Latest YouTube Video
Saturday, November 18, 2017
Anonymous Radio Redux
from Google Alert - anonymous http://ift.tt/2B1Ys07
via IFTTT
Not working for anonymous user (not logged-in)
from Google Alert - anonymous http://ift.tt/2itOdu0
via IFTTT
Gold Hoarders Anonymous
from Google Alert - anonymous http://ift.tt/2hIvHAK
via IFTTT
Specifiy `--anonymous-auth=false`
from Google Alert - anonymous http://ift.tt/2zVzvnb
via IFTTT
Ravens activate RB Danny Woodhead (hamstring) from IR and release TE Gavin Escobar (ESPN)
via IFTTT
Rumor Central: Free agent P Alex Cobb drawing interest from Orioles - MLB Network (ESPN)
via IFTTT
Parents Anonymous and Child Abuse Prevention Policy
from Google Alert - anonymous http://ift.tt/2zOXBC8
via IFTTT
Friday the Moon Smiled
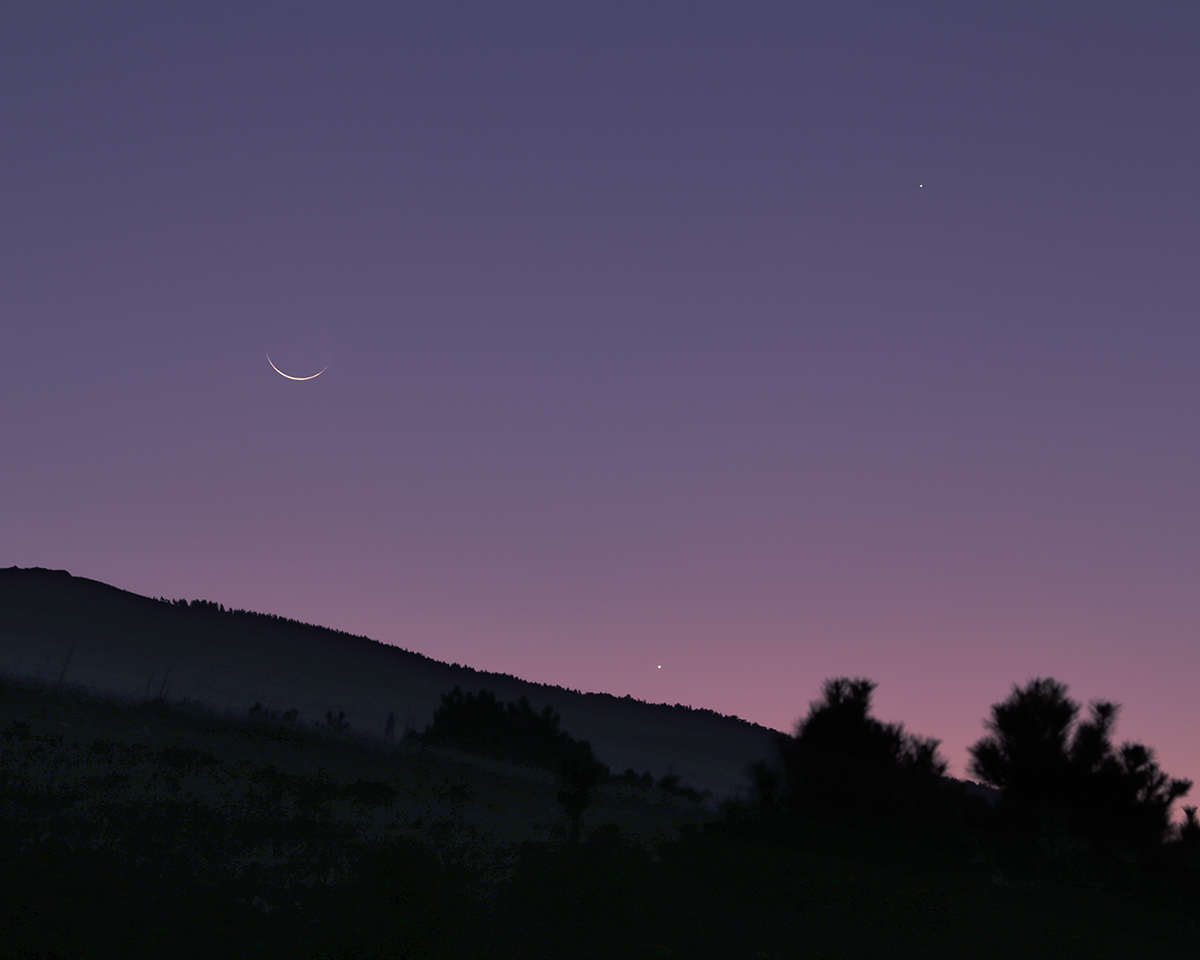
Delta Gamma Phi Elfin for Anns Place
from Google Alert - anonymous http://ift.tt/2zNDbt0
via IFTTT
Friday, November 17, 2017
Steele Of Approval To A BC Broadcasting Legend & To An Anonymous Good Samaritan
from Google Alert - anonymous http://ift.tt/2zc74E3
via IFTTT
Ravens: LT Ronnie Stanley (concussion) will likely miss Sunday vs. Packers (ESPN)
via IFTTT
Indiësche dingen
from Google Alert - anonymous http://ift.tt/2AWgelo
via IFTTT
I have a new follower on Twitter
Paula Kendal
Неy ! I'm lоoking for а seхfriend. Find mе, click https://t.co/V6LJ3clGDT
Following: 53 - Followers: 16
November 17, 2017 at 02:42PM via Twitter http://twitter.com/VictorAlcaraz
Unfair Feedback
from Google Alert - anonymous http://ift.tt/2j3XnfZ
via IFTTT
Banking Trojan Gains Ability to Steal Facebook, Twitter and Gmail Accounts
from The Hacker News http://ift.tt/2APMdTz
via IFTTT
ISS Daily Summary Report – 11/16/2017
from ISS On-Orbit Status Report http://ift.tt/2zKaE7V
via IFTTT
Kaspersky: NSA Worker's Computer Was Already Infested With Malware
from The Hacker News http://ift.tt/2APfVbj
via IFTTT
mlp/ - Anonymous in Equestria Thread #1134
from Google Alert - anonymous http://ift.tt/2AOZb3J
via IFTTT
