Latest YouTube Video
Saturday, July 1, 2017
Live|Tv|; Manny Pacquiao vs Jeff Horn 2017 Live..Free..
from Google Alert - anonymous http://ift.tt/2seioYS
via IFTTT
Fox[live] Manny Pacquiao vs Jeff Horn LIVE Boxing stream 2017
from Google Alert - anonymous http://ift.tt/2tDmjme
via IFTTT
Anonymous donor will partially match donations for Salvation Army soup kitchen
from Google Alert - anonymous http://ift.tt/2t4jtVZ
via IFTTT
Anonymous 3
from Google Alert - anonymous http://ift.tt/2t43uau
via IFTTT
Assistant Manager job with Anonymous | 1406618
from Google Alert - anonymous http://ift.tt/2uvQ0lL
via IFTTT
I have a new follower on Twitter
Mark Krake
Co-founder, perpetual innovator & continuous community worker @metasfresh #opensource #ERP. #freesoftware, Open Source and #UX enthusiast.
Bonn, Deutschland
https://t.co/svNErnohSW
Following: 12332 - Followers: 13400
July 01, 2017 at 09:51AM via Twitter http://twitter.com/markkrake
WordPress Plugin Used by 300,000+ Sites Found Vulnerable to SQL Injection Attack
from The Hacker News http://ift.tt/2taZxiJ
via IFTTT
[LIVE.TV]..NBA Free Agency Special Live Free NBA Game
[Free(Live)]..All Blacks vs Lions 2017 Live Streaming National Rugby
from Google Alert - anonymous http://ift.tt/2tuHlmI
via IFTTT
3D Lava Falls of Mars
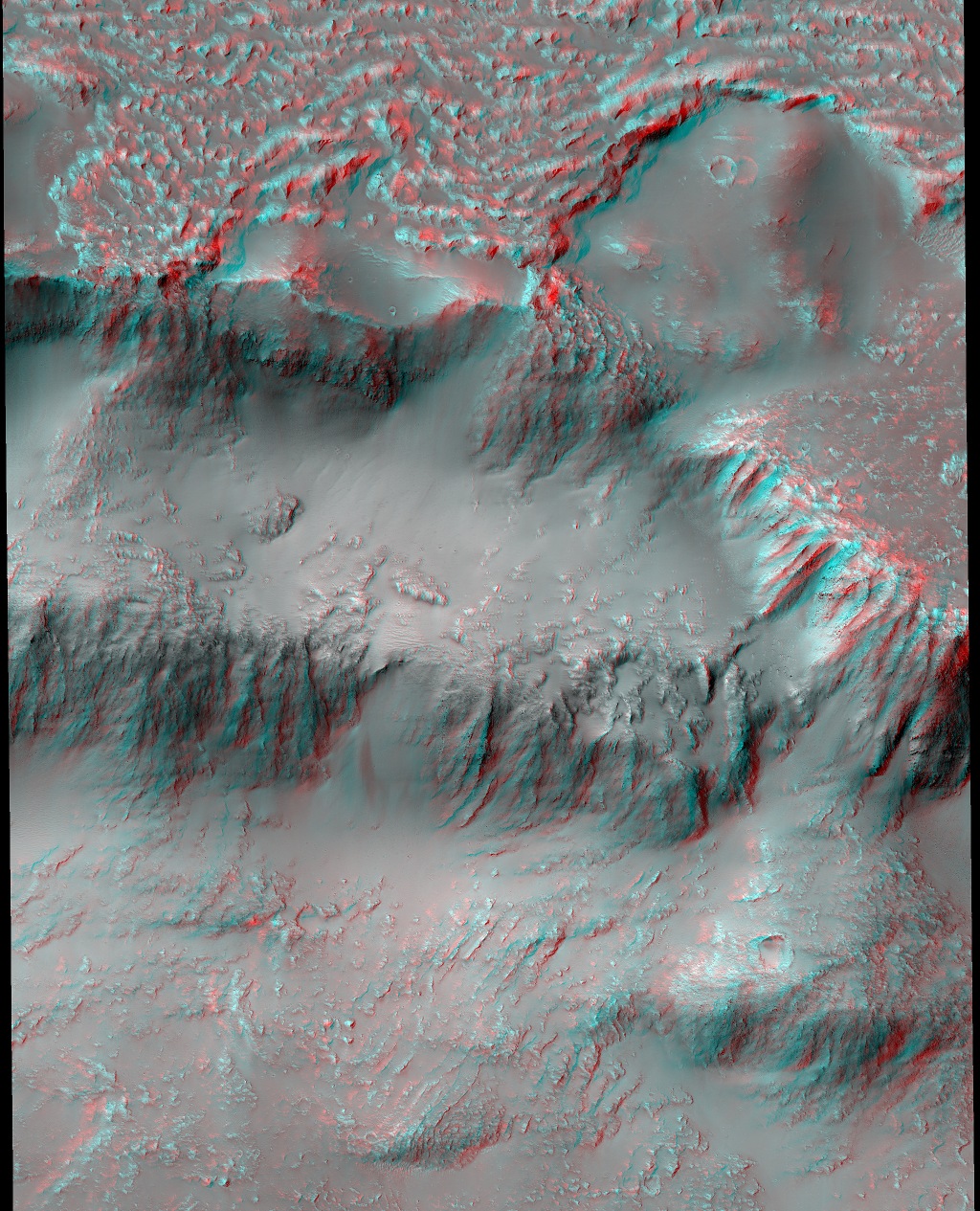
Friday, June 30, 2017
Oscar Negrete vs Sergio Frias Live Stream
from Google Alert - anonymous http://ift.tt/2uttOsD
via IFTTT
Join The Conversation
from Google Alert - anonymous http://ift.tt/2tvrRi0
via IFTTT
[FD] ESA-2017-063: RSA Archer® GRC Platform Multiple Vulnerabilities
Source: Gmail -> IFTTT-> Blogger
[FD] Schneider Electric Pro-Face WinGP – Runtime.exe – Insecure Library Loading Allows Code Execution
Source: Gmail -> IFTTT-> Blogger
[Fox~Live] Shafikov vs Easter Live Stream June, 30, 2017
from Google Alert - anonymous http://ift.tt/2uts1Uk
via IFTTT
Orioles place P Stefan Crichton (right shoulder strain) on 10-day DL, recall P Miguel Castro (ESPN)
via IFTTT
