Latest YouTube Video
Saturday, March 31, 2018
Puss in Boots
from Google Alert - anonymous https://ift.tt/2H0E5DP
via IFTTT
How add email field to anonymous user's comment?
from Google Alert - anonymous https://ift.tt/2uCVPTh
via IFTTT
Twins' combined no-hitter broken up with 2 outs in 8th inning on single by Orioles' Jonathan Schoop (ESPN)
via IFTTT
No-Hitter Watch: Twins' Kyle Gibson (6.0 IP) and Ryan Pressley have not allowed a hit through 7 innings vs. Orioles (ESPN)
via IFTTT
No-Hitter Watch: Twins' Kyle Gibson has not allowed a hit through 6 innings vs. Orioles (ESPN)
via IFTTT
Russian Hacker Who Allegedly Hacked LinkedIn and Dropbox Extradited to US
from The Hacker News https://ift.tt/2GF5Dkv
via IFTTT
Magna Carta
from Google Alert - anonymous https://ift.tt/2H0ikV0
via IFTTT
Twilight in a Western Sky
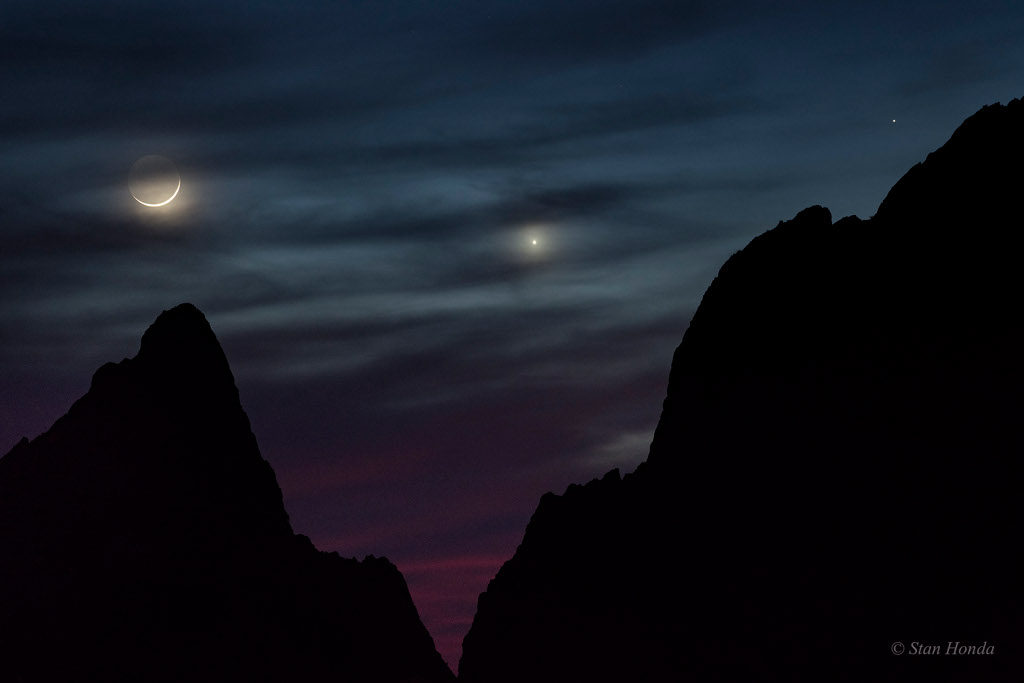
Friday, March 30, 2018
Becoming anonymous on the internet and gaining back your freedom
from Google Alert - anonymous https://ift.tt/2E8BkgK
via IFTTT
[FD] Null Pointer Deference (Denial of Service)-Kingsoft Internet Security 9+ Kernel Driver KWatch3.sys
Source: Gmail -> IFTTT-> Blogger
[FD] SSRF(Server Side Request Forgery) in Tpshop <= 2.0.6 (CVE-2017-16614)
Source: Gmail -> IFTTT-> Blogger
[FD] APPLE-SA-2018-3-29-8 iCloud for Windows 7.4
Source: Gmail -> IFTTT-> Blogger
[FD] APPLE-SA-2018-3-29-7 iTunes 12.7.4 for Windows
Source: Gmail -> IFTTT-> Blogger
[FD] APPLE-SA-2018-3-29-6 Safari 11.1
Source: Gmail -> IFTTT-> Blogger
[FD] APPLE-SA-2018-3-29-5 macOS High Sierra 10.13.4, Security Update 2018-002 Sierra, and Security Update 2018-002 El Capitan
Source: Gmail -> IFTTT-> Blogger
[FD] APPLE-SA-2018-3-29-2 watchOS 4.3
Source: Gmail -> IFTTT-> Blogger
[FD] CVE-2018-5708
Source: Gmail -> IFTTT-> Blogger
[FD] CA20180328-01: Security Notice for CA API Developer Portal
Source: Gmail -> IFTTT-> Blogger
[FD] CA20180329-01: Security Notice for CA Workload Automation AE and CA Workload Control Center
Source: Gmail -> IFTTT-> Blogger
Free-agency grades: Ravens get a B- after signings of WRs John Brown, Michael Crabtree - Jamison Hensley (ESPN)