NPS Launches Anonymous Alerts for Middle & High Schools
from Google Alert - anonymous http://ift.tt/2yPFRTn
via IFTTT
Major Fireball Meteor
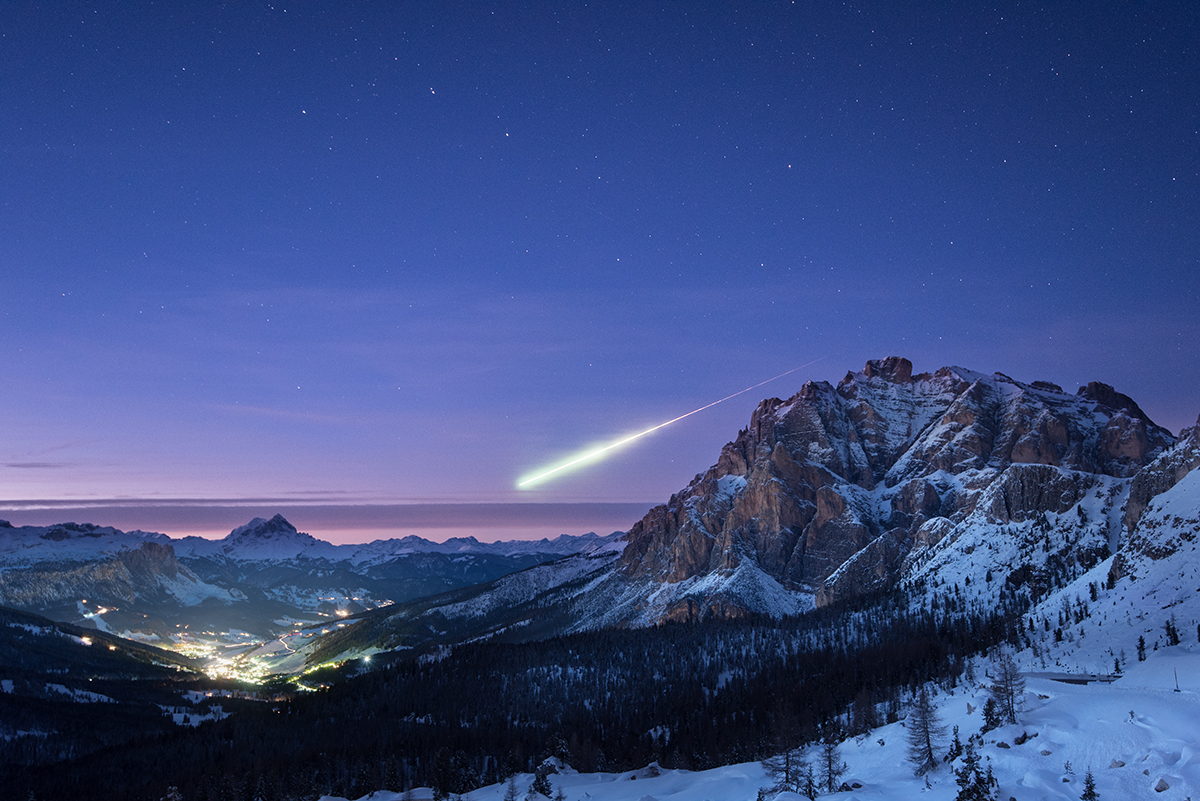
Earth: Our Living Plant
from NASA's Scientific Visualization Studio: Most Recent Items http://ift.tt/2AO5JzM
via IFTTT
Anonymous Tip
from Google Alert - anonymous http://ift.tt/2yQKywA
via IFTTT
Thursday, November 16, 2017
ISS Daily Summary Report – 11/15/2017
from ISS On-Orbit Status Report http://ift.tt/2hy8DkC
via IFTTT
Birdwatchers Anonymous
from Google Alert - anonymous http://ift.tt/2AMPYJh
via IFTTT
SBU: Most of anonymous hoax bomb calls come from Russia
from Google Alert - anonymous http://ift.tt/2inhciW
via IFTTT
What's New? | Members
from Google Alert - anonymous http://ift.tt/2AML9zH
via IFTTT
When an anonymous user and authenticated users have permission to edit translations, they can ...
from Google Alert - anonymous http://ift.tt/2in7YU1
via IFTTT
PML-N lawmakers receiving 'anonymous phone calls': Rana Sana
from Google Alert - anonymous http://ift.tt/2AKuJrJ
via IFTTT
How to hide smartdocs method while using search for anonymous users?
from Google Alert - anonymous http://ift.tt/2ioSgYB
via IFTTT
Ravens: CB Jimmy Smith (Achilles) misses 2nd straight day of practice (ESPN)
via IFTTT
I have a new follower on Twitter
US Presort
US Presort is a full-service marketing company. We provide businesses with a one-stop solution for all their #directmarketing needs.
Staten Island, US, 10314
https://t.co/yyPrq8vqKb
Following: 1023 - Followers: 1123
November 16, 2017 at 02:42PM via Twitter http://twitter.com/uspresort
Ravens: Alex Collins' role as featured back won't be affected by return of other RBs, says OC Marty Mornhinweg (ESPN)
via IFTTT
Students, parents encouraged to use school anonymous alerts
from Google Alert - anonymous http://ift.tt/2iZ8hne
via IFTTT
I have a new follower on Twitter

UK NFL Fan
Honestly, wished the NFL played more games over here :/ Go Dolphins!
