Latest YouTube Video
Saturday, December 5, 2015
Two Overeaters Anonymous Meetings Saturdays 9:45am & 11am
from Google Alert - anonymous http://ift.tt/21G5hwl
via IFTTT
Anonymous 4
from Google Alert - anonymous http://ift.tt/1OGIKKR
via IFTTT
Zeroholics Anonymous (ZA) - A Support Group
from Google Alert - anonymous http://ift.tt/1NygitA
via IFTTT
Serious, Yet Patched Flaw Exposes 6.1 Million IoT, Mobile Devices to Remote Code Execution
from The Hacker News http://ift.tt/1R0Hkft
via IFTTT
Meet The Team Behind DARK KNIGHT III: THE MASTER RACE At Midtown Comics Downtown ...
from Google Alert - anonymous http://ift.tt/1YPAlr7
via IFTTT
Silk Road Mentor 'Variety Jones' Arrested in Thailand
from The Hacker News http://ift.tt/1Q6tM20
via IFTTT
Cygnus: Bubble and Crescent
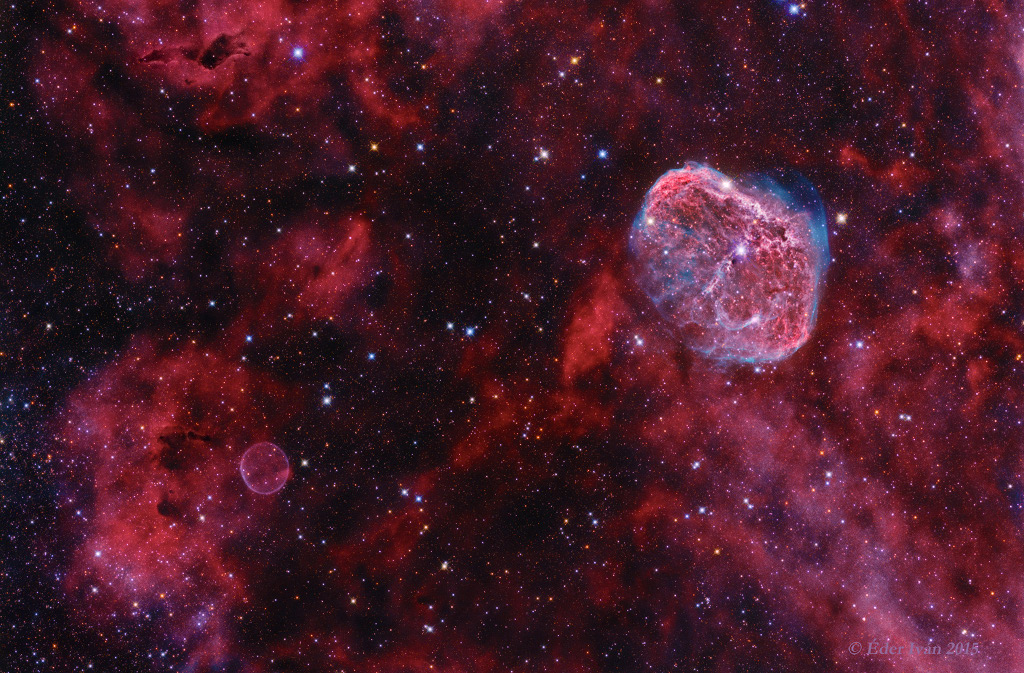
Friday, December 4, 2015
Slideshow not working for anonymous users
from Google Alert - anonymous http://ift.tt/1NxwOpk
via IFTTT
Anonymous Leaks Paris Climate Summit Officials' Private Data
from Google Alert - anonymous http://ift.tt/1PG9Hj8
via IFTTT
[FD] KL-001-2015-006 : Linksys EA6100 Wireless Router Authentication Bypass
Source: Gmail -> IFTTT-> Blogger
And the Title of "Most Vulnerable Programming Language of Year 2015" Goes to...
from The Hacker News http://ift.tt/1leOz6Z
via IFTTT
ISS Daily Summary Report – 12/3/15
from ISS On-Orbit Status Report http://ift.tt/1Itr9Vj
via IFTTT
How to Run Multiple Android apps on Windows and Mac OS X Simultaneously
from The Hacker News http://ift.tt/1IIojXp
via IFTTT
report on the publication of administrative penalties on an anonymous basis
from Google Alert - anonymous http://ift.tt/1lB94Kq
via IFTTT
Kazakhstan makes it Mandatory for its Citizens to Install Internet Backdoor
from The Hacker News http://ift.tt/1OKuu1A
via IFTTT
Separate closures for anonymous functions with distinct capture lists.
from Google Alert - anonymous http://ift.tt/1RrRBAC
via IFTTT
ALERT: This New Ransomware Steals Passwords Before Encrypting Files
from The Hacker News http://ift.tt/1N7dksx
via IFTTT
A Step-by-Step Guide — How to Install Free HTTPS/SSL Certificate on your Website
from The Hacker News http://ift.tt/1NugDt2
via IFTTT
Enceladus: Ringside Water World
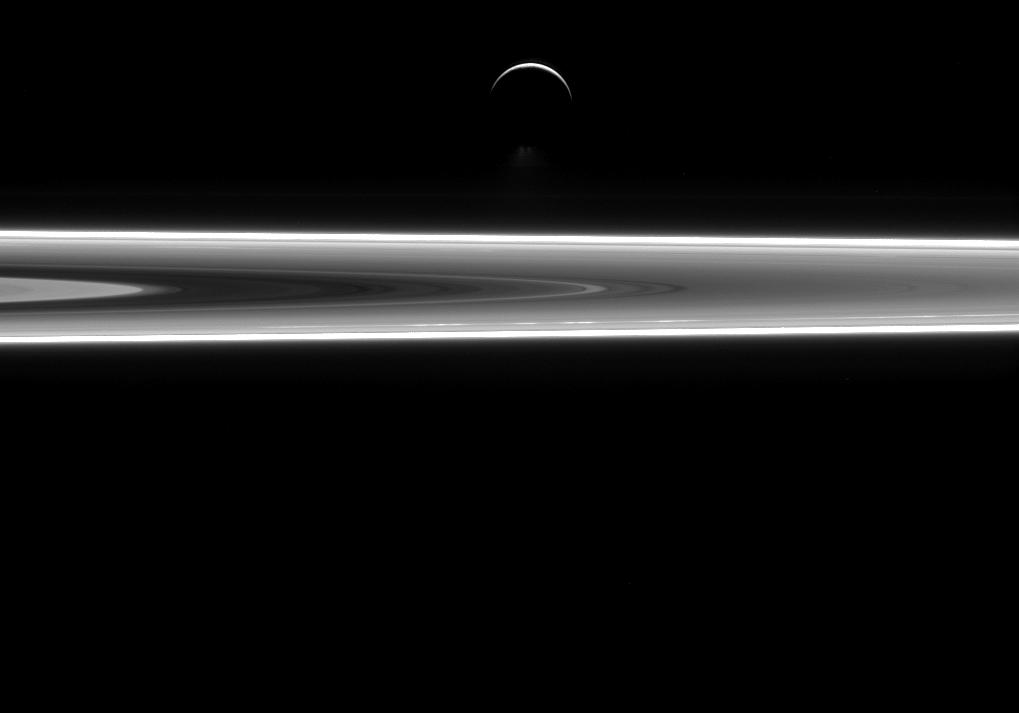
Thursday, December 3, 2015
Paris Summit Hacked By Anonymous
from Google Alert - anonymous http://ift.tt/1m0n1lP
via IFTTT
The GTR-model: a universal framework for quantum-like measurements. (arXiv:1512.00880v1 [quant-ph])
We present a very general geometrico-dynamical description of physical or more abstract entities, called the 'general tension-reduction' (GTR) model, where not only states, but also measurement-interactions can be represented, and the associated outcome probabilities calculated. Underlying the model is the hypothesis that indeterminism manifests as a consequence of unavoidable fluctuations in the experimental context, in accordance with the 'hidden-measurements interpretation' of quantum mechanics. When the structure of the state space is Hilbertian, and measurements are of the 'universal' kind, i.e., are the result of an average over all possible ways of selecting an outcome, the GTR-model provides the same predictions of the Born rule, and therefore provides a natural completed version of quantum mechanics. However, when the structure of the state space is non-Hilbertian and/or not all possible ways of selecting an outcome are available to be actualized, the predictions of the model generally differ from the quantum ones, especially when sequential measurements are considered. Some paradigmatic examples will be discussed, taken from physics and human cognition. Particular attention will be given to some known psychological effects, like question order effects and response replicability, which we show are able to generate non-Hilbertian statistics. We also suggest a realistic interpretation of the GTR-model, when applied to human cognition and decision, which we think could become the generally adopted interpretative framework in quantum cognition research.
