Latest YouTube Video
Saturday, December 10, 2016
Ravens Image: Steve Smith Sr. auctions off his cleats that were worn in Week 13 to support a domestic violence center (ESPN)
via IFTTT
[FD] CSRF vulnerability in Multisite Post Duplicator could allow an attacker to do almost anything an admin user can do (WordPress plugin)
Source: Gmail -> IFTTT-> Blogger
Rumor Central: Free agent C Welington Castillo is Orioles' top target at position - BaltimoreBaseball.com; 68 RBI in '16 (ESPN)
via IFTTT
Can I make an anonymous polls and get a list of all participant names (without knowing who ...
from Google Alert - anonymous http://ift.tt/2hjQJ4a
via IFTTT
Anonymous Leaks to the WashPost About the CIA's Russia Beliefs Are No Substitute for Evidence
from Google Alert - anonymous http://ift.tt/2gqltDo
via IFTTT
8 Venetian Songs (Anonymous)
from Google Alert - anonymous http://ift.tt/2hjNbyE
via IFTTT
Crime Coast: Mafia Wars (hack) Gold, Cash Generator -> mobile
from Google Alert - anonymous http://ift.tt/2gwbg6N
via IFTTT
New! Battle for the Throne cheat Runes Generator ~> online
from Google Alert - anonymous http://ift.tt/2gvXBfX
via IFTTT
President Obama Orders 'Full Review' of Possible Russian hacking in US Election
from The Hacker News http://ift.tt/2hi6gSc
via IFTTT
Stop Using these 2 Easily Hackable Netgear Router Models — US CERT Warns
from The Hacker News http://ift.tt/2h92YCA
via IFTTT
Anonymous Couple Brings Christmas Miracle To Gaston County Kids
from Google Alert - anonymous http://ift.tt/2gkW3C4
via IFTTT
Assimilation of OCO-2 Carbon Dioxide into the GEOS Simulation
from NASA's Scientific Visualization Studio: Most Recent Items http://ift.tt/2hf0EKH
via IFTTT
Friday, December 9, 2016
I have a new follower on Twitter
Kristin Taylor
Growing companies call on me to create & run rock star marketing programs that kickstart and supercharge their sales. Love Portland and Illini. #OnCallMarketing
Portland & Vancouver USA
https://t.co/6ANsMOMlJb
Following: 10479 - Followers: 12275
December 09, 2016 at 11:49PM via Twitter http://twitter.com/xKristinTaylorx
Secret founder returns to anonymous publishing with launch of IO
from Google Alert - anonymous http://ift.tt/2h7YM6d
via IFTTT
I have a new follower on Twitter
Coralogix
Coralogix helps software companies avoid getting lost in their log data by figuring out their production problems automatically
San Francisco, CA
https://t.co/uNE1Je1VLI
Following: 2530 - Followers: 1916
December 09, 2016 at 09:24PM via Twitter http://twitter.com/Coralogix
8 Venetian Songs (Anonymous)
from Google Alert - anonymous http://ift.tt/2hvup6n
via IFTTT
AP sources: Mattis received anonymous email in Petraeus case
from Google Alert - anonymous http://ift.tt/2gmgkvW
via IFTTT
Viagra Where To Really Anonymous
from Google Alert - anonymous http://ift.tt/2hvcEUH
via IFTTT
Food Bank receiving $100K grant from anonymous donor
from Google Alert - anonymous http://ift.tt/2h6wOaF
via IFTTT
Tip-offs anonymous
from Google Alert - anonymous http://ift.tt/2gsTdyu
via IFTTT
Brothers who tortured boys to stay anonymous
from Google Alert - anonymous http://ift.tt/2hc1qFF
via IFTTT
Ravens Podcast: NFL Nation reporter Jamison Hensley breaks down matchup against Patriots and makes his Week 14 pick (ESPN)
via IFTTT
I have a new follower on Twitter
Stephane Bureau
International Marketing Executive coaching HPE partners with Cloud Solutions. Social Business, Visual Marketing, Entrepreneur, Photographer. Tweets are my own.
Grenoble, France _ EMEA
Following: 4369 - Followers: 4777
December 09, 2016 at 10:33AM via Twitter http://twitter.com/SBUCloud
I have a new follower on Twitter
FTP Today
File Share with FTP Today: Compatibility you need. Security you can trust. File sharing built to fit your business.
Cincinnati, OH
http://t.co/VgD9a5ln13
Following: 3625 - Followers: 3937
December 09, 2016 at 10:33AM via Twitter http://twitter.com/ftptoday
[FD] Broken access control on bluemix containers
Source: Gmail -> IFTTT-> Blogger
[FD] MSIE 9 MSHTML CElement::HasFlag memory corruption
Source: Gmail -> IFTTT-> Blogger
This Ransomware Unlocks Your Files For Free If You Infect Others
from The Hacker News http://ift.tt/2gibRWs
via IFTTT
ISS Daily Summary Report – 12/08/2016
from ISS On-Orbit Status Report http://ift.tt/2hsRUNo
via IFTTT
Uber Now Tracks Your Location Even After Your Ride
from The Hacker News http://ift.tt/2hb0uRM
via IFTTT
[FD] [ESNC-2041217] Critical Security Vulnerability in PwC ACE Software for SAP Security
Source: Gmail -> IFTTT-> Blogger
[FD] Dual DHCP DNS Server 7.29 Buffer Overflow (Dos)
Source: Gmail -> IFTTT-> Blogger
[FD] Roundcube 1.2.2: Command Execution via Email
Source: Gmail -> IFTTT-> Blogger
[FD] Splunk Enterprise Server-Side Request Forgery
Source: Gmail -> IFTTT-> Blogger
[FD] CVE-2013-1306: MSIE 9 MSHTML CDispNode::InsertSiblingNode use-after-free details
Source: Gmail -> IFTTT-> Blogger
[FD] CVE-2013-1309:
Source: Gmail -> IFTTT-> Blogger
[FD] CVE-2015-1730: MSIE jscript9 JavaScriptStackWalker memory corruption details and PoC
Source: Gmail -> IFTTT-> Blogger
Oversharers Anonymous
from Google Alert - anonymous http://ift.tt/2hlRsVc
via IFTTT
Binary options anonymous
from Google Alert - anonymous http://ift.tt/2gqFqIH
via IFTTT
Thursday, December 8, 2016
I have a new follower on Twitter
you & your family
We educate expectant and new parents on pregnancy, fitness and nutrition, labor and birth and family and financial matters.