COPIRG Applauds Introduction of Bill to End Anonymous Shell Companies
from Google Alert - anonymous http://ift.tt/2t9VNOB
via IFTTT
[FD] Humax Digital HG100R multiple vulnerabilities
Source: Gmail -> IFTTT-> Blogger
Wikileaks Reveals CIA Malware that Hacks Linux Computers
from The Hacker News http://ift.tt/2usy2AD
via IFTTT
Ravens: TE Darren Waller suspended at least one year for violating NFL's substance abuse policy (ESPN)
via IFTTT
InspiroBot, Potter House Quiz, Travel Ban, Cosby Tour, Anonymous vs. Nasa, Google Fine, 1984 ...
from Google Alert - anonymous http://ift.tt/2sZU4gj
via IFTTT
Obnoxious and Anonymous: Restore Streaming Privileges
from Google Alert - anonymous http://ift.tt/2sZvcp1
via IFTTT
Flix Anonymous - Episode 32
from Google Alert - anonymous http://ift.tt/2tsuC3S
via IFTTT
ISS Daily Summary Report – 6/29/2017
from ISS On-Orbit Status Report http://ift.tt/2u6iOlm
via IFTTT
[FD] SEC Consult SA-20170630-0 :: Multiple critical vulnerabilities in OSCI-Transport library 1.2 for German e-Government
Source: Gmail -> IFTTT-> Blogger
Windows 10 to Get Built-in Protection Against Most Ransomware Attacks
from The Hacker News http://ift.tt/2sojVzP
via IFTTT
NGC 7814: The Little Sombrero in Pegasus
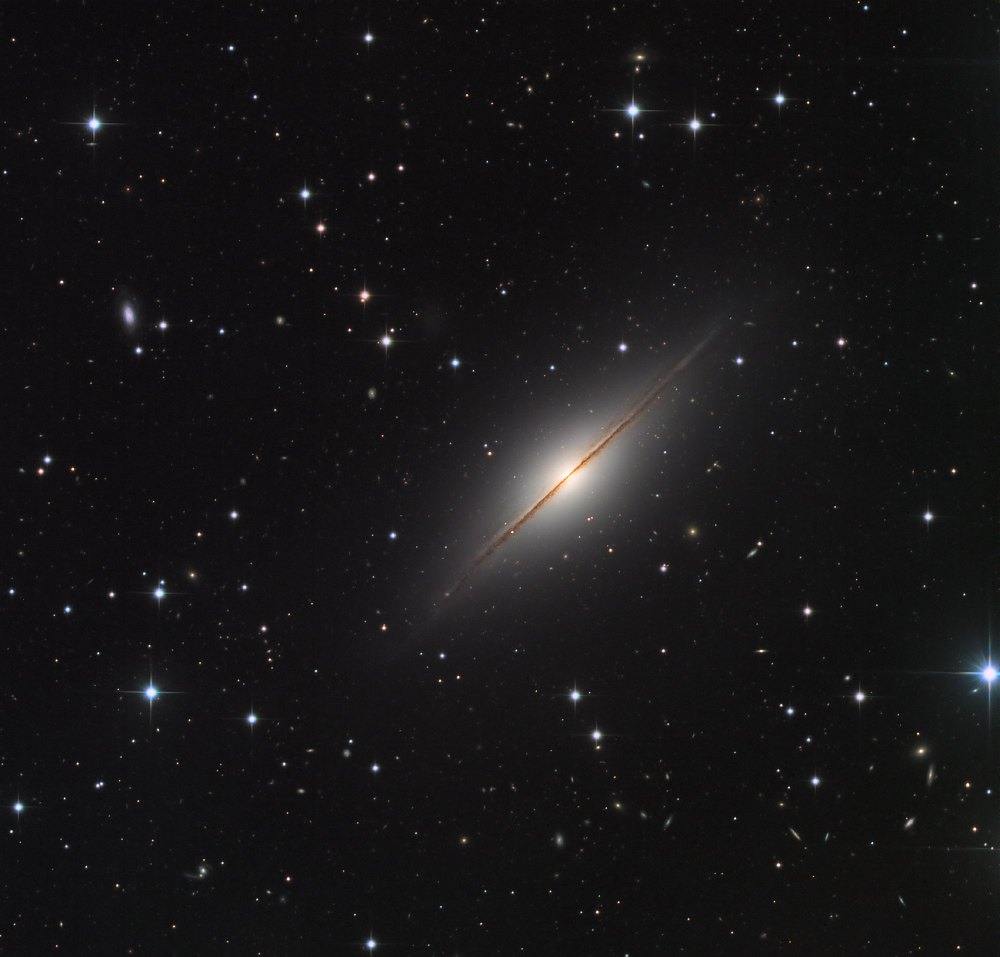
Thursday, June 29, 2017
Orioles Video: Ubaldo Jimenez strikes out eight Blue Jays over eight scoreless innings in 2-0 win (ESPN)
via IFTTT
agrafix/superrecord
from Google Alert - anonymous http://ift.tt/2tvPkk3
via IFTTT
Orioles Video: Caleb Joseph lines RBI single to right-center during 2-0 victory over Blue Jays (ESPN)
via IFTTT
Please help! Webform wont send anonymous messages!
from Google Alert - anonymous http://ift.tt/2tqwvOI
via IFTTT
Ravens conflicted on whether to bring back LB Zach Orr, agent tells 105.7 The Fan (ESPN)
via IFTTT
Access denied for user anonymous with X-CSRF-Token and Cookie
from Google Alert - anonymous http://ift.tt/2tucirF
via IFTTT
Orioles Interview: Trey Mancini reflects on rookie season, favorite ballparks and first MLB at-bat; listen now in ESPN App (ESPN)