from cs.AI updates on arXiv.org http://ift.tt/1XDOVVL
via IFTTT
Modeling Human Understanding of Complex Intentional Action with a Bayesian Nonparametric Subgoal Model. (arXiv:1512.00964v1 [cs.AI])
Most human behaviors consist of multiple parts, steps, or subtasks. These structures guide our action planning and execution, but when we observe others, the latent structure of their actions is typically unobservable, and must be inferred in order to learn new skills by demonstration, or to assist others in completing their tasks. For example, an assistant who has learned the subgoal structure of a colleague's task can more rapidly recognize and support their actions as they unfold. Here we model how humans infer subgoals from observations of complex action sequences using a nonparametric Bayesian model, which assumes that observed actions are generated by approximately rational planning over unknown subgoal sequences. We test this model with a behavioral experiment in which humans observed different series of goal-directed actions, and inferred both the number and composition of the subgoal sequences associated with each goal. The Bayesian model predicts human subgoal inferences with high accuracy, and significantly better than several alternative models and straightforward heuristics. Motivated by this result, we simulate how learning and inference of subgoals can improve performance in an artificial user assistance task. The Bayesian model learns the correct subgoals from fewer observations, and better assists users by more rapidly and accurately inferring the goal of their actions than alternative approaches.
from cs.AI updates on arXiv.org http://ift.tt/1XLANUV
via IFTTT
Neural Enquirer: Learning to Query Tables. (arXiv:1512.00965v1 [cs.AI])
We proposed Neural Enquirer as a neural network architecture to execute a SQL-like query on a knowledge-base (KB) for answers. Basically, Neural Enquirer finds the distributed representation of a query and then executes it on knowledge-base tables to obtain the answer as one of the values in the tables. Unlike similar efforts in end-to-end training of semantic parser, Neural Enquirer is fully neuralized: it not only gives distributional representation of the query and the knowledge-base, but also realizes the execution of compositional queries as a series of differentiable operations, with intermediate results (consisting of annotations of the tables at different levels) saved on multiple layers of memory. Neural Enquirer can be trained with gradient descent, with which not only the parameters of the controlling components and semantic parsing component, but also the embeddings of the tables and query words can be learned from scratch. The training can be done in an end-to-end fashion, but it can take stronger guidance, e.g., the step-by-step supervision for complicated queries, and benefit from it. Neural Enquirer is one step towards building neural network systems which seek to understand language by executing it on real-world. Our experiments show that Neural Enquirer can learn to execute fairly complicated queries on tables with rich structures.
from cs.AI updates on arXiv.org http://ift.tt/1jC8VFq
via IFTTT
A Study on Artificial Intelligence IQ and Standard Intelligent Model. (arXiv:1512.00977v1 [cs.AI])
Currently, potential threats of artificial intelligence (AI) to human have triggered a large controversy in society, behind which, the nature of the issue is whether the artificial intelligence (AI) system can be evaluated quantitatively. This article analyzes and evaluates the challenges that the AI development level is facing, and proposes that the evaluation methods for the human intelligence test and the AI system are not uniform; and the key reason for which is that none of the models can uniformly describe the AI system and the beings like human. Aiming at this problem, a standard intelligent system model is established in this study to describe the AI system and the beings like human uniformly. Based on the model, the article makes an abstract mathematical description, and builds the standard intelligent machine mathematical model; expands the Von Neumann architecture and proposes the Liufeng - Shiyong architecture; gives the definition of the artificial intelligence IQ, and establishes the artificial intelligence scale and the evaluation method; conduct the test on 50 search engines and three human subjects at different ages across the world, and finally obtains the ranking of the absolute IQ and deviation IQ ranking for artificial intelligence IQ 2014.
from cs.AI updates on arXiv.org http://ift.tt/1XDOVVD
via IFTTT
Discrete Equilibrium Sampling with Arbitrary Nonequilibrium Processes. (arXiv:1512.01027v1 [stat.CO])
We present a novel framework for performing statistical sampling, expectation estimation, and partition function approximation using \emph{arbitrary} heuristic stochastic processes defined over discrete state spaces. Using a highly parallel construction we call the \emph{sequential constraining process}, we are able to simultaneously generate states with the heuristic process and accurately estimate their probabilities, even when they are far too small to be realistically inferred by direct counting. After showing that both theoretically correct importance sampling and Markov chain Monte Carlo are possible using the sequential constraining process, we integrate it into a methodology called \emph{state space sampling}, extending the ideas of state space search from computer science to the sampling context. The methodology comprises a dynamic data structure that constructs a robust Bayesian model of the statistics generated by the heuristic process subject to an accuracy constraint, the posterior Kullback-Leibler divergence. Sampling from the dynamic structure will generally yield partial states, which are completed by recursively calling the heuristic to refine the structure and resuming the sampling. Our experiments on various Ising models suggest that state space sampling enables heuristic state generation with accurate probability estimates, demonstrated by illustrating the convergence of a simulated annealing process to the Boltzmann distribution with increasing run length. Consequently, heretofore unprecedented direct importance sampling using the \emph{final} (marginal) distribution of a generic stochastic process is allowed, potentially augmenting the range of algorithms at the Monte Carlo practitioner's disposal.
from cs.AI updates on arXiv.org http://ift.tt/1PC7dSV
via IFTTT
Querying with {\L}ukasiewicz logic. (arXiv:1512.01041v1 [cs.LO])
In this paper we present, by way of case studies, a proof of concept, based on a prototype working on a automotive data set, aimed at showing the potential usefulness of using formulas of {\L}ukasiewicz propositional logic to query databases in a fuzzy way. Our approach distinguishes itself for its stress on the purely linguistic, contraposed with numeric, formulations of queries. Our queries are expressed in the pure language of logic, and when we use (integer) numbers, these stand for shortenings of formulas on the syntactic level, and serve as linguistic hedges on the semantic one. Our case-study queries aim first at showing that each numeric-threshold fuzzy query is simulated by a {\L}ukasiewicz formula. Then they focus on the expressing power of {\L}ukasiewicz logic which easily allows for updating queries by clauses and for modifying them through a potentially infinite variety of linguistic hedges implemented with a uniform syntactic mechanism. Finally we shall hint how, already at propositional level, {\L}ukasiewicz natural semantics enjoys a degree of reflection, allowing to write syntactically simple queries that semantically work as meta-queries weighing the contribution of simpler ones.