http://t.co/RVOpAu23sT
Following: 2725 - Followers: 2875
December 08, 2016 at 11:48PM via Twitter http://twitter.com/YouandYourFam
Anonymous Stranger Pays All Overdue Lunch Balances At Elementary School
from Google Alert - anonymous http://ift.tt/2hrdJgh
via IFTTT
Interactive Elicitation of Knowledge on Feature Relevance Improves Predictions in Small Data Sets. (arXiv:1612.02487v1 [cs.AI])
Providing accurate predictions is challenging for machine learning algorithms when the number of features is larger than the number of samples in the data. Prior knowledge can improve machine learning models by indicating relevant variables and parameter values. Yet, this prior knowledge is often tacit and only available from domain experts. We present a novel approach that uses interactive visualization to elicit the tacit prior knowledge and uses it to improve the accuracy of prediction models. The main component of our approach is a user model that models the domain expert's knowledge of the relevance of different features for a prediction task. In particular, based on the expert's earlier input, the user model guides the selection of the features on which to elicit user's knowledge next. The results of a controlled user study show that the user model significantly improves prior knowledge elicitation and prediction accuracy, when predicting the relative citation counts of scientific documents in a specific domain.
from cs.AI updates on arXiv.org http://ift.tt/2hbffXD
via IFTTT
Stochastic Primal-Dual Methods and Sample Complexity of Reinforcement Learning. (arXiv:1612.02516v1 [stat.ML])
We study the online estimation of the optimal policy of a Markov decision process (MDP). We propose a class of Stochastic Primal-Dual (SPD) methods which exploit the inherent minimax duality of Bellman equations. The SPD methods update a few coordinates of the value and policy estimates as a new state transition is observed. These methods use small storage and has low computational complexity per iteration. The SPD methods find an absolute-$\epsilon$-optimal policy, with high probability, using $\mathcal{O}\left(\frac{|\mathcal{S}|^4 |\mathcal{A}|^2\sigma^2 }{(1-\gamma)^6\epsilon^2} \right)$ iterations/samples for the infinite-horizon discounted-reward MDP and $\mathcal{O}\left(\frac{|\mathcal{S}|^4 |\mathcal{A}|^2H^6\sigma^2 }{\epsilon^2} \right)$ for the finite-horizon MDP.
from cs.AI updates on arXiv.org http://ift.tt/2hbc7L7
via IFTTT
Prediction with a Short Memory. (arXiv:1612.02526v1 [cs.LG])
We consider the problem of predicting the next observation given a sequence of past observations. We show that for any distribution over observations, if the mutual information between past observations and future observations is upper bounded by $I$, then a simple Markov model over the most recent $I/\epsilon$ observations can obtain KL error $\epsilon$ with respect to the optimal predictor with access to the entire past. For a Hidden Markov Model with $n$ states, $I$ is bounded by $\log n$, a quantity that does not depend on the mixing time. We also demonstrate that the simple Markov model cannot really be improved upon: First, a window length of $I/\epsilon$ ($I/\epsilon^2$) is information-theoretically necessary for KL error ($\ell_1$ error). Second, the $d^{\Theta(I/\epsilon)}$ samples required to accurately estimate the Markov model when observations are drawn from an alphabet of size $d$ is in fact necessary for any computationally tractable algorithm, assuming the hardness of strongly refuting a certain class of CSPs.
from cs.AI updates on arXiv.org http://ift.tt/2hbc5CZ
via IFTTT
Inverses, Conditionals and Compositional Operators in Separative Valuation Algebra. (arXiv:1612.02587v1 [cs.AI])
Compositional models were introduce by Jirousek and Shenoy in the general framework of valuation-based systems. They based their theory on an axiomatic system of valuations involving not only the operations of combination and marginalisation, but also of removal. They claimed that this systems covers besides the classical case of discrete probability distributions, also the cases of Gaussian densities and belief functions, and many other systems.
Whereas their results on the compositional operator are correct, the axiomatic basis is not sufficient to cover the examples claimed above. We propose here a different axiomatic system of valuation algebras, which permits a rigorous mathematical theory of compositional operators in valuation-based systems and covers all the examples mentioned above. It extends the classical theory of inverses in semigroup theory and places thereby the present theory into its proper mathematical frame. Also this theory sheds light on the different structures of valuation-based systems, like regular algebras (represented by probability potentials), canncellative algebras (Gaussian potentials) and general separative algebras (density functions).
from cs.AI updates on arXiv.org http://ift.tt/2h9bfnp
via IFTTT
Decision Theory in an Algebraic Setting. (arXiv:1612.02660v1 [cs.AI])
In decision theory an act is a function from a set of conditions to the set of real numbers. The set of conditions is a partition in some algebra of events. The expected value of an act can be calculated when a probability measure is given. We adopt an algebraic point of view by substituting the algebra of events with a finite distributive lattice and the probability measure with a lattice valuation. We introduce a partial order on acts that generalizes the dominance relation and show that the set of acts is a lattice with respect to this order. Finally we analyze some different kinds of comparison between acts, without supposing a common set of conditions for the acts to be compared.
from cs.AI updates on arXiv.org http://ift.tt/2gqczUY
via IFTTT
Fixpoint Approximation of Strategic Abilities under Imperfect Information. (arXiv:1612.02684v1 [cs.MA])
Model checking of strategic ability under imperfect information is known to be hard. In this paper, we propose translations of ATLir formulae that provide lower and upper bounds for their truth values, and are cheaper to verify than the original specifications. Most interestingly, the lower approximation is provided by a fixpoint expression that uses a nonstandard variant of the next-step ability operator. We show the correctness of the translations, establish their computational complexity, and validate the approach by experiments with a scalable scenario of Bridge play.
from cs.AI updates on arXiv.org http://ift.tt/2h9f4Jm
via IFTTT
Learning in the Machine: Random Backpropagation and the Learning Channel. (arXiv:1612.02734v1 [cs.LG])
Random backpropagation (RBP) is a variant of the backpropagation algorithm for training neural networks, where the transpose of the forward matrices are replaced by fixed random matrices in the calculation of the weight updates. It is remarkable both because of its effectiveness, in spite of using random matrices to communicate error information, and because it completely removes the taxing requirement of maintaining symmetric weights in a physical neural system. To better understand random backpropagation, we first connect it to the notions of local learning and the learning channel. Through this connection, we derive several alternatives to RBP, including skipped RBP (SRPB), adaptive RBP (ARBP), sparse RBP, and their combinations (e.g. ASRBP) and analyze their computational complexity. We then study their behavior through simulations using the MNIST and CIFAR-10 bechnmark datasets. These simulations show that most of these variants work robustly, almost as well as backpropagation, and that multiplication by the derivatives of the activation functions is important. As a follow-up, we study also the low-end of the number of bits required to communicate error information over the learning channel. We then provide partial intuitive explanations for some of the remarkable properties of RBP and its variations. Finally, we prove several mathematical results, including the convergence to fixed points of linear chains of arbitrary length, the convergence to fixed points of linear autoencoders with decorrelated data, the long-term existence of solutions for linear systems with a single hidden layer, and the convergence to fixed points of non-linear chains, when the derivative of the activation functions is included.
