Latest YouTube Video
Saturday, June 11, 2016
Ravens: Breshad Perriman suffered partial left knee ACL tear - Schefter, Hensley; may need surgery, will get 2nd opinion (ESPN)
via IFTTT
NSA wants to Exploit Internet of Things and Biomedical Devices
from The Hacker News http://ift.tt/1toLg15
via IFTTT
Anonymous EP
from Google Alert - anonymous http://ift.tt/1toF5dp
via IFTTT
Anonymous bidder pays $3.46 million for Warren Buffett lunch
from Google Alert - anonymous http://ift.tt/25TSms6
via IFTTT
Anonymous bidder wins $3456789 lunch with Warren Buffett
from Google Alert - anonymous http://ift.tt/1YhDaSM
via IFTTT
Anonymous to Thomas Jefferson, April 18, 1803
from Google Alert - anonymous http://ift.tt/1UJzCTU
via IFTTT
Lichess.org
from Google Alert - anonymous http://ift.tt/1UJ3TSR
via IFTTT
NGC 6888: The Crescent Nebula
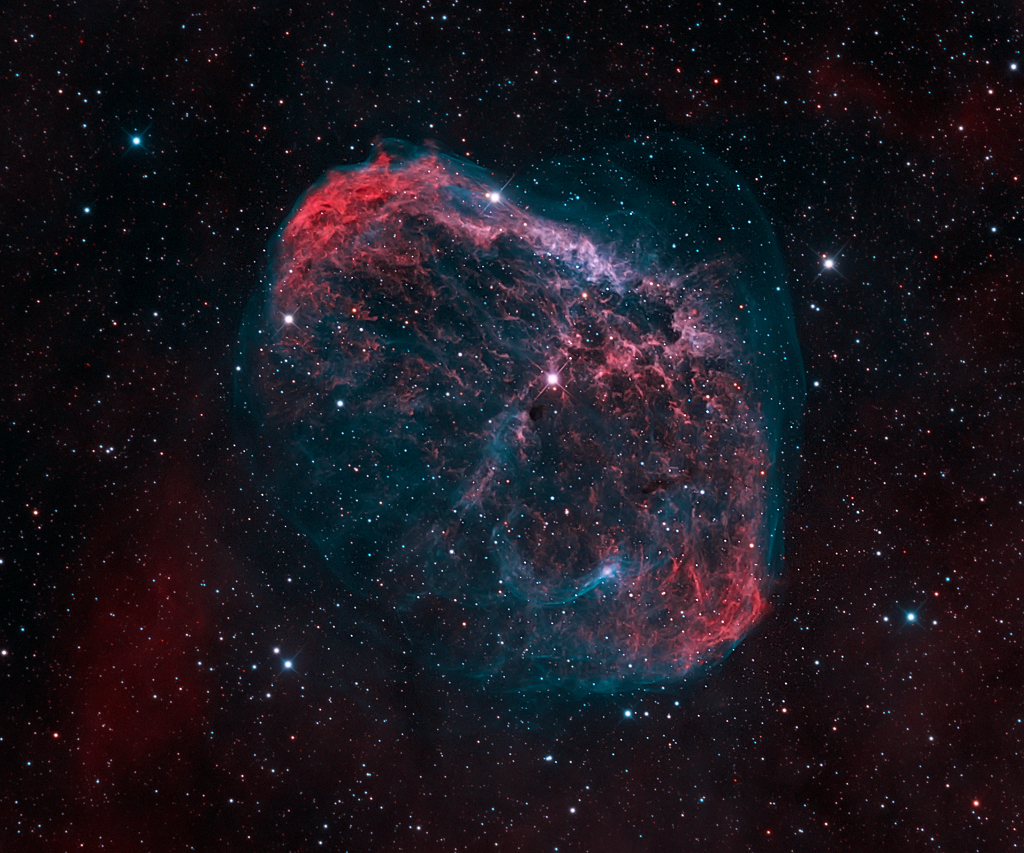
Friday, June 10, 2016
Orioles select NC State 1B Preson Palmeiro in MLB draft's 7th round (No. 211); son of Rafael Palmeiro (569 career HRs) (ESPN)
via IFTTT
Anonymous Hamburger Society
from Google Alert - anonymous http://ift.tt/1UHQUAO
via IFTTT
anonymous user see login form when post a video
from Google Alert - anonymous http://ift.tt/1VQLoBf
via IFTTT
Concerto for Flute, 2 Violins and Basso (Anonymous)
from Google Alert - anonymous http://ift.tt/1TYS4IV
via IFTTT
I have a new follower on Twitter
Tomasz Motyl
Chief Innovation Officer at @AliorBankSA, @AliorLab. #innovation, #banking, #FinTech, #DisruptiveInnovation https://t.co/3wXPpn5qpo
Poland, Krakow
https://t.co/TI69ClDOlc
Following: 2621 - Followers: 2702
June 10, 2016 at 02:09AM via Twitter http://twitter.com/tomekmot
Pluto at Night
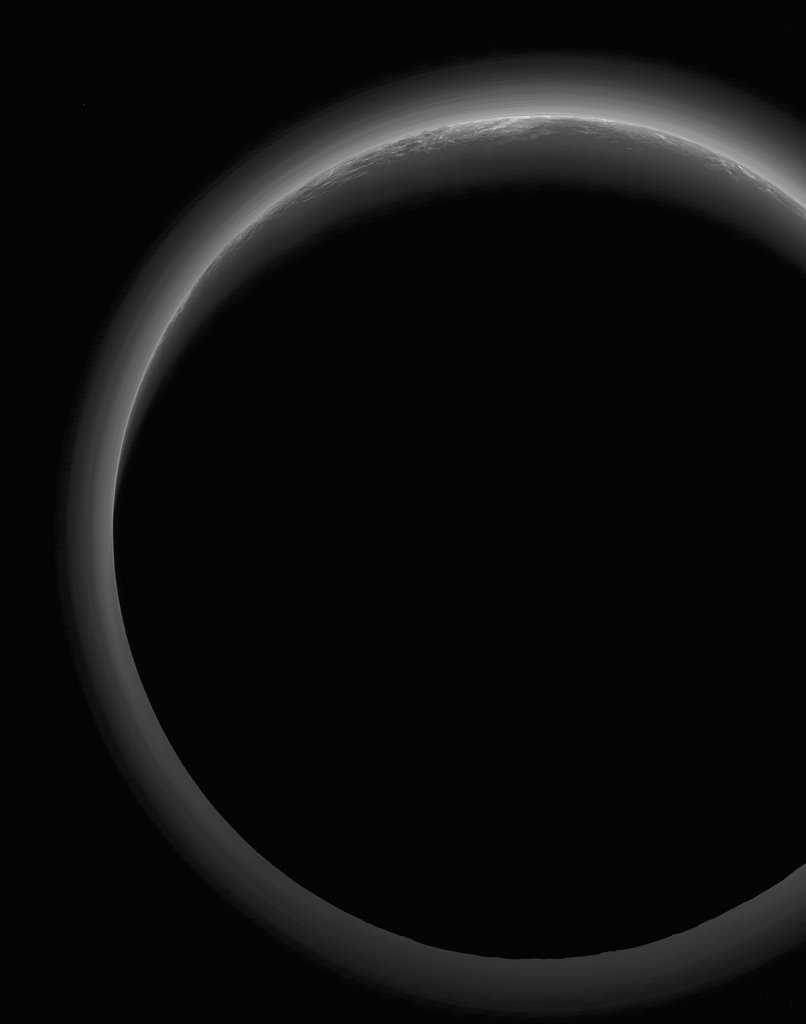
Thursday, June 9, 2016
Anonymous user issues uploading files since the release of Drupal 7.43
from Google Alert - anonymous http://ift.tt/1XJRPYw
via IFTTT
I have a new follower on Twitter
Tri-Force Consulting
IT Experts with Excellence, Integrity and Customization, Three forces above the competition
http://t.co/Am3ZVMhK5U
Following: 1360 - Followers: 1382
June 09, 2016 at 11:25PM via Twitter http://twitter.com/triforceinc
Orioles Video: Chris Davis belts game-tying HR, adds sac fly in 9th inning of 6-5 victory over the Blue Jays (ESPN)
via IFTTT
MLB Draft: Orioles select Illinois RHP Cody Sedlock No. 27 overall; full player info in the ESPN App (ESPN)
via IFTTT
A Modified Vortex Search Algorithm for Numerical Function Optimization. (arXiv:1606.02710v1 [cs.AI])
The Vortex Search (VS) algorithm is one of the recently proposed metaheuristic algorithms which was inspired from the vortical flow of the stirred fluids. Although the VS algorithm is shown to be a good candidate for the solution of certain optimization problems, it also has some drawbacks. In the VS algorithm, candidate solutions are generated around the current best solution by using a Gaussian distribution at each iteration pass. This provides simplicity to the algorithm but it also leads to some problems along. Especially, for the functions those have a number of local minimum points, to select a single point to generate candidate solutions leads the algorithm to being trapped into a local minimum point. Due to the adaptive step-size adjustment scheme used in the VS algorithm, the locality of the created candidate solutions is increased at each iteration pass. Therefore, if the algorithm cannot escape a local point as quickly as possible, it becomes much more difficult for the algorithm to escape from that point in the latter iterations. In this study, a modified Vortex Search algorithm (MVS) is proposed to overcome above mentioned drawback of the existing VS algorithm. In the MVS algorithm, the candidate solutions are generated around a number of points at each iteration pass. Computational results showed that with the help of this modification the global search ability of the existing VS algorithm is improved and the MVS algorithm outperformed the existing VS algorithm, PSO2011 and ABC algorithms for the benchmark numerical function set.