from cs.AI updates on arXiv.org http://ift.tt/1XDOYAG
via IFTTT
Bayesian Matrix Completion via Adaptive Relaxed Spectral Regularization. (arXiv:1512.01110v1 [cs.NA])
Bayesian matrix completion has been studied based on a low-rank matrix factorization formulation with promising results. However, little work has been done on Bayesian matrix completion based on the more direct spectral regularization formulation. We fill this gap by presenting a novel Bayesian matrix completion method based on spectral regularization. In order to circumvent the difficulties of dealing with the orthonormality constraints of singular vectors, we derive a new equivalent form with relaxed constraints, which then leads us to design an adaptive version of spectral regularization feasible for Bayesian inference. Our Bayesian method requires no parameter tuning and can infer the number of latent factors automatically. Experiments on synthetic and real datasets demonstrate encouraging results on rank recovery and collaborative filtering, with notably good results for very sparse matrices.
from cs.AI updates on arXiv.org http://ift.tt/1jC8VFg
via IFTTT
Deep Reinforcement Learning with Attention for Slate Markov Decision Processes with High-Dimensional States and Actions. (arXiv:1512.01124v1 [cs.AI])
Many real-world problems come with action spaces represented as feature vectors. Although high-dimensional control is a largely unsolved problem, there has recently been progress for modest dimensionalities. Here we report on a successful attempt at addressing problems of dimensionality as high as $2000$, of a particular form. Motivated by important applications such as recommendation systems that do not fit the standard reinforcement learning frameworks, we introduce Slate Markov Decision Processes (slate-MDPs). A Slate-MDP is an MDP with a combinatorial action space consisting of slates (tuples) of primitive actions of which one is executed in an underlying MDP. The agent does not control the choice of this executed action and the action might not even be from the slate, e.g., for recommendation systems for which all recommendations can be ignored. We use deep Q-learning based on feature representations of both the state and action to learn the value of whole slates. Unlike existing methods, we optimize for both the combinatorial and sequential aspects of our tasks. The new agent's superiority over agents that either ignore the combinatorial or sequential long-term value aspect is demonstrated on a range of environments with dynamics from a real-world recommendation system. Further, we use deep deterministic policy gradients to learn a policy that for each position of the slate, guides attention towards the part of the action space in which the value is the highest and we only evaluate actions in this area. The attention is used within a sequentially greedy procedure leveraging submodularity. Finally, we show how introducing risk-seeking can dramatically imporve the agents performance and ability to discover more far reaching strategies.
from cs.AI updates on arXiv.org http://ift.tt/1Ntv8lc
via IFTTT
Building Memory with Concept Learning Capabilities from Large-scale Knowledge Base. (arXiv:1512.01173v1 [cs.CL])
We present a new perspective on neural knowledge base (KB) embeddings, from which we build a framework that can model symbolic knowledge in the KB together with its learning process. We show that this framework well regularizes previous neural KB embedding model for superior performance in reasoning tasks, while having the capabilities of dealing with unseen entities, that is, to learn their embeddings from natural language descriptions, which is very like human's behavior of learning semantic concepts.
from cs.AI updates on arXiv.org http://ift.tt/1NtvaJN
via IFTTT
The Human Kernel. (arXiv:1510.07389v3 [cs.LG] UPDATED)
Bayesian nonparametric models, such as Gaussian processes, provide a compelling framework for automatic statistical modelling: these models have a high degree of flexibility, and automatically calibrated complexity. However, automating human expertise remains elusive; for example, Gaussian processes with standard kernels struggle on function extrapolation problems that are trivial for human learners. In this paper, we create function extrapolation problems and acquire human responses, and then design a kernel learning framework to reverse engineer the inductive biases of human learners across a set of behavioral experiments. We use the learned kernels to gain psychological insights and to extrapolate in human-like ways that go beyond traditional stationary and polynomial kernels. Finally, we investigate Occam's razor in human and Gaussian process based function learning.
from cs.AI updates on arXiv.org http://ift.tt/1LSTznQ
via IFTTT
Alcoholics Anonymous Humboldt Meetings
from Google Alert - anonymous http://ift.tt/1PBOHtZ
via IFTTT
Accidentally anonymous
from Google Alert - anonymous http://ift.tt/1O6iN2v
via IFTTT
I have a new follower on Twitter
Mike Kopack
#UX #Designer @Availity in Indianapolis. Motorhead, Writer, & Recovering Lego Maniac
Indianapolis, IN
http://t.co/clP8Oj1Dpd
Following: 2167 - Followers: 2226
December 03, 2015 at 04:40PM via Twitter http://twitter.com/MikeKopack
China — OPM Hack was not State-Sponsored; Blames Chinese Criminal Gangs
from The Hacker News http://ift.tt/1YLf6a8
via IFTTT
Orioles Video: Signs pointing to Chris Davis leaving, Eddie Matz explains; Baltimore \"more focused on outfielders\" (ESPN)
via IFTTT
ISS Daily Summary Report – 12/2/15
from ISS On-Orbit Status Report http://ift.tt/1Q2vjGb
via IFTTT
Security to be increased after anonymous online threat about TCC in Portsmouth
from Google Alert - anonymous http://ift.tt/1jAqq9a
via IFTTT
[FD] Multiple vulnerabilities in Huutopörssi's website (huutoporssi.fi)
Source: Gmail -> IFTTT-> Blogger
[FD] Huawei Wimax routers vulnerable to multiple threats
Source: Gmail -> IFTTT-> Blogger
Ocean City, MD's surf is at least 6.32ft high
Ocean City, MD Summary
At 2:00 AM, surf min of 6.32ft. At 8:00 AM, surf min of 6.12ft. At 2:00 PM, surf min of 5.29ft. At 8:00 PM, surf min of 4.32ft.
Surf maximum: 6.62ft (2.02m)
Surf minimum: 6.32ft (1.93m)
Tide height: 1.43ft (0.43m)
Wind direction: NW
Wind speed: 17.07 KTS
from Surfline http://ift.tt/1kVmigH
via IFTTT
[FD] BF and CE vulnerabilities in ASUS RT-G32
Source: Gmail -> IFTTT-> Blogger
[FD] [CFP] BSides San Francisco - February 2016
Source: Gmail -> IFTTT-> Blogger
Wednesday, December 2, 2015
Attribute2Image: Conditional Image Generation from Visual Attributes. (arXiv:1512.00570v1 [cs.LG])
This paper investigates a problem of generating images from visual attributes. Given the prevalent research for image recognition, the conditional image generation problem is relatively under-explored due to the challenges of learning a good generative model and handling rendering uncertainties in images. To address this, we propose a variety of attribute-conditioned deep variational auto-encoders that enjoy both effective representation learning and Bayesian modeling, from which images can be generated from specified attributes and sampled latent factors. We experiment with natural face images and demonstrate that the proposed models are capable of generating realistic faces with diverse appearance. We further evaluate the proposed models by performing attribute-conditioned image progression, transfer and retrieval. In particular, our generation method achieves superior performance in the retrieval experiment against traditional nearest-neighbor-based methods both qualitatively and quantitatively.
from cs.AI updates on arXiv.org http://ift.tt/1MXiGII
via IFTTT
Object-based World Modeling in Semi-Static Environments with Dependent Dirichlet-Process Mixtures. (arXiv:1512.00573v1 [cs.AI])
To accomplish tasks in human-centric indoor environments, robots need to represent and understand the world in terms of objects and their attributes. We refer to this attribute-based representation as a world model, and consider how to acquire it via noisy perception and maintain it over time, as objects are added, changed, and removed in the world. Previous work has framed this as multiple-target tracking problem, where objects are potentially in motion at all times. Although this approach is general, it is computationally expensive. We argue that such generality is not needed in typical world modeling tasks, where objects only change state occasionally. More efficient approaches are enabled by restricting ourselves to such semi-static environments.