from cs.AI updates on arXiv.org http://ift.tt/2ggA8w9
via IFTTT
Controlling Robot Morphology from Incomplete Measurements. (arXiv:1612.02739v1 [cs.RO])
Mobile robots with complex morphology are essential for traversing rough terrains in Urban Search & Rescue missions (USAR). Since teleoperation of the complex morphology causes high cognitive load of the operator, the morphology is controlled autonomously. The autonomous control measures the robot state and surrounding terrain which is usually only partially observable, and thus the data are often incomplete. We marginalize the control over the missing measurements and evaluate an explicit safety condition. If the safety condition is violated, tactile terrain exploration by the body-mounted robotic arm gathers the missing data.
from cs.AI updates on arXiv.org http://ift.tt/2hbebCZ
via IFTTT
Coupling Distributed and Symbolic Execution for Natural Language Queries. (arXiv:1612.02741v1 [cs.LG])
Building neural networks to query a knowledge base (a table) with natural language is an emerging research topic in NLP. The neural enquirer typically necessitates multiple steps of execution because of the compositionality of queries. In previous studies, researchers have developed either distributed enquirers or symbolic ones for table querying. The distributed enquirer is end-to-end learnable, but is weak in terms of execution efficiency and explicit interpretability. The symbolic enqurier, on the contrary, is efficient during execution; but it is very difficult to train especially at initial stages. In this paper, we propose to couple distributed and symbolic execution for natural language queries. The observation is that a fully distributed executor also exhibits meaningful, albeit imperfect, interpretation. We can thus pretrain the symbolic executor with the distributed one's intermediate execution results in a step-by-step fashion. Experiments show that our approach significantly outperforms either the distributed or symbolic executor; moreover, we have recovered more than 80% execution sequences with only groundtruth denotations during training. In summary, the coupled neural enquirer takes advantages of both distributed and symbolic executors, and has high performance, high learning efficiency, high execution efficiency, and high interpretability.
from cs.AI updates on arXiv.org http://ift.tt/2ggxMx4
via IFTTT
Hierarchy through Composition with Linearly Solvable Markov Decision Processes. (arXiv:1612.02757v1 [cs.AI])
Hierarchical architectures are critical to the scalability of reinforcement learning methods. Current hierarchical frameworks execute actions serially, with macro-actions comprising sequences of primitive actions. We propose a novel alternative to these control hierarchies based on concurrent execution of many actions in parallel. Our scheme uses the concurrent compositionality provided by the linearly solvable Markov decision process (LMDP) framework, which naturally enables a learning agent to draw on several macro-actions simultaneously to solve new tasks. We introduce the Multitask LMDP module, which maintains a parallel distributed representation of tasks and may be stacked to form deep hierarchies abstracted in space and time.
from cs.AI updates on arXiv.org http://ift.tt/2gqhrt7
via IFTTT
Safety Verification and Control for Collision Avoidance at Road Intersections. (arXiv:1612.02795v1 [math.OC])
This paper presents the design of a supervisory algorithm that monitors safety at road intersections and overrides drivers with a safe input when necessary. The design of the supervisor consists of two parts: safety verification and control design. Safety verification is the problem to determine if vehicles will be able to cross the intersection without colliding with current drivers' inputs. We translate this safety verification problem into a jobshop scheduling problem, which minimizes the maximum lateness and evaluates if the optimal cost is zero. The zero optimal cost corresponds to the case in which all vehicles can cross each conflict area without collisions. Computing the optimal cost requires solving a Mixed Integer Nonlinear Programming (MINLP) problem due to the nonlinear second-order dynamics of the vehicles. We therefore estimate this optimal cost by formulating two related Mixed Integer Linear Programming (MILP) problems that assume simpler vehicle dynamics. We prove that these two MILP problems yield lower and upper bounds of the optimal cost. We also quantify the worst case approximation errors of these MILP problems. We design the supervisor to override the vehicles with a safe control input if the MILP problem that computes the upper bound yields a positive optimal cost. We theoretically demonstrate that the supervisor keeps the intersection safe and is non-blocking. Computer simulations further validate that the algorithms can run in real time for problems of realistic size.
from cs.AI updates on arXiv.org http://ift.tt/2h9c6ER
via IFTTT
Task-Guided and Path-Augmented Heterogeneous Network Embedding for Author Identification. (arXiv:1612.02814v1 [cs.LG])
In this paper, we study the problem of author identification under double-blind review setting, which is to identify potential authors given information of an anonymized paper. Different from existing approaches that rely heavily on feature engineering, we propose to use network embedding approach to address the problem, which can automatically represent nodes into lower dimensional feature vectors. However, there are two major limitations in recent studies on network embedding: (1) they are usually general-purpose embedding methods, which are independent of the specific tasks; and (2) most of these approaches can only deal with homogeneous networks, where the heterogeneity of the network is ignored. Hence, challenges faced here are two folds: (1) how to embed the network under the guidance of the author identification task, and (2) how to select the best type of information due to the heterogeneity of the network.
To address the challenges, we propose a task-guided and path-augmented heterogeneous network embedding model. In our model, nodes are first embedded as vectors in latent feature space. Embeddings are then shared and jointly trained according to task-specific and network-general objectives. We extend the existing unsupervised network embedding to incorporate meta paths in heterogeneous networks, and select paths according to the specific task. The guidance from author identification task for network embedding is provided both explicitly in joint training and implicitly during meta path selection. Our experiments demonstrate that by using path-augmented network embedding with task guidance, our model can obtain significantly better accuracy at identifying the true authors comparing to existing methods.
