Latest YouTube Video
Saturday, May 7, 2016
Anonymous user 782fa6
from Google Alert - anonymous http://ift.tt/2745a0l
via IFTTT
Orioles Video: Chris Davis launches a pitch over center field wall with ease, drives in 2 runs in 5-2 win vs. Athletics (ESPN)
via IFTTT
Ravens: TE Mike Flacco, Joe Flacco's younger brother, trying to fulfill dream by making team at age 29 - Jamison Hensley (ESPN)
via IFTTT
Critical Qualcomm flaw puts millions of Android devices at risk
from The Hacker News http://ift.tt/1WgqsER
via IFTTT
This 10-year-old Boy becomes the youngest Bug Bounty Hacker
from The Hacker News http://ift.tt/1ZpQcMC
via IFTTT
Founder of 'Liberty Reserve' Sentenced to 20 years in Prison
from The Hacker News http://ift.tt/23wJR2S
via IFTTT
NGC 7023: The Iris Nebula

Friday, May 6, 2016
I have a new follower on Twitter
Donna Hardman
Following: 3024 - Followers: 68
May 06, 2016 at 07:32PM via Twitter http://twitter.com/olmarkova89838
Anonymous Gun Buyback!
from Google Alert - anonymous http://ift.tt/1q3LMPm
via IFTTT
New threat for State Attorney from Anonymous Florida
from Google Alert - anonymous http://ift.tt/1ZmhB1M
via IFTTT
When Anonymous had Scientology in a panic: A new document has us nostalgic for 2008
from Google Alert - anonymous http://ift.tt/1WNDPLd
via IFTTT
[FD] Give a warm welcome to Faraday v1.0.19! New GTK interface, Custom Reports & Bug fixing
Source: Gmail -> IFTTT-> Blogger
[FD] CVE Request for ManageEngine Applications Manager Build No: 12700 Information Disclosure and Un-Authenticated SQL injection.
Source: Gmail -> IFTTT-> Blogger
[FD] CVE-2016-2784: CMS Made Simple < 2.1.3 & < 1.12.2 Web server Cache Poisoning
Source: Gmail -> IFTTT-> Blogger
[FD] Swagger Editor v2.9.9 "description" Key DOM-based Cross-Site Scripting
Source: Gmail -> IFTTT-> Blogger
[FD] APPLE-SA-2016-05-03-1 Xcode 7.3.1
Source: Gmail -> IFTTT-> Blogger
ISS Daily Summary Report – 05/05/16
from ISS On-Orbit Status Report http://ift.tt/1WdQPLE
via IFTTT
People Rush to Gold in Scramble for Anonymous Currency
from Google Alert - anonymous http://ift.tt/24xgt2h
via IFTTT
George Mason faculty expresses concerns that maybe, just maybe, the
from Google Alert - anonymous http://ift.tt/21CYt1i
via IFTTT
The SONG and the Hunter
Thursday, May 5, 2016
Orioles Highlight: Kevin Gausman (8 IP, 3 H, 4 K) enjoys best start of season; Pedro Alvarez GW SF in 10th vs. Yankees (ESPN)
via IFTTT
Atlanta colleges look into anonymous Twitter rape claim
from Google Alert - anonymous http://ift.tt/1TqLVnu
via IFTTT
I have a new follower on Twitter
3xScreen Media
Video streaming experts, providing live and on-demand online video services for Agencies, Corporates, Events and Sports.
London
http://t.co/Ik2xfmGdMU
Following: 1112 - Followers: 7380
May 05, 2016 at 10:33PM via Twitter http://twitter.com/3xscreen
Anonymous Twitter Describes Alleged Gang Rape at College Party
from Google Alert - anonymous http://ift.tt/1SQvzIQ
via IFTTT
Movement Coordination in Human-Robot Teams: A Dynamical Systems Approach. (arXiv:1605.01459v1 [cs.RO])
In order to be effective teammates, robots need to be able to understand high-level human behavior to recognize, anticipate, and adapt to human motion. We have designed a new approach to enable robots to perceive human group motion in real-time, anticipate future actions, and synthesize their own motion accordingly. We explore this within the context of joint action, where humans and robots move together synchronously. In this paper, we present an anticipation method which takes high-level group behavior into account. We validate the method within a human-robot interaction scenario, where an autonomous mobile robot observes a team of human dancers, and then successfully and contingently coordinates its movements to "join the dance". We compared the results of our anticipation method to move the robot with another method which did not rely on high-level group behavior, and found our method performed better both in terms of more closely synchronizing the robot's motion to the team, and also exhibiting more contingent and fluent motion. These findings suggest that the robot performs better when it has an understanding of high-level group behavior than when it does not. This work will help enable others in the robotics community to build more fluent and adaptable robots in the future.
from cs.AI updates on arXiv.org http://ift.tt/26YruIE
via IFTTT
ODE - Augmented Training Improves Anomaly Detection in Sensor Data from Machines. (arXiv:1605.01534v1 [cs.AI])
Machines of all kinds from vehicles to industrial equipment are increasingly instrumented with hundreds of sensors. Using such data to detect anomalous behaviour is critical for safety and efficient maintenance. However, anomalies occur rarely and with great variety in such systems, so there is often insufficient anomalous data to build reliable detectors. A standard approach to mitigate this problem is to use one class methods relying only on data from normal behaviour. Unfortunately, even these approaches are more likely to fail in the scenario of a dynamical system with manual control input(s). Normal behaviour in response to novel control input(s) might look very different to the learned detector which may be incorrectly detected as anomalous. In this paper, we address this issue by modelling time-series via Ordinary Differential Equations (ODE) and utilising such an ODE model to simulate the behaviour of dynamical systems under varying control inputs. The available data is then augmented with data generated from the ODE, and the anomaly detector is retrained on this augmented dataset. Experiments demonstrate that ODE-augmented training data allows better coverage of possible control input(s) and results in learning more accurate distinctions between normal and anomalous behaviour in time-series.
from cs.AI updates on arXiv.org http://ift.tt/23tkkHO
via IFTTT
Notes on a model for fuzzy computing. (arXiv:1605.01596v1 [cs.AI])
In these notes we propose a setting for fuzzy computing in a framework similar to that of well-established theories of computation: boolean, and quantum computing. Our efforts have been directed towards stressing the formal similarities: there is a common pattern underlying these three theories. We tried to conform our approach, as much as possible, to this pattern. This work was part of a project jointly with Professor Vittorio Cafagna. Professor Cafagna passed away unexpectedly in 2007. His intellectual breadth and inspiring passion for mathematics is still very well alive.
from cs.AI updates on arXiv.org http://ift.tt/26Yrusq
via IFTTT
Improving abcdSAT by At-Least-One Recently Used Clause Management Strategy. (arXiv:1605.01622v1 [cs.LO])
We improve further the 2015 version of abcdSAT by various heuristics such as at-least-one recently used strategy, learnt clause database approximation reduction etc. Based on the requirement of different tracks at the SAT Competition 2016, we develop three versions of abcdSAT: drup, inc and lim, which participate in the competition of main (agile), incremental library and no-limit track, respectively.