from cs.AI updates on arXiv.org http://ift.tt/1Uq5fCg
via IFTTT
Theoretical Robopsychology: Samu Has Learned Turing Machines. (arXiv:1606.02767v1 [cs.AI])
From the point of view of a programmer, the robopsychology is a synonym for the activity is done by developers to implement their machine learning applications. This robopsychological approach raises some fundamental theoretical questions of machine learning. Our discussion of these questions is constrained to Turing machines. Alan Turing had given an algorithm (aka the Turing Machine) to describe algorithms. If it has been applied to describe itself then this brings us to Turing's notion of the universal machine. In the present paper, we investigate algorithms to write algorithms. From a pedagogy point of view, this way of writing programs can be considered as a combination of learning by listening and learning by doing due to it is based on applying agent technology and machine learning. As the main result we introduce the problem of learning and then we show that it cannot easily be handled in reality therefore it is reasonable to use machine learning algorithm for learning Turing machines.
from cs.AI updates on arXiv.org http://ift.tt/1tlzaWs
via IFTTT
Face valuing: Training user interfaces with facial expressions and reinforcement learning. (arXiv:1606.02807v1 [cs.HC])
An important application of interactive machine learning is extending or amplifying the cognitive and physical capabilities of a human. To accomplish this, machines need to learn about their human users' intentions and adapt to their preferences. In most current research, a user has conveyed preferences to a machine using explicit corrective or instructive feedback; explicit feedback imposes a cognitive load on the user and is expensive in terms of human effort. The primary objective of the current work is to demonstrate that a learning agent can reduce the amount of explicit feedback required for adapting to the user's preferences pertaining to a task by learning to perceive a value of its behavior from the human user, particularly from the user's facial expressions---we call this face valuing. We empirically evaluate face valuing on a grip selection task. Our preliminary results suggest that an agent can quickly adapt to a user's changing preferences with minimal explicit feedback by learning a value function that maps facial features extracted from a camera image to expected future reward. We believe that an agent learning to perceive a value from the body language of its human user is complementary to existing interactive machine learning approaches and will help in creating successful human-machine interactive applications.
from cs.AI updates on arXiv.org http://ift.tt/24E7SWo
via IFTTT
Arbitrage-Free Combinatorial Market Making via Integer Programming. (arXiv:1606.02825v1 [cs.GT])
We present a new combinatorial market maker that operates arbitrage-free combinatorial prediction markets specified by integer programs. Although the problem of arbitrage-free pricing, while maintaining a bound on the subsidy provided by the market maker, is #P-hard in the worst case, we posit that the typical case might be amenable to modern integer programming (IP) solvers. At the crux of our method is the Frank-Wolfe (conditional gradient) algorithm which is used to implement a Bregman projection aligned with the market maker's cost function, using an IP solver as an oracle. We demonstrate the tractability and improved accuracy of our approach on real-world prediction market data from combinatorial bets placed on the 2010 NCAA Men's Division I Basketball Tournament, where the outcome space is of size $2^{63}$. To our knowledge, this is the first implementation and empirical evaluation of an arbitrage-free combinatorial prediction market on this scale.
from cs.AI updates on arXiv.org http://ift.tt/1sxQp67
via IFTTT
e-Commerce product classification: our participation at cDiscount 2015 challenge. (arXiv:1606.02854v1 [cs.LG])
This report describes our participation in the cDiscount 2015 challenge where the goal was to classify product items in a predefined taxonomy of products. Our best submission yielded an accuracy score of 64.20\% in the private part of the leaderboard and we were ranked 10th out of 175 participating teams. We followed a text classification approach employing mainly linear models. The final solution was a weighted voting system which combined a variety of trained models.
from cs.AI updates on arXiv.org http://ift.tt/1Uq5KMH
via IFTTT
A Thorough Examination of the CNN/Daily Mail Reading Comprehension Task. (arXiv:1606.02858v1 [cs.CL])
Enabling a computer to understand a document so that it can answer comprehension questions is a central, yet unsolved goal of NLP. A key factor impeding its solution by machine learned systems is the limited availability of human-annotated data. Hermann et al. (2015) seek to solve this problem by creating over a million training examples by pairing CNN and Daily Mail news articles with their summarized bullet points, and show that a neural network can then be trained to give good performance on this task. In this paper, we conduct a thorough examination of this new reading comprehension task. Our primary aim is to understand what depth of language understanding is required to do well on this task. We approach this from one side by doing a careful hand-analysis of a small subset of the problems and from the other by showing that simple, carefully designed systems can obtain accuracies of 72.4% and 75.8% on these two datasets, exceeding current state-of-the-art results by over 5% and approaching what we believe is the ceiling for performance on this task.
from cs.AI updates on arXiv.org http://ift.tt/1WHiog8
via IFTTT
Understanding User Instructions by Utilizing Open Knowledge for Service Robots. (arXiv:1606.02877v1 [cs.RO])
Understanding user instructions in natural language is an active research topic in AI and robotics. Typically, natural user instructions are high-level and can be reduced into low-level tasks expressed in common verbs (e.g., `take', `get', `put'). For robots understanding such instructions, one of the key challenges is to process high-level user instructions and achieve the specified tasks with robots' primitive actions. To address this, we propose novel algorithms by utilizing semantic roles of common verbs defined in semantic dictionaries and integrating multiple open knowledge to generate task plans. Specifically, we present a new method for matching and recovering semantics of user instructions and a novel task planner that exploits functional knowledge of robot's action model. To verify and evaluate our approach, we implemented a prototype system using knowledge from several open resources. Experiments on our system confirmed the correctness and efficiency of our algorithms. Notably, our system has been deployed in the KeJia robot, which participated the annual RoboCup@Home competitions in the past three years and achieved encouragingly high scores in the benchmark tests.
from cs.AI updates on arXiv.org http://ift.tt/1sxRBq6
via IFTTT
A Cognitive Architecture for the Implementation of Emotions in Computing Systems. (arXiv:1606.02899v1 [cs.AI])
In this paper we present a new neurobiologically-inspired affective cognitive architecture: NEUCOGAR (NEUromodulating COGnitive ARchitecture). The objective of NEUCOGAR is the identification of a mapping from the influence of serotonin, dopamine and noradrenaline to the computing processes based on Von Neuman's architecture, in order to implement affective phenomena which can operate on the Turing's machine model. As basis of the modeling we use and extend the L\"ovheim Cube of Emotion with parameters of the Von Neumann architecture. Validation is conducted via simulation on a computing system of dopamine neuromodulation and its effects on the Cortex. In the experimental phase of the project, the increase of computing power and storage redistribution due to emotion stimulus modulated by the dopamine system, confirmed the soundness of the model.
from cs.AI updates on arXiv.org http://ift.tt/1Uq5gWQ
via IFTTT
Generative Topic Embedding: a Continuous Representation of Documents (Extended Version with Proofs). (arXiv:1606.02979v1 [cs.CL])
Word embedding maps words into a low-dimensional continuous embedding space by exploiting the local word collocation patterns in a small context window. On the other hand, topic modeling maps documents onto a low-dimensional topic space, by utilizing the global word collocation patterns in the same document. These two types of patterns are complementary. In this paper, we propose a generative topic embedding model to combine the two types of patterns. In our model, topics are represented by embedding vectors, and are shared across documents. The probability of each word is influenced by both its local context and its topic. A variational inference method yields the topic embeddings as well as the topic mixing proportions for each document. Jointly they represent the document in a low-dimensional continuous space. In two document classification tasks, our method performs better than eight existing methods, with fewer features. In addition, we illustrate with an example that our method can generate coherent topics even based on only one document.