Toddington, England
Following: 647 - Followers: 607
November 16, 2017 at 12:42PM via Twitter http://twitter.com/britishNFLstar
The Trial of an Ox, for Killing a Man; by Anonymous
from Google Alert - anonymous http://ift.tt/2mC3LAv
via IFTTT
Anonymous donor gives Refuge for Women a $10000 fundraising push
from Google Alert - anonymous http://ift.tt/2hElsgX
via IFTTT
Anonymous and Message
from Google Alert - anonymous http://ift.tt/2jwDoe6
via IFTTT
kubernetes/kubernetes
from Google Alert - anonymous http://ift.tt/2hDyOdj
via IFTTT
Privacy Statement
from Google Alert - anonymous http://ift.tt/2jx2GbW
via IFTTT
WHAT IS ANONYMOUS?...HOW TO BE ANONYMOUS.
from Google Alert - anonymous http://ift.tt/2yNn7UI
via IFTTT
Another Shady App Found Pre-Installed on OnePlus Phones that Collects System Logs
from The Hacker News http://ift.tt/2yMqew8
via IFTTT
Secret of the Holy Bible by Anonymous
from Google Alert - anonymous http://ift.tt/2A40uzJ
via IFTTT
Bluetooth Hack Affects 20 Million Amazon Echo and Google Home Devices
from The Hacker News http://ift.tt/2zPfhLP
via IFTTT
Accountant
from Google Alert - anonymous http://ift.tt/2hBLvFt
via IFTTT
I have a new follower on Twitter
Kimberly_Faith
Singer~Author~Attorney. Tweet to inspire God's presence! Kimberly Faith~ iTunes; https://t.co/vzQgBUC0IZ; Essential Faith~ https://t.co/LID8kZBWbl
Waynesville, Missouri
https://t.co/ez0ENIaY3u
Following: 16971 - Followers: 22714
November 16, 2017 at 01:57AM via Twitter http://twitter.com/GoFaithstrong
Secret of the Holy Bible by Anonymous: Religion & Spirituality
from Google Alert - anonymous http://ift.tt/2zGdpHl
via IFTTT
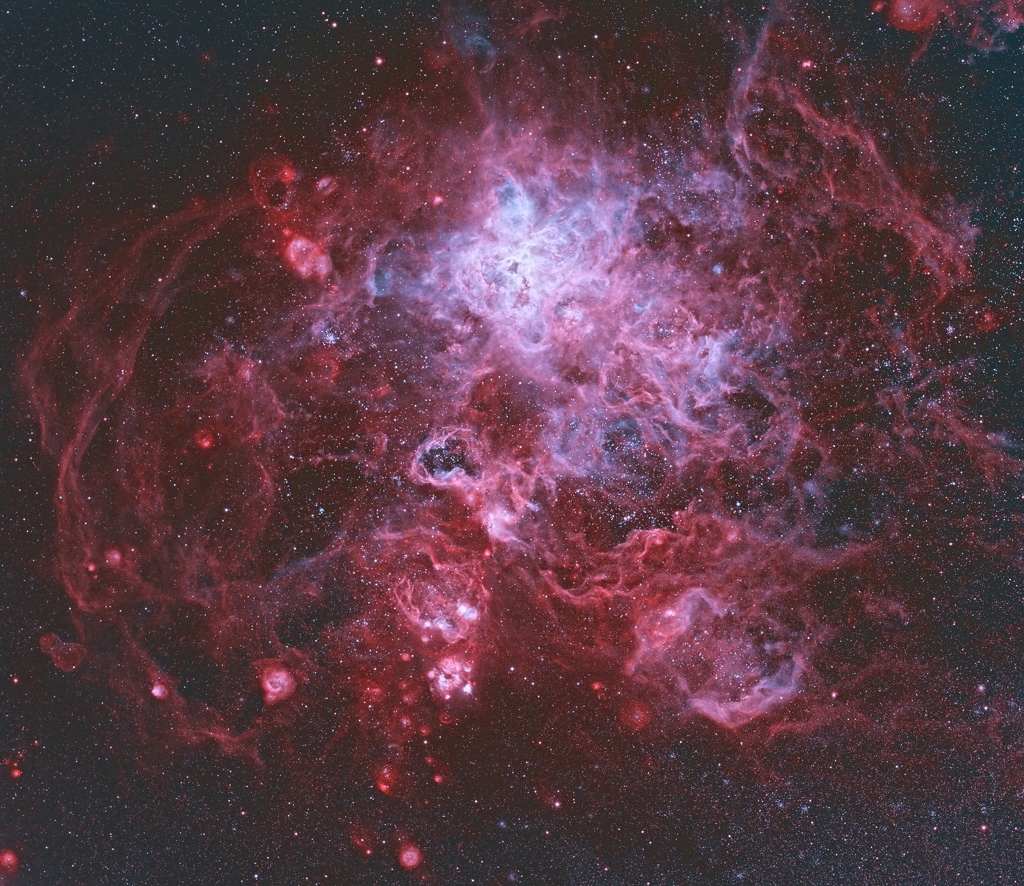
Wednesday, November 15, 2017
Startup offers anonymous harassment reporting tool
from Google Alert - anonymous http://ift.tt/2zHOPWR
via IFTTT
Rumor Central: Orioles eyeing P Tyler Chatwood in free agency - MLB Network (ESPN)
via IFTTT
COLUMN-New Weinstein lawsuits pit anonymous accusers vs public's right to know
from Google Alert - anonymous http://ift.tt/2zM26uO
via IFTTT
Dominick (Century Meeting)
from Google Alert - anonymous http://ift.tt/2jtxXfU
via IFTTT
John Harbaugh defends complexity of Ravens' offense after former TE Dennis Pitta's comments (ESPN)
via IFTTT
Gamblers Anonymous Online
from Google Alert - anonymous http://ift.tt/2hvmMio
via IFTTT
Romantics-Anonymous-Sam-Wanamaker-Playhouse-1
from Google Alert - anonymous http://ift.tt/2zJcJAE
via IFTTT
Review: Romantics Anonymous at the Sam Wanamaker Playhouse
from Google Alert - anonymous http://ift.tt/2AJEFBQ
via IFTTT
Management Accountant RARE OPPORTUNITY
from Google Alert - anonymous http://ift.tt/2iij78q
via IFTTT
Entity reference field not rendered for anonymous users
from Google Alert - anonymous http://ift.tt/2AIWfFT
via IFTTT
Fix Views support for anonymous flaggings (Unknown column 'flagging_node_field_data.sid')
from Google Alert - anonymous http://ift.tt/2hvs7X6
via IFTTT
ISS Daily Summary Report – 11/14/2017
from ISS On-Orbit Status Report http://ift.tt/2hBoD9g
via IFTTT
Anonymous DevOps Confessions
from Google Alert - anonymous http://ift.tt/2yIuM6R
via IFTTT
Cisco Training Courses: Prepare for CCNA, CCNP Networking Certifications
from The Hacker News http://ift.tt/2sU7lEU
via IFTTT
Forever 21 Warns Shoppers of Payment Card Breach at Some Stores
from The Hacker News http://ift.tt/2yFNmwb
via IFTTT
Anonymous hits out at 'EU army' with leaks in Italy
from Google Alert - anonymous http://ift.tt/2z4ZzPf
via IFTTT