We consider a previously-proposed clustering-based world modeling approach that assumed static environments, and extend it to semi-static domains by applying a dependent Dirichlet-process (DDP) mixture model. We derive a novel MAP inference algorithm under this model, subject to data association constraints. We demonstrate our approach improves computational performance in semi-static environments.
from cs.AI updates on arXiv.org http://ift.tt/1Ro8jkt
via IFTTT
Information entropy as an anthropomorphic concept. (arXiv:1503.01967v2 [cs.IT] UPDATED)
According to E.T. Jaynes and E.P. Wigner, entropy is an anthropomorphic concept in the sense that in a physical system correspond many thermodynamic systems. The physical system can be examined from many points of view each time examining different variables and calculating entropy differently. In this paper we discuss how this concept may be applied in information entropy; how Shannon's definition of entropy can fit in Jayne's and Wigner's statement. This is achieved by generalizing Shannon's notion of information entropy and this is the main contribution of the paper. Then we discuss how entropy under these considerations may be used for the comparison of password complexity and as a measure of diversity useful in the analysis of the behavior of genetic algorithms.
from cs.AI updates on arXiv.org http://ift.tt/1aW0rFl
via IFTTT
Listen, Attend, and Walk: Neural Mapping of Navigational Instructions to Action Sequences. (arXiv:1506.04089v3 [cs.CL] UPDATED)
We propose a neural sequence-to-sequence model for direction following, a task that is essential to realizing effective autonomous agents. Our alignment-based encoder-decoder model with long short-term memory recurrent neural networks (LSTM-RNN) translates natural language instructions to action sequences based upon a representation of the observable world state. We introduce a multi-level aligner that empowers our model to focus on sentence "regions" salient to the current world state by using multiple abstractions of the input sentence. In contrast to existing methods, our model uses no specialized linguistic resources (e.g., parsers) or task-specific annotations (e.g., seed lexicons). It is therefore generalizable, yet still achieves the best results reported to-date on a benchmark single-sentence dataset and competitive results for the limited-training multi-sentence setting. We analyze our model through a series of ablations that elucidate the contributions of the primary components of our model.
from cs.AI updates on arXiv.org http://ift.tt/1JSbxbL
via IFTTT
An Efficient Implementation for WalkSAT. (arXiv:1510.07217v2 [cs.AI] UPDATED)
Stochastic local search (SLS) algorithms have exhibited great effectiveness in finding models of random instances of the Boolean satisfiability problem (SAT). As one of the most widely known and used SLS algorithm, WalkSAT plays a key role in the evolutions of SLS for SAT, and also hold state-of-the-art performance on random instances. This work proposes a novel implementation for WalkSAT which decreases the redundant calculations leading to a dramatically speeding up, thus dominates the latest version of WalkSAT including its advanced variants.
from cs.AI updates on arXiv.org http://ift.tt/1S7eVCj
via IFTTT
Why Neurons Have Thousands of Synapses, A Theory of Sequence Memory in Neocortex. (arXiv:1511.00083v2 [q-bio.NC] UPDATED)
Neocortical neurons have thousands of excitatory synapses. It is a mystery how neurons integrate the input from so many synapses and what kind of large-scale network behavior this enables. It has been previously proposed that non-linear properties of dendrites enable neurons to recognize multiple patterns. In this paper we extend this idea by showing that a neuron with several thousand synapses arranged along active dendrites can learn to accurately and robustly recognize hundreds of unique patterns of cellular activity, even in the presence of large amounts of noise and pattern variation. We then propose a neuron model where some of the patterns recognized by a neuron lead to action potentials and define the classic receptive field of the neuron, whereas the majority of the patterns recognized by a neuron act as predictions by slightly depolarizing the neuron without immediately generating an action potential. We then present a network model based on neurons with these properties and show that the network learns a robust model of time-based sequences. Given the similarity of excitatory neurons throughout the neocortex and the importance of sequence memory in inference and behavior, we propose that this form of sequence memory is a universal property of neocortical tissue. We further propose that cellular layers in the neocortex implement variations of the same sequence memory algorithm to achieve different aspects of inference and behavior. The neuron and network models we introduce are robust over a wide range of parameters as long as the network uses a sparse distributed code of cellular activations. The sequence capacity of the network scales linearly with the number of synapses on each neuron. Thus neurons need thousands of synapses to learn the many temporal patterns in sensory stimuli and motor sequences.
from cs.AI updates on arXiv.org http://ift.tt/1H0Nvgd
via IFTTT
Seeing the Unseen Network: Inferring Hidden Social Ties from Respondent-Driven Sampling. (arXiv:1511.04137v2 [cs.SI] UPDATED)
Learning about the social structure of hidden and hard-to-reach populations --- such as drug users and sex workers --- is a major goal of epidemiological and public health research on risk behaviors and disease prevention. Respondent-driven sampling (RDS) is a peer-referral process widely used by many health organizations, where research subjects recruit other subjects from their social network. In such surveys, researchers observe who recruited whom, along with the time of recruitment and the total number of acquaintances (network degree) of respondents. However, due to privacy concerns, the identities of acquaintances are not disclosed. In this work, we show how to reconstruct the underlying network structure through which the subjects are recruited. We formulate the dynamics of RDS as a continuous-time diffusion process over the underlying graph and derive the likelihood for the recruitment time series under an arbitrary recruitment time distribution. We develop an efficient stochastic optimization algorithm called RENDER (REspoNdent-Driven nEtwork Reconstruction) that finds the network that best explains the collected data. We support our analytical results through an exhaustive set of experiments on both synthetic and real data.
from cs.AI updates on arXiv.org http://ift.tt/1NyCJei
via IFTTT
9 Things About Anonymous As They Wage War On Isis
from Google Alert - anonymous http://ift.tt/1NInVj6
via IFTTT
Anonymous Cyber Attacks Iceland's Government to Save the Whales
from Google Alert - anonymous http://ift.tt/21wn21a
via IFTTT
EBA reports on the publication of administrative penalties on an anonymous basis
from Google Alert - anonymous http://ift.tt/1NId1Kg
via IFTTT
Ravens: Baltimore works out QB Ryan Mallett Wednesday; Joe Flacco out for season, Matt Schaub named starter (ESPN)
via IFTTT
Orioles: Trade for Mark Trumbo brings flexibility (plays 1B, LF, RF), power (131 HRs last 5 seasons), writes Eddie Matz (ESPN)
via IFTTT
I have a new follower on Twitter
Youth Sports Aid
Tips and insights on keeping our youth safe with the athletics they are involved in.