from cs.AI updates on arXiv.org http://ift.tt/1Uq4Yz3
via IFTTT
MuFuRU: The Multi-Function Recurrent Unit. (arXiv:1606.03002v1 [cs.NE])
Recurrent neural networks such as the GRU and LSTM found wide adoption in natural language processing and achieve state-of-the-art results for many tasks. These models are characterized by a memory state that can be written to and read from by applying gated composition operations to the current input and the previous state. However, they only cover a small subset of potentially useful compositions. We propose Multi-Function Recurrent Units (MuFuRUs) that allow for arbitrary differentiable functions as composition operations. Furthermore, MuFuRUs allow for an input- and state-dependent choice of these composition operations that is learned. Our experiments demonstrate that the additional functionality helps in different sequence modeling tasks, including the evaluation of propositional logic formulae, language modeling and sentiment analysis.
from cs.AI updates on arXiv.org http://ift.tt/1WHhEaX
via IFTTT
The "Horse'' Inside: Seeking Causes Behind the Behaviours of Music Content Analysis Systems. (arXiv:1606.03044v1 [cs.SD])
Building systems that possess the sensitivity and intelligence to identify and describe high-level attributes in music audio signals continues to be an elusive goal, but one that surely has broad and deep implications for a wide variety of applications. Hundreds of papers have so far been published toward this goal, and great progress appears to have been made. Some systems produce remarkable accuracies at recognising high-level semantic concepts, such as music style, genre and mood. However, it might be that these numbers do not mean what they seem. In this paper, we take a state-of-the-art music content analysis system and investigate what causes it to achieve exceptionally high performance in a benchmark music audio dataset. We dissect the system to understand its operation, determine its sensitivities and limitations, and predict the kinds of knowledge it could and could not possess about music. We perform a series of experiments to illuminate what the system has actually learned to do, and to what extent it is performing the intended music listening task. Our results demonstrate how the initial manifestation of music intelligence in this state-of-the-art can be deceptive. Our work provides constructive directions toward developing music content analysis systems that can address the music information and creation needs of real-world users.
from cs.AI updates on arXiv.org http://ift.tt/1sxRg6J
via IFTTT
Context-Aware Bandits. (arXiv:1510.03164v3 [cs.LG] UPDATED)
We propose an efficient Context-Aware clustering of Bandits (CAB) algorithm, which can capture collaborative effects. CAB can be easily deployed in a real-world recommendation system, where multi-armed bandits have been shown to perform well in particular with respect to the cold-start problem. CAB utilizes a context-aware clustering augmented by exploration-exploitation strategies. CAB dynamically clusters the users based on the content universe under consideration. We give a theoretical analysis in the standard stochastic multi-armed bandits setting. We show the efficiency of our approach on production and real-world datasets, demonstrate the scalability, and, more importantly, the significant increased prediction performance against several state-of-the-art methods.
from cs.AI updates on arXiv.org http://ift.tt/1ZwXM9W
via IFTTT
Building and Measuring Privacy-Preserving Predictive Blacklists. (arXiv:1512.04114v3 [cs.CR] UPDATED)
Collaborative approaches to network defense are increasingly used to predict attacks as well as to speed up their detection. For instance, with highly predictive blacklisting, one aims to forecast attack sources based on alerts contributed by multiple organizations. While collaboration helps discover groups of correlated attacks targeting similar victims, it also raises important privacy concerns.
To address this challenge, we introduce a novel privacy-friendly system whereby organizations are clustered together based on the similarity of their logs, without disclosing them in the clear. Entities in the same cluster only share relevant logs and build more accurate blacklists. At the same time, we investigate how to measure the effect of collaboration on prediction and find that the state-of-the-art (non privacy-preserving) system actually achieves lower accuracy than if organizations predicted based on local alerts only. Our experiments shed light on how to improve the quality of predictions by optimizing information shared across organizations, showing that our privacy-friendly methods markedly outperform non private tools both in terms of precision and recall.
from cs.AI updates on arXiv.org http://ift.tt/1NtM7Aq
via IFTTT
Decoy Bandits Dueling on a Poset. (arXiv:1602.02706v2 [cs.LG] UPDATED)
We adress the problem of dueling bandits defined on partially ordered sets, or posets. In this setting, arms may not be comparable, and there may be several (incomparable) optimal arms. We propose an algorithm, UnchainedBandits, that efficiently finds the set of optimal arms of any poset even when pairs of comparable arms cannot be distinguished from pairs of incomparable arms, with a set of minimal assumptions. This algorithm relies on the concept of decoys, which stems from social psychology. For the easier case where the incomparability information may be accessible, we propose a second algorithm, SlicingBandits, which takes advantage of this information and achieves a very significant gain of performance compared to UnchainedBandits. We provide theoretical guarantees and experimental evaluation for both algorithms.
from cs.AI updates on arXiv.org http://ift.tt/1Q4lqCJ
via IFTTT
Filter based Taxonomy Modification for Improving Hierarchical Classification. (arXiv:1603.00772v2 [cs.AI] UPDATED)
Large scale classification of data organized as a hierarchy of classes has received significant attention in the literature. Top-Down (TD) Hierarchical Classification (HC), which exploits the hierarchical structure during the learning process is an effective method for dealing with problems at scale due to its computational benefits. However, its accuracy suffers due to error propagation i.e., prediction errors made at higher levels in the hierarchy cannot be corrected at lower levels. One of the main reasons behind errors at the higher levels is the presence of inconsistent nodes and links that are introduced due to the arbitrary process of creating these hierarchies by domain experts. In this paper, we propose two efficient data driven filter based approaches for hierarchical structure modification: (i) Flattening (local and global) approach that identifies and removes inconsistent nodes present within the hierarchy and (ii) Rewiring approach modifies parent-child relationships to improve the classification performance of learned models. Our extensive empirical evaluation of the proposed approaches on several image and text datasets shows improved performance over competing approaches.
from cs.AI updates on arXiv.org http://ift.tt/1Rqezo1
via IFTTT
Generating Natural Questions About an Image. (arXiv:1603.06059v3 [cs.CL] UPDATED)
There has been an explosion of work in the vision & language community during the past few years from image captioning to video transcription, and answering questions about images. These tasks have focused on literal descriptions of the image. To move beyond the literal, we choose to explore how questions about an image are often directed at commonsense inference and the abstract events evoked by objects in the image. In this paper, we introduce the novel task of Visual Question Generation (VQG), where the system is tasked with asking a natural and engaging question when shown an image. We provide three datasets which cover a variety of images from object-centric to event-centric, with considerably more abstract training data than provided to state-of-the-art captioning systems thus far. We train and test several generative and retrieval models to tackle the task of VQG. Evaluation results show that while such models ask reasonable questions for a variety of images, there is still a wide gap with human performance which motivates further work on connecting images with commonsense knowledge and pragmatics. Our proposed task offers a new challenge to the community which we hope furthers interest in exploring deeper connections between vision & language.
from cs.AI updates on arXiv.org http://ift.tt/1Rgxm5T
via IFTTT
On the Theory and Practice of Privacy-Preserving Bayesian Data Analysis. (arXiv:1603.07294v2 [cs.LG] UPDATED)
Bayesian inference has great promise for the privacy-preserving analysis of sensitive data, as posterior sampling automatically preserves differential privacy, an algorithmic notion of data privacy, under certain conditions (Dimitrakakis et al., 2014; Wang et al., 2015). While this one posterior sample (OPS) approach elegantly provides privacy "for free," it is data inefficient in the sense of asymptotic relative efficiency (ARE). We show that a simple alternative based on the Laplace mechanism, the workhorse of differential privacy, is as asymptotically efficient as non-private posterior inference, under general assumptions. This technique also has practical advantages including efficient use of the privacy budget for MCMC. We demonstrate the practicality of our approach on a time-series analysis of sensitive military records from the Afghanistan and Iraq wars disclosed by the Wikileaks organization.
from cs.AI updates on arXiv.org http://ift.tt/1SinXNv
via IFTTT
Audio Event Detection using Weakly Labeled Data. (arXiv:1605.02401v2 [cs.SD] UPDATED)
Acoustic event detection is essential for content analysis and description of multimedia recordings. The majority of current literature on the topic learns the detectors through fully-supervised techniques employing strongly labeled data. However, the labels available for online multimedia data are generally weak and do not provide sufficient detail for such methods to be employed. In this paper we propose a framework for learning acoustic event detectors using only weakly labeled data based on a Multiple Instance Learning (MIL) framework. We first show that audio event detection using weak data can be formulated as an MIL problem. We then suggest two frameworks for solving multiple-instance learning, one based on neural networks, and the second on support vector machines. The proposed methods can help in removing the time consuming and expensive process of manually annotating data to facilitate fully supervised learning. Our proposed framework can not only successfully detect events in a recording but can also provide temporal locations of events in the recording. This is interesting as these information were never known in the first place for weakly labeled data.
