first task, our proposed model used a triple of features (LSI, Manhattan, Jaccard), and is based on paragraph level instead of article level as in previous studies. In fact, each single-paragraph article corresponds to a particular paragraph in a huge multiple-paragraph article. For the legal question answering task, additional statistical features from information retrieval task integrated into Convolutional Neural Network contribute to higher accuracy.
from cs.AI updates on arXiv.org http://ift.tt/2m7G962
via IFTTT
Concentration Bounds for Two Timescale Stochastic Approximation with Applications to Reinforcement Learning. (arXiv:1703.05376v1 [cs.AI])
Two-timescale Stochastic Approximation (SA) algorithms are widely used in Reinforcement Learning (RL). In such methods, the iterates consist of two parts that are updated using different stepsizes. We develop the first convergence rate result for these algorithms; in particular, we provide a general methodology for analyzing two-timescale linear SA. We apply our methodology to two-timescale RL algorithms such as GTD(0), GTD2, and TDC.
from cs.AI updates on arXiv.org http://ift.tt/2mOxFO5
via IFTTT
Convolutional Recurrent Neural Networks for Small-Footprint Keyword Spotting. (arXiv:1703.05390v1 [cs.CL])
Keyword spotting (KWS) constitutes a major component of human-technology interfaces. Maximizing the detection accuracy at a low false alarm (FA) rate, while minimizing the footprint size, latency and complexity are the goals for KWS. Towards achieving them, we study Convolutional Recurrent Neural Networks (CRNNs). Inspired by large-scale state-of-the-art speech recognition systems, we combine the strengths of convolutional layers and recurrent layers to exploit local structure and long-range context. We analyze the effect of architecture parameters, and propose training strategies to improve performance. With only ~230k parameters, our CRNN model yields acceptably low latency, and achieves 97.71% accuracy at 0.5 FA/hour for 5 dB signal-to-noise ratio.
from cs.AI updates on arXiv.org http://ift.tt/2mzWnkw
via IFTTT
Look into Person: Self-supervised Structure-sensitive Learning and A New Benchmark for Human Parsing. (arXiv:1703.05446v1 [cs.CV])
Human parsing has recently attracted a lot of research interests due to its huge application potentials. However existing datasets have limited number of images and annotations, and lack the variety of human appearances and the coverage of challenging cases in unconstrained environment. In this paper, we introduce a new benchmark "Look into Person (LIP)" that makes a significant advance in terms of scalability, diversity and difficulty, a contribution that we feel is crucial for future developments in human-centric analysis. This comprehensive dataset contains over 50,000 elaborately annotated images with 19 semantic part labels, which are captured from a wider range of viewpoints, occlusions and background complexity. Given these rich annotations we perform detailed analyses of the leading human parsing approaches, gaining insights into the success and failures of these methods. Furthermore, in contrast to the existing efforts on improving the feature discriminative capability, we solve human parsing by exploring a novel self-supervised structure-sensitive learning approach, which imposes human pose structures into parsing results without resorting to extra supervision (i.e., no need for specifically labeling human joints in model training). Our self-supervised learning framework can be injected into any advanced neural networks to help incorporate rich high-level knowledge regarding human joints from a global perspective and improve the parsing results. Extensive evaluations on our LIP and the public PASCAL-Person-Part dataset demonstrate the superiority of our method.
from cs.AI updates on arXiv.org http://ift.tt/2nf5sCI
via IFTTT
Minimax Regret Bounds for Reinforcement Learning. (arXiv:1703.05449v1 [stat.ML])
We consider the problem of efficient exploration in finite horizon MDPs.We show that an optimistic modification to model-based value iteration, can achieve a regret bound $\tilde{O}( \sqrt{HSAT} + H^2S^2A+H\sqrt{T})$ where $H$ is the time horizon, $S$ the number of states, $A$ the number of actions and $T$ the time elapsed. This result improves over the best previous known bound $\tilde{O}(HS \sqrt{AT})$ achieved by the UCRL2 algorithm.The key significance of our new results is that when $T\geq H^3S^3A$ and $SA\geq H$, it leads to a regret of $\tilde{O}(\sqrt{HSAT})$ that matches the established lower bounds of $\Omega(\sqrt{HSAT})$ up to a logarithmic factor. Our analysis contain two key insights. We use careful application of concentration inequalities to the optimal value function as a whole, rather than to the transitions probabilities (to improve scaling in $S$), and we use "exploration bonuses" based on Bernstein's inequality, together with using a recursive -Bellman-type- Law of Total Variance (to improve scaling in $H$).
from cs.AI updates on arXiv.org http://ift.tt/2nxwaEf
via IFTTT
Efficient Online Learning for Optimizing Value of Information: Theory and Application to Interactive Troubleshooting. (arXiv:1703.05452v1 [cs.AI])
We consider the optimal value of information (VoI) problem, where the goal is to sequentially select a set of tests with a minimal cost, so that one can efficiently make the best decision based on the observed outcomes. Existing algorithms are either heuristics with no guarantees, or scale poorly (with exponential run time in terms of the number of available tests). Moreover, these methods assume a known distribution over the test outcomes, which is often not the case in practice. We propose an efficient sampling-based online learning framework to address the above issues. First, assuming the distribution over hypotheses is known, we propose a dynamic hypothesis enumeration strategy, which allows efficient information gathering with strong theoretical guarantees. We show that with sufficient amount of samples, one can identify a near-optimal decision with high probability. Second, when the parameters of the hypotheses distribution are unknown, we propose an algorithm which learns the parameters progressively via posterior sampling in an online fashion. We further establish a rigorous bound on the expected regret. We demonstrate the effectiveness of our approach on a real-world interactive troubleshooting application and show that one can efficiently make high-quality decisions with low cost.