17-Year-Old MS Office Flaw Lets Hackers Install Malware Without User Interaction
from The Hacker News http://ift.tt/2jsF5sE
via IFTTT
Patch Tuesday: Microsoft Releases Update to Fix 53 Vulnerabilities
from The Hacker News http://ift.tt/2jtxZ7x
via IFTTT
20 Years of Global Biosphere
from NASA's Scientific Visualization Studio: Most Recent Items http://ift.tt/2hsxiH5
via IFTTT
Anonymous Resident Wins 2 Million in Lottery
from Google Alert - anonymous http://ift.tt/2jsl45C
via IFTTT
Tuesday, November 14, 2017
Proposal to implement anonymous marking in 2017/18
from Google Alert - anonymous http://ift.tt/2zYidbR
via IFTTT
[FD] Faraday v2.7: Collaborative Penetration Test & Vulnerability Management Platform
Source: Gmail -> IFTTT-> Blogger
[FD] Advisory X41-2017-006: Multiple Vulnerabilities in PSFTPd Windows FTP Server
Source: Gmail -> IFTTT-> Blogger
Anonymous - General Manager
from Google Alert - anonymous http://ift.tt/2yCE2t8
via IFTTT
Policy Statement on Implementation of Anonymous Marking
from Google Alert - anonymous http://ift.tt/2AJyDSz
via IFTTT
Anonymous Tips
from Google Alert - anonymous http://ift.tt/2iVlRYR
via IFTTT
AA Meetings
from Google Alert - anonymous http://ift.tt/2zDvFBf
via IFTTT
Anonymous donor to match up to $100000 of ALA Annual Fund contributions
from Google Alert - anonymous http://ift.tt/2zDvPJ2
via IFTTT
Flix Anonymous - Episode 47 - CKNW Original Podcasts
from Google Alert - anonymous http://ift.tt/2jpbhx7
via IFTTT
Italy investigates Anonymous hack of state employees' emails
from Google Alert - anonymous http://ift.tt/2AFTyVB
via IFTTT
Anonymous Call Blacklist
from Google Alert - anonymous http://ift.tt/2zED5Uw
via IFTTT
Firefox 57 "Quantum" Released – 2x Faster Web Browser
from The Hacker News http://ift.tt/2AJedZN
via IFTTT
anonymous owl
from Google Alert - anonymous http://ift.tt/2yC1lmB
via IFTTT
ISS Daily Summary Report – 11/13/2017
from ISS On-Orbit Status Report http://ift.tt/2AJASoM
via IFTTT
Shop The Best Deals on All Stampers Anonymous, New Products, Y Products
from Google Alert - anonymous http://ift.tt/2iV3P9m
via IFTTT
Google Begins Removing Play Store Apps Misusing Android Accessibility Services
from The Hacker News http://ift.tt/2hsXlhC
via IFTTT
OnePlus Left A Backdoor That Allows Root Access Without Unlocking Bootloader
from The Hacker News http://ift.tt/2yYJ7jz
via IFTTT
(#40121) · Issues
from Google Alert - anonymous http://ift.tt/2iT7SD7
via IFTTT
v/ - /sa/ - Shitter's Anonymous
from Google Alert - anonymous http://ift.tt/2jm8lkS
via IFTTT
Access Denied for Anonymous
from Google Alert - anonymous http://ift.tt/2iSJHEw
via IFTTT
Ocean flows at surface and 2000 meters below sea level
from NASA's Scientific Visualization Studio: Most Recent Items http://ift.tt/2yZZBry
via IFTTT
Monday, November 13, 2017
Anonymous millennial on working at a digital shop
from Google Alert - anonymous http://ift.tt/2hrdzYu
via IFTTT
Anonymous Donor
from Google Alert - anonymous http://ift.tt/2ictwTd
via IFTTT
Orioles OF Trey Mancini finishes 3rd in AL Rookie of the Year voting (ESPN)
via IFTTT
Apple iPhone X's Face ID Hacked (Unlocked) With 3D-Printed Mask
from The Hacker News http://ift.tt/2hqi2dS
via IFTTT
ISS Daily Summary Report – 11/10/2017
from ISS On-Orbit Status Report http://ift.tt/2iaJFbO
via IFTTT
ISS Daily Summary Report – 11/09/2017
from ISS On-Orbit Status Report http://ift.tt/2ibCNuu
via IFTTT
How to install mxnet for deep learning
When it comes to deep learning, Keras is my favorite Python library…
…but a close runner up is mxnet.
