Latest YouTube Video
Saturday, March 3, 2018
Anonymous Art Show
from Google Alert - anonymous http://ift.tt/2FcZ5G3
via IFTTT
Orioles: 1B Chris Davis (elbow/forearm) sidelined 3-5 days; MRI revealed no structural damage (ESPN)
via IFTTT
Ravens should feel the heat from 3-year playoff drought, says GM Ozzie Newsome (ESPN)
via IFTTT
Friday, March 2, 2018
Ravens: Robbery charge against CB Marlon Humphrey dismissed (ESPN)
via IFTTT
[FD] KL-001-2018-007 : Sophos UTM 9 loginuser Privilege Escalation via confd Service
Source: Gmail -> IFTTT-> Blogger
[FD] Content Injection in Samsung Display Solutions Application for Android [CVE-2018-6019]
Source: Gmail -> IFTTT-> Blogger
[FD] CSRF vulnerabilities in D-Link DGS-3000-10TC
Source: Gmail -> IFTTT-> Blogger
[FD] Another TCP based IDS bypass technique. CVE-2018-6794
Source: Gmail -> IFTTT-> Blogger
[FD] CVE-2018-7449 SEGGER embOS/IP FTP Server v3.22 / FTP CMDs Denial Of Service
Source: Gmail -> IFTTT-> Blogger
[FD] DualDesk v20 "Proxy.exe" Server / Denial Of Service - CVE-2018-7583
Source: Gmail -> IFTTT-> Blogger
Ravens: Ozzie Newsome says biggest project in his final season as GM is to get Joe Flacco better targets (ESPN)
via IFTTT
ISS Daily Summary Report – 3/01/2018
from ISS On-Orbit Status Report http://ift.tt/2Ff0cZ4
via IFTTT
Biggest-Ever DDoS Attack (1.35 Tbs) Hits Github Website
from The Hacker News http://ift.tt/2F5ZzNY
via IFTTT
Thursday, March 1, 2018
Missing profile images for anonymous visitors when Social Private Files is turned on
from Google Alert - anonymous http://ift.tt/2F3ix7P
via IFTTT
ALCOHOLICS ANONYMOUS 208-235-1444 AL-ANON 208-232-2692
from Google Alert - anonymous http://ift.tt/2oIj1t4
via IFTTT
JotForm Review: Poor poor service by Anonymous Reviewer
from Google Alert - anonymous http://ift.tt/2FexaZe
via IFTTT
The Lunar X
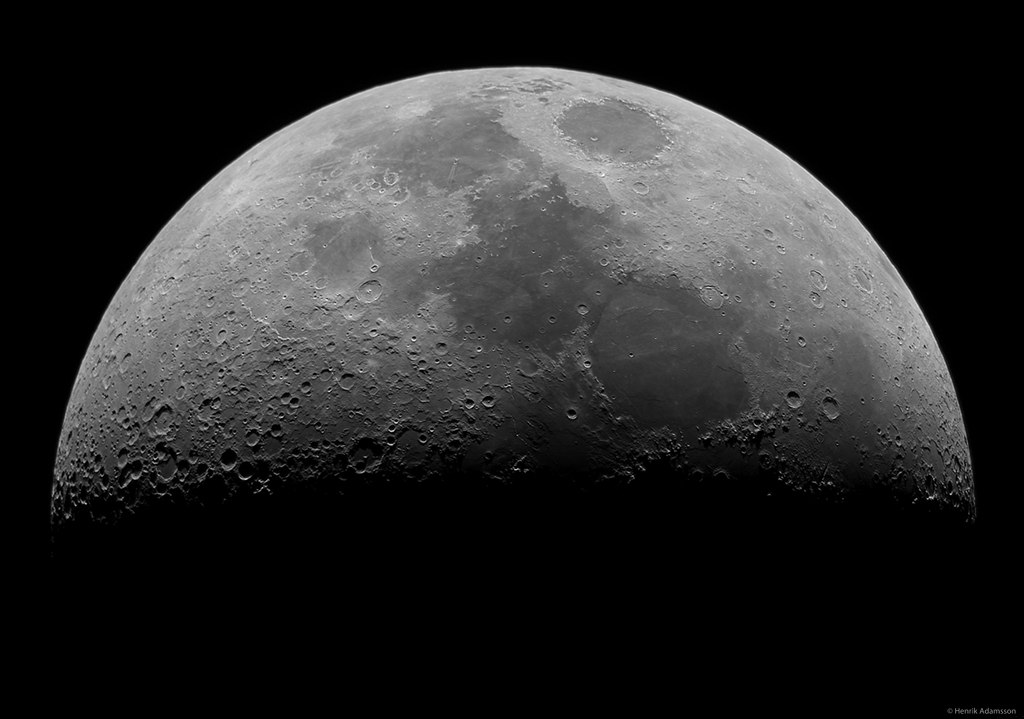
Wednesday, February 28, 2018
Anonymous - Executive Chef
from Google Alert - anonymous http://ift.tt/2oxAkOv
via IFTTT
Greatest Castlevania ever
from Google Alert - anonymous http://ift.tt/2GQLft5
via IFTTT
Anonymous female agency veteran on what needs to change
from Google Alert - anonymous http://ift.tt/2EZaehm
via IFTTT
Anonymous-Feedback-LTI 0.1.1
from Google Alert - anonymous http://ift.tt/2ow8t12
via IFTTT
Why Ravens will be active, but not break the bank in free agency - Jamison Hensley (ESPN)
via IFTTT
Apple Moves iCloud Data and Encryption Keys for Chinese Users to China
from The Hacker News http://ift.tt/2CsN0Pl
via IFTTT
A Simple Bug Revealed Admins of Facebook Pages — Find Out How