from cs.AI updates on arXiv.org http://ift.tt/2nfkdW7
via IFTTT
Database Learning: Toward a Database that Becomes Smarter Every Time. (arXiv:1703.05468v1 [cs.DB])
In today's databases, previous query answers rarely benefit answering future queries. For the first time, to the best of our knowledge, we change this paradigm in an approximate query processing (AQP) context. We make the following observation: the answer to each query reveals some degree of knowledge about the answer to another query because their answers stem from the same underlying distribution that has produced the entire dataset. Exploiting and refining this knowledge should allow us to answer queries more analytically, rather than by reading enormous amounts of raw data. Also, processing more queries should continuously enhance our knowledge of the underlying distribution, and hence lead to increasingly faster response times for future queries.
We call this novel idea---learning from past query answers---Database Learning. We exploit the principle of maximum entropy to produce answers, which are in expectation guaranteed to be more accurate than existing sample-based approximations. Empowered by this idea, we build a query engine on top of Spark SQL, called Verdict. We conduct extensive experiments on real-world query traces from a large customer of a major database vendor. Our results demonstrate that database learning supports 73.7% of these queries, speeding them up by up to 23.0x for the same accuracy level compared to existing AQP systems.
from cs.AI updates on arXiv.org http://ift.tt/2mOCCX6
via IFTTT
ParaGraphE: A Library for Parallel Knowledge Graph Embedding. (arXiv:1703.05614v1 [cs.AI])
Knowledge graph embedding aims at translating the knowledge graph into numerical representations by transforming the entities and relations into con- tinuous low-dimensional vectors. Recently, many methods [1, 5, 3, 2, 6] have been proposed to deal with this problem, but existing single-thread implemen- tations of them are time-consuming for large-scale knowledge graphs. Here, we design a unified parallel framework to parallelize these methods, which achieves a significant time reduction without in uencing the accuracy. We name our framework as ParaGraphE, which provides a library for parallel knowledge graph embedding. The source code can be downloaded from https: //github.com/LIBBLE/LIBBLE-MultiThread/tree/master/ParaGraphE.
from cs.AI updates on arXiv.org http://ift.tt/2nfbL9q
via IFTTT
Semantic Change Detection with Hypermaps. (arXiv:1604.07513v2 [cs.CV] UPDATED)
Change detection is the study of detecting changes between two different images of a scene taken at different times. By the detected change areas, however, a human cannot understand how different the two images. Therefore, a semantic understanding is required in the change detection research such as disaster investigation. The paper proposes the concept of semantic change detection, which involves intuitively inserting semantic meaning into detected change areas. We mainly focus on the novel semantic segmentation in addition to a conventional change detection approach. In order to solve this problem and obtain a high-level of performance, we propose an improvement to the hypercolumns representation, hereafter known as hypermaps, which effectively uses convolutional maps obtained from convolutional neural networks (CNNs). We also employ multi-scale feature representation captured by different image patches. We applied our method to the TSUNAMI Panoramic Change Detection dataset, and re-annotated the changed areas of the dataset via semantic classes. The results show that our multi-scale hypermaps provided outstanding performance on the re-annotated TSUNAMI dataset.
from cs.AI updates on arXiv.org http://ift.tt/24iDm62
via IFTTT
Improving Policy Gradient by Exploring Under-appreciated Rewards. (arXiv:1611.09321v3 [cs.LG] UPDATED)
This paper presents a novel form of policy gradient for model-free reinforcement learning (RL) with improved exploration properties. Current policy-based methods use entropy regularization to encourage undirected exploration of the reward landscape, which is ineffective in high dimensional spaces with sparse rewards. We propose a more directed exploration strategy that promotes exploration of under-appreciated reward regions. An action sequence is considered under-appreciated if its log-probability under the current policy under-estimates its resulting reward. The proposed exploration strategy is easy to implement, requiring small modifications to an implementation of the REINFORCE algorithm. We evaluate the approach on a set of algorithmic tasks that have long challenged RL methods. Our approach reduces hyper-parameter sensitivity and demonstrates significant improvements over baseline methods. Our algorithm successfully solves a benchmark multi-digit addition task and generalizes to long sequences. This is, to our knowledge, the first time that a pure RL method has solved addition using only reward feedback.
from cs.AI updates on arXiv.org http://ift.tt/2gA9tyq
via IFTTT
Optimal Detection of Faulty Traffic Sensors Used in Route Planning. (arXiv:1702.02628v2 [cs.AI] UPDATED)
In a smart city, real-time traffic sensors may be deployed for various applications, such as route planning. Unfortunately, sensors are prone to failures, which result in erroneous traffic data. Erroneous data can adversely affect applications such as route planning, and can cause increased travel time. To minimize the impact of sensor failures, we must detect them promptly and accurately. However, typical detection algorithms may lead to a large number of false positives (i.e., false alarms) and false negatives (i.e., missed detections), which can result in suboptimal route planning. In this paper, we devise an effective detector for identifying faulty traffic sensors using a prediction model based on Gaussian Processes. Further, we present an approach for computing the optimal parameters of the detector which minimize losses due to false-positive and false-negative errors. We also characterize critical sensors, whose failure can have high impact on the route planning application. Finally, we implement our method and evaluate it numerically using a real-world dataset and the route planning platform OpenTripPlanner.