from cs.AI updates on arXiv.org http://ift.tt/1s8SANF
via IFTTT
Unsupervised Learning for Physical Interaction through Video Prediction. (arXiv:1605.07157v3 [cs.LG] UPDATED)
A core challenge for an agent learning to interact with the world is to predict how its actions affect objects in its environment. Many existing methods for learning the dynamics of physical interactions require labeled object information. However, to scale real-world interaction learning to a variety of scenes and objects, acquiring labeled data becomes increasingly impractical. To learn about physical object motion without labels, we develop an action-conditioned video prediction model that explicitly models pixel motion, by predicting a distribution over pixel motion from previous frames. Because our model explicitly predicts motion, it is partially invariant to object appearance, enabling it to generalize to previously unseen objects. To explore video prediction for real-world interactive agents, we also introduce a dataset of 50,000 robot interactions involving pushing motions, including a test set with novel objects. In this dataset, accurate prediction of videos conditioned on the robot's future actions amounts to learning a "visual imagination" of different futures based on different courses of action. Our experiments show that our proposed method not only produces more accurate video predictions, but also more accurately predicts object motion, when compared to prior methods.
from cs.AI updates on arXiv.org http://ift.tt/1Ua9BQ7
via IFTTT
MLB: Orioles 3B Manny Machado suspended 4 games, fined $2,500 by league for role in bench-clearing brawl - reports (ESPN)
via IFTTT
Atixnet Anonymous Edit
from Google Alert - anonymous http://ift.tt/1UiBrvf
via IFTTT
ISS Daily Summary Report – 06/08/16
from ISS On-Orbit Status Report http://ift.tt/1tcYHQN
via IFTTT
Microsoft creates its own FreeBSD VM Image for Azure Cloud Computing Platform
from The Hacker News http://ift.tt/1TXrhN7
via IFTTT
Warning! 32 Million Twitter Passwords May Have Been Hacked and Leaked
from The Hacker News http://ift.tt/219Jqvk
via IFTTT
The Horsehead Nebula in Infrared from Hubble
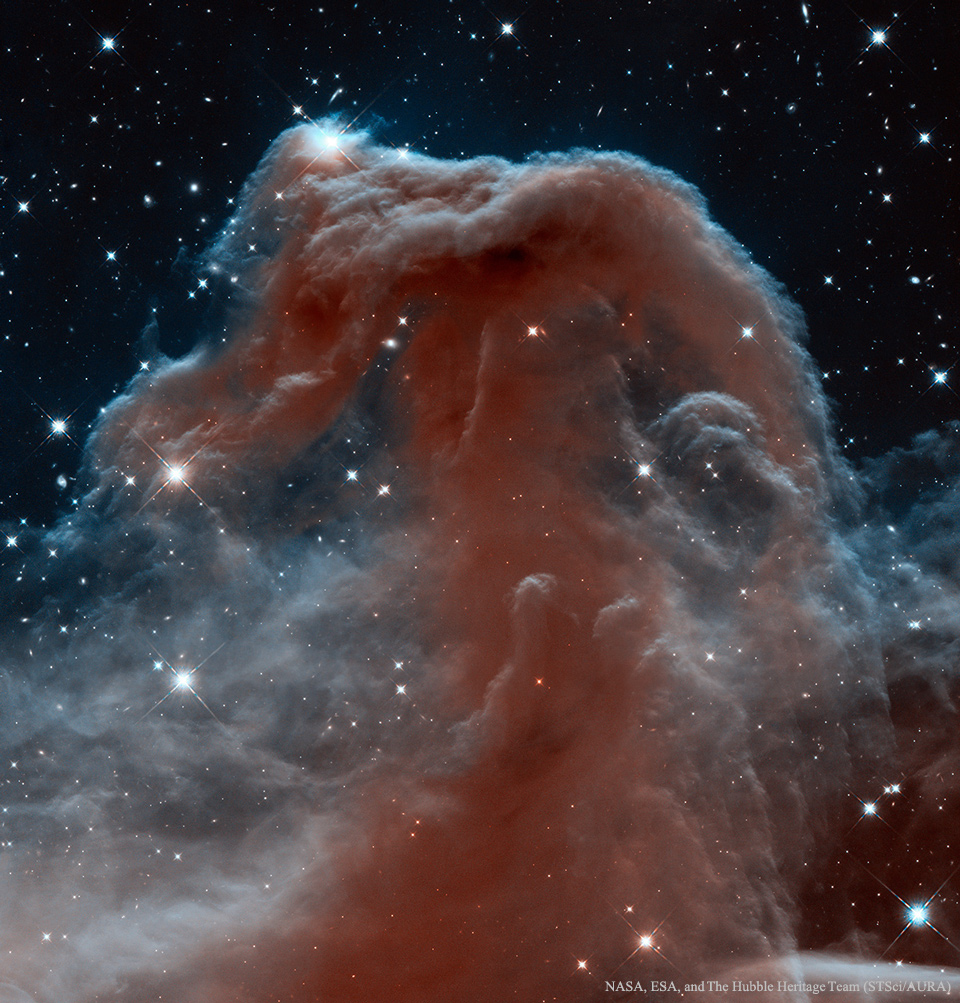
Wednesday, June 8, 2016
Orioles Video: Chris Tillman strikes out 9 over 7.1 innings in 4-0 victory over Royals to complete sweep (ESPN)
via IFTTT
All-Europe Young People in Alcoholics Anonymous Convention 2016
from Google Alert - anonymous http://ift.tt/1tbd7RG
via IFTTT
Active Long Term Memory Networks. (arXiv:1606.02355v1 [cs.LG])
Continual Learning in artificial neural networks suffers from interference and forgetting when different tasks are learned sequentially. This paper introduces the Active Long Term Memory Networks (A-LTM), a model of sequential multi-task deep learning that is able to maintain previously learned association between sensory input and behavioral output while acquiring knew knowledge. A-LTM exploits the non-convex nature of deep neural networks and actively maintains knowledge of previously learned, inactive tasks using a distillation loss. Distortions of the learned input-output map are penalized but hidden layers are free to transverse towards new local optima that are more favorable for the multi-task objective. We re-frame the McClelland's seminal Hippocampal theory with respect to Catastrophic Inference (CI) behavior exhibited by modern deep architectures trained with back-propagation and inhomogeneous sampling of latent factors across epochs. We present empirical results of non-trivial CI during continual learning in Deep Linear Networks trained on the same task, in Convolutional Neural Networks when the task shifts from predicting semantic to graphical factors and during domain adaptation from simple to complex environments. We present results of the A-LTM model's ability to maintain viewpoint recognition learned in the highly controlled iLab-20M dataset with 10 object categories and 88 camera viewpoints, while adapting to the unstructured domain of Imagenet with 1,000 object categories.
from cs.AI updates on arXiv.org http://ift.tt/1XFXe32
via IFTTT
SE3-Nets: Learning Rigid Body Motion using Deep Neural Networks. (arXiv:1606.02378v1 [cs.LG])
We introduce SE3-Nets, which are deep networks designed to model rigid body motion from raw point cloud data. Based only on pairs of depth images along with an action vector and point wise data associations, SE3-Nets learn to segment effected object parts and predict their motion resulting from the applied force. Rather than learning point wise flow vectors, SE3-Nets predict SE3 transformations for different parts of the scene. Using simulated depth data of a table top scene and a robot manipulator, we show that the structure underlying SE3-Nets enables them to generate a far more consistent prediction of object motion than traditional flow based networks.
from cs.AI updates on arXiv.org http://ift.tt/1U86VCZ
via IFTTT
Deep Learning Convolutional Networks for Multiphoton Microscopy Vasculature Segmentation. (arXiv:1606.02382v1 [cs.CV])
Recently there has been an increasing trend to use deep learning frameworks for both 2D consumer images and for 3D medical images. However, there has been little effort to use deep frameworks for volumetric vascular segmentation. We wanted to address this by providing a freely available dataset of 12 annotated two-photon vasculature microscopy stacks. We demonstrated the use of deep learning framework consisting both 2D and 3D convolutional filters (ConvNet). Our hybrid 2D-3D architecture produced promising segmentation result. We derived the architectures from Lee et al. who used the ZNN framework initially designed for electron microscope image segmentation. We hope that by sharing our volumetric vasculature datasets, we will inspire other researchers to experiment with vasculature dataset and improve the used network architectures.