from The Hacker News http://ift.tt/2HRhxWj
via IFTTT
Orioles: Why RP Mychal Givens makes list of MLB's most under-the-radar players - David Schoenfield (ESPN)
via IFTTT
Format Manuscript/Mixed Material
from Google Alert - anonymous http://ift.tt/2EXDYew
via IFTTT
Webform 4.16 file upload gives Invalid POST data for anonymous users
from Google Alert - anonymous http://ift.tt/2CO75uM
via IFTTT
[FD] SEC Consult SA-20180228-0 :: Insecure Direct Object Reference vulnerability in TestLink Open Source Test Management
Source: Gmail -> IFTTT-> Blogger
Classes At Saginaw's Thompson Middle School Cancelled Due To Anonymous Threat
from Google Alert - anonymous http://ift.tt/2BUdKXQ
via IFTTT
Wilbon cites anonymous players & teams who think the Rockets are
from Google Alert - anonymous http://ift.tt/2sZk9Ox
via IFTTT
Memcached Servers Abused for Massive Amplification DDoS Attacks
from The Hacker News http://ift.tt/2CqaULg
via IFTTT
Tuesday, February 27, 2018
How to spam someones email anonymously
from Google Alert - anonymous http://ift.tt/2ovfCPg
via IFTTT
Trip advisor is NOT anonymous! - TripAdvisor Support Forum
from Google Alert - anonymous http://ift.tt/2CLpYPe
via IFTTT
2018 NFL combine preview: Ravens should focus on every offensive position - Jamison Hensley (ESPN)
via IFTTT
Google anonymous proxy
from Google Alert - anonymous http://ift.tt/2BWcxyX
via IFTTT
[FD] Download Protection Bypass in Google’s Chrome (multiple)
Source: Gmail -> IFTTT-> Blogger
[FD] Auto-detection of Compressed Files in Apple’s macOS
Source: Gmail -> IFTTT-> Blogger
[FD] ActivePDF Toolkit < 8.1.0 multiple RCE
Source: Gmail -> IFTTT-> Blogger
Security Analyst
from Google Alert - anonymous http://ift.tt/2CoELUq
via IFTTT
ISS Daily Summary Report – 2/26/2018
from ISS On-Orbit Status Report http://ift.tt/2HP8J3i
via IFTTT
I have a new follower on Twitter

Mark Bland
▪️ Host of @theqnow ▪️Music Exec w/ a couple plaques▪️Former Pro Wrestler ▪️ #Stl Native 💯▪️.262 batting average in softball https://t.co/Tvkw8CsV8O
St Louis, MO/World Wide
https://t.co/xIuwPorgDD
Following: 16676 - Followers: 87837
February 27, 2018 at 10:07AM via Twitter http://twitter.com/markbland
Hacker Who Never Hacked Anyone Gets 33-Month Prison Sentence
from The Hacker News http://ift.tt/2F5PeVD
via IFTTT
mlp/ - Magicless Anonymous #28
from Google Alert - anonymous http://ift.tt/2HRBkoG
via IFTTT
8th St.'s surf is at least 9.54ft high
8th St. Summary
At 2:00 AM, surf min of 9.54ft. At 8:00 AM, surf min of 9.59ft. At 2:00 PM, surf min of 9.54ft. At 8:00 PM, surf min of 9.35ft.