from cs.AI updates on arXiv.org http://ift.tt/23tkmj2
via IFTTT
LSTM-based Mixture-of-Experts for Knowledge-Aware Dialogues. (arXiv:1605.01652v1 [cs.AI])
We introduce an LSTM-based method for dynamically integrating several word-prediction experts to obtain a conditional language model which can be good simultaneously at several subtasks. We illustrate this general approach with an application to dialogue where we integrate a neural chat model, good at conversational aspects, with a neural question-answering model, good at retrieving precise information from a knowledge-base, and show how the integration combines the strengths of the independent components. We hope that this focused contribution will attract attention on the benefits of using such mixtures of experts in NLP.
from cs.AI updates on arXiv.org http://ift.tt/1Tqxobo
via IFTTT
Brain Emotional Learning-Based Prediction Model (For Long-Term Chaotic Prediction Applications). (arXiv:1605.01681v1 [cs.AI])
This study suggests a new prediction model for chaotic time series inspired by the brain emotional learning of mammals. We describe the structure and function of this model, which is referred to as BELPM (Brain Emotional Learning-Based Prediction Model). Structurally, the model mimics the connection between the regions of the limbic system, and functionally it uses weighted k nearest neighbors to imitate the roles of those regions. The learning algorithm of BELPM is defined using steepest descent (SD) and the least square estimator (LSE). Two benchmark chaotic time series, Lorenz and Henon, have been used to evaluate the performance of BELPM. The obtained results have been compared with those of other prediction methods. The results show that BELPM has the capability to achieve a reasonable accuracy for long-term prediction of chaotic time series, using a limited amount of training data and a reasonably low computational time.
from cs.AI updates on arXiv.org http://ift.tt/26YrsAz
via IFTTT
A note on adjusting $R^2$ for using with cross-validation. (arXiv:1605.01703v1 [cs.LG])
We show how to adjust the coefficient of determination ($R^2$) when used for measuring predictive accuracy via leave-one-out cross-validation.
from cs.AI updates on arXiv.org http://ift.tt/1T1yygn
via IFTTT
Learning with Memory Embeddings. (arXiv:1511.07972v8 [cs.AI] UPDATED)
Embedding learning, a.k.a. representation learning, has been shown to be able to model large-scale semantic knowledge graphs. A key concept is a mapping of the knowledge graph to a tensor representation whose entries are predicted by models using latent representations of generalized entities. Latent variable models are well suited to deal with the high dimensionality and sparsity of typical knowledge graphs. In recent publications the embedding models were extended to also consider time evolutions, time patterns and subsymbolic representations. In this paper we map embedding models, which were developed purely as solutions to technical problems for modelling temporal knowledge graphs, to various cognitive memory functions, in particular to semantic and concept memory, episodic memory, sensory memory, short-term memory, and working memory. We discuss learning, query answering, the path from sensory input to semantic decoding, and the relationship between episodic memory and semantic memory. We introduce a number of hypotheses on human memory that can be derived from the developed mathematical models.
from cs.AI updates on arXiv.org http://ift.tt/1NdF4iH
via IFTTT
Multilingual Twitter Sentiment Classification: The Role of Human Annotators. (arXiv:1602.07563v2 [cs.CL] UPDATED)
What are the limits of automated Twitter sentiment classification? We analyze a large set of manually labeled tweets in different languages, use them as training data, and construct automated classification models. It turns out that the quality of classification models depends much more on the quality and size of training data than on the type of the model trained. Experimental results indicate that there is no statistically significant difference between the performance of the top classification models. We quantify the quality of training data by applying various annotator agreement measures, and identify the weakest points of different datasets. We show that the model performance approaches the inter-annotator agreement when the size of the training set is sufficiently large. However, it is crucial to regularly monitor the self- and inter-annotator agreements since this improves the training datasets and consequently the model performance. Finally, we show that there is strong evidence that humans perceive the sentiment classes (negative, neutral, and positive) as ordered.
from cs.AI updates on arXiv.org http://ift.tt/1VFBkrU
via IFTTT
U.S. developing Technology to Identify and Track Hackers Worldwide
from The Hacker News http://ift.tt/1T13FJ5
via IFTTT
Anonymous Twitter account sparks gang rape probe at 2 colleges
from Google Alert - anonymous http://ift.tt/1T11lSn
via IFTTT
Ravens: Secretary of the Navy Ray Mabus \"confident\" that Keenan Reynolds can play football and honor service commitment (ESPN)
via IFTTT
MTurk IDs Are Not Anonymous
from Google Alert - anonymous http://ift.tt/1Yb02S2
via IFTTT
Ravens: Even 8-year-old fans asking about Joe Flacco's left knee - Jamison Hensley; his response - \"it's getting better\" (ESPN)
via IFTTT
SI Releases Annual Anonymous Tour Pro Survey
from Google Alert - anonymous http://ift.tt/23rU2G6
via IFTTT
Want to Use Quantum Computer? IBM launches One for Free
from The Hacker News http://ift.tt/1WKH1Ho
via IFTTT
ISS Daily Summary Report – 05/04/16
from ISS On-Orbit Status Report http://ift.tt/1UBmfd3
via IFTTT
High-Severity OpenSSL Vulnerability allows Hackers to Decrypt HTTPS Traffic
from The Hacker News http://ift.tt/1QSLX68
via IFTTT
Hacker is Selling 272 Million Email Passwords for Just $1
from The Hacker News http://ift.tt/1ZgIp3q
via IFTTT
Anonymous user 723c52
from Google Alert - anonymous http://ift.tt/1T2QnyR
via IFTTT
anonymous feedback for registered users and guests
from Google Alert - anonymous http://ift.tt/1W9k15K
via IFTTT
A Mercury Transit Sequence

Wednesday, May 4, 2016
A Comparative Evaluation of Approximate Probabilistic Simulation and Deep Neural Networks as Accounts of Human Physical Scene Understanding. (arXiv:1605.01138v1 [cs.AI])
Humans demonstrate remarkable abilities to predict physical events in complex scenes. Two classes of models for physical scene understanding have recently been proposed: "Intuitive Physics Engines", or IPEs, which posit that people make predictions by running approximate probabilistic simulations in causal mental models similar in nature to video-game physics engines, and memory-based models, which make judgments based on analogies to stored experiences of previously encountered scenes and physical outcomes. Versions of the latter have recently been instantiated in convolutional neural network (CNN) architectures. Here we report four experiments that, to our knowledge, are the first rigorous comparisons of simulation-based and CNN-based models, where both approaches are concretely instantiated in algorithms that can run on raw image inputs and produce as outputs physical judgments such as whether a stack of blocks will fall. Both approaches can achieve super-human accuracy levels and can quantitatively predict human judgments to a similar degree, but only the simulation-based models generalize to novel situations in ways that people do, and are qualitatively consistent with systematic perceptual illusions and judgment asymmetries that people show.