from cs.AI updates on arXiv.org http://ift.tt/2ggBYNg
via IFTTT
Max-Product Belief Propagation for Linear Programming: Applications to Combinatorial Optimization. (arXiv:1412.4972v4 [cs.AI] UPDATED)
The max-product Belief Propagation (BP) is a popular message-passing heuristic for approximating a maximum-a-posteriori (MAP) assignment in a joint distribution represented by a graphical model (GM). In the past years, it has been shown that BP can solve a few classes of Linear Programming (LP) formulations to combinatorial optimization problems including maximum weight matching, shortest path and network flow, i.e., BP can be used as a message-passing solver for certain combinatorial optimizations. However, those LPs and corresponding BP analysis are very sensitive to underlying problem setups, and it has been not clear what extent these results can be generalized to. In this paper, we obtain a generic criteria that BP converges to the optimal solution of given LP, and show that it is satisfied in LP formulations associated to many classical combinatorial optimization problems including maximum weight perfect matching, shortest path, traveling salesman, cycle packing, vertex/edge cover and network flow.
from cs.AI updates on arXiv.org http://ift.tt/1weVCOY
via IFTTT
An unexpected unity among methods for interpreting model predictions. (arXiv:1611.07478v3 [cs.AI] UPDATED)
Understanding why a model made a certain prediction is crucial in many data science fields. Interpretable predictions engender appropriate trust and provide insight into how the model may be improved. However, with large modern datasets the best accuracy is often achieved by complex models even experts struggle to interpret, which creates a tension between accuracy and interpretability. Recently, several methods have been proposed for interpreting predictions from complex models by estimating the importance of input features. Here, we present how a model-agnostic additive representation of the importance of input features unifies current methods. This representation is optimal, in the sense that it is the only set of additive values that satisfies important properties. We show how we can leverage these properties to create novel visual explanations of model predictions. The thread of unity that this representation weaves through the literature indicates that there are common principles to be learned about the interpretation of model predictions that apply in many scenarios.
from cs.AI updates on arXiv.org http://ift.tt/2fneUw3
via IFTTT
I have a new follower on Twitter
Tanium
15-Second Visibility and Control Over Every Endpoint. Even Across the Largest Networks. #SeeTheTruth https://t.co/a62R2EABKi
Emeryville, CA
http://t.co/yo56HE4WJO
Following: 424 - Followers: 3084
December 08, 2016 at 07:48PM via Twitter http://twitter.com/Tanium
Anonymous social app Yik Yak slashes workforce
from Google Alert - anonymous http://ift.tt/2ghzBi1
via IFTTT
Maria da academy asian 2 download vidio
from Google Alert - anonymous http://ift.tt/2h8L6VT
via IFTTT
[FD] AST-2016-009:
Source: Gmail -> IFTTT-> Blogger
[FD] AST-2016-008: Crash on SDP offer or answer from endpoint using Opus
Source: Gmail -> IFTTT-> Blogger
I have a new follower on Twitter
Inbound Online
Inbound Online Marketing creates successful digital marketing channels. We share what we think are the best strategies.
https://t.co/oBtcSe2hI8
Following: 908 - Followers: 19995
December 08, 2016 at 05:33PM via Twitter http://twitter.com/inboundonline
Maria da academy asian 2 download vidio
from Google Alert - anonymous http://ift.tt/2h8BEBX
via IFTTT
Rumor Central: Orioles have discussed trade for OF Jarrod Dyson with Royals - FanRag Sports; 30 SBs in 2016 (ESPN)
via IFTTT
Yahoo Flaw Allowed Hackers to Read Anyone's Emails
from The Hacker News http://ift.tt/2h14WF8
via IFTTT
quit smoking Costa Mesa Nicotine Anonymous
from Google Alert - anonymous http://ift.tt/2h0j2q4
via IFTTT
Report to the Tip-Off Anonymous Hotline
from Google Alert - anonymous http://ift.tt/2hoO6wD
via IFTTT
Ravens: John Harbaugh feels he has "good relationship" with Bill Belichick despite Deflategate tension - Jamison Hensley (ESPN)
via IFTTT
Russia proposes 10 Year in Prison Sentence for Hackers and Malware Authors
from The Hacker News http://ift.tt/2hotYeb
via IFTTT
ISS Daily Summary Report – 12/07/2016
from ISS On-Orbit Status Report http://ift.tt/2gZWAgX
via IFTTT
Turkish Hackers Are Giving Away Prizes For Participating In DDoS Attacks
from The Hacker News http://ift.tt/2gZmaCv
via IFTTT
Alcoholics Anonymous Sobriety Chips
from Google Alert - anonymous http://ift.tt/2hnIRxx
via IFTTT
Shoe Addicts Anonymous
from Google Alert - anonymous http://ift.tt/2gZbaVR
via IFTTT
Worldwide Events
from Google Alert - anonymous http://ift.tt/2hjmSvB
via IFTTT
NGC 4696: Filaments around a Black Hole
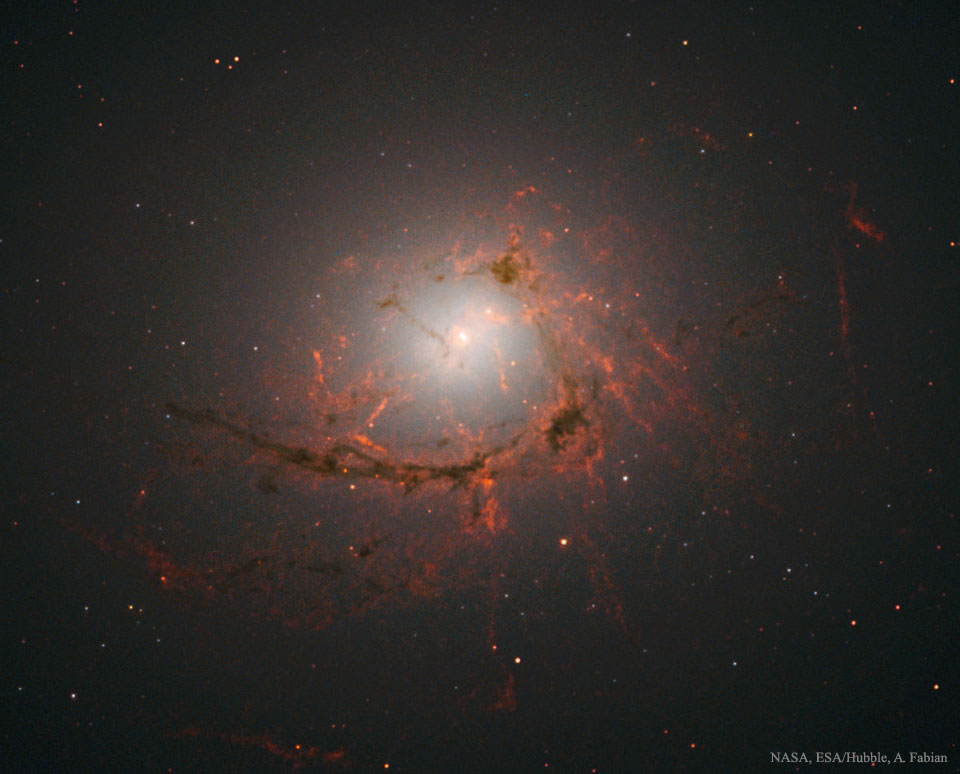
Wednesday, December 7, 2016
Watch Anonymous (2016)
from Google Alert - anonymous http://ift.tt/2gd2z2B
via IFTTT
Rumor Central: Return to Baltimore still a possibility for free-agent C Matt Wieters - MASNsports.com (ESPN)
via IFTTT
Effect of Reward Function Choices in Risk-Averse Reinforcement Learning. (arXiv:1612.02088v1 [cs.AI])
This paper studies Value-at-Risk problems in finite-horizon Markov decision processes (MDPs) with finite state space and two forms of reward function. Firstly we study the effect of reward function on two criteria in a short-horizon MDP. Secondly, for long-horizon MDPs, we estimate the total reward distribution in a finite-horizon Markov chain (MC) with the help of spectral theory and the central limit theorem, and present a transformation algorithm for the MCs with a three-argument reward function and a salvage reward.