from cs.AI updates on arXiv.org http://ift.tt/2kUgRHa
via IFTTT
Asymmetric Tri-training for Unsupervised Domain Adaptation. (arXiv:1702.08400v2 [cs.CV] UPDATED)
Deep-layered models trained on a large number of labeled samples boost the accuracy of many tasks. It is important to apply such models to different domains because collecting many labeled samples in various domains is expensive. In unsupervised domain adaptation, one needs to train a classifier that works well on a target domain when provided with labeled source samples and unlabeled target samples. Although many methods aim to match the distributions of source and target samples, simply matching the distribution cannot ensure accuracy on the target domain. To learn discriminative representations for the target domain, we assume that artificially labeling target samples can result in a good representation. Tri-training leverages three classifiers equally to give pseudo-labels to unlabeled samples, but the method does not assume labeling samples generated from a different domain.In this paper, we propose an asymmetric tri-training method for unsupervised domain adaptation, where we assign pseudo-labels to unlabeled samples and train neural networks as if they are true labels. In our work, we use three networks asymmetrically. By asymmetric, we mean that two networks are used to label unlabeled target samples and one network is trained by the samples to obtain target-discriminative representations. We evaluate our method on digit recognition and sentiment analysis datasets. Our proposed method achieves state-of-the-art performance on the benchmark digit recognition datasets of domain adaptation.
from cs.AI updates on arXiv.org http://ift.tt/2mEPLke
via IFTTT
Entreprenuers Anonymous
from Google Alert - anonymous http://ift.tt/2nKRpRX
via IFTTT
Overeaters Anonymous (OA) Newcomers Weekly Meeting
from Google Alert - anonymous http://ift.tt/2nKpDoG
via IFTTT
DG Comp encourages anonymous tip-offs
from Google Alert - anonymous http://ift.tt/2nsYf2H
via IFTTT
Heretofore Anonymous Reservoir Becomes Latest Crack in California's Water System
from Google Alert - anonymous http://ift.tt/2nKxliI
via IFTTT
Anonymous Jobs
from Google Alert - anonymous http://ift.tt/2nsZD5n
via IFTTT
Display help text to anonymous
from Google Alert - anonymous http://ift.tt/2nKtgex
via IFTTT
Greater Atlanta Overeaters Anonymous Convention
from Google Alert - anonymous http://ift.tt/2nt4sLI
via IFTTT
Presenting a anonymous call with CallKit
from Google Alert - anonymous http://ift.tt/2nKGM1D
via IFTTT
[FD] Skype Insecure Library Loading Vulnerability (api-ms-win-core-winrt-string-l1-1-0.dll)
Source: Gmail -> IFTTT-> Blogger
[FD] USB Pratirodh Insecure Password Storage Information Disclosure Vulnerability
Source: Gmail -> IFTTT-> Blogger
[FD] Axis Camera Multiple Vulnerabilities
Source: Gmail -> IFTTT-> Blogger
[FD] Microsoft Windows "LoadUvsTable()" Buffer Overflow Vulnerability
Source: Gmail -> IFTTT-> Blogger
Ravens receive B+ grade for free agency moves; \"bolstered their defense with two major investments\" - Jamison Hensley (ESPN)
via IFTTT
Linux Kernel Gets Patch For Years-Old Serious Vulnerability
from The Hacker News http://ift.tt/2nw4eR6
via IFTTT
ISS Daily Summary Report – 3/15/2017
from ISS On-Orbit Status Report http://ift.tt/2mMTkpW
via IFTTT
[FD] SEC Consult SA-20170316-0 :: Authenticated command injection in multiple Ubiquiti Networks products
Source: Gmail -> IFTTT-> Blogger
Yahoo! Hack! How It Took Just One-Click to Execute Biggest Data Breach in History
from The Hacker News http://ift.tt/2mMdVe6
via IFTTT
The Cone Nebula from Hubble
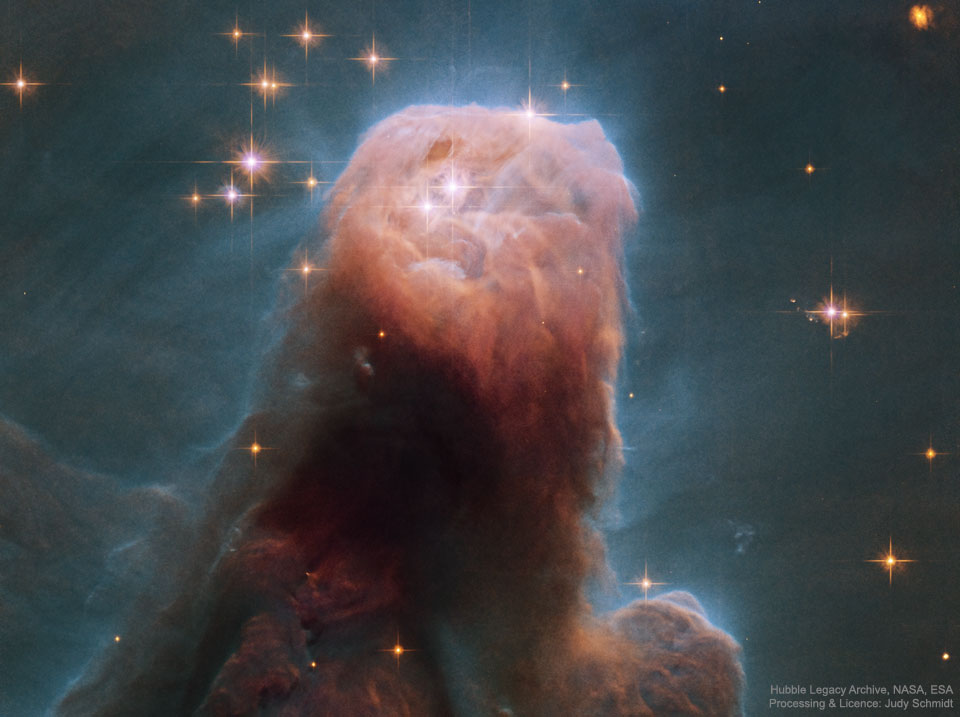
It's Fappening Again! Private Photos of Emma Watson and Others Leaked Online
from The Hacker News http://ift.tt/2nuGXyL
via IFTTT
Leaky Radiation Belts
from NASA's Scientific Visualization Studio: Most Recent Items http://ift.tt/2muUUuK
via IFTTT
Wednesday, March 15, 2017
Anonymous hack instagram
from Google Alert - anonymous http://ift.tt/2mQrjiV
via IFTTT
Fuzzy Model Tree For Early Effort Estimation. (arXiv:1703.04565v1 [cs.SE])
Use Case Points (UCP) is a well-known method to estimate the project size, based on Use Case diagram, at early phases of software development. Although the Use Case diagram is widely accepted as a de-facto model for analyzing object oriented software requirements over the world, UCP method did not take sufficient amount of attention because, as yet, there is no consensus on how to produce software effort from UCP. This paper aims to study the potential of using Fuzzy Model Tree to derive effort estimates ...
from cs.AI updates on arXiv.org http://ift.tt/2m24KZI
via IFTTT