from cs.AI updates on arXiv.org http://ift.tt/21ynqLq
via IFTTT
A Step from Probabilistic Programming to Cognitive Architectures. (arXiv:1605.01180v1 [cs.AI])
Probabilistic programming is considered as a framework, in which basic components of cognitive architectures can be represented in unified and elegant fashion. At the same time, necessity of adopting some component of cognitive architectures for extending capabilities of probabilistic programming languages is pointed out. In particular, implicit specification of generative models via declaration of concepts and links between them is proposed, and usefulness of declarative knowledge for achieving efficient inference is briefly discussed.
from cs.AI updates on arXiv.org http://ift.tt/1rvJslD
via IFTTT
Ontology-Mediated Queries: Combined Complexity and Succinctness of Rewritings via Circuit Complexity. (arXiv:1605.01207v1 [cs.DB])
We give solutions to two fundamental computational problems in ontology-based data access with the W3C standard ontology language OWL 2 QL: the succinctness problem for first-order rewritings of ontology-mediated queries (OMQs), and the complexity problem for OMQ answering. We classify OMQs according to the shape of their conjunctive queries (treewidth, the number of leaves) and the existential depth of their ontologies. For each of these classes, we determine the combined complexity of OMQ answering, and whether all OMQs in the class have polynomial-size first-order, positive existential, and nonrecursive datalog rewritings. We obtain the succinctness results using hypergraph programs, a new computational model for Boolean functions, which makes it possible to connect the size of OMQ rewritings and circuit complexity.
from cs.AI updates on arXiv.org http://ift.tt/1SZzGBf
via IFTTT
Learning from the memory of Atari 2600. (arXiv:1605.01335v1 [cs.LG])
We train a number of neural networks to play games Bowling, Breakout and Seaquest using information stored in the memory of a video game console Atari 2600. We consider four models of neural networks which differ in size and architecture: two networks which use only information contained in the RAM and two mixed networks which use both information in the RAM and information from the screen. As the benchmark we used the convolutional model proposed in NIPS and received comparable results in all considered games. Quite surprisingly, in the case of Seaquest we were able to train RAM-only agents which behave better than the benchmark screen-only agent. Mixing screen and RAM did not lead to an improved performance comparing to screen-only and RAM-only agents.
from cs.AI updates on arXiv.org http://ift.tt/1WJHrOn
via IFTTT
Gated Graph Sequence Neural Networks. (arXiv:1511.05493v3 [cs.LG] UPDATED)
Graph-structured data appears frequently in domains including chemistry, natural language semantics, social networks, and knowledge bases. In this work, we study feature learning techniques for graph-structured inputs. Our starting point is previous work on Graph Neural Networks (Scarselli et al., 2009), which we modify to use gated recurrent units and modern optimization techniques and then extend to output sequences. The result is a flexible and broadly useful class of neural network models that has favorable inductive biases relative to purely sequence-based models (e.g., LSTMs) when the problem is graph-structured. We demonstrate the capabilities on some simple AI (bAbI) and graph algorithm learning tasks. We then show it achieves state-of-the-art performance on a problem from program verification, in which subgraphs need to be matched to abstract data structures.
from cs.AI updates on arXiv.org http://ift.tt/1HXcQTm
via IFTTT
Semantics for probabilistic programming: higher-order functions, continuous distributions, and soft constraints. (arXiv:1601.04943v3 [cs.PL] UPDATED)
We study the semantic foundation of expressive probabilistic programming languages, that support higher-order functions, continuous distributions, and soft constraints (such as Anglican, Church, and Venture). We define a metalanguage (an idealised version of Anglican) for probabilistic computation with the above features, develop both operational and denotational semantics, and prove soundness, adequacy, and termination. They involve measure theory, stochastic labelled transition systems, and functor categories, but admit intuitive computational readings, one of which views sampled random variables as dynamically allocated read-only variables. We apply our semantics to validate nontrivial equations underlying the correctness of certain compiler optimisations and inference algorithms such as sequential Monte Carlo simulation. The language enables defining probability distributions on higher-order functions, and we study their properties.
from cs.AI updates on arXiv.org http://ift.tt/1ltdYJ2
via IFTTT
I have a new follower on Twitter
Healthy Living
Bringing you the latest #health news & alternative treatment solutions. Featured alternative treatment: https://t.co/mNNsYj1mLj
United States
https://t.co/pZlRVSPb7g
Following: 2316 - Followers: 2091
May 04, 2016 at 07:28PM via Twitter http://twitter.com/HealthLivingUSA
Ravens Video: Players show off well-timed photobombing skills on unsuspecting fans at draft party (ESPN)
via IFTTT
Anonymous attacks Greek Central Bank and vows to take down more banks' sites
from Google Alert - anonymous http://ift.tt/1Y8kLpF
via IFTTT
Anonymous Hacker Group Plans To Attack Banks Worldwide
from Google Alert - anonymous http://ift.tt/1Tm5JZc
via IFTTT
Update theme By Anonymous?
from Google Alert - anonymous http://ift.tt/1rnj3G9
via IFTTT
ISS Daily Summary Report – 05/03/16
from ISS On-Orbit Status Report http://ift.tt/1rnfZtC
via IFTTT
Warning — Widely Popular ImageMagick Tool Vulnerable to Remote Code Execution
from The Hacker News http://ift.tt/1SNcXpT
via IFTTT
Anonymous attack Greek central bank, warns others
from Google Alert - anonymous http://ift.tt/1NV9Y1F
via IFTTT
Anonymous Subscriptions
from Google Alert - anonymous http://ift.tt/1SLoidm
via IFTTT
Aurora over Sweden
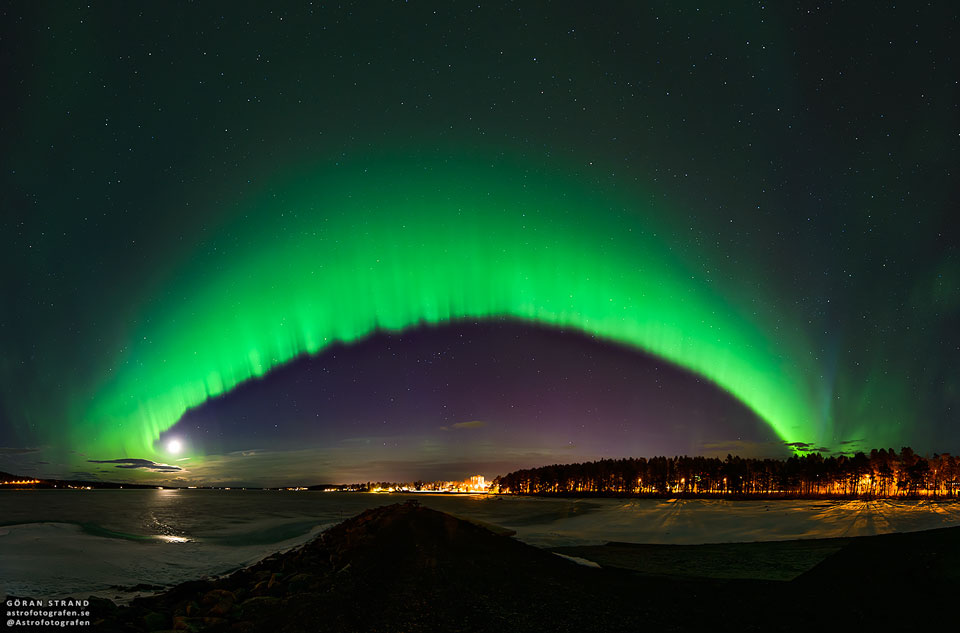
Tuesday, May 3, 2016
Orioles Video: Hot-hitting Mark Trumbo (.337 BA) belts a solo HR and a 2-run shot in 4-1 victory over the Yankees (ESPN)
via IFTTT
Disjunctive Normal Form Schemes for Heterogeneous Attributed Graphs. (arXiv:1605.00686v1 [cs.AI])
Several 'edge-discovery' applications over graph-based data models are known to have worst-case quadratic complexity, even if the discovered edges are sparse. One example is the generic link discovery problem between two graphs, which has invited research interest in several communities. Specific versions of this problem include link prediction in social networks, ontology alignment between metadata-rich RDF data, approximate joins, and entity resolution between instance-rich data. As large datasets continue to proliferate, reducing quadratic complexity to make the task practical is an important research problem. Within the entity resolution community, the problem is commonly referred to as blocking. A particular class of learnable blocking schemes is known as Disjunctive Normal Form (DNF) blocking schemes, and has emerged as state-of-the art for homogeneous (i.e. same-schema) tabular data. Despite the promise of these schemes, a formalism or learning framework has not been developed for them when input data instances are generic, attributed graphs possessing both node and edge heterogeneity. With such a development, the complexity-reducing scope of DNF schemes becomes applicable to a variety of problems, including entity resolution and type alignment between heterogeneous RDF graphs, and link prediction in networks represented as attributed graphs. This paper presents a graph-theoretic formalism for DNF schemes, and investigates their learnability in an optimization framework. Experimentally, the DNF schemes learned on pairs of heterogeneous RDF graphs are demonstrated to achieve high complexity-reductions (98.25% across ten RDF test cases) at little cost to coverage, and with high reliability (<2.5% standard deviation). Finally, one extant class of RDF blocking schemes is shown to be a special case of DNF schemes.