from cs.AI updates on arXiv.org http://ift.tt/1XFWQl4
via IFTTT
Deep Successor Reinforcement Learning. (arXiv:1606.02396v1 [stat.ML])
Learning robust value functions given raw observations and rewards is now possible with model-free and model-based deep reinforcement learning algorithms. There is a third alternative, called Successor Representations (SR), which decomposes the value function into two components -- a reward predictor and a successor map. The successor map represents the expected future state occupancy from any given state and the reward predictor maps states to scalar rewards. The value function of a state can be computed as the inner product between the successor map and the reward weights. In this paper, we present DSR, which generalizes SR within an end-to-end deep reinforcement learning framework. DSR has several appealing properties including: increased sensitivity to distal reward changes due to factorization of reward and world dynamics, and the ability to extract bottleneck states (subgoals) given successor maps trained under a random policy. We show the efficacy of our approach on two diverse environments given raw pixel observations -- simple grid-world domains (MazeBase) and the Doom game engine.
from cs.AI updates on arXiv.org http://ift.tt/1XFWZVI
via IFTTT
Structured Convolution Matrices for Energy-efficient Deep learning. (arXiv:1606.02407v1 [cs.NE])
We derive a relationship between network representation in energy-efficient neuromorphic architectures and block Toplitz convolutional matrices. Inspired by this connection, we develop deep convolutional networks using a family of structured convolutional matrices and achieve state-of-the-art trade-off between energy efficiency and classification accuracy for well-known image recognition tasks. We also put forward a novel method to train binary convolutional networks by utilising an existing connection between noisy-rectified linear units and binary activations.
from cs.AI updates on arXiv.org http://ift.tt/1U86Xe1
via IFTTT
Learning Language Games through Interaction. (arXiv:1606.02447v1 [cs.CL])
We introduce a new language learning setting relevant to building adaptive natural language interfaces. It is inspired by Wittgenstein's language games: a human wishes to accomplish some task (e.g., achieving a certain configuration of blocks), but can only communicate with a computer, who performs the actual actions (e.g., removing all red blocks). The computer initially knows nothing about language and therefore must learn it from scratch through interaction, while the human adapts to the computer's capabilities. We created a game in a blocks world and collected interactions from 100 people playing it. First, we analyze the humans' strategies, showing that using compositionality and avoiding synonyms correlates positively with task performance. Second, we compare computer strategies, showing how to quickly learn a semantic parsing model from scratch, and that modeling pragmatics further accelerates learning for successful players.
from cs.AI updates on arXiv.org http://ift.tt/1XFXhff
via IFTTT
Exploring Implicit Human Responses to Robot Mistakes in a Learning from Demonstration Task. (arXiv:1606.02485v1 [cs.RO])
As robots enter human environments, they will be expected to accomplish a tremendous range of tasks. It is not feasible for robot designers to pre-program these behaviors or know them in advance, so one way to address this is through end-user programming, such as via learning from demonstration (LfD). While significant work has been done on the mechanics of enabling robot learning from human teachers, one unexplored aspect is enabling mutual feedback between both the human teacher and robot during the learning process, i.e., implicit learning. In this paper, we explore one aspect of this mutual understanding, grounding sequences, where both a human and robot provide non-verbal feedback to signify their mutual understanding during interaction. We conducted a study where people taught an autonomous humanoid robot a dance, and performed gesture analysis to measure people's responses to the robot during correct and incorrect demonstrations.
from cs.AI updates on arXiv.org http://ift.tt/1XFY9jX
via IFTTT
Symbolic Music Data Version 1.0. (arXiv:1606.02542v1 [cs.SD])
In this document, we introduce a new dataset designed for training machine learning models of symbolic music data. Five datasets are provided, one of which is from a newly collected corpus of 20K midi files. We describe our preprocessing and cleaning pipeline, which includes the exclusion of a number of files based on scores from a previously developed probabilistic machine learning model. We also define training, testing and validation splits for the new dataset, based on a clustering scheme which we also describe. Some simple histograms are included.
from cs.AI updates on arXiv.org http://ift.tt/1U87htd
via IFTTT
DISCO Nets: DISsimilarity COefficient Networks. (arXiv:1606.02556v1 [cs.CV])
We present a new type of probabilistic model which we call DISsimilarity COefficient Networks (DISCO Nets). DISCO Nets allow us to efficiently sample from a posterior distribution parametrised by a neural network. During training, DISCO Nets are learned by minimising the dissimilarity coefficient between the true distribution and the estimated distribution. This allows us to tailor the training to the loss related to the task at hand. We empirically show that (i) by modeling uncertainty on the output value, DISCO Nets outperform equivalent non-probabilistic predictive networks and (ii) DISCO Nets accurately model the uncertainty of the output, outperforming existing probabilistic models based on deep neural networks.
from cs.AI updates on arXiv.org http://ift.tt/1XFXfnp
via IFTTT
Towards End-to-End Learning for Dialog State Tracking and Management using Deep Reinforcement Learning. (arXiv:1606.02560v1 [cs.AI])
This paper presents an end-to-end framework for task-oriented dialog systems using a variant of Deep Recurrent Q-Networks (DRQN). The model is able to interface with a relational database and jointly learn policies for both language understanding and dialog strategy. Moreover, we propose a hybrid algorithm that combines the strength of reinforcement learning and supervised learning to achieve faster learning speed. We evaluated the proposed model on a 20 Question Game conversational game simulator. Results show that the proposed method outperforms the modular-based baseline and learns a distributed representation of the latent dialog state.
from cs.AI updates on arXiv.org http://ift.tt/1rdPnej
via IFTTT
DialPort: Connecting the Spoken Dialog Research Community to Real User Data. (arXiv:1606.02562v1 [cs.AI])
This paper describes a new spoken dialog portal that connects systems produced by the spoken dialog academic research community and gives them access to real users. We introduce a distributed, multi-modal, multi-agent prototype dialog framework that affords easy integration with various remote resources, ranging from end-to-end dialog systems to external knowledge APIs. To date, the DialPort portal has successfully connected to the multi-domain spoken dialog system at Cambridge University, the NOAA (National Oceanic and Atmospheric Administration) weather API and the Yelp API.
from cs.AI updates on arXiv.org http://ift.tt/1U87WuX
via IFTTT
Convolution by Evolution: Differentiable Pattern Producing Networks. (arXiv:1606.02580v1 [cs.NE])
In this work we introduce a differentiable version of the Compositional Pattern Producing Network, called the DPPN. Unlike a standard CPPN, the topology of a DPPN is evolved but the weights are learned. A Lamarckian algorithm, that combines evolution and learning, produces DPPNs to reconstruct an image. Our main result is that DPPNs can be evolved/trained to compress the weights of a denoising autoencoder from 157684 to roughly 200 parameters, while achieving a reconstruction accuracy comparable to a fully connected network with more than two orders of magnitude more parameters. The regularization ability of the DPPN allows it to rediscover (approximate) convolutional network architectures embedded within a fully connected architecture. Such convolutional architectures are the current state of the art for many computer vision applications, so it is satisfying that DPPNs are capable of discovering this structure rather than having to build it in by design. DPPNs exhibit better generalization when tested on the Omniglot dataset after being trained on MNIST, than directly encoded fully connected autoencoders. DPPNs are therefore a new framework for integrating learning and evolution.
from cs.AI updates on arXiv.org http://ift.tt/1XFXa33
via IFTTT
The Dark Side of Ethical Robots. (arXiv:1606.02583v1 [cs.RO])
Concerns over the risks associated with advances in Artificial Intelligence have prompted calls for greater efforts toward robust and beneficial AI, including machine ethics. Recently, roboticists have responded by initiating the development of so-called ethical robots. These robots would, ideally, evaluate the consequences of their actions and morally justify their choices. This emerging field promises to develop extensively over the next years. However, in this paper, we point out an inherent limitation of the emerging field of ethical robots. We show that building ethical robots also necessarily facilitates the construction of unethical robots. In three experiments, we show that it is remarkably easy to modify an ethical robot so that it behaves competitively, or even aggressively. The reason for this is that the specific AI, required to make an ethical robot, can always be exploited to make unethical robots. Hence, the development of ethical robots will not guarantee the responsible deployment of AI. While advocating for ethical robots, we conclude that preventing the misuse of robots is beyond the scope of engineering, and requires instead governance frameworks underpinned by legislation. Without this, the development of ethical robots will serve to increase the risks of robotic malpractice instead of diminishing it.
from cs.AI updates on arXiv.org http://ift.tt/1U87FIp
via IFTTT
Simplified Boardgames. (arXiv:1606.02645v1 [cs.AI])
We formalize Simplified Boardgames language, which describes a subclass of arbitrary board games. The language structure is based on the regular expressions, which makes the rules easily machine-processable while keeping the rules concise and fairly human-readable.