Learning best K analogies from data distribution for case-based software effort estimation. (arXiv:1703.04567v1 [cs.SE])
Case-Based Reasoning (CBR) has been widely used to generate good software effort estimates. The predictive performance of CBR is a dataset dependent and subject to extremely large space of configuration possibilities. Regardless of the type of adaptation technique, deciding on the optimal number of similar cases to be used before applying CBR is a key challenge. In this paper we propose a new technique based on Bisecting k-medoids clustering algorithm to better understanding the structure of a dataset and discovering the ...
from cs.AI updates on arXiv.org http://ift.tt/2m27bvx
via IFTTT
Minimizing Maximum Regret in Commitment Constrained Sequential Decision Making. (arXiv:1703.04587v1 [cs.AI])
In cooperative multiagent planning, it can often be beneficial for an agent to make commitments about aspects of its behavior to others, allowing them in turn to plan their own behaviors without taking the agent's detailed behavior into account. Extending previous work in the Bayesian setting, we consider instead a worst-case setting in which the agent has a set of possible environments (MDPs) it could be in, and develop a commitment semantics that allows for probabilistic guarantees on the agent's behavior in any of the environments it could end up facing. Crucially, an agent receives observations (of reward and state transitions) that allow it to potentially eliminate possible environments and thus obtain higher utility by adapting its policy to the history of observations. We develop algorithms and provide theory and some preliminary empirical results showing that they ensure an agent meets its commitments with history-dependent policies while minimizing maximum regret over the possible environments.
from cs.AI updates on arXiv.org http://ift.tt/2nGxi7p
via IFTTT
A computational investigation of sources of variability in sentence comprehension difficulty in aphasia. (arXiv:1703.04677v1 [cs.CL])
We present a computational evaluation of three hypotheses about sources of deficit in sentence comprehension in aphasia: slowed processing, intermittent deficiency, and resource reduction. The ACT-R based Lewis & Vasishth 2005 model is used to implement these three proposals. Slowed processing is implemented as slowed default production-rule firing time; intermittent deficiency as increased random noise in activation of chunks in memory; and resource reduction as reduced goal activation. As data, we considered subject vs. object relatives presented in a self-paced listening modality to 56 individuals with aphasia (IWA) and 46 matched controls. The participants heard the sentences and carried out a picture verification task to decide on an interpretation of the sentence. These response accuracies are used to identify the best parameters (for each participant) that correspond to the three hypotheses mentioned above. We show that controls have more tightly clustered (less variable) parameter values than IWA; specifically, compared to controls, among IWA there are more individuals with low goal activations, high noise, and slow default action times. This suggests that (i) individual patients show differential amounts of deficit along the three dimensions of slowed processing, intermittent deficient, and resource reduction, (ii) overall, there is evidence for all three sources of deficit playing a role, and (iii) IWA have a more variable range of parameter values than controls. In sum, this study contributes a proof of concept of a quantitative implementation of, and evidence for, these three accounts of comprehension deficits in aphasia.
from cs.AI updates on arXiv.org http://ift.tt/2mQpv9E
via IFTTT
Understanding Black-box Predictions via Influence Functions. (arXiv:1703.04730v1 [stat.ML])
How can we explain the predictions of a black-box model? In this paper, we use influence functions -- a classic technique from robust statistics -- to trace a model's prediction through the learning algorithm and back to its training data, identifying the points most responsible for a given prediction. Applying ideas from second-order optimization, we scale up influence functions to modern machine learning settings and show that they can be applied to high-dimensional black-box models, even in non-convex and non-differentiable settings. We give a simple, efficient implementation that requires only oracle access to gradients and Hessian-vector products. On linear models and convolutional neural networks, we demonstrate that influence functions are useful for many different purposes: to understand model behavior, debug models and detect dataset errors, and even identify and exploit vulnerabilities to adversarial training-set attacks.