from cs.AI updates on arXiv.org http://ift.tt/2gcAlFo
via IFTTT
A Multi-Pass Approach to Large-Scale Connectomics. (arXiv:1612.02120v1 [q-bio.QM])
The field of connectomics faces unprecedented "big data" challenges. To reconstruct neuronal connectivity, automated pixel-level segmentation is required for petabytes of streaming electron microscopy data. Existing algorithms provide relatively good accuracy but are unacceptably slow, and would require years to extract connectivity graphs from even a single cubic millimeter of neural tissue. Here we present a viable real-time solution, a multi-pass pipeline optimized for shared-memory multicore systems, capable of processing data at near the terabyte-per-hour pace of multi-beam electron microscopes. The pipeline makes an initial fast-pass over the data, and then makes a second slow-pass to iteratively correct errors in the output of the fast-pass. We demonstrate the accuracy of a sparse slow-pass reconstruction algorithm and suggest new methods for detecting morphological errors. Our fast-pass approach provided many algorithmic challenges, including the design and implementation of novel shallow convolutional neural nets and the parallelization of watershed and object-merging techniques. We use it to reconstruct, from image stack to skeletons, the full dataset of Kasthuri et al. (463 GB capturing 120,000 cubic microns) in a matter of hours on a single multicore machine rather than the weeks it has taken in the past on much larger distributed systems.
from cs.AI updates on arXiv.org http://ift.tt/2h7O1Pf
via IFTTT
Measuring the non-asymptotic convergence of sequential Monte Carlo samplers using probabilistic programming. (arXiv:1612.02161v1 [cs.AI])
A key limitation of sampling algorithms for approximate inference is that it is difficult to quantify their approximation error. Widely used sampling schemes, such as sequential importance sampling with resampling and Metropolis-Hastings, produce output samples drawn from a distribution that may be far from the target posterior distribution. This paper shows how to upper-bound the symmetric KL divergence between the output distribution of a broad class of sequential Monte Carlo (SMC) samplers and their target posterior distributions, subject to assumptions about the accuracy of a separate gold-standard sampler. The proposed method applies to samplers that combine multiple particles, multinomial resampling, and rejuvenation kernels. The experiments show the technique being used to estimate bounds on the divergence of SMC samplers for posterior inference in a Bayesian linear regression model and a Dirichlet process mixture model.
from cs.AI updates on arXiv.org http://ift.tt/2gDYuAP
via IFTTT
Knowledge Representation in Graphs using Convolutional Neural Networks. (arXiv:1612.02255v1 [cs.AI])
Knowledge Graphs (KG) constitute a flexible representation of complex relationships between entities particularly useful for biomedical data. These KG, however, are very sparse with many missing edges (facts) and the visualisation of the mesh of interactions nontrivial. Here we apply a compositional model to embed nodes and relationships into a vectorised semantic space to perform graph completion. A visualisation tool based on Convolutional Neural Networks and Self-Organised Maps (SOM) is proposed to extract high-level insights from the KG. We apply this technique to a subset of CTD, containing interactions of compounds with human genes / proteins and show that the performance is comparable to the one obtained by structural models.
from cs.AI updates on arXiv.org http://ift.tt/2gmb4Xw
via IFTTT
Extend natural neighbor: a novel classification method with self-adaptive neighborhood parameters in different stages. (arXiv:1612.02310v1 [cs.AI])
Various kinds of k-nearest neighbor (KNN) based classification methods are the bases of many well-established and high-performance pattern-recognition techniques, but both of them are vulnerable to their parameter choice. Essentially, the challenge is to detect the neighborhood of various data sets, while utterly ignorant of the data characteristic. This article introduces a new supervised classification method: the extend natural neighbor (ENaN) method, and shows that it provides a better classification result without choosing the neighborhood parameter artificially. Unlike the original KNN based method which needs a prior k, the ENaNE method predicts different k in different stages. Therefore, the ENaNE method is able to learn more from flexible neighbor information both in training stage and testing stage, and provide a better classification result.
from cs.AI updates on arXiv.org http://ift.tt/2gcB5KL
via IFTTT
Fair task allocation in transportation. (arXiv:1505.07434v3 [cs.AI] UPDATED)
Task allocation problems have traditionally focused on cost optimization. However, more and more attention is being given to cases in which cost should not always be the sole or major consideration. In this paper we study a fair task allocation problem in transportation where an optimal allocation not only has low cost but more importantly, it distributes tasks as even as possible among heterogeneous participants who have different capacities and costs to execute tasks. To tackle this fair minimum cost allocation problem we analyze and solve it in two parts using two novel polynomial-time algorithms. We show that despite the new fairness criterion, the proposed algorithms can solve the fair minimum cost allocation problem optimally in polynomial time. In addition, we conduct an extensive set of experiments to investigate the trade-off between cost minimization and fairness. Our experimental results demonstrate the benefit of factoring fairness into task allocation. Among the majority of test instances, fairness comes with a very small price in terms of cost.
from cs.AI updates on arXiv.org http://ift.tt/1exr6u1
via IFTTT
Chinese Poetry Generation with Planning based Neural Network. (arXiv:1610.09889v2 [cs.CL] UPDATED)
Chinese poetry generation is a very challenging task in natural language processing. In this paper, we propose a novel two-stage poetry generating method which first plans the sub-topics of the poem according to the user's writing intent, and then generates each line of the poem sequentially, using a modified recurrent neural network encoder-decoder framework. The proposed planning-based method can ensure that the generated poem is coherent and semantically consistent with the user's intent. A comprehensive evaluation with human judgments demonstrates that our proposed approach outperforms the state-of-the-art poetry generating methods and the poem quality is somehow comparable to human poets.