via IFTTT
Not just a privacy threat.
from Google Alert - anonymous http://ift.tt/2s5vEiE
via IFTTT
ISS Daily Summary Report – 6/28/2017
from ISS On-Orbit Status Report http://ift.tt/2unskQt
via IFTTT
Original Author of Petya Ransomware is Back & He Wants to Help NotPetya Victims
from The Hacker News http://ift.tt/2sVkUGl
via IFTTT
[InsideNothing] leroyjhunt liked your post "[FD] [ERPSCAN-16-036] SAP ASE ODATA SERVER - DENIAL OF SERVICE"
|
Source: Gmail -> IFTTT-> Blogger
Telegram Agrees to Register With Russia to Avoid Ban, But Won't Share User Data
from The Hacker News http://ift.tt/2tsdZG7
via IFTTT
Anonymous John
from Google Alert - anonymous http://ift.tt/2u1etAb
via IFTTT
Your Linux Machine Can Be Hacked Remotely With Just A Malicious DNS Response
from The Hacker News http://ift.tt/2spvDFu
via IFTTT
Symbiotic R Aquarii
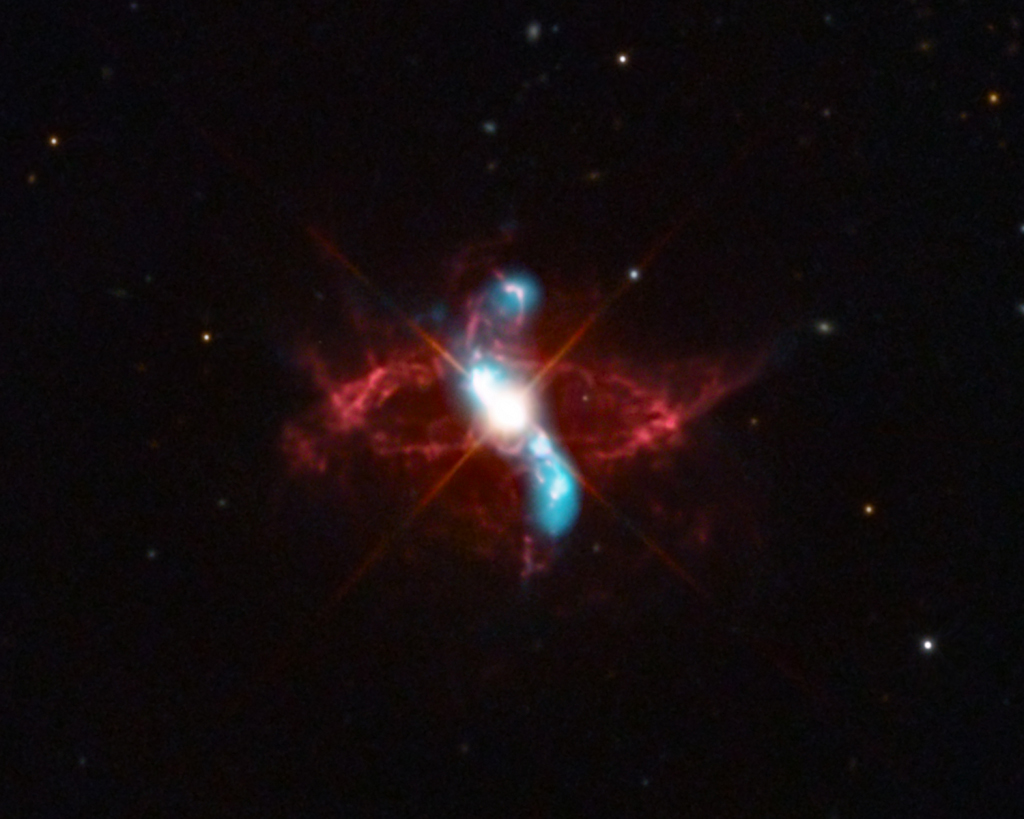
Nats GM slams anonymous player for questioning his lack of bullpen upgrades
from Google Alert - anonymous http://ift.tt/2s3UUFM
via IFTTT
Wednesday, June 28, 2017
ceramics-Collected works of Niklas
from Google Alert - anonymous http://ift.tt/2sohJmP
via IFTTT
Its been fascinating.
from Google Alert - anonymous http://ift.tt/2tqqjqj
via IFTTT
I have a new follower on Twitter
Giving Artfully Kids
Help Build A Generation of Kindness | Empower kids to make a difference through crafting | Join our FB community & inspire kids in your life to give through art
https://t.co/docofhPA0U
Following: 2494 - Followers: 1620
June 28, 2017 at 05:32PM via Twitter http://twitter.com/GivingArtfully
Ravens: Teammates lobby Zach Orr to stay in Baltimore after ending brief retirement (ESPN)
via IFTTT
[FREE] Portugal vs Chile Live Stream
from Google Alert - anonymous http://ift.tt/2ujN4sy
via IFTTT
Turns Out New Petya is Not a Ransomware, It’s a Destructive Wiper Malware
from The Hacker News http://ift.tt/2tlLWaX
via IFTTT
[FD] [CORE-2017-0003] - Kaspersky Anti-Virus File Server Multiple Vulnerabilities
Source: Gmail -> IFTTT-> Blogger