from cs.AI updates on arXiv.org http://ift.tt/24oLh5k
via IFTTT
A heuristic algorithm for a single vehicle static bike sharing rebalancing problem. (arXiv:1605.00702v1 [cs.AI])
The static bike rebalancing problem (SBRP) concerns the task of repositioning bikes among stations in self-service bike-sharing systems. This problem can be seen as a variant of the one-commodity pickup and delivery vehicle routing problem, where multiple visits are allowed to be performed at each station, i.e., the demand of a station is allowed to be split. Moreover, a vehicle may temporarily drop its load at a station, leaving it in excess or, alternatively, collect more bikes from a station (even all of them), thus leaving it in default. Both cases require further visits in order to meet the actual demands of such station. This paper deals with a particular case of the SBRP, in which only a single vehicle is available and the objective is to find a least-cost route that meets the demand of all stations and does not violate the minimum (zero) and maximum (vehicle capacity) load limits along the tour. Therefore, the number of bikes to be collected or delivered at each station should be appropriately determined in order to respect such constraints. We propose an iterated local search (ILS) based heuristic to solve the problem. The ILS algorithm was tested on 980 benchmark instances from the literature and the results obtained are quite competitive when compared to other existing methods. Moreover, our heuristic was capable of finding most of the known optimal solutions and also of improving the results on a number of open instances.
from cs.AI updates on arXiv.org http://ift.tt/26RiFR1
via IFTTT
Obstacle evasion using fuzzy logic in a sliding blades problem environment. (arXiv:1605.00787v1 [cs.AI])
This paper discusses obstacle avoidance using fuzzy logic and shortest path algorithm. This paper also introduces the sliding blades problem and illustrates how a drone can navigate itself through the swinging blade obstacles while tracing a semi-optimal path and also maintaining constant velocity
from cs.AI updates on arXiv.org http://ift.tt/1Za6SHF
via IFTTT
Online Learning of Commission Avoidant Portfolio Ensembles. (arXiv:1605.00788v1 [cs.AI])
We present a novel online ensemble learning strategy for portfolio selection. The new strategy controls and exploits any set of commission-oblivious portfolio selection algorithms. The strategy handles transaction costs using a novel commission avoidance mechanism. We prove a logarithmic regret bound for our strategy with respect to optimal mixtures of the base algorithms. Numerical examples validate the viability of our method and show significant improvement over the state-of-the-art.
from cs.AI updates on arXiv.org http://ift.tt/26RiHse
via IFTTT
Fast Simulation of Probabilistic Boolean Networks (Technical Report). (arXiv:1605.00854v1 [cs.CE])
Probabilistic Boolean networks (PBNs) is an important mathematical framework widely used for modelling and analysing biological systems. PBNs are suited for modelling large biological systems, which more and more often arise in systems biology. However, the large system size poses a~significant challenge to the analysis of PBNs, in particular, to the crucial analysis of their steady-state behaviour. Numerical methods for performing steady-state analyses suffer from the state-space explosion problem, which makes the utilisation of statistical methods the only viable approach. However, such methods require long simulations of PBNs, rendering the simulation speed a crucial efficiency factor. For large PBNs and high estimation precision requirements, a slow simulation speed becomes an obstacle. In this paper, we propose a structure-based method for fast simulation of PBNs. This method first performs a network reduction operation and then divides nodes into groups for parallel simulation. Experimental results show that our method can lead to an approximately 10 times speedup for computing steady-state probabilities of a real-life biological network.
from cs.AI updates on arXiv.org http://ift.tt/1Za6RDM
via IFTTT
Cascading A*: a Parallel Approach to Approximate Heuristic Search. (arXiv:1406.0955v2 [cs.AI] UPDATED)
In this paper, we proposed a new approximate heuristic search algorithm: Cascading A*, which is a two-phrase algorithm combining A* and IDA* by a new concept "envelope ball". The new algorithm CA* is efficient, able to generate approximate solution and any-time solution, and parallel friendly.
from cs.AI updates on arXiv.org http://ift.tt/1mc4Lyt
via IFTTT
Incorporating Knowledge into Structural Equation Models using Auxiliary Variables. (arXiv:1511.02995v3 [stat.ME] UPDATED)
In this paper, we extend graph-based identification methods by allowing background knowledge in the form of non-zero parameter values. Such information could be obtained, for example, from a previously conducted randomized experiment, from substantive understanding of the domain, or even an identification technique. To incorporate such information systematically, we propose the addition of auxiliary variables to the model, which are constructed so that certain paths will be conveniently cancelled. This cancellation allows the auxiliary variables to help conventional methods of identification (e.g., single-door criterion, instrumental variables, half-trek criterion), as well as model testing (e.g., d-separation, over-identification). Moreover, by iteratively alternating steps of identification and adding auxiliary variables, we can improve the power of existing identification methods via a bootstrapping approach that does not require external knowledge. We operationalize this method for simple instrumental sets (a generalization of instrumental variables) and show that the resulting method is able to identify at least as many models as the most general identification method for linear systems known to date. We further discuss the application of auxiliary variables to the tasks of model testing and z-identification.