Surf maximum: 11.39ft (3.47m)
Surf minimum: 9.54ft (2.91m)
Tide height: 0.32ft (0.1m)
Wind direction: NNE
Wind speed: 7.29 KTS
from Surfline http://ift.tt/1kVmigH
via IFTTT
[FD] SEC Consult SA-20180227-0 :: OS command injection, arbitrary file upload & SQL injection in ClipBucket
Source: Gmail -> IFTTT-> Blogger
AKG Anonymous Beethoven Painting c1910 t
from Google Alert - anonymous http://ift.tt/2FBH6Ks
via IFTTT
Any anonymous claiming of UBTC offers out there BitcoinAirdrops
from Google Alert - anonymous http://ift.tt/2EWPanJ
via IFTTT
Looking for a way to post anonymously
from Google Alert - anonymous http://ift.tt/2Fz3CDw
via IFTTT
Can't disable google maps file loading
from Google Alert - anonymous http://ift.tt/2EU3eT0
via IFTTT
Phone-Cracking Firm Found a Way to Unlock Any iPhone Model
from The Hacker News http://ift.tt/2CKQXun
via IFTTT
Monday, February 26, 2018
Customizing the anonymous welcome page
from Google Alert - anonymous http://ift.tt/2CJteKY
via IFTTT
large crowd of anonymous blurred people at a trade show hall
from Google Alert - anonymous http://ift.tt/2Co56Sw
via IFTTT
San Benito High School Anonymous Tip Line
from Google Alert - anonymous http://ift.tt/2oyqx9X
via IFTTT
Orioles P Kevin Gausman leaves Monday's game vs. Tigers after collision at home plate (ESPN)
via IFTTT
Chauffeur in Worthing (BN14) | Anonymous
from Google Alert - anonymous http://ift.tt/2orHsfa
via IFTTT
Assistant general manager
from Google Alert - anonymous http://ift.tt/2ovtmbQ
via IFTTT
fusion360-anonymous on Sketchfab - Sketchfab
from Google Alert - anonymous http://ift.tt/2osFOd4
via IFTTT
Offseason QB Confidence Index: How the Ravens feel about their depth chart (ESPN)
via IFTTT
Flaw in Popular μTorrent Software Lets Hackers Control Your PC Remotely
from The Hacker News http://ift.tt/2F6ZaOp
via IFTTT
Face detection with OpenCV and deep learning
Today I’m going to share a little known secret with you regarding the OpenCV library:
You can perform fast, accurate face detection with OpenCV using a pre-trained deep learning face detector model shipped with the library.
You may already know that OpenCV ships out-of-the-box with pre-trained Haar cascades that can be used for face detection…
…but I’m willing to bet that you don’t know about the “hidden” deep learning-based face detector that has been part of OpenCV since OpenCV 3.3.
In the remainder of today’s blog post I’ll discuss:
- Where this “hidden” deep learning face detector lives in the OpenCV library
- How you can perform face detection in images using OpenCV and deep learning
- How you can perform face detection in video using OpenCV and deep learning
As we’ll see, it’s easily to swap out Haar cascades for their more accurate deep learning face detector counterparts.
To learn more about face detection with OpenCV and deep learning, just keep reading!
Looking for the source code to this post?
Jump right to the downloads section.
Face detection with OpenCV and deep learning
Today’s blog post is broken down into three parts.
In the first part we’ll discuss the origin of the more accurate OpenCV face detectors and where they live inside the OpenCV library.
From there I’ll demonstrate how you can perform face detection in images using OpenCV and deep learning.
I’ll then wrap up the blog post discussing how you can apply face detection to video streams using OpenCV and deep learning as well.
Where do these “better” face detectors live in OpenCV and where did they come from?
Back in August 2017, OpenCV 3.3 was officially released, bringing it with it a highly improved “deep neural networks” (
dnn) module.
This module supports a number of deep learning frameworks, including Caffe, TensorFlow, and Torch/PyTorch.
The primary contributor to the
dnnmodule, Aleksandr Rybnikov, has put a huge amount of work into making this module possible (and we owe him a big round of thanks and applause).
And since the release of OpenCV 3.3, I’ve been sharing a number of deep learning OpenCV tutorials, including:
- Deep Learning with OpenCV
- Object detection with deep learning and OpenCV
- Real-time object detection with deep learning and OpenCV
- Deep learning on the Raspberry Pi with OpenCV
- Raspberry Pi: Deep learning object detection with OpenCV
- Deep learning: How OpenCV’s blobFromImage works
However, what most OpenCV users do not know is that Rybnikov has included a more accurate, deep learning-based face detector included in the official release of OpenCV (although it can be a bit hard to find if you don’t know where to look).