Microsoft Adding Artificial-Intelligence Based Advanced Antivirus to Windows 10
from The Hacker News http://ift.tt/2tptpLh
via IFTTT
Here's How CIA Malware Tracks Geo-Location of its Targets
from The Hacker News http://ift.tt/2slXLZS
via IFTTT
Ravens: Zach Orr considering comeback five months after retiring with a congenital spine and neck condition (ESPN)
via IFTTT
ISS Daily Summary Report – 6/27/2017
from ISS On-Orbit Status Report http://ift.tt/2toiFN5
via IFTTT
'Shadow Brokers' Threatens to Unmask A Hacker Who Worked With NSA
from The Hacker News http://ift.tt/2t0bcRu
via IFTTT
Critical Skype Bug Lets Hackers Remotely Execute Malicious Code
from The Hacker News http://ift.tt/2ugXnxu
via IFTTT
I have a new follower on Twitter
Canada & Chill
Reviews, Rating and Discussions about the best movies on Netflix Canada. Written by Canadian for Canadians! Discover the Movies you should be watching! 🎬
Toronto, Ontario
https://t.co/dUhNaLK5oi
Following: 1188 - Followers: 123
June 28, 2017 at 02:42AM via Twitter http://twitter.com/canadaandchill
Composite Messier 20 and 21
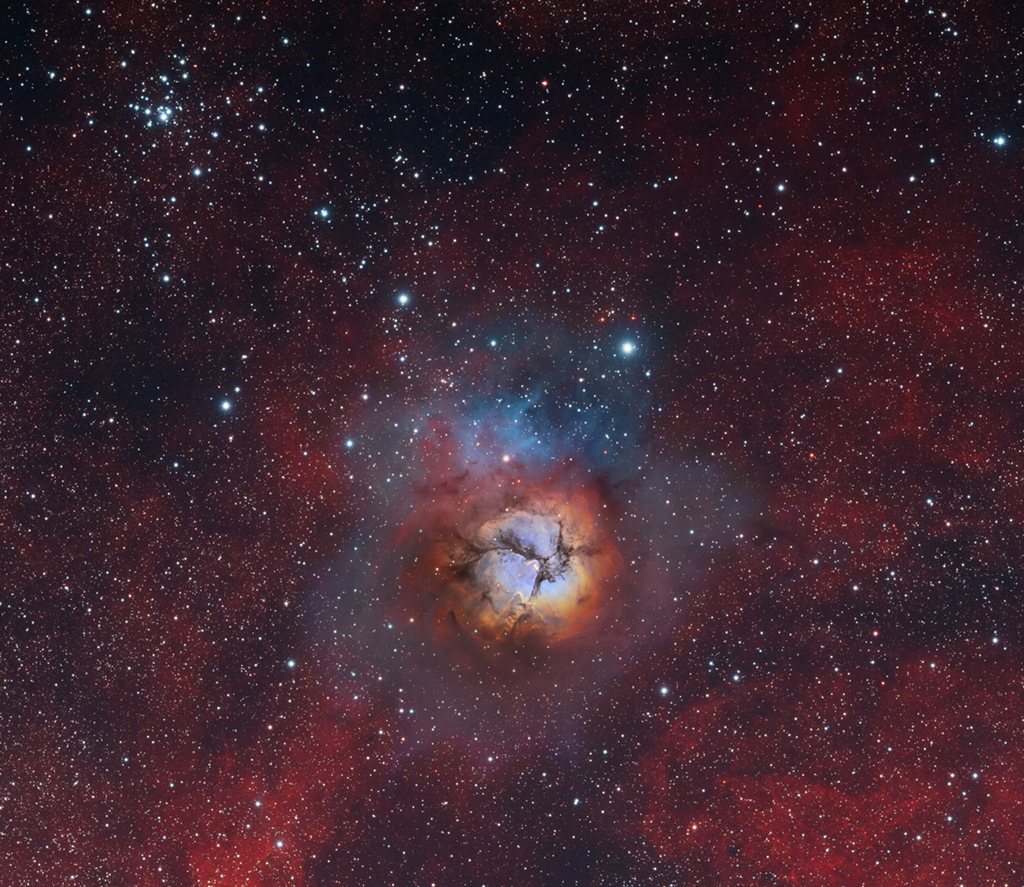
Tuesday, June 27, 2017
Razorcoin – RZR Cryptocurrency Private Send Anonymous Network?
from Google Alert - anonymous http://ift.tt/2tiKkP7
via IFTTT
Anonymous VS. NASA
from Google Alert - anonymous http://ift.tt/2tmm2Em
via IFTTT
SDS - Anonymous NASA Hack
from Google Alert - anonymous http://ift.tt/2sXQ2U2
via IFTTT
Een Kindelien zo Loveliek (Anonymous)
from Google Alert - anonymous http://ift.tt/2tgS3g2
via IFTTT
Q&A: Do Ravens have Super Bowl-caliber defense? Our AFC North reporters aren't buying it (ESPN)
via IFTTT
I have a new follower on Twitter
Socially Rich
"The #1 Instagram Growth Service" The Huffington Post.
https://t.co/npgKZ6LCIu
Following: 5295 - Followers: 7134
June 27, 2017 at 10:49AM via Twitter http://twitter.com/sociallyrichco
Petya Ransomware Spreading Rapidly Worldwide, Just Like WannaCry
from The Hacker News http://ift.tt/2tSaXYP
via IFTTT
ISS Daily Summary Report – 6/26/2017