Tulsa, OK.
http://t.co/jhmdUZjvDu
Following: 3231 - Followers: 2101
December 02, 2015 at 11:55AM via Twitter http://twitter.com/youthsports_aid
Our anonymous egg donors get $7000 + MORE! Apply to the BEST program!
from Google Alert - anonymous http://ift.tt/1PvTWLw
via IFTTT
Boston Briefing: Red Sox pay the price for an ace; Gronk thinks refs are targeting him; Bruins in action Wednesday night (ESPN)
via IFTTT
anonymous,uncategorized,misc,general,other
from Google Alert - anonymous http://ift.tt/1lXbBix
via IFTTT
Sponsors and donors
from Google Alert - anonymous http://ift.tt/1lXbDan
via IFTTT
Ocean City, MD's surf is at least 6.32ft high
Ocean City, MD Summary
At 2:00 AM, surf min of 3.21ft. At 8:00 AM, surf min of 6.32ft. At 2:00 PM, surf min of 2.13ft. At 8:00 PM, surf min of 2.92ft.
Surf maximum: 7.32ft (2.23m)
Surf minimum: 6.32ft (1.93m)
Tide height: 2.35ft (0.72m)
Wind direction: SE
Wind speed: 19.24 KTS
from Surfline http://ift.tt/1kVmigH
via IFTTT
ISS Daily Summary Report – 12/1/15
from ISS On-Orbit Status Report http://ift.tt/1MW2l7o
via IFTTT
Patent Troll — 66 Big Companies Sued For Using HTTPS Encryption
from The Hacker News http://ift.tt/1YHdTAp
via IFTTT
Adobe to Kill 'FLASH', but by Just Renaming it as 'Adobe Animate CC'
from The Hacker News http://ift.tt/1RlDE7s
via IFTTT
Nebulae in Aurigae
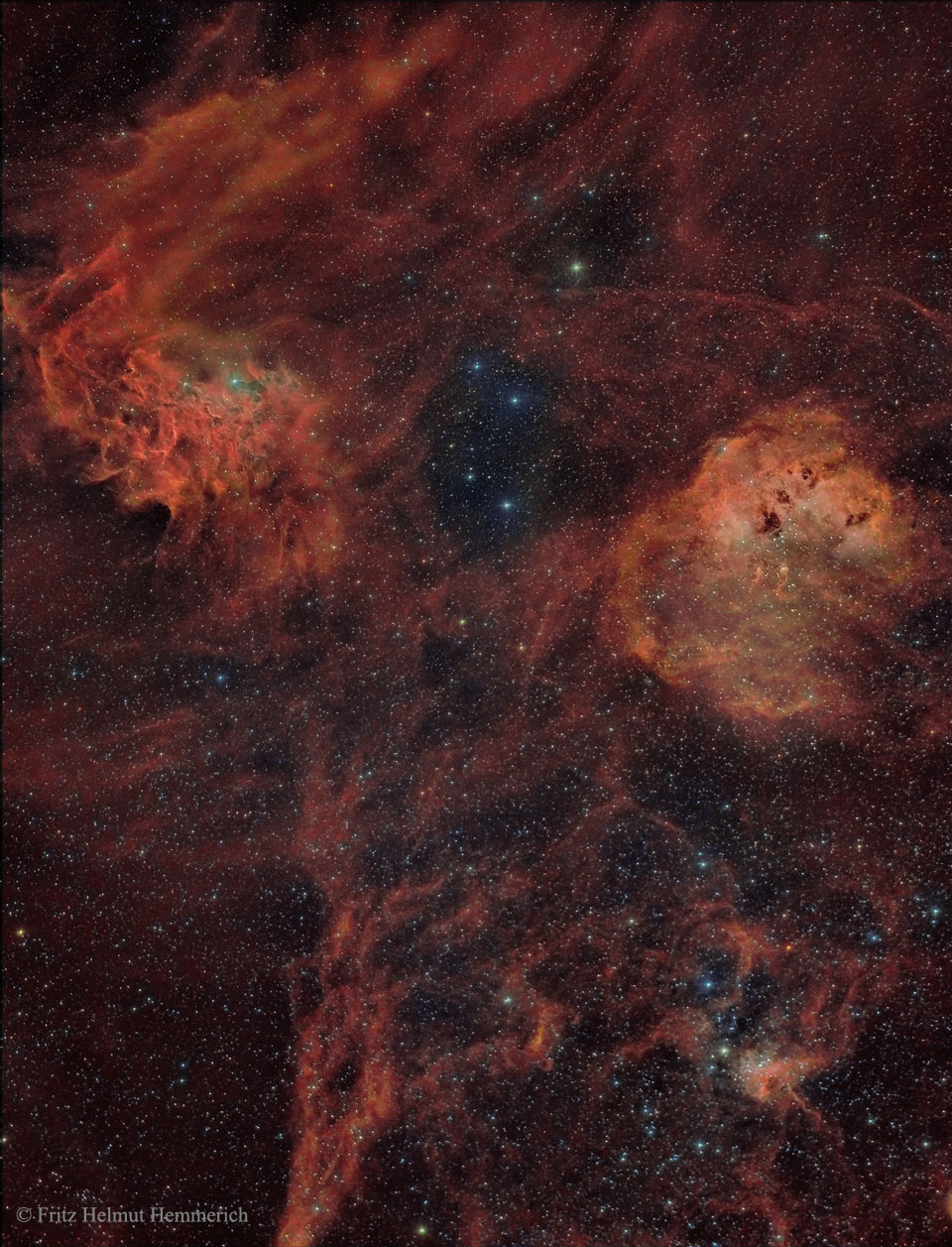
A Quarter Century US Forest Disturbance History from Landsat - the NAFD-NEX Products
from NASA's Scientific Visualization Studio: Most Recent Items http://ift.tt/1LN06PI
via IFTTT
Tuesday, December 1, 2015
Free Anonymous HIV Testing
from Google Alert - anonymous http://ift.tt/1InObwO
via IFTTT
Orioles: Mark Trumbo has been acquired from Mariners for C Steve Clevenger, according to Jim Bowden, multiple reports (ESPN)
via IFTTT
Symbolic Neutrosophic Theory. (arXiv:1512.00047v1 [cs.AI])
Symbolic (or Literal) Neutrosophic Theory is referring to the use of abstract symbols (i.e. the letters T, I, F, or their refined indexed letters Tj, Ik, Fl) in neutrosophics. We extend the dialectical triad thesis-antithesis-synthesis to the neutrosophic tetrad thesis-antithesis-neutrothesis-neutrosynthesis. The we introduce the neutrosophic system that is a quasi or (t,i,f) classical system, in the sense that the neutrosophic system deals with quasi-terms (concepts, attributes, etc.). Then the notions of Neutrosophic Axiom, Neutrosophic Deducibility, Degree of Contradiction (Dissimilarity) of Two Neutrosophic Axioms, etc. Afterwards a new type of structures, called (t, i, f) Neutrosophic Structures, and we show particular cases of such structures in geometry and in algebra. Also, a short history of the neutrosophic set, neutrosophic numerical components and neutrosophic literal components, neutrosophic numbers, etc. We construct examples of splitting the literal indeterminacy (I) into literal subindeterminacies (I1, I2, and so on, Ir), and to define a multiplication law of these literal subindeterminacies in order to be able to build refined I neutrosophic algebraic structures. We define three neutrosophic actions and their properties. We then introduce the prevalence order on T,I,F with respect to a given neutrosophic operator. And the refinement of neutrosophic entities A, neutA, and antiA. Then we extend the classical logical operators to neutrosophic literal (symbolic) logical operators and to refined literal (symbolic) logical operators, and we define the refinement neutrosophic literal (symbolic) space. We introduce the neutrosophic quadruple numbers (a+bT+cI+dF) and the refined neutrosophic quadruple numbers. Then we define an absorbance law, based on a prevalence order, in order to multiply the neutrosophic quadruple numbers.