The Caffe-based face detector can be found in the
face_detectorsub-directory of the dnn samples:
When using OpenCV’s deep neural network module with Caffe models, you’ll need two sets of files:
- The .prototxt file(s) which define the model architecture (i.e., the layers themselves)
- The .caffemodel file which contains the weights for the actual layers
Both files are required to when using models trained using Caffe for deep learning.
However, you’ll only find the prototxt files here in the GitHub repo.
The weight files are not included in the OpenCV
samplesdirectory and it requires a bit more digging to find them…
Where can I can I get the more accurate OpenCV face detectors?
For your convenience, I have included both the:
- Caffe prototxt files
- and Caffe model weight files
…inside the “Downloads” section of this blog post.
To skip to the downloads section, just click here.
How does the OpenCV deep learning face detector work?
OpenCV’s deep learning face detector is based on the Single Shot Detector (SSD) framework with a ResNet base network (unlike other OpenCV SSDs that you may have seen which typically use MobileNet as the base network).
A full review of SSDs and ResNet is outside the scope of this blog post, so if you’re interested in learning more about Single Shot Detectors (including how to train your own custom deep learning object detectors), start with this article here on the PyImageSearch blog and then take a look at my book, Deep Learning for Computer Vision with Python, which includes in-depth discussions and code enabling you to train your own object detectors.
Face detection in images with OpenCV and deep learning
In this first example we’ll learn how to apply face detection with OpenCV to single input images.
In the next section we’ll learn how to modify this code and apply face detection with OpenCV to videos, video streams, and webcams.
Open up a new file, name it
detect_faces.py, and insert the following code:
# import the necessary packages import numpy as np import argparse import cv2 # construct the argument parse and parse the arguments ap = argparse.ArgumentParser() ap.add_argument("-i", "--image", required=True, help="path to input image") ap.add_argument("-p", "--prototxt", required=True, help="path to Caffe 'deploy' prototxt file") ap.add_argument("-m", "--model", required=True, help="path to Caffe pre-trained model") ap.add_argument("-c", "--confidence", type=float, default=0.5, help="minimum probability to filter weak detections") args = vars(ap.parse_args())
Here we are importing our required packages (Lines 2-4) and parsing command line arguments (Lines 7-16).
We have three required arguments:
-
--image
: The path to the input image. -
--prototxt
: The path to the Caffe prototxt file. -
--model
: The path to the pretrained Caffe model.
An optional argument,
--confidence, can overwrite the default threshold of 0.5 if you wish.
From there lets load our model and create a blob from our image:
# load our serialized model from disk print("[INFO] loading model...") net = cv2.dnn.readNetFromCaffe(args["prototxt"], args["model"]) # load the input image and construct an input blob for the image # by resizing to a fixed 300x300 pixels and then normalizing it image = cv2.imread(args["image"]) (h, w) = image.shape[:2] blob = cv2.dnn.blobFromImage(cv2.resize(image, (300, 300)), 1.0, (300, 300), (104.0, 177.0, 123.0))
First, we load our model using our
--prototxtand
--modelfile paths. We store the model as
net(Line 20).
Then we load the
image(Line 24), extract the dimensions (Line 25), and create a
blob(Lines 26 and 27).
The
dnn.blobFromImagetakes care of pre-processing which includes setting the
blobdimensions and normalization. If you’re interested in learning more about the
dnn.blobFromImagefunction, I review in detail in this blog post.
Next, we’ll apply face detection:
# pass the blob through the network and obtain the detections and # predictions print("[INFO] computing object detections...") net.setInput(blob) detections = net.forward()
To detect faces, we pass the
blobthrough the
neton Lines 32 and 33.
And from there we’ll loop over the
detectionsand draw boxes around the detected faces:
# loop over the detections for i in range(0, detections.shape[2]): # extract the confidence (i.e., probability) associated with the # prediction confidence = detections[0, 0, i, 2] # filter out weak detections by ensuring the `confidence` is # greater than the minimum confidence if confidence > args["confidence"]: # compute the (x, y)-coordinates of the bounding box for the # object box = detections[0, 0, i, 3:7] * np.array([w, h, w, h]) (startX, startY, endX, endY) = box.astype("int") # draw the bounding box of the face along with the associated # probability text = "{:.2f}%".format(confidence * 100) y = startY - 10 if startY - 10 > 10 else startY + 10 cv2.rectangle(image, (startX, startY), (endX, endY), (0, 0, 255), 2) cv2.putText(image, text, (startX, y), cv2.FONT_HERSHEY_SIMPLEX, 0.45, (0, 0, 255), 2) # show the output image cv2.imshow("Output", image) cv2.waitKey(0)
We begin looping over the detections on Line 36.