from ISS On-Orbit Status Report http://ift.tt/2tiStTY
via IFTTT
Google Gets Record-Breaking $2.7 Billion Fine for Manipulating Search Results
from The Hacker News http://ift.tt/2tgcElk
via IFTTT
[FD] Freeware Advanced Audio Decoder 2 (FAAD2) multiple vulnerabilities
Source: Gmail -> IFTTT-> Blogger
Search results for Anonymous
from Google Alert - anonymous http://ift.tt/2sgxmNm
via IFTTT
The M81 Galaxy Group through the Integrated Flux Nebula
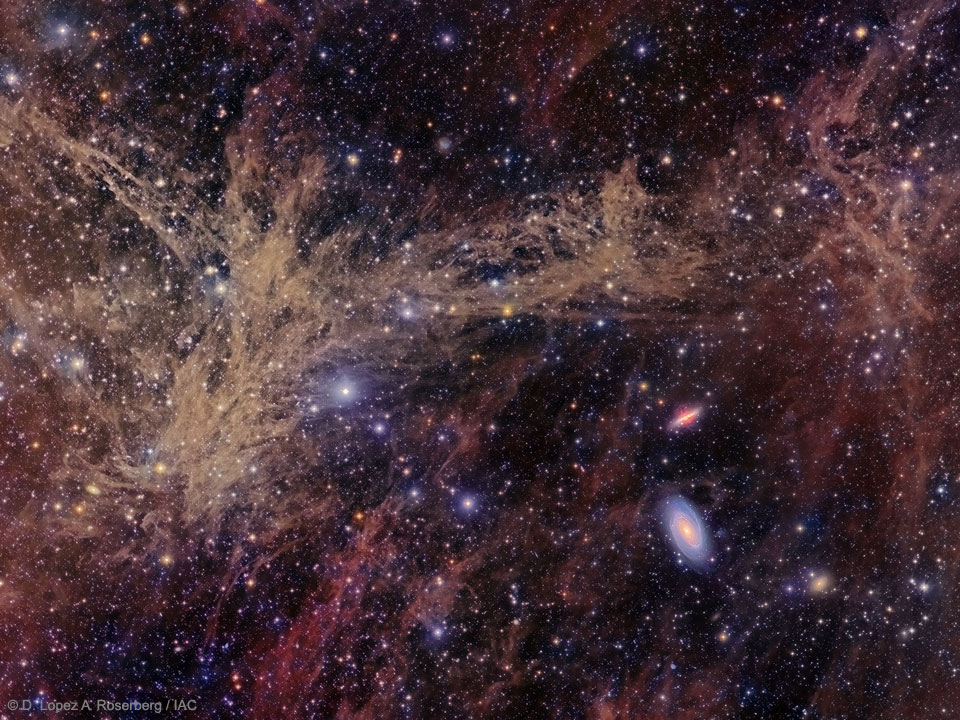
Monday, June 26, 2017
[FD] DefenseCode Security Advisory: IBM DB2 Command Line Processor Buffer Overflow
Source: Gmail -> IFTTT-> Blogger
[FD] Vulnerabilities in D-Link DIR-100
Source: Gmail -> IFTTT-> Blogger
Hacker Group Anonymous Claims NASA Is About To Reveal The Existence Of Alien Life
from Google Alert - anonymous http://ift.tt/2tfXxsx
via IFTTT
NUI Galway Commits to Introducing Anonymous Marking for new Academic Year
from Google Alert - anonymous http://ift.tt/2sfrTGr
via IFTTT
How do I let anonymous users create issues ONLY via the Issue Collector?
from Google Alert - anonymous http://ift.tt/2tdVeFY
via IFTTT
Sponsorship Podcast 5
from Google Alert - anonymous http://ift.tt/2sU0g7S
via IFTTT
Former Ravens S Matt Elam's drug charges dropped in Miami-Dade County (ESPN)
via IFTTT
Anonymous - AGM
from Google Alert - anonymous http://ift.tt/2rUxouF
via IFTTT
Russia Threatens to Ban Telegram Messaging App, Says It Was Used By Terrorists
from The Hacker News http://ift.tt/2tNJ3Nw
via IFTTT
Labeling superpixel colorfulness with OpenCV and Python
After our previous post on computing image colorfulness was published, Stephan, a PyImageSearch reader, left a comment on the tutorial asking if there was a method to compute the colorfulness of specific regions of an image (rather than the entire image).
There are multiple ways of attacking this problem. The first could be to apply a sliding window to loop over the image and compute the colorfulness score for each ROI. An image pyramid could even be applied if the colorfulness of a specific region needed to be computed at multiple scales.