from cs.AI updates on arXiv.org http://ift.tt/2eOgs5m
via IFTTT
Tuning Recurrent Neural Networks with Reinforcement Learning. (arXiv:1611.02796v3 [cs.LG] UPDATED)
Sequence models can be trained using supervised learning and a next-step prediction objective. This approach, however, suffers from known failure modes. For example, it is notoriously difficult to ensure multi-step generated sequences have coherent global structure. Motivated by the fact that reinforcement learning (RL) can be used to impose arbitrary properties on generated data by choosing appropriate reward functions, in this paper we propose a novel approach for sequence training which combines Maximum Likelihood (ML) and RL training. We refine a sequence predictor by optimizing for some imposed reward functions, while maintaining good predictive properties learned from data. We propose efficient ways to solve this by augmenting deep Q-learning with a cross-entropy reward and deriving novel off-policy methods for RNNs from stochastic optimal control (SOC). We explore the usefulness of our approach in the context of music generation. An LSTM is trained on a large corpus of songs to predict the next note in a musical sequence. This Note-RNN is then refined using RL, where the reward function is a combination of rewards based on rules of music theory, as well as the output of another trained Note-RNN. We show that by combining ML and RL, this RL Tuner method can not only produce more pleasing melodies, but that it can significantly reduce unwanted behaviors and failure modes of the RNN.
from cs.AI updates on arXiv.org http://ift.tt/2gcycJD
via IFTTT
Composing Music with Grammar Argumented Neural Networks and Note-Level Encoding. (arXiv:1611.05416v2 [cs.LG] UPDATED)
Creating aesthetically pleasing pieces of art, including music, has been a long-term goal for artificial intelligence research. Despite recent successes of long-short term memory (LSTM) recurrent neural networks (RNNs) in sequential learning, LSTM neural networks have not, by themselves, been able to generate natural-sounding music conforming to music theory. To transcend this inadequacy, we put forward a novel method for music composition that combines the LSTM with Grammars motivated by music theory. The main tenets of music theory are encoded as grammar argumented (GA) filters on the training data, such that the machine can be trained to generate music inheriting the naturalness of human-composed pieces from the original dataset while adhering to the rules of music theory. Unlike previous approaches, pitches and durations are encoded as one semantic entity, which we refer to as note-level encoding. This allows easy implementation of music theory grammars, as well as closer emulation of the thinking pattern of a musician. Although the GA rules are applied to the training data and never directly to the LSTM music generation, our machine still composes music that possess high incidences of diatonic scale notes, small pitch intervals and chords, in deference to music theory.
from cs.AI updates on arXiv.org http://ift.tt/2fiAsdL
via IFTTT
The BIN_COUNTS Constraint: Filtering and Applications. (arXiv:1611.08942v2 [cs.AI] UPDATED)
We introduce the BIN_COUNTS constraint, which deals with the problem of counting the number of decision variables in a set which are assigned values that lie in given bins. We illustrate a decomposition and a filtering algorithm that achieves generalised arc consistency. We contrast the filtering power of these two approaches and we discuss a number of applications. We show that BIN_COUNTS can be employed to develop a decomposition for the $\chi^2$ test constraint, a new statistical constraint that we introduce in this work. We also show how this new constraint can be employed in the context of the Balanced Academic Curriculum Problem and of the Balanced Nursing Workload Problem. For both these problems we carry out numerical studies involving our reformulations. Finally, we present a further application of the $\chi^2$ test constraint in the context of confidence interval analysis.
from cs.AI updates on arXiv.org http://ift.tt/2gAe9o9
via IFTTT
One Anonymous Syrian Collective's Struggle Against the Spectacle of Indignity
from Google Alert - anonymous http://ift.tt/2gczPCe
via IFTTT
Grant anonymous access to a folder
from Google Alert - anonymous http://ift.tt/2gWzaJ6
via IFTTT
NFL Image: Ravens' Steve Smith Sr. wears Yoda costume while addressing media; "been a few decades since I wore a onesie" (ESPN)
via IFTTT
Feinstein: A Message To Anonymous Dope Bowl Official
from Google Alert - anonymous http://ift.tt/2h3Tq9B
via IFTTT
I have a new follower on Twitter
Perseids Project
Perseids enables engagement of students and scholars at all levels of expertise to create, share and publish their data and research practices.
Medford, MA
https://t.co/vD7K9zJi16
Following: 5002 - Followers: 3662
December 07, 2016 at 01:28PM via Twitter http://twitter.com/PerseidsProject
Orioles not interested in Jose Bautista, because "our fans don't like" him, GM Dan Duquette tells Sportsnet Radio 590 (ESPN)
via IFTTT
Hacker who stole Celebrity Emails, Sex Tapes, Movie Scripts Gets 5 Years in Prison
from The Hacker News http://ift.tt/2hjbqfh
via IFTTT
Anonymous Hamburger Society
from Google Alert - anonymous http://ift.tt/2hj9FyR
via IFTTT
5-Year-Old Linux Kernel Local Privilege Escalation Flaw Discovered
from The Hacker News http://ift.tt/2gaBKvZ
via IFTTT
advagg exchanges domain for server ip to link css for anonymous users [#2833643] | Drupal.org
from Google Alert - anonymous http://ift.tt/2h2Vqi5
via IFTTT
ISS Daily Summary Report – 12/06/2016
from ISS On-Orbit Status Report http://ift.tt/2hifuMQ
via IFTTT
Hacking Millions with Just an Image — Recipe: Pixels, Ads & Exploit Kit
from The Hacker News http://ift.tt/2g9nAX9
via IFTTT
Aurora over Jupiters South Pole from Juno
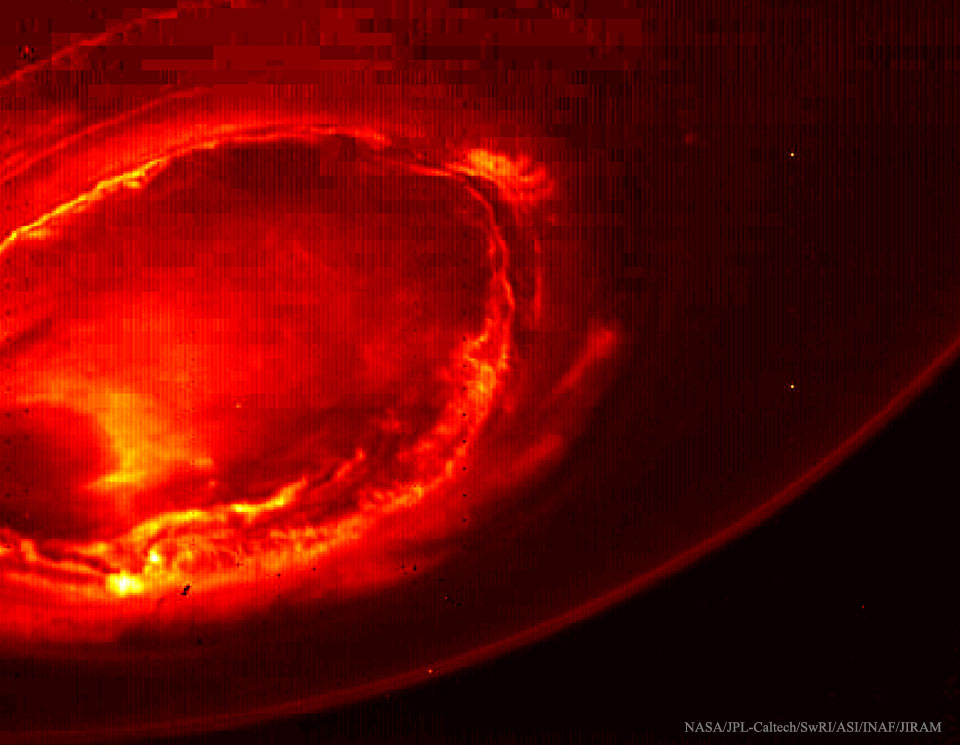
Tuesday, December 6, 2016