From there, we extract the
confidence(Line 39) and compare it to the confidence threshold (Line 43). We perform this check to filter out weak detections.
If the confidence meets the minimum threshold, we proceed to draw a rectangle and along with the probability of the detection on Lines 46-56.
To accomplish this, we first calculate the (x, y)-coordinates of the bounding box (Lines 46 and 47).
We then build our confidence
textstring (Line 51) which contains the probability of the detection.
In case the our
textwould go off-image (such as when the face detection occurs at the very top of an image), we shift it down by 10 pixels (Line 52).
Our face rectangle and confidence
textis drawn on the
imageon Lines 53-56.
From there we loop back for additional detections following the process again. If no
detectionsremain, we’re ready to show our output
imageon the screen (Lines 59 and 60).
Face detection in images with OpenCV results
Let’s try out the OpenCV deep learning face detector.
Make sure you use the “Downloads” section of this blog post to download:
- The source code used in this blog post
- The Caffe prototxt files for deep learning face detection
- The Caffe weight files used for deep learning face detection
- The example images used in this post
From there, open up a terminal and execute the following command:
$ python detect_faces.py --image rooster.jpg --prototxt deploy.prototxt.txt \ --model res10_300x300_ssd_iter_140000.caffemodel
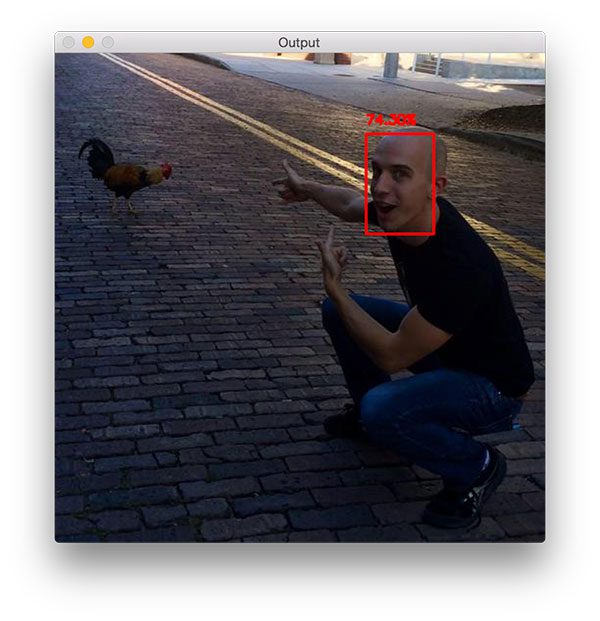
Figure 3: My face is detected in this image with 74% confidence using the OpenCV deep learning face detector.
The above photo is of me during my first trip to Ybor City in Florida, where chickens are allowed to roam free throughout the city. There are even laws protecting the chickens which I thought was very cool. Even though I grew up in rural farmland, I was still totally surprised to see a rooster crossing the road — which of course spawned many “Why did the chicken cross the road?” jokes.
Here you can see my face is detected with 74.30% confidence, even though my face is at an angle. OpenCV’s Haar cascades are notorious for missing faces that are not at a “straight on” angle, but by using OpenCV’s deep learning face detectors, we are able to detect my face.
And now we’ll see how another example works, this time with three faces:
$ python detect_faces.py --image iron_chic.jpg --prototxt deploy.prototxt.txt \ --model res10_300x300_ssd_iter_140000.caffemodel
This photo was taken in Gainesville, FL after one of my favorite bands finished up a show at Loosey’s, a popular bar and music venue in the area. Here you can see my fiance (left), me (middle), and Jason (right), a member of the band.
I’m incredibly impressed that OpenCV can detect Trisha’s face, despite the lighting conditions and shadows cast on her face in the dark venue (and with 86.81% probability!)
Again, this just goes to show how much better (in terms of accuracy) the deep learning OpenCV face detectors are over their standard Haar cascade counterparts shipped with the library.
Face detection in video and webcam with OpenCV and deep learning
Now that we have learned how to apply face detection with OpenCV to single images, let’s also apply face detection to videos, video streams, and webcams.
Luckily for us, most of our code in the previous section on face detection with OpenCV in single images can be reused here!
Open up a new file, name it
detect_faces_video.py, and insert the following code:
# import the necessary packages from imutils.video import VideoStream import numpy as np import argparse import imutils import time import cv2 # construct the argument parse and parse the arguments ap = argparse.ArgumentParser() ap.add_argument("-p", "--prototxt", required=True, help="path to Caffe 'deploy' prototxt file") ap.add_argument("-m", "--model", required=True, help="path to Caffe pre-trained model") ap.add_argument("-c", "--confidence", type=float, default=0.5, help="minimum probability to filter weak detections") args = vars(ap.parse_args())
Compared to above, we will need to import three additional packages:
VideoStream,
imutils, and
time.