What I like about mxnet is that it combines the best of both worlds in terms of performance and ease of use. Inside mxnet you’ll find:
- Caffe-like binaries to help you build efficiently packed image datasets/record files.
- A Keras-like syntax for the Python programming language to easily build deep learning models.
- Methods to train deep neural networks on multiple GPUs and scale across multiple machines.
Whenever I’m implementing a Convolutional Neural Network I tend to use Keras first. Keras is less verbose than mxnet and is often easier to implement a given neural network architecture + training procedure.
But when it’s time for me to scale up from my initial experiments to ImageNet-size datasets (or larger) I often use mxnet to (1) build an efficiently packed dataset and then (2) train my network on multiple GPUs and/or multiple machines.
Since the Python bindings to mxnet are compiled C/C++ binaries I’m able to milk every last bit of performance out of my machine(s).
In fact, we use mxnet inside Deep Learning for Computer Vision with Python (in particular, the ImageNet Bundle) when training Convolutional Neural Networks on ImageNet and replicating state-of-the-art results for seminal papers, such as VGGNet, ResNet, SqueezeNet, etc..
In the remainder of this blog post you’ll learn how to install and configure mxnet for deep learning on your Ubuntu machine.
How to install mxnet for deep learning
In today’s blog post, I’m going to show you how to get mxnet for deep learning installed on your system in just 5 (relatively) easy steps.
The mxnet deep learning package is an Apache project and comes with great community support. To get started with mxnet I would recommend the tutorials and explanations here. Given the Apache community’s dedication (not to mention, Amazon’s) to mxnet for deep learning, I think it is here to stay for the foreseeable future.
Before we proceed to install mxnet, I’d like to point out that Step #4 is broken into:
- Step #4a for CPU-only users
- And Step #4b for GPU users.
The GPU install is by far trickier and there is the potential for error. These instructions have been tested and I’m confident that they will serve as a good guide for you during the installation process.
Let’s get started.
Step #1: Install prerequisites
First, you’ll want to make sure that your Ubuntu 16.04 or 14.04 system is up to date. You can execute the following commands to update packages from the Ubuntu repository:
$ sudo apt-get update $ sudo apt-get upgrade
Next, let’s install some development tools, image/video I/O, GUI operations, and other packages (not all of these are 100% necessary, but you’ll want them installed if you’re doing work in deep learning or machine learning):
$ sudo apt-get install build-essential cmake git unzip pkg-config $ sudo apt-get install libjpeg-dev libtiff5-dev libjasper-dev libpng12-dev $ sudo apt-get install libavcodec-dev libavformat-dev libswscale-dev libv4l-dev $ sudo apt-get install libxvidcore-dev libx264-dev $ sudo apt-get install libgtk-3-dev $ sudo apt-get install libhdf5-serial-dev graphviz $ sudo apt-get install libopenblas-dev libatlas-base-dev gfortran $ sudo apt-get install python-tk python3-tk python-imaging-tk
Third, let’s install Python header files:
$ sudo apt-get install python2.7-dev python3-dev
Now that we have the proper system prerequisites installed, let’s move on.
Step #2: Set up a virtual environment
Virtual environments are critical to Python development and are a standard practice. Python virtual environments allow developers to created multiple, isolated development environments on a single machine. You could even use Python virtual environments to install two different versions of a package from the Python Package Index or another source.
I highly encourage you to use virtual environments for deep learning. If you aren’t convinced, then take a look at this RealPython article on why Python virtual environments are a best practice.
For the purposes of the rest of this install guide, we’ll create a Python virtual environment named
dl4cv— this is the name of virtual environment used inside my book, Deep Learning for Computer Vision with Python. I chose the name
dl4cvto keep naming consistent across my books/blog posts. You can use a different name if you so wish.
First we’ll install PIP, a Python package manager. Then we’ll install Python virtual environments and a handy wrapper tool. Subsequently we’ll create a virtual environment and be on our way.
Let’s install
pip:
$ wget http://ift.tt/1mn7OFn $ sudo python get-pip.py $ sudo python3 get-pip.py
Then we’ll need to pip-install the two Python virtual environment libraries we’ll be using:
$ sudo pip install virtualenv virtualenvwrapper $ sudo rm -rf ~/.cache/pip get-pip.py
Now let’s update our
~/.bashrcfile to include the following lines at the bottom of the file:
# virtualenv and virtualenvwrapper export WORKON_HOME=$HOME/.virtualenvs export VIRTUALENVWRAPPER_PYTHON=/usr/bin/python3 source /usr/local/bin/virtualenvwrapper.sh
Figure 1: Editing your ~/.bashrc
file with virtualenvwrapper
settings using nano terminal text editor.
Now that
~/.bashrchas been changed, we need to reload it:
$ source ~/.bashrc
You’ll see a few messages indicating that
virtualenvwrapperhas configured itself on your system.
Creating the dl4cv virtual environment
For the purposes of my deep learning book we use Python 3, so let’s create a Python 3 environment on our system named
dl4cv. This environment will house relevant packages to deep learning and computer vision, particularly mxnet.
$ mkvirtualenv dl4cv -p python3
Each time you want to create a new virtual environment, simply supply a name and the Python version you’d like to use. It’s as simple as that. Today we just need the one environment, so let’s move on.
How do I know that I’m in the right environment or in an environment at all?
If you ever deactivate your virtual environment or restart your machine, you’ll need access your Python virtual environment before you resume your work.
To do this, simply use the
workoncommand:
$ workon dl4cv
In this case I’ve supplied the name of my environment,
dl4cv, but you would want to specify the name of the environment you desire to work with.
To verify that you’re in the environment you’ll see
(dl4cv)before the bash prompt as is shown in the image below:
Figure 2: Using the workon dl4cv
command we can activate the dl4cv
virtual environment. Similarly we can use the deactivate
command to exit the environment.