from cs.AI updates on arXiv.org http://ift.tt/1OD10FS
via IFTTT
I have a new follower on Twitter
K Grimes
Its time to build mass. Using my program you will hit your goal
https://t.co/KWStblqDgI
Following: 281 - Followers: 2
May 03, 2016 at 07:32PM via Twitter http://twitter.com/RoachMadalyn
Bicinium No.23 (Anonymous)
from Google Alert - anonymous http://ift.tt/1rSrVV5
via IFTTT
Bicinium No.24 (Anonymous)
from Google Alert - anonymous http://ift.tt/1Z9Marv
via IFTTT
Orioles: Manny Machado's \"value soars even higher\" with move to SS following injury to J.J. Hardy, Eddie Matz writes (ESPN)
via IFTTT
Craig Wright Will Move Satoshi Nakamoto's Bitcoin to Prove His Claim
from The Hacker News http://ift.tt/26PT4Ih
via IFTTT
[FD] LSE Leading Security Experts GmbH - LSE-2016-02-03 - OXID eShop Path Traversal Vulnerability
Source: Gmail -> IFTTT-> Blogger
[FD] CVE-2016-3627 CVE-2016-3705: libxml2: stack overflow in xml validator (parser)
Source: Gmail -> IFTTT-> Blogger
[FD] Mobile Security Framework (MobSF) v0.9.2 Released
Source: Gmail -> IFTTT-> Blogger
[FD] Moxa MiiNePort - Multiple Vulnerabilities
Source: Gmail -> IFTTT-> Blogger
[FD] real dangers of gsm setups
Source: Gmail -> IFTTT-> Blogger
[FD] Garage4hackers Ranchoddas Webcast Series CTF Challenge
Source: Gmail -> IFTTT-> Blogger
[FD] New BlackArch Linux ISOs (2016.04.28) and Installer released
Source: Gmail -> IFTTT-> Blogger
[FD] CVE-2016-3078: php: integer overflow in ZipArchive::getFrom*
Source: Gmail -> IFTTT-> Blogger
Russian Hacker Who Stole From Banks Ordered to Pay $7 Million
from The Hacker News http://ift.tt/23lHX53
via IFTTT
Brazil blocks WhatsApp for 72-Hours — Here's Why
from The Hacker News http://ift.tt/21sCkD1
via IFTTT
I have a new follower on Twitter
CraigBloem
Entrepreneur, Marketing Authority, Business Leader. CEO & Founder https://t.co/enZ8JpzBci (LogoMix Inc.), formerly cofounder at Performable acquired by HubSpot.
Boston, MA
https://t.co/enZ8JpzBci
Following: 1717 - Followers: 1783
May 03, 2016 at 01:32AM via Twitter http://twitter.com/craigbloem
I have a new follower on Twitter
Jan Oldenburg
Focused on digital health and participatory medicine. Chief editor of Engage! Transforming Healthcare Through Digital Patient Engagement.
Boise ID
https://t.co/H0cGCuegk3
Following: 8282 - Followers: 11252
May 03, 2016 at 12:31AM via Twitter http://twitter.com/janoldenburg
Crossing Mars

Monday, May 2, 2016
Coupon error for anonymous user
from Google Alert - anonymous http://ift.tt/1SI11Jk
via IFTTT
Deep Convolutional Neural Networks on Cartoon Functions. (arXiv:1605.00031v1 [cs.IT])
Wiatowski and B\"olcskei, 2015, proved that deformation stability and vertical translation invariance of deep convolutional neural network-based feature extractors are guaranteed by the network structure per se rather than the specific convolution kernels and non-linearities. While the translation invariance result applies to square-integrable functions, the deformation stability bound holds for band-limited functions only. Many signals of practical relevance (such as natural images) exhibit, however, sharp and curved discontinuities and are hence not band-limited. The main contribution of this paper is a deformation stability result that takes these structural properties into account. Specifically, we establish deformation stability bounds for the class of cartoon functions introduced by Donoho, 2001.
from cs.AI updates on arXiv.org http://ift.tt/1rfvCmO
via IFTTT
Higher Order Recurrent Neural Networks. (arXiv:1605.00064v1 [cs.NE])
In this paper, we study novel neural network structures to better model long term dependency in sequential data. We propose to use more memory units to keep track of more preceding states in recurrent neural networks (RNNs), which are all recurrently fed to the hidden layers as feedback through different weighted paths. By extending the popular recurrent structure in RNNs, we provide the models with better short-term memory mechanism to learn long term dependency in sequences. Analogous to digital filters in signal processing, we call these structures as higher order RNNs (HORNNs). Similar to RNNs, HORNNs can also be learned using the back-propagation through time method. HORNNs are generally applicable to a variety of sequence modelling tasks. In this work, we have examined HORNNs for the language modeling task using two popular data sets, namely the Penn Treebank (PTB) and English text8 data sets. Experimental results have shown that the proposed HORNNs yield the state-of-the-art performance on both data sets, significantly outperforming the regular RNNs as well as the popular LSTMs.
from cs.AI updates on arXiv.org http://ift.tt/24kwdG0
via IFTTT
Indoor Massive MIMO: Uplink Pilot Mitigation Using Channel State Information Map. (arXiv:1605.00082v1 [cs.NI])
Massive MIMO brings both motivations and challenges to develop the 5th generation Mobile wireless technology. The promising number of users and the high bitrate offered per unit area are challenged by uplink pilot contamination due to pilot reuse and a limited number of orthogonal pilot sequences. This paper proposes a solution to mitigate uplink pilot contamination in an indoor scenario where multi-cell share the same pool of pilot sequences, that are supposed to be less than the number of users. This can be done by reducing uplink pilots using Channel State Information (CSI) prediction. The proposed method is based on machine learning approach, where a quantized version of Channel State Information (QCSI) is learned during estimation session and stored at the Base Station (BS) to be exploited for future CSI prediction. The learned QCSI are represented by a weighted directed graph, which is responsible to monitor and predict the CSI of User Terminals (UTs) in the local cell. We introduce an online learning algorithm to create and update this graph which we call CSI map. Simulation results show an increase in the downlink sum-rate and a significant feedback reduction.
from cs.AI updates on arXiv.org http://ift.tt/1rfvALM
via IFTTT
Look-ahead before you leap: end-to-end active recognition by forecasting the effect of motion. (arXiv:1605.00164v1 [cs.CV])
Visual recognition systems mounted on autonomous moving agents face the challenge of unconstrained data, but simultaneously have the opportunity to improve their performance by moving to acquire new views of test data. In this work, we first show how a recurrent neural network-based system may be trained to perform end-to-end learning of motion policies suited for the "active recognition" setting. Further, we hypothesize that active vision requires an agent to have the capacity to reason about the effects of its motions on its view of the world. To verify this hypothesis, we attempt to induce this capacity in our active recognition pipeline, by simultaneously learning to forecast the effects of the agent's motions on its internal representation of its cumulative knowledge obtained from all past views. Results across two challenging datasets confirm both that our end-to-end system successfully learns meaningful policies for active recognition, and that "learning to look ahead" further boosts recognition performance.