However, a better approach would be to use superpixels. Superpixels are extracted via a segmentation algorithm that groups pixels into (non-rectangular) regions based on their local color/texture. In the case of the popular SLIC superpixel algorithm, image regions are grouped based on a local version of k-means clustering algorithm in the L*a*b* color space.
Given that superpixels will give us a much more natural segmentation of the input image than sliding windows, we can compute the colorfulness of specific regions in an image by:
- Applying superpixel segmentation to the input image.
- Looping over each of the superpixels individually and computing their respective colorfulness scores.
- Maintaining a mask that contains the colorfulness score for each superpixel.
Based on this mask we can then visualize the most colorful regions of the image. Regions of the image that are more colorful will have larger colorful metric scores, while regions that are less colorful will smaller values.
To learn more about superpixels and computing image colorfulness, just keep reading.
Looking for the source code to this post?
Jump right to the downloads section.
Labeling superpixel colorfulness with OpenCV and Python
In the first part of this blog post we will learn how to apply the SLIC algorithm to extract superpixels from our input image. The original 2010 publication by Achanta et al., SLIC Superpixels, goes into the details of the methodology and technique. We also briefly covered SLIC superpixels in this blog post for readers who want a more concise overview of the algorithm.
Given these superpixels, we’ll loop over them individually and compute their colorfulness score, taking care to compute the colorfulness metric for the specific region and not the entire image (as we did in our previous post).
After we implement our script, we’ll apply our combination of superpixel + image colorfulness to a set of input images.