Rumor Central: Orioles have called about free-agent 1B Chris Carter - FanRag Sports; .222, NL-best 41 home runs in 2016 (ESPN)
via IFTTT
5870 Watch Actors Anonymous Movie Online Full Version
from Google Alert - anonymous http://ift.tt/2h4tE82
via IFTTT
Improving the Performance of Neural Networks in Regression Tasks Using Drawering. (arXiv:1612.01589v1 [cs.LG])
The method presented extends a given regression neural network to make its performance improve. The modification affects the learning procedure only, hence the extension may be easily omitted during evaluation without any change in prediction. It means that the modified model may be evaluated as quickly as the original one but tends to perform better.
This improvement is possible because the modification gives better expressive power, provides better behaved gradients and works as a regularization. The knowledge gained by the temporarily extended neural network is contained in the parameters shared with the original neural network.
The only cost is an increase in learning time.
from cs.AI updates on arXiv.org http://ift.tt/2g8xI2q
via IFTTT
AI Researchers, Video Games Are Your Friends!. (arXiv:1612.01608v1 [cs.AI])
If you are an artificial intelligence researcher, you should look to video games as ideal testbeds for the work you do. If you are a video game developer, you should look to AI for the technology that makes completely new types of games possible. This chapter lays out the case for both of these propositions. It asks the question "what can video games do for AI", and discusses how in particular general video game playing is the ideal testbed for artificial general intelligence research. It then asks the question "what can AI do for video games", and lays out a vision for what video games might look like if we had significantly more advanced AI at our disposal. The chapter is based on my keynote at IJCCI 2015, and is written in an attempt to be accessible to a broad audience.
from cs.AI updates on arXiv.org http://ift.tt/2gAzpXj
via IFTTT
Fleet Size and Mix Split-Delivery Vehicle Routing. (arXiv:1612.01691v1 [cs.AI])
In the classic Vehicle Routing Problem (VRP) a fleet of of vehicles has to visit a set of customers while minimising the operations' costs. We study a rich variant of the VRP featuring split deliveries, an heterogeneous fleet, and vehicle-commodity incompatibility constraints. Our goal is twofold: define the cheapest routing and the most adequate fleet.
To do so, we split the problem into two interdependent components: a fleet design component and a routing component. First, we define two Mixed Integer Programming (MIP) formulations for each component. Then we discuss several improvements in the form of valid cuts and symmetry breaking constraints.
The main contribution of this paper is a comparison of the four resulting models for this Rich VRP. We highlight their strengths and weaknesses with extensive experiments.
Finally, we explore a lightweight integration with Constraint Programming (CP). We use a fast CP model which gives good solutions and use the solution to warm-start our models.
from cs.AI updates on arXiv.org http://ift.tt/2gQi93B
via IFTTT
Factored Contextual Policy Search with Bayesian Optimization. (arXiv:1612.01746v1 [cs.LG])
Scarce data is a major challenge to scaling robot learning to truly complex tasks, as we need to generalize locally learned policies over different "contexts". Bayesian optimization approaches to contextual policy search (CPS) offer data-efficient policy learning that generalize over a context space. We propose to improve data- efficiency by factoring typically considered contexts into two components: target- type contexts that correspond to a desired outcome of the learned behavior, e.g. target position for throwing a ball; and environment type contexts that correspond to some state of the environment, e.g. initial ball position or wind speed. Our key observation is that experience can be directly generalized over target-type contexts. Based on that we introduce Factored Contextual Policy Search with Bayesian Optimization for both passive and active learning settings. Preliminary results show faster policy generalization on a simulated toy problem.
from cs.AI updates on arXiv.org http://ift.tt/2h0LNR5
via IFTTT
On a Well-behaved Relational Generalisation of Rough Set Approximations. (arXiv:1612.01857v1 [cs.AI])
We examine non-dual relational extensions of rough set approximations and find an extension which satisfies surprisingly many of the usual rough set properties. We then use this definition to give an explanation for an observation made by Samanta and Chakraborty in their recent paper [P. Samanta and M.K. Chakraborty. Interface of rough set systems and modal logics: A survey. \textit{Transactions on Rough Sets XIX}, pages 114-137, 2015].
from cs.AI updates on arXiv.org http://ift.tt/2gAvF8x
via IFTTT
Knowing When to Look: Adaptive Attention via A Visual Sentinel for Image Captioning. (arXiv:1612.01887v1 [cs.CV])
Attention-based neural encoder-decoder frameworks have been widely adopted for image captioning. Most methods force visual attention to be active for every generated word. However, the decoder likely requires little to no visual information from the image to predict non-visual words such as "the" and "of". Other words that may seem visual can often be predicted reliably just from the language model e.g., "sign" after "behind a red stop" or "phone" following "talking on a cell". In this paper, we propose a novel adaptive attention model with a visual sentinel. At each time step, our model decides whether to attend to the image (and if so, to which regions) or to the visual sentinel. The model decides whether to attend to the image and where, in order to extract meaningful information for sequential word generation. We test our method on the COCO image captioning 2015 challenge dataset and Flickr30K. Our approach sets the new state-of-the-art by a significant margin.