from cs.AI updates on arXiv.org http://ift.tt/24kwdpk
via IFTTT
Common-Description Learning: A Framework for Learning Algorithms and Generating Subproblems from Few Examples. (arXiv:1605.00241v1 [cs.AI])
Current learning algorithms face many difficulties in learning simple patterns and using them to learn more complex ones. They also require more examples than humans do to learn the same pattern, assuming no prior knowledge. In this paper, a new learning framework is introduced that is called common-description learning (CDL). This framework has been tested on 32 small multi-task datasets, and the results show that it was able to learn complex algorithms from a few number of examples. The final model is perfectly interpretable and its depth depends on the question. What is meant by depth here is that whenever needed, the model learns to break down the problem into simpler subproblems and solves them using previously learned models. Finally, we explain the capabilities of our framework in discovering complex relations in data and how it can help in improving language understanding in machines.
from cs.AI updates on arXiv.org http://ift.tt/1rfvAvp
via IFTTT
A Self-Taught Artificial Agent for Multi-Physics Computational Model Personalization. (arXiv:1605.00303v1 [cs.CE])
Personalization is the process of fitting a model to patient data, a critical step towards application of multi-physics computational models in clinical practice. Designing robust personalization algorithms is often a tedious, time-consuming, model- and data-specific process. We propose to use artificial intelligence concepts to learn this task, inspired by how human experts manually perform it. The problem is reformulated in terms of reinforcement learning. In an off-line phase, Vito, our self-taught artificial agent, learns a representative decision process model through exploration of the computational model: it learns how the model behaves under change of parameters. The agent then automatically learns an optimal strategy for on-line personalization. The algorithm is model-independent; applying it to a new model requires only adjusting few hyper-parameters of the agent and defining the observations to match. The full knowledge of the model itself is not required. Vito was tested in a synthetic scenario, showing that it could learn how to optimize cost functions generically. Then Vito was applied to the inverse problem of cardiac electrophysiology and the personalization of a whole-body circulation model. The obtained results suggested that Vito could achieve equivalent, if not better goodness of fit than standard methods, while being more robust (up to 11% higher success rates) and with faster (up to seven times) convergence rate. Our artificial intelligence approach could thus make personalization algorithms generalizable and self-adaptable to any patient and any model.
from cs.AI updates on arXiv.org http://ift.tt/24kwdpg
via IFTTT
Coalition Formability Semantics with Conflict-Eliminable Sets of Arguments. (arXiv:1605.00495v1 [cs.AI])
We consider abstract-argumentation-theoretic coalition formability in this work. Taking a model from political alliance among political parties, we will contemplate profitability, and then formability, of a coalition. As is commonly understood, a group forms a coalition with another group for a greater good, the goodness measured against some criteria. As is also commonly understood, however, a coalition may deliver benefits to a group X at the sacrifice of something that X was able to do before coalition formation, which X may be no longer able to do under the coalition. Use of the typical conflict-free sets of arguments is not very fitting for accommodating this aspect of coalition, which prompts us to turn to a weaker notion, conflict-eliminability, as a property that a set of arguments should primarily satisfy. We require numerical quantification of attack strengths as well as of argument strengths for its characterisation. We will first analyse semantics of profitability of a given conflict-eliminable set forming a coalition with another conflict-eliminable set, and will then provide four coalition formability semantics, each of which formalises certain utility postulate(s) taking the coalition profitability into account.
from cs.AI updates on arXiv.org http://ift.tt/1rfvC6g
via IFTTT
Graph Clustering Bandits for Recommendation. (arXiv:1605.00596v1 [stat.ML])
We investigate an efficient context-dependent clustering technique for recommender systems based on exploration-exploitation strategies through multi-armed bandits over multiple users. Our algorithm dynamically groups users based on their observed behavioral similarity during a sequence of logged activities. In doing so, the algorithm reacts to the currently served user by shaping clusters around him/her but, at the same time, it explores the generation of clusters over users which are not currently engaged. We motivate the effectiveness of this clustering policy, and provide an extensive empirical analysis on real-world datasets, showing scalability and improved prediction performance over state-of-the-art methods for sequential clustering of users in multi-armed bandit scenarios.
from cs.AI updates on arXiv.org http://ift.tt/24kwd8Y
via IFTTT
Clustering Markov Decision Processes For Continual Transfer. (arXiv:1311.3959v4 [cs.AI] UPDATED)
We present algorithms to effectively represent a set of Markov decision processes (MDPs), whose optimal policies have already been learned, by a smaller source subset for lifelong, policy-reuse-based transfer learning in reinforcement learning. This is necessary when the number of previous tasks is large and the cost of measuring similarity counteracts the benefit of transfer. The source subset forms an `$\epsilon$-net' over the original set of MDPs, in the sense that for each previous MDP $M_p$, there is a source $M^s$ whose optimal policy has $<\epsilon$ regret in $M_p$. Our contributions are as follows. We present EXP-3-Transfer, a principled policy-reuse algorithm that optimally reuses a given source policy set when learning for a new MDP. We present a framework to cluster the previous MDPs to extract a source subset. The framework consists of (i) a distance $d_V$ over MDPs to measure policy-based similarity between MDPs; (ii) a cost function $g(\cdot)$ that uses $d_V$ to measure how good a particular clustering is for generating useful source tasks for EXP-3-Transfer and (iii) a provably convergent algorithm, MHAV, for finding the optimal clustering. We validate our algorithms through experiments in a surveillance domain.
from cs.AI updates on arXiv.org http://ift.tt/1hQHbuA
via IFTTT
A hybrid swarm-based algorithm for single-objective optimization problems involving high-cost analyses. (arXiv:1402.5830v2 [math.OC] UPDATED)
In many technical fields, single-objective optimization procedures in continuous domains involve expensive numerical simulations. In this context, an improvement of the Artificial Bee Colony (ABC) algorithm, called the Artificial super-Bee enhanced Colony (AsBeC), is presented. AsBeC is designed to provide fast convergence speed, high solution accuracy and robust performance over a wide range of problems. It implements enhancements of the ABC structure and hybridizations with interpolation strategies. The latter are inspired by the quadratic trust region approach for local investigation and by an efficient global optimizer for separable problems. Each modification and their combined effects are studied with appropriate metrics on a numerical benchmark, which is also used for comparing AsBeC with some effective ABC variants and other derivative-free algorithms. In addition, the presented algorithm is validated on two recent benchmarks adopted for competitions in international conferences. Results show remarkable competitiveness and robustness for AsBeC.
from cs.AI updates on arXiv.org http://ift.tt/1hMn553
via IFTTT
A Repeated Signal Difference for Recognising Patterns. (arXiv:1604.05170v2 [cs.NE] UPDATED)
This paper describes a new mechanism that might help with defining pattern sequences, by the fact that it can produce an upper bound on the ensemble value that can persistently oscillate with the actual values produced from each pattern. With every firing event, a node also receives an on/off feedback switch. If the node fires, then it sends a feedback result depending on the input signal strength. If the input signal is positive or larger, it can store 'on' switch feedback for the next iteration. If the signal is negative or smaller, it can store an 'off' switch feedback for the next iteration. If the node does not fire, then it does not affect the current feedback situation and receives the switch command produced by the last active pattern event for the same neuron. The upper bound therefore also represents the largest or most enclosing pattern set and the lower value is for the actual set of firing patterns. If the pattern sequence repeats, it will oscillate between the two values, allowing them to be recognised and measured more easily, over time. Tests show that changing the sequence ordering can also be measured.