from cs.AI updates on arXiv.org http://ift.tt/1XFX0sK
via IFTTT
Safe and Efficient Off-Policy Reinforcement Learning. (arXiv:1606.02647v1 [cs.LG])
In this work, we take a fresh look at some old and new algorithms for off-policy, return-based reinforcement learning. Expressing these in a common form, we derive a novel algorithm, Retrace($\lambda$), with three desired properties: (1) low variance; (2) safety, as it safely uses samples collected from any behaviour policy, whatever its degree of "off-policyness"; and (3) efficiency, as it makes the best use of samples collected from near on-policy behaviour policies. We analyse the contractive nature of the related operator under both off-policy policy evaluation and control settings and derive online sample-based algorithms. To our knowledge, this is the first return-based off-policy control algorithm converging a.s. to $Q^*$ without the GLIE assumption (Greedy in the Limit with Infinite Exploration). As a corollary, we prove the convergence of Watkins' Q($\lambda$), which was still an open problem. We illustrate the benefits of Retrace($\lambda$) on a standard suite of Atari 2600 games.
from cs.AI updates on arXiv.org http://ift.tt/1U86Uis
via IFTTT
Deep Reinforcement Learning with a Natural Language Action Space. (arXiv:1511.04636v5 [cs.AI] UPDATED)
This paper introduces a novel architecture for reinforcement learning with deep neural networks designed to handle state and action spaces characterized by natural language, as found in text-based games. Termed a deep reinforcement relevance network (DRRN), the architecture represents action and state spaces with separate embedding vectors, which are combined with an interaction function to approximate the Q-function in reinforcement learning. We evaluate the DRRN on two popular text games, showing superior performance over other deep Q-learning architectures. Experiments with paraphrased action descriptions show that the model is extracting meaning rather than simply memorizing strings of text.
from cs.AI updates on arXiv.org http://ift.tt/1MiWHMd
via IFTTT
Hand Pose Estimation through Semi-Supervised and Weakly-Supervised Learning. (arXiv:1511.06728v2 [cs.CV] UPDATED)
We propose a method for hand pose estimation based on a deep regressor trained on two different kinds of input. Raw depth data is fused with an intermediate representation in the form of a segmentation of the hand into parts. This intermediate representation contains important topological information and provides useful cues for reasoning about joint locations. The mapping from raw depth to segmentation maps is learned in a semi/weakly-supervised way from two different datasets: (i) a synthetic dataset created through a rendering pipeline including densely labeled ground truth (pixelwise segmentations); and (ii) a dataset with real images for which ground truth joint positions are available, but not dense segmentations. Loss for training on real images is generated from a patch-wise restoration process, which aligns tentative segmentation maps with a large dictionary of synthetic poses. The underlying premise is that the domain shift between synthetic and real data is smaller in the intermediate representation, where labels carry geometric and topological meaning, than in the raw input domain. Experiments on the NYU dataset show that the proposed training method decreases error on joints over direct regression of joints from depth data by 15.7%.
from cs.AI updates on arXiv.org http://ift.tt/1MwAdas
via IFTTT
Iterative Hierarchical Optimization for Misspecified Problems (IHOMP). (arXiv:1602.03348v2 [cs.LG] UPDATED)
For complex, high-dimensional Markov Decision Processes (MDPs), it may be necessary to represent the policy with function approximation. A problem is misspecified whenever, the representation cannot express any policy with acceptable performance. We introduce IHOMP : an approach for solving misspecified problems. IHOMP iteratively learns a set of context specialized options and combines these options to solve an otherwise misspecified problem. Our main contribution is proving that IHOMP enjoys theoretical convergence guarantees. In addition, we extend IHOMP to exploit Option Interruption (OI) enabling it to decide where the learned options can be reused. Our experiments demonstrate that IHOMP can find near-optimal solutions to otherwise misspecified problems and that OI can further improve the solutions.
from cs.AI updates on arXiv.org http://ift.tt/1KcDiiK
via IFTTT
Unbounded Human Learning: Optimal Scheduling for Spaced Repetition. (arXiv:1602.07032v2 [cs.AI] UPDATED)
In the study of human learning, there is broad evidence that our ability to retain information improves with repeated exposure and decays with delay since last exposure. This plays a crucial role in the design of educational software, leading to a trade-off between teaching new material and reviewing what has already been taught. A common way to balance this trade-off is spaced repetition, which uses periodic review of content to improve long-term retention. Though spaced repetition is widely used in practice, e.g., in electronic flashcard software, there is little formal understanding of the design of these systems. Our paper addresses this gap in three ways. First, we mine log data from spaced repetition software to establish the functional dependence of retention on reinforcement and delay. Second, we use this memory model to develop a stochastic model for spaced repetition systems. We propose a queueing network model of the Leitner system for reviewing flashcards, along with a heuristic approximation that admits a tractable optimization problem for review scheduling. Finally, we empirically evaluate our queueing model through a Mechanical Turk experiment, verifying a key qualitative prediction of our model: the existence of a sharp phase transition in learning outcomes upon increasing the rate of new item introductions.
from cs.AI updates on arXiv.org http://ift.tt/1RlTjT0
via IFTTT
Incorporating Copying Mechanism in Sequence-to-Sequence Learning. (arXiv:1603.06393v3 [cs.CL] UPDATED)
We address an important problem in sequence-to-sequence (Seq2Seq) learning referred to as copying, in which certain segments in the input sequence are selectively replicated in the output sequence. A similar phenomenon is observable in human language communication. For example, humans tend to repeat entity names or even long phrases in conversation. The challenge with regard to copying in Seq2Seq is that new machinery is needed to decide when to perform the operation. In this paper, we incorporate copying into neural network-based Seq2Seq learning and propose a new model called CopyNet with encoder-decoder structure. CopyNet can nicely integrate the regular way of word generation in the decoder with the new copying mechanism which can choose sub-sequences in the input sequence and put them at proper places in the output sequence. Our empirical study on both synthetic data sets and real world data sets demonstrates the efficacy of CopyNet. For example, CopyNet can outperform regular RNN-based model with remarkable margins on text summarization tasks.
from cs.AI updates on arXiv.org http://ift.tt/1S1WM6Q
via IFTTT
Classifying Options for Deep Reinforcement Learning. (arXiv:1604.08153v2 [cs.LG] UPDATED)
In this paper we combine one method for hierarchical reinforcement learning - the options framework - with deep Q-networks (DQNs) through the use of different "option heads" on the policy network, and a supervisory network for choosing between the different options. We utilise our setup to investigate the effects of architectural constraints in subtasks with positive and negative transfer, across a range of network capacities. We empirically show that our augmented DQN has lower sample complexity when simultaneously learning subtasks with negative transfer, without degrading performance when learning subtasks with positive transfer.
from cs.AI updates on arXiv.org http://ift.tt/1TeXHBL
via IFTTT
Learning Representations for Counterfactual Inference. (arXiv:1605.03661v2 [stat.ML] UPDATED)
Observational studies are rising in importance due to the widespread accumulation of data in fields such as healthcare, education, employment and ecology. We consider the task of answering counterfactual questions such as, "Would this patient have lower blood sugar had she received a different medication?". We propose a new algorithmic framework for counterfactual inference which brings together ideas from domain adaptation and representation learning. In addition to a theoretical justification, we perform an empirical comparison with previous approaches to causal inference from observational data. Our deep learning algorithm significantly outperforms the previous state-of-the-art.
from cs.AI updates on arXiv.org http://ift.tt/1TBylhx
via IFTTT
Centenary receives $2 million anonymous estate gift
from Google Alert - anonymous http://ift.tt/1tiCqSj
via IFTTT
Ravens: Timmy Jernigan says he's \\"going to be the bigger person\\" in dustup with Warren Sapp over switching to his No. 99 (ESPN)
via IFTTT
Hino de Coroação de Nossa Senhora do Rosário (Anonymous)
from Google Alert - anonymous http://ift.tt/1XaT2ao
via IFTTT
I have a new follower on Twitter
Joel Comm
Keynote Speaker, NY Times Best-Selling author, Futurist and Social Influencer - For Sales/PR/Promo https://t.co/qcHBn8dS9x #DoGoodStuff - Life in Beta
Northern Colorado
https://t.co/9VVUt1FbMs
Following: 413374 - Followers: 490094
June 08, 2016 at 01:26PM via Twitter http://twitter.com/joelcomm
Hosting Migrate JS throws anonymous function error
from Google Alert - anonymous http://ift.tt/1WF1NJZ
via IFTTT
[FD] SQL Injection Vulnerabilities found in European Commisssion & European Parliament
Source: Gmail -> IFTTT-> Blogger
Stanford rape victim's statement on remaining anonymous illustrates her fortitude, general heroism.