from cs.AI updates on arXiv.org http://ift.tt/1Tt5w8H
via IFTTT
Inferring Interpersonal Relations in Narrative Summaries. (arXiv:1512.00112v1 [cs.CL])
Characterizing relationships between people is fundamental for the understanding of narratives. In this work, we address the problem of inferring the polarity of relationships between people in narrative summaries. We formulate the problem as a joint structured prediction for each narrative, and present a model that combines evidence from linguistic and semantic features, as well as features based on the structure of the social community in the text. We also provide a clustering-based approach that can exploit regularities in narrative types. e.g., learn an affinity for love-triangles in romantic stories. On a dataset of movie summaries from Wikipedia, our structured models provide more than a 30% error-reduction over a competitive baseline that considers pairs of characters in isolation.
from cs.AI updates on arXiv.org http://ift.tt/1lWmzFe
via IFTTT
Learning Using 1-Local Membership Queries. (arXiv:1512.00165v1 [cs.LG])
Classic machine learning algorithms learn from labelled examples. For example, to design a machine translation system, a typical training set will consist of English sentences and their translation. There is a stronger model, in which the algorithm can also query for labels of new examples it creates. E.g, in the translation task, the algorithm can create a new English sentence, and request its translation from the user during training. This combination of examples and queries has been widely studied. Yet, despite many theoretical results, query algorithms are almost never used. One of the main causes for this is a report (Baum and Lang, 1992) on very disappointing empirical performance of a query algorithm. These poor results were mainly attributed to the fact that the algorithm queried for labels of examples that are artificial, and impossible to interpret by humans.
In this work we study a new model of local membership queries (Awasthi et al., 2012), which tries to resolve the problem of artificial queries. In this model, the algorithm is only allowed to query the labels of examples which are close to examples from the training set. E.g., in translation, the algorithm can change individual words in a sentence it has already seen, and then ask for the translation. In this model, the examples queried by the algorithm will be close to natural examples and hence, hopefully, will not appear as artificial or random. We focus on 1-local queries (i.e., queries of distance 1 from an example in the training sample). We show that 1-local membership queries are already stronger than the standard learning model. We also present an experiment on a well known NLP task of sentiment analysis. In this experiment, the users were asked to provide more information than merely indicating the label. We present results that illustrate that this extra information is beneficial in practice.
from cs.AI updates on arXiv.org http://ift.tt/21tDE9V
via IFTTT
LSTM Neural Reordering Feature for Statistical Machine Translation. (arXiv:1512.00177v1 [cs.CL])
Artificial neural networks are powerful models, which have been widely applied into many aspects of machine translation, such as language modeling and translation modeling. Though notable improvements have been made in these areas, the reordering problem still remains a challenge in statistical machine translations. In this paper, we present a novel neural reordering model that directly models word pairs and alignment. By utilizing LSTM recurrent neural networks, much longer context could be learned for reordering prediction. Experimental results on NIST OpenMT12 Arabic-English and Chinese-English 1000-best rescoring task show that our LSTM neural reordering feature is robust and achieves significant improvements over various baseline systems.
from cs.AI updates on arXiv.org http://ift.tt/1Tt5y0j
via IFTTT
Evaluating Morphological Computation in Muscle and DC-motor Driven Models of Human Hopping. (arXiv:1512.00250v1 [cs.AI])
In the context of embodied artificial intelligence, morphological computation refers to processes which are conducted by the body (and environment) that otherwise would have to be performed by the brain. Exploiting environmental and morphological properties is an important feature of embodied systems. The main reason is that it allows to significantly reduce the controller complexity. An important aspect of morphological computation is that it cannot be assigned to an embodied system per se, but that it is, as we show, behavior- and state-dependent. In this work, we evaluate two different measures of morphological computation that can be applied in robotic systems and in computer simulations of biological movement. As an example, these measures were evaluated on muscle and DC-motor driven hopping models. We show that a state-dependent analysis of the hopping behaviors provides additional insights that cannot be gained from the averaged measures alone. This work includes algorithms and computer code for the measures.
from cs.AI updates on arXiv.org http://ift.tt/21tDE9J
via IFTTT
A Hybrid Intelligent Model for Software Cost Estimation. (arXiv:1512.00306v1 [cs.SE])
Accurate software development effort estimation is critical to the success of software projects. Although many techniques and algorithmic models have been developed and implemented by practitioners, accurate software development effort prediction is still a challenging endeavor in the field of software engineering, especially in handling uncertain and imprecise inputs and collinear characteristics. In this paper, a hybrid in-telligent model combining a neural network model integrated with fuzzy model (neuro-fuzzy model) has been used to improve the accuracy of estimating software cost. The performance of the proposed model is assessed by designing and conducting evaluation with published project and industrial data. Results have shown that the proposed model demonstrates the ability of improving the estimation accuracy by 18% based on the Mean Magnitude of Relative Error (MMRE) criterion.
from cs.AI updates on arXiv.org http://ift.tt/1Tt5vSk
via IFTTT
Taxonomy grounded aggregation of classifiers with different label sets. (arXiv:1512.00355v1 [cs.AI])
We describe the problem of aggregating the label predictions of diverse classifiers using a class taxonomy. Such a taxonomy may not have been available or referenced when the individual classifiers were designed and trained, yet mapping the output labels into the taxonomy is desirable to integrate the effort spent in training the constituent classifiers. A hierarchical taxonomy representing some domain knowledge may be different from, but partially mappable to, the label sets of the individual classifiers. We present a heuristic approach and a principled graphical model to aggregate the label predictions by grounding them into the available taxonomy. Our model aggregates the labels using the taxonomy structure as constraints to find the most likely hierarchically consistent class. We experimentally validate our proposed method on image and text classification tasks.
from cs.AI updates on arXiv.org http://ift.tt/21tDDTt
via IFTTT
A New Approach for Scalable Analysis of Microbial Communities. (arXiv:1512.00397v1 [q-bio.GN])
Microbial communities play important roles in the function and maintenance of various biosystems, ranging from human body to the environment. Current methods for analysis of microbial communities are typically based on taxonomic phylogenetic alignment using 16S rRNA metagenomic or Whole Genome Sequencing data. In typical characterizations of microbial communities, studies deal with billions of micobial sequences, aligning them to a phylogenetic tree. We introduce a new approach for the efficient analysis of microbial communities. Our new reference-free analysis tech- nique is based on n-gram sequence analysis of 16S rRNA data and reduces the processing data size dramatically (by 105 fold), without requiring taxonomic alignment. The proposed approach is applied to characterize phenotypic microbial community differ- ences in different settings. Specifically, we applied this approach in classification of microbial com- munities across different body sites, characterization of oral microbiomes associated with healthy and diseased individuals, and classification of microbial communities longitudinally during the develop- ment of infants. Different dimensionality reduction methods are introduced that offer a more scalable analysis framework, while minimizing the loss in classification accuracies. Among dimensionality re- duction techniques, we propose a continuous vector representation for microbial communities, which can widely be used for deep learning applications in microbial informatics.