from cs.AI updates on arXiv.org http://ift.tt/23UuRhj
via IFTTT
Anonymous user 839d2f
from Google Alert - anonymous http://ift.tt/23jWT3R
via IFTTT
Ravens not picking up S Matt Elam's 5th-year $5.6M contract option; missed all of 2015 with torn biceps (ESPN)
via IFTTT
Orioles: SS J.J. Hardy expected to miss 6-8 weeks with hairline fracture in left foot; INF Ryan Flaherty to be recalled (ESPN)
via IFTTT
Wrongly made questionnaire anonymous
from Google Alert - anonymous http://ift.tt/24jdYkb
via IFTTT
Ravens Video: Todd McShay says team may have had a historic 4th round in draft; \"I've never seen anything like it\" (ESPN)
via IFTTT
Charles Finney School gets anonymous $1 million donation
from Google Alert - anonymous http://ift.tt/1TG2v5D
via IFTTT
Ravens: Tears were shed in draft room with the selection of Navy QB Keenan Reynolds, Jamison Hensley writes (ESPN)
via IFTTT
Accessing RPi.GPIO and GPIO Zero with OpenCV + Python
I can’t believe this is the first time I am writing a blog post on GPIO and the Raspberry Pi. It’s a pretty big mistake on my part. I should have written this post much earlier.
You see, on average, I receive 1-3 emails per week along the lines of:
When I use the ‘cv’ virtual environment, I can access my OpenCV bindings. But then I can’t import RPi.GPIO. When I switch out of the virtual environment (and use the system Python), I can use RPi.GPIO, but I can’t import OpenCV. What gives?
The reason for this problem is that RPi.GPIO/GPIO Zero were not installed into your Python virtual environment! To fix this issue, all you need to do is use
pipto install them into your virtual environment — from there, you’ll be good to go.
But to be honest with you, I don’t think that’s the real issue here!
The real problem is not having a sufficient understanding of what Python virtual environments are and why we use them. Utilizing Python virtual environments is a best practice that you need to become comfortable with.
In the remainder of this blog post, I’ll gently introduce the concept of Python virtual environments. And from there, we’ll learn how to install RPi.GPIO and GPIO Zero into the same Python virtual environment as our OpenCV bindings, allowing us to access both OpenCV and RPi.GPIO/GPIO Zero at the same time!
Keep reading to find out how…
Looking for the source code to this post?
Jump right to the downloads section.
Accessing RPi.GPIO and GPIO Zero with OpenCV + Python
Before we learn how to (correctly) install RPi.GPIO and GPIO Zero on our Raspberry Pi, we first need to review the concept of Python virtual environments.
If you’ve ever followed one of the Raspberry Pi + OpenCV install tutorials on the PyImageSearch blog, you’ll know that I’m a huge fan of Python virtual environments and recommend them for nearly every project.
However, it seems that I haven’t done a good enough job explaining what Python virtual environments are and why we use them. The following section should help clear up any questions.
What are Python virtual environments?
At the very core, Python virtual environments allow us to create isolated, independent environments for each of our Python projects. This implies that each project can have its own set of dependencies, regardless of which dependencies another project has.
So why in the world would we want to create a virtual environment for each of our projects?
Consider this: Suppose we are software contractors and are hired by a company to develop ProjectA. But before we have completed ProjectA, a second company hires us to develop ProjectB. We notice that both ProjectA and ProjectB have a dependency on LibraryA…but the problem is that ProjectA requires v1.0.0 of LibraryA while ProjectB requires v2.0.0!
This is a real issue for Python because we cannot install two different versions of the same library into the same
site-packagesdirectory (i.e., where Python stores 3rd party libraries, such as the ones you download and install from
pip, GitHub, etc.).
So, what do we do?
Do we run out to the nearest Apple Store and buy a new MacBook Pro so we can use one laptop to develop ProjectA and the other to develop ProjectB? I really hope not. That would get expensive quick.
Do use a web host like Linode, Digital Ocean, or Amazon EC2 and spin-up a new instance for each project? This is a better solution, and is highly applicable in some cases, but in our specific instance, it’s overkill.
Or do we use Python virtual environments?
You guessed it — we go with Python virtual environments.
In this case, all we need to do is create a virtual environment for each project, that way there is a separate, isolated, and independent environment for all projects:
Figure 1: Each Python environment we create is separate and independent from the others.
This allows us to install completely different dependencies for ProjectA and ProjectB, ensuring we can finish development of both projects on the same computer.
Pretty neat, right?
Of course, there are many more benefits to using Python virtual environments, but instead of listing them all out, please refer to this excellent Python virtual environments primer on the RealPython blog.
But Adrian, I’m already using Python virtual environments!
If you followed any of the OpenCV install tutorials on the PyImageSearch blog, then you’re already using Python virtual environments.
So how can you find out for sure?
If you need to execute
workon <virtual environment name>before executing your Python script to import OpenCV, then guess what, you’re using Python virtual environments.
But here is the real problem…
You probably installed RPi.GPIO and GPIO Zero incorrectly
“Incorrect” is the wrong word to use here — but I needed to get your attention. When you went to install RPi.GPIO or GPIO Zero, I’m willing to bet you used
apt-get. Your command probably looked (something) like this:
$ sudo apt-get install python-rpi.gpio python-gpiozero
This command will download RPi.GPIO and GPIO Zero from the official Raspbian package repository and install them on your system.
The problem is that
apt-getwill install packages into the system install of Python and not your Python virtual environments.
And this is exactly why you run into problems like:
I can import OpenCV in the ‘cv’ virtual environment, but I can’t import RPi.GPIO. On the other hand, I can import RPi.GPIO outside of the ‘cv’ environment, but then I can’t import cv2.
How do you resolve this issue?
You just need to install your libraries into your virtual environment rather than the system version of Python, which can easily be accomplished by
workonand
pip.
This brings me to my next point:
I do not recommend using
apt-getto install Python libraries.
You’re probably thinking, “But Adrian, using
apt-getis so easy! It’s just one command and then I’m done!”
I’ve got news for you — using
pipis also one command. And it’s just as easy.
To get back to my point, not only will
apt-getinstall these libraries into the system Python (rather than your virtual environment), there is also the issue that
apt-getpackages are normally a bit out of date.
And guess what happens when you want to install the newest version of a given library?
Hint: You run into the exact problem detailed above — you’ll be trying to install two different versions of the same library into the same
site-packagesdirectory, which simply cannot happen (due to how Python works).
Instead, you should be using the Python package manager, pip, to install your Python packages into virtual environments. For more information on
pip, how it works, and why we use it, please refer to this article.
Installing RPi.GPIO and GPIO Zero “correctly”
Let’s go ahead and get RPi.GPIO and GPIO zero installed into our Python virtual environment. To start, first use the
workoncommand to enter your Python virtual environment:
$ workon <virtual environment name>
Note: You may need to execute
source ~/.profileprior to running the
workoncommand so that the virtual environment startup scripts are loaded.