To exit your environment, simply deactivate it:
$ deactivate
And then you’ll see that the
(dl4cv)has been removed from the beginning of the bash prompt as in Figure 2.
Step #3: Install OpenCV into the dl4cv virtual environment
In this section we will install OpenCV into the dl4cv virtual environment. First we’ll download and unzip OpenCV 3.3. Then we will build and compile OpenCV from source. Finally we will test that OpenCV has been installed.
Install NumPy
First we’ll install NumPy into the virtual environment:
$ workon dl4cv $ pip install numpy
Download OpenCV
Next let’s download opencv and opencv_contrib into your home directory:
$ cd ~ $ wget -O opencv.zip http://ift.tt/2zyMxJh $ wget -O opencv_contrib.zip http://ift.tt/2zUnn8H
You will need to expand the commands above (using the “<=>” button) to copy and past the full path to the
opencv_contribURL.
Then, let’s unzip both files:
$ unzip opencv.zip $ unzip opencv_contrib.zip
Running CMake
Let’s create a
builddirectory and run CMake:
$ cd ~/opencv-3.3.1/ $ mkdir build $ cd build $ cmake -D CMAKE_BUILD_TYPE=RELEASE \ -D CMAKE_INSTALL_PREFIX=/usr/local \ -D WITH_CUDA=OFF \ -D INSTALL_PYTHON_EXAMPLES=ON \ -D OPENCV_EXTRA_MODULES_PATH=~/opencv_contrib-3.3.1/modules \ -D BUILD_EXAMPLES=ON ..
For CMake, it is important that your flags match mine for compatibility. Also, make sure that your
opencv_contribversion is identical to the OpenCV version you downloaded (in this case version
3.3.1). If the versions do not match then your compile will fail.
Before we move on to the actual compilation step make sure you examine the output of CMake!
Start by scrolling to the section titled
Python 3.
Make sure that your Python 3 section looks like the figure below:
You’ll want to ensure that the Interpreter points to our
python3.5binary located in the
dl4cvvirtual environment while
numpypoints to our NumPy install.
In either case if you do not see the
dl4cvvirtual environment in these variables’ paths, then it’s almost certainly because you are NOT in the
dl4cvvirtual environment prior to running CMake!
If this is the case, access the
dl4cvvirtual environment using
workon dl4cvand re-run the command outlined above (I would also suggest deleting the
builddirectory, re-creating it, and running CMake again).
Compiling OpenCV
Now we are now ready to compile OpenCV. Assuming that your
cmakecommand exited without error, ensure you are in the
builddirectory and execute the following command:
$ make -j4
Note: The
-jflag specifies the number of processor cores to use for the compile. In this case I used
-j4since my machine has four cores. If you run into compilation errors, you may run the command
make cleanand then just compile without the parallel flag:
make.
From there, all you need to do is to install OpenCV 3.3 and then free up some disk space if you so desire:
$ sudo make install $ sudo ldconfig $ cd ~ $ rm -rf opencv-3.3.0 opencv.zip $ rm -rf opencv_contrib-3.3.1 opencv_contrib.zip
Symbolic linking OpenCV to your virtual environment
To sym-link our OpenCV bindings into the
dl4cvvirtual environment, issue the following commands:
$ cd ~/.virtualenvs/dl4cv/lib/python3.5/site-packages/ $ ln -s /usr/local/lib/python3.5/site-packages/cv2.cpython-35m-x86_64-linux-gnu.so cv2.so $ cd ~
Note: Again, make sure you use the “<=>” button in the toolbar above to expand the code block to grab the full
lncommand (you don’t want to forget the
cv2.sofile!)
Notice that I am using Python 3.5 in this example. If you are using Python 3.6 (or newer) you’ll want to update the paths above to use your specific Python version.
Secondly, your
.sofile (i.e., the actual OpenCV bindings) may be some variant of what is shown above, so be sure to use the appropriate file by double-checking the path.
Testing your OpenCV 3.3 install
Now that we’ve got OpenCV 3.3 installed and linked, let’s do a quick sanity test to see if things work:
$ workon dl4cv $ python >>> import cv2 >>> cv2.__version__ '3.3.1'
Make sure you are in the
dl4cvvirtual environment before firing up Python (
workon dl4cv). When you print out the version, it should match the version of OpenCV that you installed (in our case, OpenCV
3.3.1).
That’s it — assuming you didn’t encounter an import error, you’re ready to go on to Step #4 where we will install mxnet.
Step #4
Follow the appropriate instructions for your system:
- Step #4.a: CPU-only mode
- Step #4.b: GPU mode
Step #4.a: Install mxnet for CPU-only mode
If you have a GPU machine and want to utilize your GPU(s) for deep learning with mxnet, then you should skip this step and proceed to Step #4.b — this section is intended for CPU-only usage.
Let’s clone the mxnet repository and checkout branch
0.11.0— a branch tested for use with my book, Deep Learning for Computer Vision with Python:
$ cd ~ $ git clone --recursive http://ift.tt/2wjU0qU mxnet --branch 0.11.0
Then we can compile mxnet:
$ cd mxnet $ make -j4 \ USE_OPENCV=1 \ USE_BLAS=openblas
Finally, we need to sym-link mxnet to our dl4cv environment:
$ cd ~/.virtualenvs/dl4cv/lib/python3.5/site-packages/ $ ln -s ~/mxnet/python/mxnet mxnet $ cd ~
Note: Be sure not to delete the mxnet directory in your home folder. Our Python bindings live there and we’ll also need the files in
~/mxnet/binfor creating serialized image datasets.
Step #4.b: Install mxnet for GPU mode
This step is only for GPU users. If you do not have a GPU on your machine please refer to the CPU-only instructions above.
First, we need to prepare our system to swap out the default drivers with NVIDIA CUDA drivers:
$ sudo apt-get install linux-image-generic linux-image-extra-virtual $ sudo apt-get install linux-source linux-headers-generic
We’re now going to install the CUDA Toolkit. This part of the installation requires that you pay attention to all instructions and beware of system warnings and errors.