If you don’t have
imutilsin your virtual environment, you can install it via:
$ pip install imutils
Our command line arguments are mostly the same, except we do not have an
--imagepath argument this time. We’ll be using our webcam’s video feed instead.
From there we’ll load our model and initialize the video stream:
# load our serialized model from disk print("[INFO] loading model...") net = cv2.dnn.readNetFromCaffe(args["prototxt"], args["model"]) # initialize the video stream and allow the camera sensor to warm up print("[INFO] starting video stream...") vs = VideoStream(src=0).start() time.sleep(2.0)
Loading the model is the same as above.
We initialize a
VideoStreamobject specifying camera with index zero as the source (in general this would be your laptop’s built in camera or your desktop’s first camera detected).
A few quick notes here:
- Raspberry Pi + picamera users can replace Line 25 with
vs = VideoStream(usePiCamera=True).start()
if you wish to use the Raspberry Pi camera module. - If you to parse a video file (rather than a video stream) swap out the
VideoStream
class forFileVideoStream
. You can learn more about the FileVideoStream class in this blog post.
We then allow the camera sensor to warm up for 2 seconds (Line 26).
From there we loop over the frames and compute face detections with OpenCV:
# loop over the frames from the video stream while True: # grab the frame from the threaded video stream and resize it # to have a maximum width of 400 pixels frame = vs.read() frame = imutils.resize(frame, width=400) # grab the frame dimensions and convert it to a blob (h, w) = frame.shape[:2] blob = cv2.dnn.blobFromImage(cv2.resize(frame, (300, 300)), 1.0, (300, 300), (104.0, 177.0, 123.0)) # pass the blob through the network and obtain the detections and # predictions net.setInput(blob) detections = net.forward()
This block should look mostly familiar to the static image version in the previous section.
In this block, we’re reading a
framefrom the video stream (Line 32), creating a
blob(Lines 37 and 38), and passing the
blobthrough the deep neural
netto obtain face detections (Lines 42 and 43).
We can now loop over the detections, compare to the confidence threshold, and draw face boxes + confidence values on the screen:
# loop over the detections for i in range(0, detections.shape[2]): # extract the confidence (i.e., probability) associated with the # prediction confidence = detections[0, 0, i, 2] # filter out weak detections by ensuring the `confidence` is # greater than the minimum confidence if confidence < args["confidence"]: continue # compute the (x, y)-coordinates of the bounding box for the # object box = detections[0, 0, i, 3:7] * np.array([w, h, w, h]) (startX, startY, endX, endY) = box.astype("int") # draw the bounding box of the face along with the associated # probability text = "{:.2f}%".format(confidence * 100) y = startY - 10 if startY - 10 > 10 else startY + 10 cv2.rectangle(frame, (startX, startY), (endX, endY), (0, 0, 255), 2) cv2.putText(frame, text, (startX, y), cv2.FONT_HERSHEY_SIMPLEX, 0.45, (0, 0, 255), 2)
For a detailed review of this code block, please review the previous section where we perform face detection to still, static images. The code here is nearly identical.
Now that our OpenCV face detections have been drawn, let’s display the frame on the screen and wait for a keypress:
# show the output frame cv2.imshow("Frame", frame) key = cv2.waitKey(1) & 0xFF # if the `q` key was pressed, break from the loop if key == ord("q"): break # do a bit of cleanup cv2.destroyAllWindows() vs.stop()
We display the
frameon the screen until the “q” key is pressed at which point we
breakout of the loop and perform cleanup.
Face detection in video and webcam with OpenCV results
To try out the OpenCV deep learning face detector make sure you use the “Downloads” section of this blog post to grab:
- The source code used in this blog post
- The Caffe prototxt files for deep learning face detection
- The Caffe weight files used for deep learning face detection
Once you have downloaded the files, running the deep learning OpenCV face detector with a webcam feed is easy with this simple command:
$ python detect_faces_video.py --prototxt deploy.prototxt.txt \ --model res10_300x300_ssd_iter_140000.caffemodel
You can see a full video demonstration, including my commentary, in the following video:
Summary
In today’s blog post you discovered a little known secret about the OpenCV library — OpenCV ships out-of-the-box with a more accurate face detector (as compared to OpenCV’s Haar cascades).
The more accurate OpenCV face detector is deep learning based, and in particular, utilizes the Single Shot Detector (SSD) framework with ResNet as the base network.