from Google Alert - anonymous http://ift.tt/1t8ZiTO
via IFTTT
μTorrent Forum Hacked; Change your Password Immediately
from The Hacker News http://ift.tt/1t8BI9M
via IFTTT
I have a new follower on Twitter
Charles Boicey
Chief Innovation Officer advancing healthcare with an affordable cloud, multi-tenant, Healthcare Analytics Ecosystem utilizing supported open source technology
Jacksonville Beach
https://t.co/8GOcQOlOSt
Following: 2236 - Followers: 2700
June 08, 2016 at 08:14AM via Twitter http://twitter.com/N2InformaticsRN
How to Run Android on your iPhone using this 3D Printed Phone Case
from The Hacker News http://ift.tt/1TVgJhn
via IFTTT
ISS Daily Summary Report – 06/07/16
from ISS On-Orbit Status Report http://ift.tt/24yY7J5
via IFTTT
Anonymous hacktivists attack world's banks, London Stock Exchange
from Google Alert - anonymous http://ift.tt/1TVcFOh
via IFTTT
University Pays Hackers $20,000 to get back its Ransomware Infected Files
from The Hacker News http://ift.tt/1t8bUdD
via IFTTT
Night on Venus in Infrared from Orbiting Akatsuki
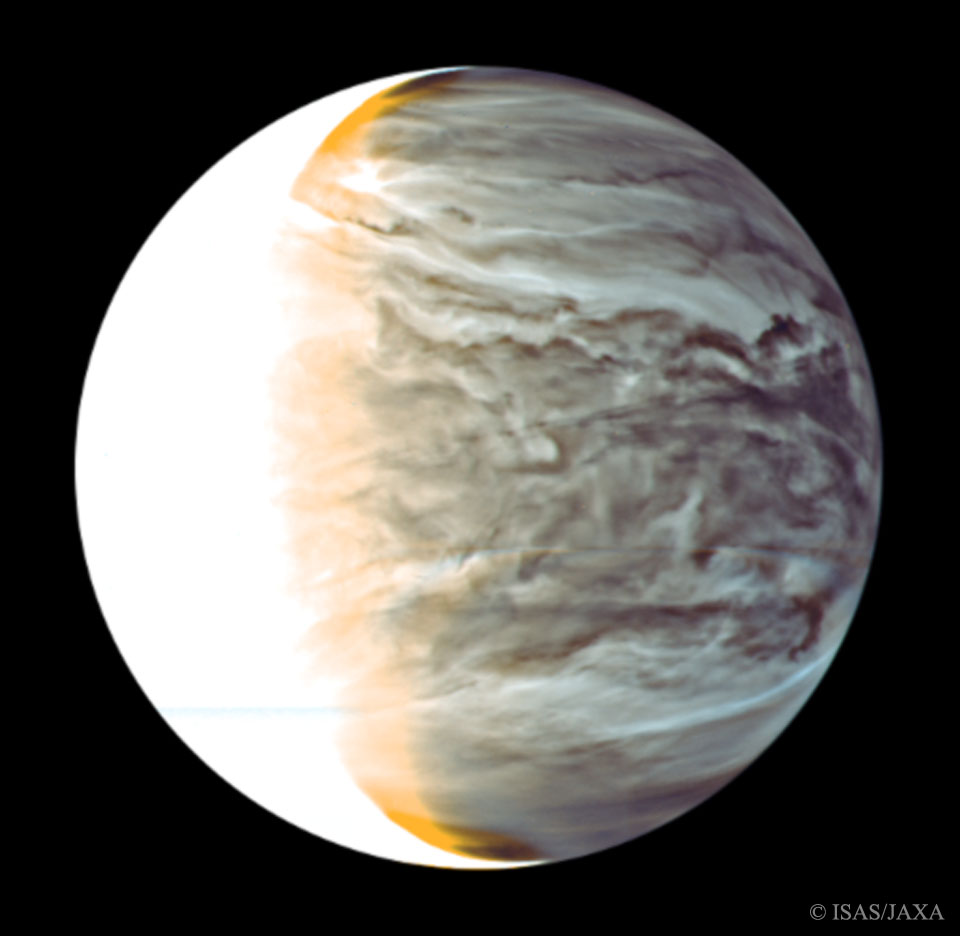
Orioles Video: Manny Machado says \"I don't regret anything\" after getting hit, charging mound; \"99 (mph) is no joke\" (ESPN)
via IFTTT
Stanford Victim Remains Anonymous
from Google Alert - anonymous http://ift.tt/24y3Y1o
via IFTTT
Tuesday, June 7, 2016
Orioles Video: Buck Showalter not upset Manny Machado charged mound; \"I don't like when my guys are put in harm's way\" (ESPN)
via IFTTT
I have a new follower on Twitter
OptimalPlus
The leader in Manufacturing Intelligence for semiconductors and electronics
http://t.co/o4HhEan88Z
Following: 3158 - Followers: 3313
June 07, 2016 at 10:34PM via Twitter http://twitter.com/OptimalPlus
Preliminaries of a Space Situational Awareness Ontology. (arXiv:1606.01924v1 [cs.AI])
Space situational awareness (SSA) is vital for international safety and security, and the future of space travel. By improving SSA data-sharing we improve global SSA. Computational ontology should provide one means toward that goal. This paper develops the ontology of the SSA domain and takes steps in the creation of a Space Situational Awareness Ontology. It follows (Rovetto, 2015) and outlines objectives, requirements and desiderata; and describes the SSA domain and discipline of ontology. The purposes of the SSA ontology are to explore the potential for ontology development and engineering to (i) represent SSA data, general knowledge, and domain objects, (ii) clearly annotate and express the meaning of that orbital, near-earth and deep-space da-ta, and (iii) foster SSA data sharing among SSA actors and space object catalogs. By improving global SSA via actionable data- and knowledge-exchange, we can achieve the broader goals (and motivations) of (iv) advancing our capacity for planetary defense from near- or deep-space ob-ects, and (v) improving spaceflight safety for future generations.
from cs.AI updates on arXiv.org http://ift.tt/1PFIOgb
via IFTTT
Consistency and Trust in Peer Data Exchange Systems. (arXiv:1606.01930v1 [cs.DB])
We propose and investigate a semantics for "peer data exchange systems" where different peers are related by data exchange constraints and trust relationships. These two elements plus the data at the peers' sites and their local integrity constraints are made compatible via a semantics that characterizes sets of "solution instances" for the peers. They are the intended -possibly virtual- instances for a peer that are obtained through a data repair semantics that we introduce and investigate. The semantically correct answers from a peer to a query, the so-called "peer consistent answers", are defined as those answers that are invariant under all its different solution instances. We show that solution instances can be specified as the models of logic programs with a stable model semantics. The repair semantics is based on null values as used in SQL databases, and is also of independent interest for repairs of single databases with respect to integrity constraints.
from cs.AI updates on arXiv.org http://ift.tt/1Zw9kc5
via IFTTT
Assisted Energy Management in Smart Microgrids. (arXiv:1606.01949v1 [cs.AI])
Demand response provides utilities with a mechanism to share with end users the stochasticity resulting from the use of renewable sources. Pricing is accordingly used to reflect energy availability, to allocate such a limited resource to those loads that value it most. However, the strictly competitive mechanism can result in service interruption in presence of competing demand. To solve this issue we investigate on the use of forward contracts, i.e., service level agreements priced to reflect the expectation of future supply and demand curves. Given the limited resources of microgrids, service interruption is an opposite objective to the one of service availability. We firstly design policy-based brokers and identify then a learning broker based on artificial neural networks. We show the latter being progressively minimizing the reimbursement costs and maximizing the overall profit.
from cs.AI updates on arXiv.org http://ift.tt/1PFJphK
via IFTTT
Human vs. Computer Go: Review and Prospect. (arXiv:1606.02032v1 [cs.AI])
The Google DeepMind challenge match in March 2016 was a historic achievement for computer Go development. This article discusses the development of computational intelligence (CI) and its relative strength in comparison with human intelligence for the game of Go. We first summarize the milestones achieved for computer Go from 1998 to 2016. Then, the computer Go programs that have participated in previous IEEE CIS competitions as well as methods and techniques used in AlphaGo are briefly introduced. Commentaries from three high-level professional Go players on the five AlphaGo versus Lee Sedol games are also included. We conclude that AlphaGo beating Lee Sedol is a huge achievement in artificial intelligence (AI) based largely on CI methods. In the future, powerful computer Go programs such as AlphaGo are expected to be instrumental in promoting Go education and AI real-world applications.
from cs.AI updates on arXiv.org http://ift.tt/1Zw9AaZ
via IFTTT
Sorting out symptoms: design and evaluation of the 'babylon check' automated triage system. (arXiv:1606.02041v1 [cs.AI])
Prior to seeking professional medical care it is increasingly common for patients to use online resources such as automated symptom checkers. Many such systems attempt to provide a differential diagnosis based on the symptoms elucidated from the user, which may lead to anxiety if life or limb-threatening conditions are part of the list, a phenomenon termed 'cyberchondria' [1]. Systems that provide advice on where to seek help, rather than a diagnosis, are equally popular, and in our view provide the most useful information. In this technical report we describe how such a triage system can be modelled computationally, how medical insights can be translated into triage flows, and how such systems can be validated and tested. We present babylon check, our commercially deployed automated triage system, as a case study, and illustrate its performance in a large, semi-naturalistic deployment study.