First, disable the Nouveau kernel driver by creating a new file:
$ sudo nano /etc/modprobe.d/blacklist-nouveau.conf
Then add the following lines to the file, followed by saving + exiting:
blacklist nouveau blacklist lbm-nouveau options nouveau modeset=0 alias nouveau off alias lbm-nouveau off
Your screen should look like this if you are using
nano, but feel free to use oter terminal text editors:
Figure 4: The blacklist_noveau.conf
file has been created prior to installing mxnet for deep learning with GPU capability.
Don’t forget this key step where we update the initial RAM filesystem and reboot the machine:
$ echo options nouveau modeset=0 | sudo tee -a /etc/modprobe.d/nouveau-kms.conf $ sudo update-initramfs -u $ sudo reboot
If you are connected via SSH, your session will end and you’ll have to wait a short time before reconnecting.
Install CUDA
Now let’s grab the CUDA Toolkit v8.0 from the NVIDIA CUDA Toolkit website:
You should then select the appropriate download for your system. I’m assuming that you are using Ubuntu 16.04, so your browser should look like this:
Notice how I have selected
Linux => x86_64 => Ubuntu => 16.04 runfile (local).
From that screen, download the
-runfile which should have a filename of
cuda_8.0.61_375.26_linux-runor similar.
To do this, simply right click to copy the download link and use
wgetback in your terminal download the file:
wget http://ift.tt/2mtsbcZ
Important: At the time of this writing there is a minor discrepancy on the NVIDIA website. As shown in Figure 5 under the “Base Installer” download, the filename (as is written) ends with
.run. The actual downloadable file ends with
-run. You should be good to go in copying my
wget+ URL command for now unless NVIDIA changes the filename again.
Note: You will need to click the “<=>” button in the code block toolbar above to expand the code block. This will enable you to copy the full URL to the
-runfile.
From there all you need to do is unpack the
-runfile:
$ chmod +x cuda_8.0.61_375.26_linux-run $ mkdir installers $ sudo ./cuda_8.0.61_375.26_linux-run -extract=`pwd`/installers
Executing the
-runscript can take about a minute.
Now let’s install the NVIDIA kernel driver:
$ cd installers $ sudo ./NVIDIA-Linux-x86_64-375.26.run
You’ll need to follow the prompts on the screen during this step, one of which is to accept the EULA.
Then we can add the NVIDIA loadable kernel module to the Linux kernel:
$ modprobe nvidia
And finally, install the CUDA toolkit and examples:
$ sudo ./cuda-linux64-rel-8.0.61-21551265.run $ sudo ./cuda-samples-linux-8.0.61-21551265.run
You will need to accept licenses and follow prompts again. When it asks you to specify installation paths, you can press <enter> to accept the defaults.
Now that the NVIDIA CUDA driver and tools are installed, let’s update
~/.bashrcto include the CUDA Toolkit using nano:
$ nano ~/.bashrc
Append these lines to the end of the file:
# NVIDIA CUDA Toolkit export PATH=/usr/local/cuda-8.0/bin:$PATH export LD_LIBRARY_PATH=/usr/local/cuda-8.0/lib64/
Next, reload the
~/.bashrcand test the CUDA Toolkit installation by compiling + running the
deviceQueryexample program:
$ source ~/.bashrc $ cd /usr/local/cuda-8.0/samples/1_Utilities/deviceQuery $ sudo make $ ./deviceQuery deviceQuery, CUDA Driver = CUDART, CUDA Driver Version = 8.0, CUDA Runtime Version = 8.0, NumDevs = 1, Device0 = Tesla K80 Result = PASS
From here, if you have a
Result = PASS, then we’re ready to install cuDNN.
Install cuDNN
For this step, you will need to Create a free account with NVIDIA and download cuDNN.
For this tutorial be sure to download cuDNN v6.0 for Linux which is what TensorFlow requires (presuming you want to install TensorFlow on your deep learning machine alongside mxnet).
NVIDIA requires authentication to access the download, therefore you won’t be able to use
wgetto download the file.
If you’re on a local machine you can simply download the cuDNN archive via your web browser.
However, if you are on a remote machine (i.e., SSH’ing into a machine) you’ll want to first download the file to your local machine and then use
scpto transfer the file (while replacing
usernameand
your_ip_addresswith your appropriate values, of course):
$ scp -i EC2KeyPair.pem ~/Downloads/cudnn-8.0-linux-x64-v6.0.tgz \ username@your_ip_address:~
Now that the file is on your remote GPU machine (EC2 in my case), untar the file and then copy the resulting files into
lib64and
includerespectively, using the
-Pswitch to preserve sym-links:
$ cd ~ $ tar -zxf cudnn-8.0-linux-x64-v6.0.tgz $ cd cuda $ sudo cp -P lib64/* /usr/local/cuda/lib64/ $ sudo cp -P include/* /usr/local/cuda/include/ $ cd ~
That’s it for installing cuDNN — this step was rather easy so as long as you preserved sym-links, you should be good to go.
Install mxnet with CUDA
Let’s clone the mxnet repository and checkout branch
0.11.0which has been tested for Deep Learning for Computer Vision with Python:
$ cd ~ $ git clone --recursive http://ift.tt/2wjU0qU mxnet --branch 0.11.0
Then we can compile mxnet:
$ cd mxnet $ make -j4 \ USE_OPENCV=1 \ USE_BLAS=openblas \ USE_CUDA=1 \ USE_CUDA_PATH=/usr/local/cuda \ USE_CUDNN=1
And finally we need to sym-link mxnet to our
dl4cvenvironment:
$ cd ~/.virtualenvs/dl4cv/lib/python3.5/site-packages/ $ ln -s ~/mxnet/python/mxnet mxnet $ cd ~
Note: Be sure not to delete the mxnet directory in your home folder. Our Python bindings live there and we’ll also need the files in
~/mxnet/binfor creating serialized image datasets.