from cs.AI updates on arXiv.org http://ift.tt/1Tt5xK3
via IFTTT
Fast k-Nearest Neighbour Search via Dynamic Continuous Indexing. (arXiv:1512.00442v1 [cs.DS])
Existing methods for retrieving k-nearest neighbours suffer from the curse of dimensionality. We argue this is caused in part by inherent deficiencies of space partitioning, which is the underlying strategy used by almost all existing methods. We devise a new strategy that avoids partitioning the vector space and present a novel randomized algorithm that runs in time linear in dimensionality and sub-linear in the size of the dataset and takes space constant in dimensionality and linear in the size of the dataset. The proposed algorithm allows fine-grained control over accuracy and speed on a per-query basis, automatically adapts to variations in dataset density, supports dynamic updates to the dataset and is easy-to-implement. We show appealing theoretical properties and demonstrate empirically that the proposed algorithm outperforms locality-sensitivity hashing (LSH) in terms of approximation quality and speed.
from cs.AI updates on arXiv.org http://ift.tt/1OEnOCm
via IFTTT
Efficient local search limitation strategy for single machine total weighted tardiness scheduling with sequence-dependent setup times. (arXiv:1501.05882v3 [cs.AI] UPDATED)
This paper concerns the single machine total weighted tardiness scheduling with sequence-dependent setup times, usually referred as $1|s_{ij}|\sum w_jT_j$. In this $\mathcal{NP}$-hard problem, each job has an associated processing time, due date and a weight. For each pair of jobs $i$ and $j$, there may be a setup time before starting to process $j$ in case this job is scheduled immediately after $i$. The objective is to determine a schedule that minimizes the total weighted tardiness, where the tardiness of a job is equal to its completion time minus its due date, in case the job is completely processed only after its due date, and is equal to zero otherwise. Due to its complexity, this problem is most commonly solved by heuristics. The aim of this work is to develop a simple yet effective limitation strategy that speeds up the local search procedure without a significant loss in the solution quality. Such strategy consists of a filtering mechanism that prevents unpromising moves to be evaluated. The proposed strategy has been embedded in a local search based metaheuristic from the literature and tested in classical benchmark instances. Computational experiments revealed that the limitation strategy enabled the metaheuristic to be extremely competitive when compared to other algorithms from the literature, since it allowed the use of a large number of neighborhood structures without a significant increase in the CPU time and, consequently, high quality solutions could be achieved in a matter of seconds. In addition, we analyzed the effectiveness of the proposed strategy in two other well-known metaheuristics. Further experiments were also carried out on benchmark instances of problem $1|s_{ij}|\sum T_j$.
from cs.AI updates on arXiv.org http://ift.tt/1zLuHwX
via IFTTT
Amazon Anonymous in Christmas campaign appeal
from Google Alert - anonymous http://ift.tt/1TsXLzI
via IFTTT
anonymous,uncategorized,misc,general,other
from Google Alert - anonymous http://ift.tt/1OvfsyB
via IFTTT
Donate today and your gift will be TRIPLED!
Source: Gmail -> IFTTT-> Blogger
Ravens: Baltimore (4-7) up five spots to No. 25 in Week 13 NFL power rankings; open here for full rankings (ESPN)
via IFTTT
I have a new follower on Twitter
Bradley Milner
Following: 1952 - Followers: 244
December 01, 2015 at 10:17AM via Twitter http://twitter.com/milner_bradley
ISS Daily Summary Report – 11/30/15
from ISS On-Orbit Status Report http://ift.tt/1l4XebR
via IFTTT
I have a new follower on Twitter
Santa Claus
Having a well earned break after 2014’s effort, will be back sometime in September for #Christmas #2015 :) #xmas
Following: 12273 - Followers: 23222
December 01, 2015 at 08:02AM via Twitter http://twitter.com/AmazingSanta
Pro PoS — This Stealthy Point-of-Sale Malware Could Steal Your Christmas
from The Hacker News http://ift.tt/1XDSDct
via IFTTT
I have a new follower on Twitter
Surfholidays.com
Accommodation & Surf Guiding/Lessons in the World's best surf locations.
Global
http://t.co/w84uWpmQ0T
Following: 5124 - Followers: 6634
December 01, 2015 at 06:29AM via Twitter http://twitter.com/surfholidays
WhatsApp Blocks Links to Telegram Messenger (Its biggest Competitor)
from The Hacker News http://ift.tt/1jwGBnG
via IFTTT
Ocean City, MD's surf is at least 5.15ft high
Ocean City, MD Summary
At 2:00 AM, surf min of 3.91ft. At 8:00 AM, surf min of 5.15ft. At 2:00 PM, surf min of 5.06ft. At 8:00 PM, surf min of 4.44ft.