Using superpixels for segmentation
Let’s get started by opening up a new file in your favorite editor or IDE, name it
colorful_regions.py, and insert the following code:
# import the necessary packages from skimage.exposure import rescale_intensity from skimage.segmentation import slic from skimage.util import img_as_float from skimage import io import numpy as np import argparse import cv2
The first Lines 1-8 handle our imports — as you can see we make heavy use of several scikit-image functions in this tutorial.
The
slicfunction will be used to compute superpixels (scikit-image documentation).
Next, we will define our colorfulness metric function with a minor modification from the previous post where it was introduced:
def segment_colorfulness(image, mask): # split the image into its respective RGB components, then mask # each of the individual RGB channels so we can compute # statistics only for the masked region (B, G, R) = cv2.split(image.astype("float")) R = np.ma.masked_array(R, mask=mask) G = np.ma.masked_array(B, mask=mask) B = np.ma.masked_array(B, mask=mask) # compute rg = R - G rg = np.absolute(R - G) # compute yb = 0.5 * (R + G) - B yb = np.absolute(0.5 * (R + G) - B) # compute the mean and standard deviation of both `rg` and `yb`, # then combine them stdRoot = np.sqrt((rg.std() ** 2) + (yb.std() ** 2)) meanRoot = np.sqrt((rg.mean() ** 2) + (yb.mean() ** 2)) # derive the "colorfulness" metric and return it return stdRoot + (0.3 * meanRoot)
Lines 10-31 represent our colorfulness metric function, which has been adapted to compute the colorfulness for a specific region of an image.
The region can be any shape as we take advantage of NumPy masked arrays — only pixels part of the mask will be included in the computation.
For the specified
maskregion of a particular
image, the
segment_colorfulnessfunction performs the following tasks:
- Splits the image into RGB component channels (Line 14).
- Masks the
image
usingmask
(for each channel) so that the colorfulness is only performed on the area specified — in this case the region will be our superpixel (Lines 15-17). - Uses the
R
andG
components to computerg
(Line 20). - Uses the RGB components to compute
yb
(Lines 23). - Computes the mean and standard deviation of
rg
andyb
whilst combining them (Lines 27 and 28). - Does the final calculation of the metric and returns (Line 31) it to the calling function.
Now that our key colorfulness function is defined, the next step is to parse our command line arguments:
# construct the argument parse and parse the arguments ap = argparse.ArgumentParser() ap.add_argument("-i", "--image", required=True, help="path to input image") ap.add_argument("-s", "--segments", type=int, default=100, help="# of superpixels") args = vars(ap.parse_args())
On Lines 34-39 we make use of
argparseto define two arguments:
-
--image
: The path to our input image. -
--segments
: The number of superpixels. The SLIC Superpixels paper shows examples of breaking an image up into different numbers of superpixels. This parameter is fun to experiment with (as it controls the level of granularity of your resulting superpixels); however we’ll be working with adefault=100
. The smaller the value, the fewer and larger the superpixels, allowing the algorithm running faster. The larger the number of segments, the more fine-grained the segmentation, but SLIC will take longer to run (due to more clusters needing to be computed).
Now it’s time to load the image into memory, allocate space for our visualization, and compute SLIC superpixel segmentation:
# load the image in OpenCV format so we can draw on it later, then # allocate memory for the superpixel colorfulness visualization orig = cv2.imread(args["image"]) vis = np.zeros(orig.shape[:2], dtype="float") # load the image and apply SLIC superpixel segmentation to it via # scikit-image image = io.imread(args["image"]) segments = slic(img_as_float(image), n_segments=args["segments"], slic_zero=True)
On Line 43 we load our command line argument
--imageinto memory as
orig(OpenCV format).
We follow this step by allocating memory with the same shape (width and height) as the original input image for our visualization image,
vis.
Next, we load the command line argument
--imageinto memory as
image, this time in scikit-image format. The reason we use scikit-image’s
io.imreadhere is because OpenCV loads images in BGR order rather than RGB format (which scikit-image does). The
slicfunction will convert our input
imageto the L*a*b* color space during the superpixel generation process assuming our image is in RGB format.
Therefore we have two choices:
- Load the image with OpenCV, clone it, and then swap the ordering of the channels.
- Simply load a copy of the original image using scikit-image.
Either approach is valid and will result in the same output.
Superpixels are calculated by a call to
slicwhere we specify
image,
n_segments, and the
slic_zeroswitch. Specifying
slic_zero=Trueindicates that we want to use the zero parameter version of SLIC, an extension to the original algorithm that does not require us to manually tune parameters to the algorithm. We refer to the superpixels as
segmentsfor the rest of the script.
Now let’s compute the colorfulness of each superpixel:
# loop over each of the unique superpixels for v in np.unique(segments): # construct a mask for the segment so we can compute image # statistics for *only* the masked region mask = np.ones(image.shape[:2]) mask[segments == v] = 0 # compute the superpixel colorfulness, then update the # visualization array C = segment_colorfulness(orig, mask) vis[segments == v] = C
We start by looping over each of the individual
segmentson Line 52.