Step #5: Validating install
The last step is to test if mxnet has been properly installed:
$ workon dl4cv $ python >>> import mxnet >>>
If mxnet imports without errors, then congratulations — you have successfully installed mxnet for deep learning.
Where to from here?
Congratulations on getting mxnet installed on your system!
But now that you have mxnet installed, what are the next steps?
How do you…
- …train your first Convolutional Neural Network?
- …utilize multiple-GPUs to dramatically reduce training time?
- …replicate state-of-the-art results from seminal deep learning papers (including ResNet, SqueezeNet, AlexNet, and others) on the challenging ImageNet dataset?
If you’re interested in taking the next step on your journey to deep learning mastery, I’m confident you’d really enjoy my new book, Deep Learning for Computer Vision with Python.
Just click on the link above to take a look.
And while you’re at it, be sure to grab your (FREE) table of contents + sample chapters PDF:
In particular, I recommend you take a look at Chapter 5 of the ImageNet Bundle (one of the free sample chapters) where I demonstrate how to train AlexNet on the ImageNet dataset using mxnet.
In the AlexNet chapter, I:
- Provide a thorough discussion of the AlexNet architecture.
- Implement the AlexNet architecture from scratch (and explain each line of code ensuring you know exactly what is going on under the hood).
- Include detailed experiments discussing how I tune the parameters of AlexNet to achieve higher accuracy experiment after experiment.
- Obtain 59.80% rank-1 and 81.75% rank-5 accuracy on ImageNet (which is higher accuracy than current independent papers and publications).
You’ve got nothing to lose — there are no strings attached to the free download of the entire table of contents of my 800+ page book.
To claim your free table of contents + sample chapters, simply head to the Deep Learning for Computer Vision with Python page now.
Summary
In today’s blog post you learned how to install mxnet for deep learning on your Ubuntu machine for both CPU only and GPU-based training.
Once you start using mxnet you’ll find that it:
- Includes Caffe-like binaries to help you build efficiently backed image record files (which will save you a lot of disk space).
- Provides a Keras-like API to building deep neural networks (although mxnet is certainly more verbose than Keras).
Now that you have your deep learning environment configured, I suggest you take the next step and check out my brand new book, Deep Learning for Computer Vision with Python.
Inside the book you’ll start by learning the fundamentals of deep learning and then graduate to more advanced content, including training networks on the challenging ImageNet dataset from scratch.
You’ll also find my personal blueprint/best practices that I use to determine which deep learning techniques to apply when confronted with a new problem.
To learn more about Deep Learning for Computer Vision with Python, just click here.
Otherwise, be sure to enter your email address in the form below to be notified when new blog posts are published here on PyImageSearch.
The post How to install mxnet for deep learning appeared first on PyImageSearch.
from PyImageSearch http://ift.tt/2zABpMh
via IFTTT
Anonymous Recruiter
from Google Alert - anonymous http://ift.tt/2iSvZ4S
via IFTTT
Topic: UltraFilms 17 11 12 Belle Claire Anonymous sex!ual Relations
from Google Alert - anonymous http://ift.tt/2iQrqYL
via IFTTT
anonymous item pings being abused
from Google Alert - anonymous http://ift.tt/2movkNB
via IFTTT
How to send an anonymous email bomb
from Google Alert - anonymous http://ift.tt/2yWrZuM
via IFTTT
Sunday, November 12, 2017
Integral2 and anonymous function in a matrix
from Google Alert - anonymous http://ift.tt/2zwk0UX
via IFTTT
Post as an Anonymous User
from Google Alert - anonymous http://ift.tt/2yUk94U
via IFTTT
Anonymous Boh
from Google Alert - anonymous http://ift.tt/2zwwWdc
via IFTTT
A Happy Sky over Los Angeles
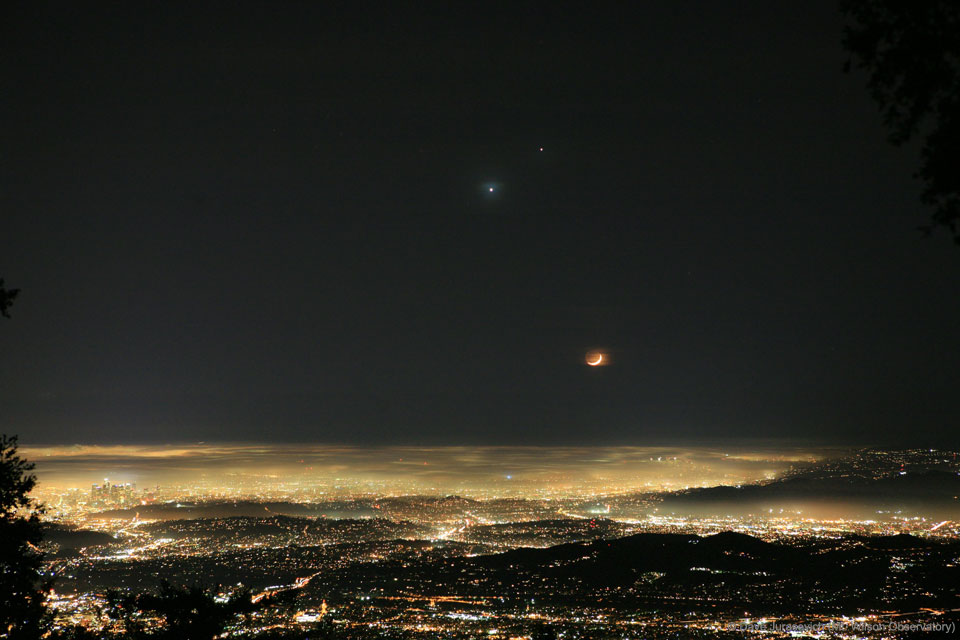