You can tell you are in a Python virtual environment if the name of the environment appears in parenthesis before your prompt:
Figure 2: I can tell I am in the “cv” virtual environment because I can see the text “(cv)” before my prompt.
In this case, I have entered the
cvvirtual environment and I can verify this because I see the text “(cv)” before my prompt.
From there, we can let
pipinstall RPi.GPIO and GPIO Zero for us:
$ pip install RPi.GPIO $ pip install gpiozero
Lastly, let’s test the install and ensure we can import RPi.GPIO, GPIO Zero, and OpenCV together:
$ python >>> import RPi.GPIO >>> import gpiozero >>> import cv2 >>>
Note: I’ve made the assumption that the virtual environment you are using already has OpenCV installed in it. My
cvvirtual environment has OpenCV already installed, so by using
pipto install the
RPi.GPIOand
gpiozeroto install the respective GPIO packages, I’m able to access all three libraries from within the same environment.
Once you can import these libraries in the same environment, we’re ready to move on.
Hardware
For this blog post, I used my Raspberry Pi 3 and the TrafficHAT board, a really cool module for the Raspberry Pi that allows you to get started quickly and easily with GPIO programming:
Figure 3: The TrafficHAT module for the Raspberry Pi, which includes 3 LED lights, a buzzer, and push button, all of which are programmable via GPIO.
As you can see, the TrafficHAT includes 3 big LED lights, a push-button, and a buzzer.
Note: It’s called a “hat” because we simply need to set it on top of the GPIO pins — no breakout board, extra cables, or soldering is required.
Once we have the TrafficHAT installed on the Raspberry Pi, we can program it using nearly any programming language (provided the language can access the GPIO pins), but for the purposes of this blog post, we’ll be using Python + RPi.GPIO and GPIO Zero.
Using RPi.GPIO + OpenCV
Let’s go ahead and write some code to access the TrafficHAT board using the RPi.GPIO library. We’ll also utilize OpenCV to load an image from file and display it to our screen.
Open up a new file, name it
gpio_demo.py, and insert the following code:
# import the necessary packages import RPi.GPIO as GPIO import time import cv2 # load the input image and display it to our screen print("click on the image and press any key to continue...") image = cv2.imread("hoover_dam.jpg") cv2.imshow("Image", image) cv2.waitKey(0) print("moving on...") # set the GPIO mode GPIO.setmode(GPIO.BCM) # loop over the LEDs on the TrafficHat and light each one # individually for i in (22, 23, 24): GPIO.setup(i, GPIO.OUT) GPIO.output(i, GPIO.HIGH) time.sleep(3.0) GPIO.output(i, GPIO.LOW) # perform a bit of cleanup GPIO.cleanup()
Lines 2-4 handle importing our required Python packages. We then load the input image from disk and display it to our screen on Lines 8-10. Our script will pause execution until we click on the active image window and press any key on our keyboard.
From there, we loop over each of the LEDs on the TrafficHAT (Line 18). For each of these lights, we:
- Turn the LED light on.
- Wait 3 seconds.
- Turn the light off and continue looping.
To execute
gpio_demo.py, make sure you are in the
cvvirtual environment (or whatever virtual environment you are using to store your OpenCV bindings + GPIO libraries) by using the
workoncommand:
$ workon cv
We can then run the
gpio_demo.pyscript:
$ python gpio_demo.py
As the output image demonstrates, we can see that our
hoover_dam.jpgimage is displayed to the screen and the green LED lights is shining brightly on the TrafficHAT:
Figure 4: Loading an image to my screen using OpenCV and then lighting up the green LED using GPIO.
What about root?
But what if we wanted to execute
gpio_demo.pyas the root user? What do we do then?
We have two options here.
The first option is to use the
sudocommand inside our Python virtual environment, like this:
$ sudo python gpio_demo.py
Note: Make sure you are in your Python virtual environment before executing your script with
sudo; otherwise, you will not have access to the libraries installed in your virtual environment.
The second option is to launch a root shell, access our Python virtual environment, and then execute the script:
$ sudo /bin/bash $ source /home/pi/.profile $ workon cv $ python gpio_demo.py
Which one is best?
To be honest, it doesn’t really matter.
There are some cases where using
sudois easier. And there are others where it’s nice to simply have a root shell pulled up. Use whichever option you’re more comfortable with — just be careful when executing commands as root!
Using RPI Zero + OpenCV
Now that we’ve explored RPi.GPIO, let’s re-create the same Python script, but this time using the GPIO Zero library. Open up a different file, name it
gpiozero_demo.py, and insert the following code:
# import the necessary packages from gpiozero import TrafficHat import time import cv2 # load the input image and display it to our screen print("click on the image and press any key to continue...") image = cv2.imread("hoover_dam.jpg") cv2.imshow("Image", image) cv2.waitKey(0) print("moving on...") # initialize the TrafficHAT, then create the list of lights th = TrafficHat() lights = (th.lights.green, th.lights.amber, th.lights.red) # loop over the lights and turn them on one-by-one for light in lights: light.on() time.sleep(3.0) light.off()
Lines 2-4 again handle importing our required packages. What’s really interesting here is that the
gpiozerolibrary has a
TrafficHatclass, which enables us to easily interface with the TrafficHAT module.
Lines 8-10 handle loading and displaying our input image to sour screen.
We can then initialize the
TrafficHatobject and construct the list of
lightson Lines 14 and 15.
Finally, Lines 18-21 handle looping over each of the
lights, turning each on individually, waiting 3 seconds, and then turning the
lightoff before moving to the next one.
Just like the RPi.GPIO example, we first need to access our Python virtual environment and then execute our script:
$ workon cv $ python gpiozero_demo.py
As the script executes, we can see an image displayed to our screen and the LEDs lighting up:
Figure 5: A second example of using OpenCV to display an image and then utilizing GPIO to illuminate an LED.
Note: To execute this script as root, follow the instructions detailed in the previous section.
Summary
In this blog post, I started by reviewing what Python virtual environments are and why we use them. Simply put, Python virtual environments allow us to create independent, isolated development environments for each project we work on, ensuring we don’t run into version dependency issues. Furthermore, virtual environments allow us to keep our system install of Python clean and tidy.
Once we understood the basics of Python virtual environments, I detailed how to (correctly) install RPi.GPIO and GPIO Zero such that we can access both GPIO libraries and OpenCV at the same time.
We then developed a simple Python script that loads an image from disk using OpenCV, displays it to our screen, and then lights up various LEDs on the TrafficHAT.
In the next blog post, we’ll create a more advanced GPIO + OpenCV script, this time lighting up LEDs and buzzing the buzzer whenever a pre-defined visual action takes place.
Be sure to enter your email address in the form below to be notified when the next blog post goes live, you won’t want to miss it!
Downloads:
The post Accessing RPi.GPIO and GPIO Zero with OpenCV + Python appeared first on PyImageSearch.
from PyImageSearch http://ift.tt/1QNnA9P
via IFTTT