Thanks to the hard work of Aleksandr Rybnikov and the other contributors to OpenCV’s
dnnmodule, we can enjoy these more accurate OpenCV face detectors in our own applications.
The deep learning face detectors can be hard to find in the OpenCV library, so
for your convenience, I have put gathered the Caffe prototxt and weight files for you — just use the “Downloads” form below to download the (more accurate) deep learning-based OpenCV face detector.
See you next week with another great computer vision + deep learning tutorial!
Downloads:
The post Face detection with OpenCV and deep learning appeared first on PyImageSearch.
from PyImageSearch http://ift.tt/2CnHc9G
via IFTTT
Will anonymous external sharing be disabled: New ways to govern access of external users
from Google Alert - anonymous http://ift.tt/2CJ5u9M
via IFTTT
ISS Daily Summary Report – 2/23/2018
from ISS On-Orbit Status Report http://ift.tt/2CJ99Eu
via IFTTT
8th St.'s surf is at least 9.04ft high
8th St. Summary
At 2:00 AM, surf min of 9.04ft. At 8:00 AM, surf min of 9.36ft. At 2:00 PM, surf min of 9.41ft. At 8:00 PM, surf min of 9.43ft.
Surf maximum: 10.8ft (3.29m)
Surf minimum: 9.04ft (2.75m)
Tide height: -0.52ft (-0.16m)
Wind direction: N
Wind speed: 11.35 KTS
from Surfline http://ift.tt/1kVmigH
via IFTTT
nodebb-plugin-anonymous
from Google Alert - anonymous http://ift.tt/2CHW4LQ
via IFTTT
Android P Will Block Background Apps from Accessing Your Camera, Microphone
from The Hacker News http://ift.tt/2BOZfEC
via IFTTT
Passing Jupiter
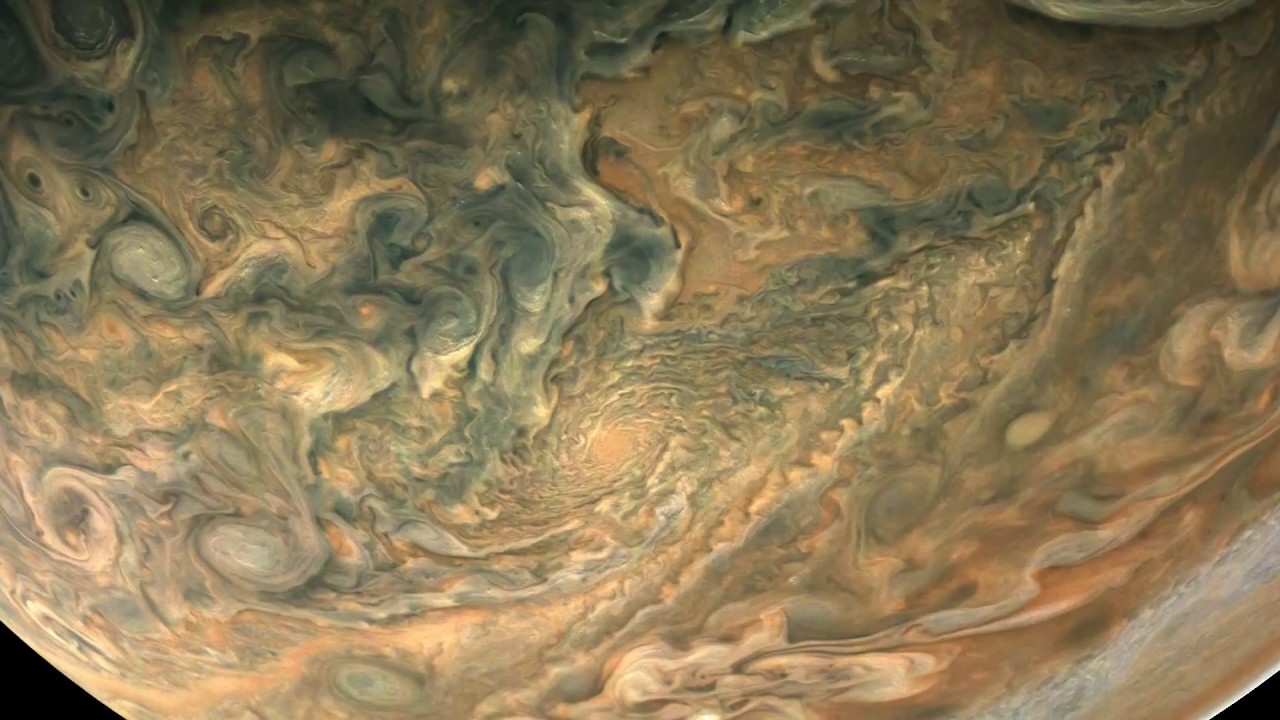