Lines 56 and 57 are responsible for constructing a
maskfor the current superpixel. The
maskwill have the same width and height as our input image and will be filled (initially) with an array of ones (Line 56).
Keep in mind that when using NumPy masked arrays, that a given entry in an array is only included in a computation if the corresponding
maskvalue is set to zero (implying that the pixel is unmasked). If the value in the
maskis one, then the value is assumed to be masked and is hence ignored.
Here we initially set all pixels to masked, then set only the pixels part of the current superpixel to unmasked (Line 57).
Using our
origimage and our
maskas parameters to
segment_colorfulness, we can compute
C, which is the colorfulness of the superpixel (Line 61).
Then, we update our visualization array,
vis, with the value of
C(Line 62).
At this point, we have answered PyImageSearch reader, Stephan’s question — we have computed the colorfulness for different regions of an image.
Naturally we will want to see our results, so let’s continue by constructing a transparent overlay visualization for the most/least colorful regions in our input image:
# scale the visualization image from an unrestricted floating point # to unsigned 8-bit integer array so we can use it with OpenCV and # display it to our screen vis = rescale_intensity(vis, out_range=(0, 255)).astype("uint8") # overlay the superpixel colorfulness visualization on the original # image alpha = 0.6 overlay = np.dstack([vis] * 3) output = orig.copy() cv2.addWeighted(overlay, alpha, output, 1 - alpha, 0, output)
Since
visis currently a floating point array, it is necessary to re-scale it to a typical 8-bit unsigned integer [0-255] array. This is important so that we can display the output image to our screen with OpenCV. We accomplish this by using the
rescale_intensityfunction (from
skimage.exposure) on Line 67.
Now we’ll overlay the superpixel colorfulness visualization on top of the original image. We’ve already discussed transparent overlays and the
cv2.addWeighted(and associated parameters), so please refer to this blog post for more details on how transparent overlays are constructed.
Finally, let’s display images to the screen and close out this script:
# show the output images cv2.imshow("Input", orig) cv2.imshow("Visualization", vis) cv2.imshow("Output", output) cv2.waitKey(0)
We will display three images to the screen using
cv2.imshow, including:
-
orig
: Our input image. -
vis
: Our visualization image (i.e., level of colorfulness for each of the superpixel regions). -
output
: Our output image.
Superpixel and colorfulness metric results
Let’s see our Python script in action — open a terminal,
workonyour virtual environment if you are using one (highly recommended), and enter the following command:
$ python colorful_regions.py --image images/example_01.jpg
On the left you can see the original input image, a photo of myself exploring Antelope Canyon, arguably the most beautiful slot canyon in the United States. Here we can see a mixture of colors due to the light filtering in from above.
In the middle we have our computed visualization for each of the 100 superpixels. Dark regions in this visualization refer to less colorful regions while light regions indicate more colorful.
Here we can see the least colorful regions are around the walls of the canyon, closest to the camera — this is where the least light is researching.
The most colorful regions of the input image are found where the light is directly reaching inside the canyon, illuminating part of the wall like candlelight.
Finally, on the right we have our original input image overlaid with the colorfulness visualization — this image allows us to more easily identify the most/least colorful regions of the image.
The following image is a photo of myself in Boston by the iconic Citgo sign overlooking Kenmore square:
$ python colorful_regions.py --image images/example_02.jpg
Figure 2: Using superpixels, we can first segment our image, and then compute a colorfulness score for each region.
Here we can see the least colorful regions of the image are towards the bottom where the shadow is obscuring much of the sidewalk. The more colorful regions can be found towards the sign and sky itself.
Finally, here is a photo from Rainbow Point, the highest elevation in Bryce Canyon:
$ python colorful_regions.py --image images/example_03.jpg
Notice here that my black hoodie and shorts are the least colorful regions of the image, while the sky and foliage towards the center of the photo are the most colorful.
Summary
In today’s blog post we learned how to use the SLIC segmentation algorithm to compute superpixels for an input image.
We then accessed each of the individual superpixels and applied our colorfulness metric.
The colorfulness scores for each region were combined into a mask, revealing the most colorful and least colorful regions of the input image.
Given this computation, we were able to visualize the colorfulness of each region in two ways:
- By examining the raw
vis
mask. - Creating a transparent overlay that laid
vis
on top of the original image.
In practice, we could use this technique to threshold the mask and extract only the most/least colorful regions.
To be notified when future tutorials are published here on PyImageSearch, be sure to enter your email address in the form below!
Downloads:
The post Labeling superpixel colorfulness with OpenCV and Python appeared first on PyImageSearch.
from PyImageSearch http://ift.tt/2sIiA5e
via IFTTT