Surf maximum: 6.15ft (1.88m)
Surf minimum: 5.15ft (1.57m)
Tide height: 1.2ft (0.37m)
Wind direction: NW
Wind speed: 6.55 KTS
from Surfline http://ift.tt/1kVmigH
via IFTTT
Toymaker VTech Hack Exposes 4.8 Million Customers, including Photos of Children
from The Hacker News http://ift.tt/1l4shVf
via IFTTT
In the Center of Spiral Galaxy NGC 3521
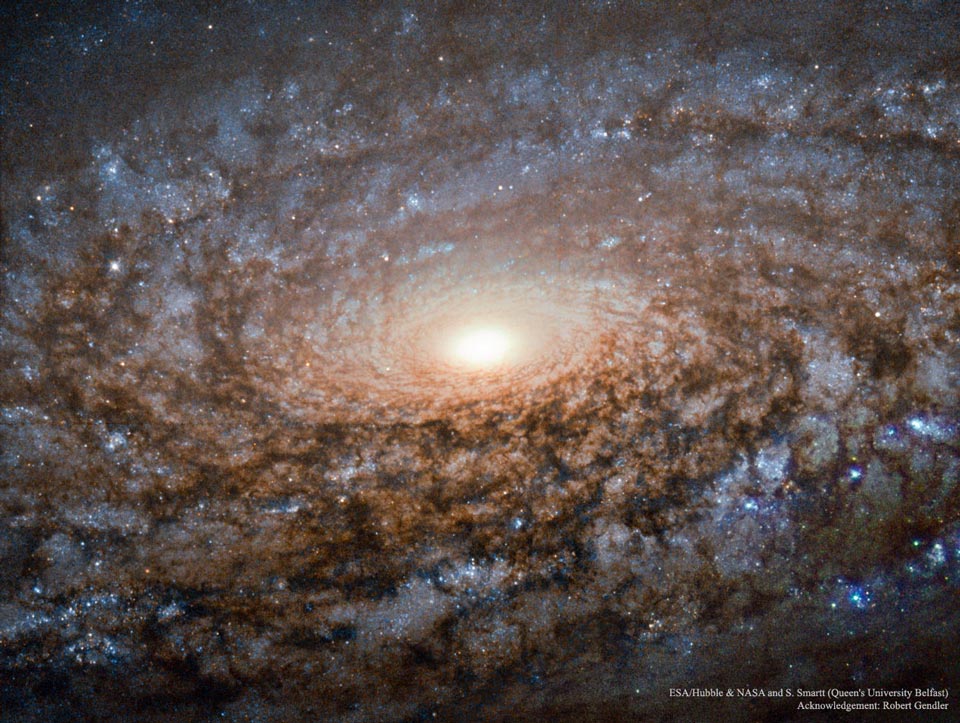
Ravens: With the game on the line, it was the special teams play that \"saved the day\" for Baltimore - Jamison Hensley (ESPN)
via IFTTT
NFL Video: Ravens Will Hill reacts to returning blocked FG for the game winning TD; \"I was just praying for a block\" (ESPN)
via IFTTT
Monday, November 30, 2015
NFL: Ravens block a FG, return it 64-yards for the game winning TD as time expires; Baltimore defeats Cleveland 33-27 (ESPN)
via IFTTT
NFL: Ravens block a FG, return it 64-yards for the game winning TD as time expires; Baltimore defeats Cleveland 33-27 (ESPN)
via IFTTT
anonymous,uncategorized,misc,general,other
from Google Alert - anonymous http://ift.tt/1TpN2pM
via IFTTT
Ravens: TE Maxx Williams has been ruled out for the remainder of the game with a concussion; live on ESPN/WatchESPN (ESPN)
via IFTTT
NFL Video: Ravens WR Kaelin Clay returns a punt up the sideline for an 82-yard TD; Baltimore leads Cleveland 7-0 on ESPN (ESPN)
via IFTTT
Ravens take on division rival Browns at 8:30 pm ET on ESPN/WatchESPN; Baltimore lost to Cleveland 33-30 in Week 5 (ESPN)
via IFTTT
Ravens take on division rival Browns at 8:30 pm ET on ESPN/WatchESPN; Baltimore lost to Cleveland 33-30 in Week 5 (ESPN)
via IFTTT
Browns take on division rival Ravens at 8:30 pm ET on ESPN/WatchESPN; Cleveland defeated Baltimore 33-30 in Week 5 (ESPN)
via IFTTT
HIV and AIDS
from Google Alert - anonymous http://ift.tt/1IkQFvT
via IFTTT
Semantic Folding Theory And its Application in Semantic Fingerprinting. (arXiv:1511.08855v1 [cs.AI])
Human language is recognized as a very complex domain since decades. No computer system has been able to reach human levels of performance so far. The only known computational system capable of proper language processing is the human brain. While we gather more and more data about the brain, its fundamental computational processes still remain obscure. The lack of a sound computational brain theory also prevents the fundamental understanding of Natural Language Processing. As always when science lacks a theoretical foundation, statistical modeling is applied to accommodate as many sampled real-world data as possible. An unsolved fundamental issue is the actual representation of language (data) within the brain, denoted as the Representational Problem. Starting with Jeff Hawkins' Hierarchical Temporal Memory (HTM) theory, a consistent computational theory of the human cortex, we have developed a corresponding theory of language data representation: The Semantic Folding Theory. The process of encoding words, by using a topographic semantic space as distributional reference frame into a sparse binary representational vector is called Semantic Folding and is the central topic of this document. Semantic Folding describes a method of converting language from its symbolic representation (text) into an explicit, semantically grounded representation that can be generically processed by Hawkins' HTM networks. As it turned out, this change in representation, by itself, can solve many complex NLP problems by applying Boolean operators and a generic similarity function like the Euclidian Distance. Many practical problems of statistical NLP systems, like the high cost of computation, the fundamental incongruity of precision and recall , the complex tuning procedures etc., can be elegantly overcome by applying Semantic Folding.
from cs.AI updates on arXiv.org http://ift.tt/1l3NI94
via IFTTT
Column-Oriented Datalog Materialization for Large Knowledge Graphs (Extended Technical Report). (arXiv:1511.08915v1 [cs.DB])
The evaluation of Datalog rules over large Knowledge Graphs (KGs) is essential for many applications. In this paper, we present a new method of materializing Datalog inferences, which combines a column-based memory layout with novel optimization methods that avoid redundant inferences at runtime. The pro-active caching of certain subqueries further increases efficiency. Our empirical evaluation shows that this approach can often match or even surpass the performance of state-of-the-art systems, especially under restricted resources.
from cs.AI updates on arXiv.org http://ift.tt/1SsW2K1
via IFTTT
Bootstrapping Ternary Relation Extractors. (arXiv:1511.08952v1 [cs.CL])
Binary relation extraction methods have been widely studied in recent years. However, few methods have been developed for higher n-ary relation extraction. One limiting factor is the effort required to generate training data. For binary relations, one only has to provide a few dozen pairs of entities per relation, as training data. For ternary relations (n=3), each training instance is a triplet of entities, placing a greater cognitive load on people. For example, many people know that Google acquired Youtube but not the dollar amount or the date of the acquisition and many people know that Hillary Clinton is married to Bill Clinton by not the location or date of their wedding. This makes higher n-nary training data generation a time consuming exercise in searching the Web. We present a resource for training ternary relation extractors. This was generated using a minimally supervised yet effective approach. We present statistics on the size and the quality of the dataset.
from cs.AI updates on arXiv.org http://ift.tt/1NZdv97
via IFTTT
Robotic Search & Rescue via Online Multi-task Reinforcement Learning. (arXiv:1511.08967v1 [cs.AI])
Reinforcement learning (RL) is a general and well-known method that a robot can use to learn an optimal control policy to solve a particular task. We would like to build a versatile robot that can learn multiple tasks, but using RL for each of them would be prohibitively expensive in terms of both time and wear-and-tear on the robot. To remedy this problem, we use the Policy Gradient Efficient Lifelong Learning Algorithm (PG-ELLA), an online multi-task RL algorithm that enables the robot to efficiently learn multiple consecutive tasks by sharing knowledge between these tasks to accelerate learning and improve performance. We implemented and evaluated three RL methods--Q-learning, policy gradient RL, and PG-ELLA--on a ground robot whose task is to find a target object in an environment under different surface conditions. In this paper, we discuss our implementations as well as present an empirical analysis of their learning performance.
from cs.AI updates on arXiv.org http://ift.tt/1l3NJd9
via IFTTT
Solving Transition-Independent Multi-agent MDPs with Sparse Interactions (Extended version). (arXiv:1511.09047v1 [cs.AI])
In cooperative multi-agent sequential decision making under uncertainty, agents must coordinate to find an optimal joint policy that maximises joint value. Typical algorithms exploit additive structure in the value function, but in the fully-observable multi-agent MDP setting (MMDP) such structure is not present. We propose a new optimal solver for transition-independent MMDPs, in which agents can only affect their own state but their reward depends on joint transitions. We represent these dependencies compactly in conditional return graphs (CRGs). Using CRGs the value of a joint policy and the bounds on partially specified joint policies can be efficiently computed. We propose CoRe, a novel branch-and-bound policy search algorithm building on CRGs. CoRe typically requires less runtime than the available alternatives and finds solutions to problems previously unsolvable.
from cs.AI updates on arXiv.org http://ift.tt/1NZdxOa
via IFTTT