from cs.AI updates on arXiv.org http://ift.tt/1PFHk5I
via IFTTT
Towards Playlist Generation Algorithms Using RNNs Trained on Within-Track Transitions. (arXiv:1606.02096v1 [cs.AI])
We introduce a novel playlist generation algorithm that focuses on the quality of transitions using a recurrent neural network (RNN). The proposed model assumes that optimal transitions between tracks can be modelled and predicted by internal transitions within music tracks. We introduce modelling sequences of high-level music descriptors using RNNs and discuss an experiment involving different similarity functions, where the sequences are provided by a musical structural analysis algorithm. Qualitative observations show that the proposed approach can effectively model transitions of music tracks in playlists.
from cs.AI updates on arXiv.org http://ift.tt/1Zw9kca
via IFTTT
Adapting Sampling Interval of Sensor Networks Using On-Line Reinforcement Learning. (arXiv:1606.02193v1 [cs.NI])
Monitoring Wireless Sensor Networks (WSNs) are composed by sensor nodes that report temperature, relative humidity and other environmental parameters. The time between two successive measurements is a critical parameter to set during the WSN configuration, because it can impact the WSN's lifetime, the wireless medium contention and the quality of the reported data. As trends in monitored parameters can significantly vary between scenarios and within time, identifying a sampling interval suitable for several cases is also challenging. In this work, we propose a dynamic sampling rate adaptation scheme based on reinforcement learning, able to tune sensors' sampling interval on-the-fly, according to environmental conditions and application requirements. The main goal is to set the sampling interval to the best value possible so as to avoid oversampling and save energy, while not missing environmental changes that can be relevant for the application. In simulations, our mechanism could reduce up to 73% the total number of transmissions compared to a fixed strategy and, simultaneously, keep the average quality of information provided by the WSN. The inherent flexibility of the reinforcement learning algorithm facilitates its use in several scenarios, so as to exploit the broad scope of the Internet of Things.
from cs.AI updates on arXiv.org http://ift.tt/1PFJ7rt
via IFTTT
Multi-resource defensive strategies for patrolling games with alarm systems. (arXiv:1606.02221v1 [cs.AI])
Security Games employ game theoretical tools to derive resource allocation strategies in security domains. Recent works considered the presence of alarm systems, even suffering various forms of uncertainty, and showed that disregarding alarm signals may lead to arbitrarily bad strategies. The central problem with an alarm system, unexplored in other Security Games, is finding the best strategy to respond to alarm signals for each mobile defensive resource. The literature provides results for the basic single-resource case, showing that even in that case the problem is computationally hard. In this paper, we focus on the challenging problem of designing algorithms scaling with multiple resources. First, we focus on finding the minimum number of resources assuring non-null protection to every target. Then, we deal with the computation of multi-resource strategies with different degrees of coordination among resources. For each considered problem, we provide a computational analysis and propose algorithmic methods.
from cs.AI updates on arXiv.org http://ift.tt/1Zw9pfR
via IFTTT
Emotional Intensity analysis in Bipolar subjects. (arXiv:1606.02231v1 [cs.AI])
The massive availability of digital repositories of human thought opens radical novel way of studying the human mind. Natural language processing tools and computational models have evolved such that many mental conditions are predicted by analysing speech. Transcription of interviews and discourses are analyzed using syntactic, grammatical or sentiment analysis to infer the mental state. Here we set to investigate if classification of Bipolar and control subjects is possible. We develop the Emotion Intensity Index based on the Dictionary of Affect, and find that subjects categories are distinguishable. Using classical classification techniques we get more than 75\% of labeling performance. These results sumed to previous studies show that current automated speech analysis is capable of identifying altered mental states towards a quantitative psychiatry.
from cs.AI updates on arXiv.org http://ift.tt/1PFI8r5
via IFTTT
A Formal Calculus for International Relations Computation and Evaluation. (arXiv:1606.02239v1 [cs.CR])
This publication presents a relation computation or calculus for international relations using a mathematical modeling. It examined trust for international relations and its calculus, which related to Bayesian inference, Dempster-Shafer theory and subjective logic. Based on an observation in the literature, we found no literature discussing the calculus method for the international relations. To bridge this research gap, we propose a relation algebra method for international relations computation. The proposed method will allow a relation computation which is previously subjective and incomputable. We also present three international relations as case studies to demonstrate the proposed method is a real-world scenario. The method will deliver the relation computation for the international relations that to support decision makers in a government such as foreign ministry, defense ministry, presidential or prime minister office. The Department of Defense (DoD) may use our method to determine a nation that can be identified as a friendly, neutral or hostile nation.
from cs.AI updates on arXiv.org http://ift.tt/1Zw9ekS
via IFTTT
Adaptive Skills, Adaptive Partitions (ASAP). (arXiv:1602.03351v2 [cs.LG] UPDATED)
We introduce the Adaptive Skills, Adaptive Partitions (ASAP) framework that (1) learns skills (i.e., temporally extended actions or options) as well as (2) where to apply them. We believe that both (1) and (2) are necessary for a truly general skill learning framework, which is a key building block needed to scale up to lifelong learning agents. The ASAP framework can also solve related new tasks simply by adapting where it applies its existing learned skills. We prove that ASAP converges to a local optimum under natural conditions. Finally, our experimental results, which include a RoboCup domain, demonstrate the ability of ASAP to learn where to reuse skills as well as solve multiple tasks with considerably less experience than solving each task from scratch.
from cs.AI updates on arXiv.org http://ift.tt/1KcDjDb
via IFTTT
A Discrete and Bounded Envy-Free Cake Cutting Protocol for Any Number of Agents. (arXiv:1604.03655v5 [cs.DS] UPDATED)
We consider the well-studied cake cutting problem in which the goal is to find an envy-free allocation based on queries from the agents. The problem has received attention in computer science, mathematics, and economics. It has been a major open problem whether there exists a bounded and discrete envy-free protocol. We resolve the problem by proposing a discrete and bounded envy-free protocol for any number of agents.
from cs.AI updates on arXiv.org http://ift.tt/1Q85mzr
via IFTTT
Distributed Clustering of Linear Bandits in Peer to Peer Networks. (arXiv:1604.07706v3 [cs.LG] UPDATED)
We provide two distributed confidence ball algorithms for solving linear bandit problems in peer to peer networks with limited communication capabilities. For the first, we assume that all the peers are solving the same linear bandit problem, and prove that our algorithm achieves the optimal asymptotic regret rate of any centralised algorithm that can instantly communicate information between the peers. For the second, we assume that there are clusters of peers solving the same bandit problem within each cluster, and we prove that our algorithm discovers these clusters, while achieving the optimal asymptotic regret rate within each one. Through experiments on several real-world datasets, we demonstrate the performance of proposed algorithms compared to the state-of-the-art.
from cs.AI updates on arXiv.org http://ift.tt/1WoMi7e
via IFTTT
Plagiarism Detection in Polyphonic Music using Monaural Signal Separation. (arXiv:1503.00022v1 [cs.SD] CROSS LISTED)
Given the large number of new musical tracks released each year, automated approaches to plagiarism detection are essential to help us track potential violations of copyright. Most current approaches to plagiarism detection are based on musical similarity measures, which typically ignore the issue of polyphony in music. We present a novel feature space for audio derived from compositional modelling techniques, commonly used in signal separation, that provides a mechanism to account for polyphony without incurring an inordinate amount of computational overhead. We employ this feature representation in conjunction with traditional audio feature representations in a classification framework which uses an ensemble of distance features to characterize pairs of songs as being plagiarized or not. Our experiments on a database of about 3000 musical track pairs show that the new feature space characterization produces significant improvements over standard baselines.
from cs.AI updates on arXiv.org http://ift.tt/1B4IFbk
via IFTTT
Ravens Image: Joe Flacco, Dennis Pitta, Justin Tucker and others gather to watch Monday's episode of \"The Bachelorette\" (ESPN)
via IFTTT
[FD] Microsoft Education - Code Execution Vulnerability
Source: Gmail -> IFTTT-> Blogger
[FD] Wordpress Levo-Slideshow 2.3 - Arbitrary File Upload Vulnerability
Source: Gmail -> IFTTT-> Blogger
[FD] Wordpress Levo-Slideshow v2.3 - Persistent Vulnerability
Source: Gmail -> IFTTT-> Blogger