from cs.AI updates on arXiv.org http://ift.tt/2nGADDN
via IFTTT
Towards Moral Autonomous Systems. (arXiv:1703.04741v1 [cs.AI])
Both the ethics of autonomous systems and the problems of their technical implementation have by now been studied in some detail. Less attention has been given to the areas in which these two separate concerns meet. This paper, written by both philosophers and engineers of autonomous systems, addresses a number of issues in machine ethics that are located at precisely the intersection between ethics and engineering. We first discuss different approaches towards the conceptual design of autonomous systems and their implications on the ethics implementation in such systems. Then we examine problematic areas regarding the specification and verification of ethical behavior in autonomous systems, particularly with a view towards the requirements of future legislation. We discuss transparency and accountability issues that will be crucial for any future wide deployment of autonomous systems in society. Finally we consider the, often overlooked, possibility of intentional misuse of AI systems and the possible dangers arising out of deliberately unethical design, implementation, and use of autonomous robots.
from cs.AI updates on arXiv.org http://ift.tt/2mQoT40
via IFTTT
Weighted Voting Via No-Regret Learning. (arXiv:1703.04756v1 [cs.GT])
Voting systems typically treat all voters equally. We argue that perhaps they should not: Voters who have supported good choices in the past should be given higher weight than voters who have supported bad ones. To develop a formal framework for desirable weighting schemes, we draw on no-regret learning. Specifically, given a voting rule, we wish to design a weighting scheme such that applying the voting rule, with voters weighted by the scheme, leads to choices that are almost as good as those endorsed by the best voter in hindsight. We derive possibility and impossibility results for the existence of such weighting schemes, depending on whether the voting rule and the weighting scheme are deterministic or randomized, as well as on the social choice axioms satisfied by the voting rule.
from cs.AI updates on arXiv.org http://ift.tt/2nGp7YT
via IFTTT
FastQA: A Simple and Efficient Neural Architecture for Question Answering. (arXiv:1703.04816v1 [cs.CL])
Recent development of large-scale question answering (QA) datasets triggered a substantial amount of research into end-to-end neural architectures for QA. Increasingly complex systems have been conceived without comparison to a simpler neural baseline system that would justify their complexity. In this work, we propose a simple heuristic that guided the development of FastQA, an efficient end-to-end neural model for question answering that is very competitive with existing models. We further demonstrate, that an extended version (FastQAExt) achieves state-of-the-art results on recent benchmark datasets, namely SQuAD, NewsQA and MsMARCO, outperforming most existing models. However, we show that increasing the complexity of FastQA to FastQAExt does not yield any systematic improvements. We argue that the same holds true for most existing systems that are similar to FastQAExt. A manual analysis reveals that our proposed heuristic explains most predictions of our model, which indicates that modeling a simple heuristic is enough to achieve strong performance on extractive QA datasets. The overall strong performance of FastQA puts results of existing, more complex models into perspective.
from cs.AI updates on arXiv.org http://ift.tt/2mQFO6r
via IFTTT
Exploring the Combination Rules of D Numbers From a Perspective of Conflict Redistribution. (arXiv:1703.04862v1 [cs.AI])
Dempster-Shafer theory of evidence is widely applied to uncertainty modelling and knowledge reasoning because of its advantages in dealing with uncertain information. But some conditions or requirements, such as exclusiveness hypothesis and completeness constraint, limit the development and application of that theory to a large extend. To overcome the shortcomings and enhance its capability of representing the uncertainty, a novel model, called D numbers, has been proposed recently. However, many key issues, for example how to implement the combination of D numbers, remain unsolved. In the paper, we have explored the combination of D Numbers from a perspective of conflict redistribution, and proposed two combination rules being suitable for different situations for the fusion of two D numbers. The proposed combination rules can reduce to the classical Dempster's rule in Dempster-Shafer theory under a certain conditions. Numerical examples and discussion about the proposed rules are also given in the paper.
from cs.AI updates on arXiv.org http://ift.tt/2nGs7EM
via IFTTT
Emergence of Grounded Compositional Language in Multi-Agent Populations. (arXiv:1703.04908v1 [cs.AI])
By capturing statistical patterns in large corpora, machine learning has enabled significant advances in natural language processing, including in machine translation, question answering, and sentiment analysis. However, for agents to intelligently interact with humans, simply capturing the statistical patterns is insufficient. In this paper we investigate if, and how, grounded compositional language can emerge as a means to achieve goals in multi-agent populations. Towards this end, we propose a multi-agent learning environment and learning methods that bring about emergence of a basic compositional language. This language is represented as streams of abstract discrete symbols uttered by agents over time, but nonetheless has a coherent structure that possesses a defined vocabulary and syntax. We also observe emergence of non-verbal communication such as pointing and guiding when language communication is unavailable.
from cs.AI updates on arXiv.org http://ift.tt/2mQtcfp
via IFTTT
Syntax-Preserving Belief Change Operators for Logic Programs. (arXiv:1703.04912v1 [cs.AI])
Recent methods have adapted the well-established AGM and belief base frameworks for belief change to cover belief revision in logic programs. In this study here, we present two new sets of belief change operators for logic programs. They focus on preserving the explicit relationships expressed in the rules of a program, a feature that is missing in purely semantic approaches that consider programs only in their entirety. In particular, operators of the latter class fail to satisfy preservation and support, two important properties for belief change in logic programs required to ensure intuitive results.