Sunday, February 25, 2018
Orioles and 1B/DH Pedro Alvarez agree to minor league contract - multiple reports (ESPN)
via IFTTT
philadelphia76ersvswashingtonlivestream
from Google Alert - anonymous http://ift.tt/2BPTUwK
via IFTTT
Toby K. (Unity Day, 2018)
from Google Alert - anonymous http://ift.tt/2F4vhyd
via IFTTT
atlantanascar2018livestream
from Google Alert - anonymous http://ift.tt/2ETa4UI
via IFTTT
Anonymous user 3743a8
from Google Alert - anonymous http://ift.tt/2ETa1Iw
via IFTTT
Progress Continues On Anonymous Bitcoin Transactions
from Google Alert - anonymous http://ift.tt/2F0Xfv3
via IFTTT
[FD] Search engine of leaks
Source: Gmail -> IFTTT-> Blogger
[FD] [CVE-2018-1000088] Stored XSS vulnerability in Doorkeeper gem v2.1.0 - v4.2.5
Source: Gmail -> IFTTT-> Blogger
[FD] BSides Denver 2018 CFP is open
Source: Gmail -> IFTTT-> Blogger
8th St.'s surf is at least 5.69ft high
8th St. Summary
At 2:00 AM, surf min of 3.47ft. At 8:00 AM, surf min of 5.69ft. At 2:00 PM, surf min of 6.05ft. At 8:00 PM, surf min of 7.73ft.
Surf maximum: 6.81ft (2.07m)
Surf minimum: 5.69ft (1.74m)
Tide height: 4.03ft (1.23m)
Wind direction: NNE
Wind speed: 17.69 KTS
from Surfline http://ift.tt/1kVmigH
via IFTTT
"Unaccountable at the Founding: The Originalist Case for Anonymous Juri" by Kory A. Langhofer
from Google Alert - anonymous http://ift.tt/2ETRHzd
via IFTTT
Geoip2 anonymous ip database download
from Google Alert - anonymous http://ift.tt/2CHztyT
via IFTTT
AE Aurigae and the Flaming Star Nebula
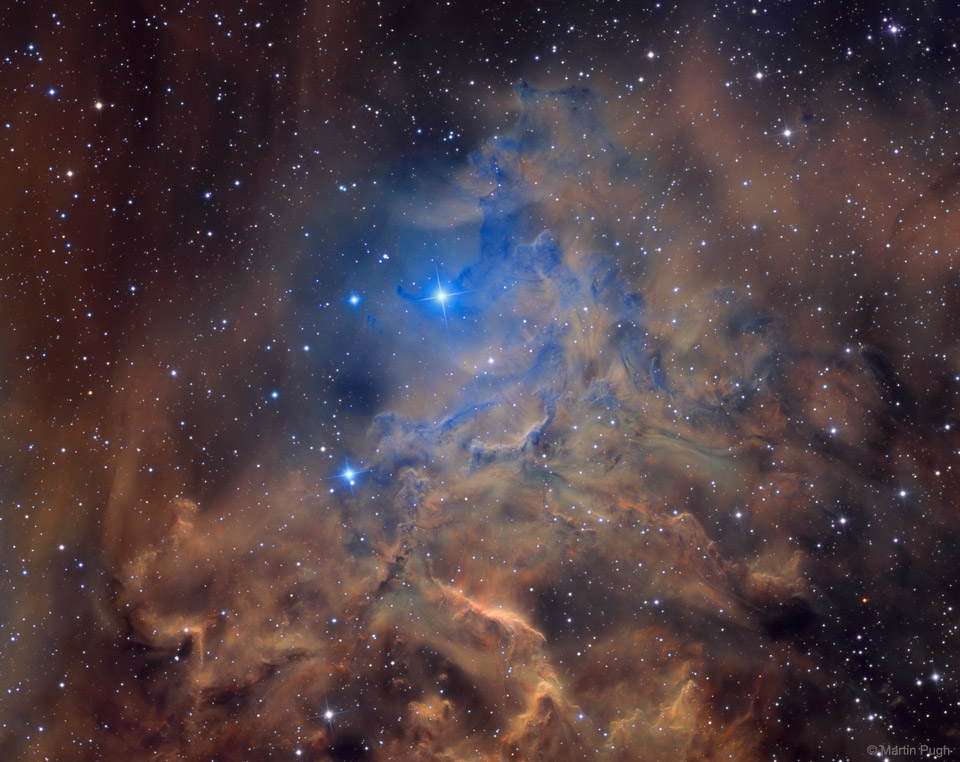