from cs.AI updates on arXiv.org http://ift.tt/2gQg0or
via IFTTT
Cross-Lingual Predicate Mapping Between Linked Data Ontologies. (arXiv:1612.01892v1 [cs.AI])
Ontologies in different natural languages often differ in quality in terms of richness of schema or richness of internal links. This difference is markedly visible when comparing a rich English language ontology with a non-English language counterpart. Discovering alignment between them is a useful endeavor as it serves as a starting point in bridging the disparity. In particular, our work is motivated by the absence of inter-language links for predicates in the localised versions of DBpedia. In this paper, we propose and demonstrate an ad-hoc system to find possible owl:equivalentProperty links between predicates in ontologies of different natural languages. We seek to achieve this mapping by using pre-existing inter-language links of the resources connected by the given predicate. Thus, our methodology stresses on semantic similarity rather than lexical. Moreover, through an evaluation, we show that our system is capable of outperforming a baseline system that is similar to the one used in recent OAEI campaigns.
from cs.AI updates on arXiv.org http://ift.tt/2gAE3EY
via IFTTT
Correlation Alignment for Unsupervised Domain Adaptation. (arXiv:1612.01939v1 [cs.CV])
In this chapter, we present CORrelation ALignment (CORAL), a simple yet effective method for unsupervised domain adaptation. CORAL minimizes domain shift by aligning the second-order statistics of source and target distributions, without requiring any target labels. In contrast to subspace manifold methods, it aligns the original feature distributions of the source and target domains, rather than the bases of lower-dimensional subspaces. It is also much simpler than other distribution matching methods. CORAL performs remarkably well in extensive evaluations on standard benchmark datasets. We first describe a solution that applies a linear transformation to source features to align them with target features before classifier training. For linear classifiers, we propose to equivalently apply CORAL to the classifier weights, leading to added efficiency when the number of classifiers is small but the number and dimensionality of target examples are very high. The resulting CORAL Linear Discriminant Analysis (CORAL-LDA) outperforms LDA by a large margin on standard domain adaptation benchmarks. Finally, we extend CORAL to learn a nonlinear transformation that aligns correlations of layer activations in deep neural networks (DNNs). The resulting Deep CORAL approach works seamlessly with DNNs and achieves state-of-the-art performance on standard benchmark datasets. Our code is available at:~\url{http://ift.tt/2g8wjJn}
from cs.AI updates on arXiv.org http://ift.tt/2g8t3gS
via IFTTT
Coactive Critiquing: Elicitation of Preferences and Features. (arXiv:1612.01941v1 [cs.AI])
When faced with complex choices, users refine their own preference criteria as they explore the catalogue of options. In this paper we propose an approach to preference elicitation suited for this scenario. We extend Coactive Learning, which iteratively collects manipulative feedback, to optionally query example critiques. User critiques are integrated into the learning model by dynamically extending the feature space. Our formulation natively supports constructive learning tasks, where the option catalogue is generated on-the-fly. We present an upper bound on the average regret suffered by the learner. Our empirical analysis highlights the promise of our approach.
from cs.AI updates on arXiv.org http://ift.tt/2gQ8ABx
via IFTTT
Review of state-of-the-arts in artificial intelligence with application to AI safety problem. (arXiv:1605.04232v2 [cs.AI] UPDATED)
Here, I review current state-of-the-arts in many areas of AI to estimate when it's reasonable to expect human level AI development. Predictions of prominent AI researchers vary broadly from very pessimistic predictions of Andrew Ng to much more moderate predictions of Geoffrey Hinton and optimistic predictions of Shane Legg, DeepMind cofounder. Given huge rate of progress in recent years and this broad range of predictions of AI experts, AI safety questions are also discussed.
from cs.AI updates on arXiv.org http://ift.tt/1V4ZXRj
via IFTTT
Deviant Learning Algorithm: Learning Sparse Mismatch Representations through Time and Space. (arXiv:1609.01459v3 [cs.AI] UPDATED)
Predictive coding (PDC) has recently attracted attention in the neuroscience and computing community as a candidate unifying paradigm for neuronal studies and artificial neural network implementations particularly targeted at unsupervised learning systems. The Mismatch Negativity (MMN) has also recently been studied in relation to PC and found to be a useful ingredient in neural predictive coding systems. Backed by the behavior of living organisms, such networks are particularly useful in forming spatio-temporal transitions and invariant representations of the input world. However, most neural systems still do not account for large number of synapses even though this has been shown by a few machine learning researchers as an effective and very important component of any neural system if such a system is to behave properly. Our major point here is that PDC systems with the MMN effect in addition to a large number of synapses can greatly improve any neural learning system's performance and ability to make decisions in the machine world. In this paper, we propose a novel bio-mimetic computational intelligence algorithm -- the Deviant Learning Algorithm, inspired by these key ideas and functional properties of recent brain-cognitive discoveries and theories. We also show by numerical experiments guided by theoretical insights, how our invented bio-mimetic algorithm can achieve competitive predictions even with very small problem specific data.
from cs.AI updates on arXiv.org http://ift.tt/2cqVki6
via IFTTT
Contextual RNN-GANs for Abstract Reasoning Diagram Generation. (arXiv:1609.09444v2 [cs.CV] UPDATED)
Understanding, predicting, and generating object motions and transformations is a core problem in artificial intelligence. Modeling sequences of evolving images may provide better representations and models of motion and may ultimately be used for forecasting, simulation, or video generation. Diagrammatic Abstract Reasoning is an avenue in which diagrams evolve in complex patterns and one needs to infer the underlying pattern sequence and generate the next image in the sequence. For this, we develop a novel Contextual Generative Adversarial Network based on Recurrent Neural Networks (Context-RNN-GANs), where both the generator and the discriminator modules are based on contextual history (modeled as RNNs) and the adversarial discriminator guides the generator to produce realistic images for the particular time step in the image sequence. We evaluate the Context-RNN-GAN model (and its variants) on a novel dataset of Diagrammatic Abstract Reasoning, where it performs competitively with 10th-grade human performance but there is still scope for interesting improvements as compared to college-grade human performance. We also evaluate our model on a standard video next-frame prediction task, achieving improved performance over comparable state-of-the-art.
from cs.AI updates on arXiv.org http://ift.tt/2dDIEW0
via IFTTT