We address this shortcoming of existing approaches by introducing partial meet and ensconcement constructions for logic program belief change, which allow us to define syntax-preserving operators that satisfy preservation and support. Our work is novel in that our constructions not only preserve more information from a logic program during a change operation than existing ones, but they also facilitate natural definitions of contraction operators, the first in the field to the best of our knowledge.
In order to evaluate the rationality of our operators, we translate the revision and contraction postulates from the AGM and belief base frameworks to the logic programming setting. We show that our operators fully comply with the belief base framework and formally state the interdefinability between our operators. We further propose an algorithm that is based on modularising a logic program to reduce partial meet and ensconcement revisions or contractions to performing the operation only on the relevant modules of that program. Finally, we compare our approach to two state-of-the-art logic program revision methods and demonstrate that our operators address the shortcomings of one and generalise the other method.
from cs.AI updates on arXiv.org http://ift.tt/2nGw0cO
via IFTTT
Resilience: A Criterion for Learning in the Presence of Arbitrary Outliers. (arXiv:1703.04940v1 [cs.LG])
We introduce a criterion, resilience, which allows properties of a dataset (such as its mean or best low rank approximation) to be robustly computed, even in the presence of a large fraction of arbitrary additional data. Resilience is a weaker condition than most other properties considered so far in the literature, and yet enables robust estimation in a broader variety of settings, including the previously unstudied problem of robust mean estimation in $\ell_p$-norms.
from cs.AI updates on arXiv.org http://ift.tt/2mQqd6J
via IFTTT
Neural Programming by Example. (arXiv:1703.04990v1 [cs.AI])
Programming by Example (PBE) targets at automatically inferring a computer program for accomplishing a certain task from sample input and output. In this paper, we propose a deep neural networks (DNN) based PBE model called Neural Programming by Example (NPBE), which can learn from input-output strings and induce programs that solve the string manipulation problems. Our NPBE model has four neural network based components: a string encoder, an input-output analyzer, a program generator, and a symbol selector. We demonstrate the effectiveness of NPBE by training it end-to-end to solve some common string manipulation problems in spreadsheet systems. The results show that our model can induce string manipulation programs effectively. Our work is one step towards teaching DNN to generate computer programs.
from cs.AI updates on arXiv.org http://ift.tt/2muTl0T
via IFTTT
Fuzzy Rankings: Properties and Applications. (arXiv:1703.05201v1 [cs.AI])
In practice, a ranking of objects with respect to given set of criteria is of considerable importance. However, due to lack of knowledge, information of time pressure, decision makers might not be able to provide a (crisp) ranking of objects from the top to the bottom. Instead, some objects might be ranked equally, or better than other objects only to some degree. In such cases, a generalization of crisp rankings to fuzzy rankings can be more useful. The aim of the article is to introduce the notion of a fuzzy ranking and to discuss its several properties, namely orderings, similarity and indecisiveness. The proposed approach can be used both for group decision making or multiple criteria decision making when uncertainty is involved.
from cs.AI updates on arXiv.org http://ift.tt/2nGthQq
via IFTTT
On Inconsistency Indices and Inconsistency Axioms in Pairwise Comparisons. (arXiv:1703.05204v1 [cs.AI])
Pairwise comparisons are an important tool of modern (multiple criteria) decision making. Since human judgments are often inconsistent, many studies focused on the ways how to express and measure this inconsistency, and several inconsistency indices were proposed as an alternative to Saaty inconsistency index and inconsistency ratio for reciprocal pairwise comparisons matrices. This paper aims to: firstly, introduce a new measure of inconsistency of pairwise comparisons and to prove its basic properties; secondly, to postulate an additional axiom, an upper boundary axiom, to an existing set of axioms; and the last, but not least, the paper provides proofs of satisfaction of this additional axiom by selected inconsistency indices as well as it provides their numerical comparison.
from cs.AI updates on arXiv.org http://ift.tt/2mQqxCw
via IFTTT
InScript: Narrative texts annotated with script information. (arXiv:1703.05260v1 [cs.CL])
This paper presents the InScript corpus (Narrative Texts Instantiating Script structure). InScript is a corpus of 1,000 stories centered around 10 different scenarios. Verbs and noun phrases are annotated with event and participant types, respectively. Additionally, the text is annotated with coreference information. The corpus shows rich lexical variation and will serve as a unique resource for the study of the role of script knowledge in natural language processing.
from cs.AI updates on arXiv.org http://ift.tt/2nGxgwj
via IFTTT
Recurrent Orthogonal Networks and Long-Memory Tasks. (arXiv:1602.06662v2 [cs.NE] UPDATED)
Although RNNs have been shown to be powerful tools for processing sequential data, finding architectures or optimization strategies that allow them to model very long term dependencies is still an active area of research. In this work, we carefully analyze two synthetic datasets originally outlined in (Hochreiter and Schmidhuber, 1997) which are used to evaluate the ability of RNNs to store information over many time steps. We explicitly construct RNN solutions to these problems, and using these constructions, illuminate both the problems themselves and the way in which RNNs store different types of information in their hidden states. These constructions furthermore explain the success of recent methods that specify unitary initializations or constraints on the transition matrices.
from cs.AI updates on arXiv.org http://ift.tt/1QWI68w
via IFTTT
A Logic of Knowing Why. (arXiv:1609.06405v2 [cs.AI] UPDATED)
When we say "I know why he was late", we know not only the fact that he was late, but also an explanation of this fact. We propose a logical framework of "knowing why" inspired by the existing formal studies on why-questions, scientific explanation, and justification logic. We introduce the Ky_i operator into the language of epistemic logic to express "agent i knows why phi" and propose a Kripke-style semantics of such expressions in terms of knowing an explanation of phi. We obtain two sound and complete axiomatizations w.r.t. two different model classes depending on different assumptions about introspection.
from cs.AI updates on arXiv.org http://ift.tt/2cSFI7V
via IFTTT
Find Your Own Way: Weakly-Supervised Segmentation of Path Proposals for Urban Autonomy. (arXiv:1610.01238v2 [cs.RO] UPDATED)
We present a weakly-supervised approach to segmenting proposed drivable paths in images with the goal of autonomous driving in complex urban environments. Using recorded routes from a data collection vehicle, our proposed method generates vast quantities of labelled images containing proposed paths and obstacles without requiring manual annotation, which we then use to train a deep semantic segmentation network. With the trained network we can segment proposed paths and obstacles at run-time using a vehicle equipped with only a monocular camera without relying on explicit modelling of road or lane markings. We evaluate our method on the large-scale KITTI and Oxford RobotCar datasets and demonstrate reliable path proposal and obstacle segmentation in a wide variety of environments under a range of lighting, weather and traffic conditions. We illustrate how the method can generalise to multiple path proposals at intersections and outline plans to incorporate the system into a framework for autonomous urban driving.
from cs.AI updates on arXiv.org http://ift.tt/2dLIhIK
via IFTTT
Learning to Play Guess Who? and Inventing a Grounded Language as a Consequence. (arXiv:1611.03218v4 [cs.AI] UPDATED)
Acquiring your first language is an incredible feat and not easily duplicated. Learning to communicate using nothing but a few pictureless books, a corpus, would likely be impossible even for humans. Nevertheless, this is the dominating approach in most natural language processing today. As an alternative, we propose the use of situated interactions between agents as a driving force for communication, and the framework of Deep Recurrent Q-Networks for evolving a shared language grounded in the provided environment. We task the agents with interactive image search in the form of the game Guess Who?. The images from the game provide a non trivial environment for the agents to discuss and a natural grounding for the concepts they decide to encode in their communication. Our experiments show that the agents learn not only to encode physical concepts in their words, i.e. grounding, but also that the agents learn to hold a multi-step dialogue remembering the state of the dialogue from step to step.
from cs.AI updates on arXiv.org http://ift.tt/2eGC8Nq
via IFTTT
Deep Learning of Robotic Tasks using Strong and Weak Human Supervision. (arXiv:1612.01086v2 [cs.AI] UPDATED)
We propose a scheme for training a computerized agent to perform complex human tasks such as highway steering. The scheme is designed to follow a natural learning process whereby a human instructor teaches a computerized trainee. The learning process consists of five elements: (i) unsupervised feature learning; (ii) supervised imitation learning; (iii) supervised reward induction; (iv) supervised safety module construction; and (v) reinforcement learning. We implemented the last four elements of the scheme using deep convolutional networks and applied it to successfully create a computerized agent capable of autonomous highway steering over the well-known racing game Assetto Corsa. We demonstrate that the use of the last four elements is essential to effectively carry out the steering task using vision alone, without access to a driving simulator internals, and operating in wall-clock time. This is made possible also through the introduction of a safety network, a novel way for preventing the agent from performing catastrophic mistakes during the reinforcement learning stage.
from cs.AI updates on arXiv.org http://ift.tt/2g42U2H
via IFTTT
Highway and Residual Networks learn Unrolled Iterative Estimation. (arXiv:1612.07771v3 [cs.NE] UPDATED)
The past year saw the introduction of new architectures such as Highway networks and Residual networks which, for the first time, enabled the training of feedforward networks with dozens to hundreds of layers using simple gradient descent. While depth of representation has been posited as a primary reason for their success, there are indications that these architectures defy a popular view of deep learning as a hierarchical computation of increasingly abstract features at each layer.
In this report, we argue that this view is incomplete and does not adequately explain several recent findings. We propose an alternative viewpoint based on unrolled iterative estimation -- a group of successive layers iteratively refine their estimates of the same features instead of computing an entirely new representation. We demonstrate that this viewpoint directly leads to the construction of Highway and Residual networks. Finally we provide preliminary experiments to discuss the similarities and differences between the two architectures.
from cs.AI updates on arXiv.org http://ift.tt/2i7d4Sz
via IFTTT
Application of Fuzzy Logic in Design of Smart Washing Machine. (arXiv:1701.01654v2 [cs.SY] UPDATED)
Washing machine is of great domestic necessity as it frees us from the burden of washing our clothes and saves ample of our time. This paper will cover the aspect of designing and developing of Fuzzy Logic based, Smart Washing Machine. The regular washing machine (timer based) makes use of multi-turned timer based start-stop mechanism which is mechanical as is prone to breakage. In addition to its starting and stopping issues, the mechanical timers are not efficient with respect of maintenance and electricity usage. Recent developments have shown that merger of digital electronics in optimal functionality of this machine is possible and nowadays in practice. A number of international renowned companies have developed the machine with the introduction of smart artificial intelligence. Such a machine makes use of sensors and smartly calculates the amount of run-time (washing time) for the main machine motor. Realtime calculations and processes are also catered in optimizing the run-time of the machine. The obvious result is smart time management, better economy of electricity and efficiency of work. This paper deals with the indigenization of FLC (Fuzzy Logic Controller) based Washing Machine, which is capable of automating the inputs and getting the desired output (wash-time).
from cs.AI updates on arXiv.org http://ift.tt/2jsbOx5
via IFTTT
Residual LSTM: Design of a Deep Recurrent Architecture for Distant Speech Recognition. (arXiv:1701.03360v2 [cs.LG] UPDATED)
In this paper, a novel architecture for a deep recurrent neural network, residual LSTM is introduced. A plain LSTM has an internal memory cell that can learn long term dependencies of sequential data. It also provides a temporal shortcut path to avoid vanishing or exploding gradients in the temporal domain. The residual LSTM provides an additional spatial shortcut path from lower layers for efficient training of deep networks with multiple LSTM layers. Compared with the previous work, highway LSTM, residual LSTM separates a spatial shortcut path with temporal one by using output layers, which can help to avoid a conflict between spatial and temporal-domain gradient flows. Furthermore, residual LSTM reuses the output projection matrix and the output gate of LSTM to control the spatial information flow instead of additional gate networks, which effectively reduces more than 10% of network parameters. An experiment for distant speech recognition on the AMI SDM corpus shows that 10-layer plain and highway LSTM networks presented 13.7% and 6.2% increase in WER over 3-layer aselines, respectively. On the contrary, 10-layer residual LSTM networks provided the lowest WER 41.0%, which corresponds to 3.3% and 2.8% WER reduction over plain and highway LSTM networks, respectively.
from cs.AI updates on arXiv.org http://ift.tt/2jBW6vq
via IFTTT
Actor-Critic Reinforcement Learning with Simultaneous Human Control and Feedback. (arXiv:1703.01274v2 [cs.AI] UPDATED)
This paper contributes a first study into how different human users deliver simultaneous control and feedback signals during human-robot interaction. As part of this work, we formalize and present a general interactive learning framework for online cooperation between humans and reinforcement learning agents. In many human-machine interaction settings, there is a growing gap between the degrees-of-freedom of complex semi-autonomous systems and the number of human control channels. Simple human control and feedback mechanisms are required to close this gap and allow for better collaboration between humans and machines on complex tasks. To better inform the design of concurrent control and feedback interfaces, we present experimental results from a human-robot collaborative domain wherein the human must simultaneously deliver both control and feedback signals to interactively train an actor-critic reinforcement learning robot. We compare three experimental conditions: 1) human delivered control signals, 2) reward-shaping feedback signals, and 3) simultaneous control and feedback. Our results suggest that subjects provide less feedback when simultaneously delivering feedback and control signals and that control signal quality is not significantly diminished. Our data suggest that subjects may also modify when and how they provide feedback. Through algorithmic development and tuning informed by this study, we expect semi-autonomous actions of robotic agents can be better shaped by human feedback, allowing for seamless collaboration and improved performance in difficult interactive domains.
from cs.AI updates on arXiv.org http://ift.tt/2mtDSBs
via IFTTT
A Compact DNN: Approaching GoogLeNet-Level Accuracy of Classification and Domain Adaptation. (arXiv:1703.04071v1 [cs.CV] CROSS LISTED)
Recently, DNN model compression based on network architecture design, e.g., SqueezeNet, attracted a lot attention. No accuracy drop on image classification is observed on these extremely compact networks, compared to well-known models. An emerging question, however, is whether these model compression techniques hurt DNN's learning ability other than classifying images on a single dataset. Our preliminary experiment shows that these compression methods could degrade domain adaptation (DA) ability, though the classification performance is preserved. Therefore, we propose a new compact network architecture and unsupervised DA method in this paper. The DNN is built on a new basic module Conv-M which provides more diverse feature extractors without significantly increasing parameters. The unified framework of our DA method will simultaneously learn invariance across domains, reduce divergence of feature representations, and adapt label prediction. Our DNN has 4.1M parameters, which is only 6.7% of AlexNet or 59% of GoogLeNet. Experiments show that our DNN obtains GoogLeNet-level accuracy both on classification and DA, and our DA method slightly outperforms previous competitive ones. Put all together, our DA strategy based on our DNN achieves state-of-the-art on sixteen of total eighteen DA tasks on popular Office-31 and Office-Caltech datasets.
from cs.AI updates on arXiv.org http://ift.tt/2mDO0Fw
via IFTTT
McShay Mock Draft 3.0: Ravens grab Washington WR John Ross at No. 16; \"Wide receiver has become a priority\" - Jamison Hensley (ESPN)
via IFTTT
US Charges Two Russian Spies & Two Hackers For Hacking 500 Million Yahoo Accounts
from The Hacker News http://ift.tt/2nb3VgW
via IFTTT
Ravens will release C Jeremy Zuttah Thursday after not being able to trade him - multiple reports; creates $2.392M in cap space (ESPN)
via IFTTT
I have a new follower on Twitter
Anna Puopolo
Host of the Anna Show - CEO/Founder @Kidviewed Daughter of @jpuopolo and @laurapuopolo. Entrepreneur in training an Princess Leia wannabe!
Cambridge, Ontario
https://t.co/KOnVoDFGG7
Following: 4861 - Followers: 2714
March 15, 2017 at 11:48AM via Twitter http://twitter.com/annapuopolo
How One Photo Could Have Hacked Your WhatsApp and Telegram Accounts
from The Hacker News http://ift.tt/2mZPtrL
via IFTTT
ISS Daily Summary Report – 3/14/2017
from ISS On-Orbit Status Report http://ift.tt/2nnlI56
via IFTTT