via IFTTT
Law360's Satisfaction Survey
from Google Alert - anonymous https://ift.tt/2GVCOhl
via IFTTT
Farm supervisor
from Google Alert - anonymous https://ift.tt/2uy33rU
via IFTTT
Thursday, March 29, 2018
▶ Adam Jones smacks 11th-inning walk-off homer in Orioles' 3-2 win over Twins (ESPN)
via IFTTT
Messrs. Maskelyne and Cooke from England's home of mystery
from Google Alert - anonymous https://ift.tt/2GkPLo8
via IFTTT
Microsoft's Meltdown Patch Made Windows 7 PCs More Insecure
from The Hacker News https://ift.tt/2Gyjzgn
via IFTTT
ISS Daily Summary Report – 3/28/2018
from ISS On-Orbit Status Report https://ift.tt/2GDsL2Q
via IFTTT
Apple macOS Bug Reveals Passwords for APFS Encrypted Volumes in Plaintext
from The Hacker News https://ift.tt/2E2Rx7e
via IFTTT
NGC 2023 in the Horsehead s Shadow
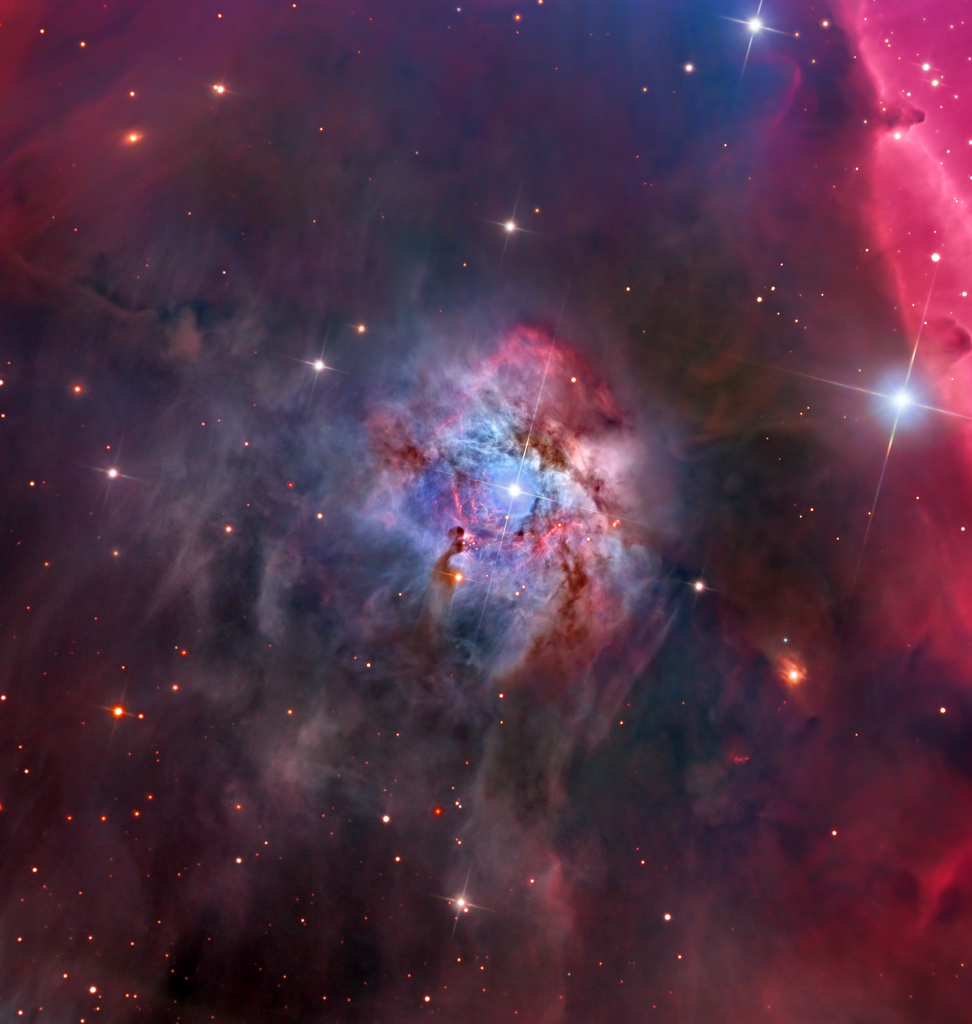
Wednesday, March 28, 2018
📈 MLB Power Rankings: Orioles No. 20 to begin 2018 season (ESPN)
via IFTTT
ISS Daily Summary Report – 3/27/2018
from ISS On-Orbit Status Report https://ift.tt/2GDfszx
via IFTTT
Will the Ravens draft a QB? We rate all 32 teams' chances of selecting a signal-caller (ESPN)
via IFTTT
An interview with Kwabena Agyeman, co-creator of OpenMV and microcontroller expert
After publishing last week’s blog post on reading barcodes with Python and OpenMV, I received a lot of emails from readers asking questions about embedded computer vision and how microcontrollers can be used for computer vision.
Instead of trying to address these questions myself, I thought it would be best to bring in a true expert — Kwabena Agyeman, co-founder of OpenMV, a small, affordable, and expandable embedded computer vision device.
Kwabena is modest in this interview and denies being an expert (a true testament to his kind character and demeanor), but trust me, meeting him and chatting with him is a humbling experience. His knowledge of embedded programming and microcontroller design is incredible. I could listen to him talk about embedded computer vision all day.
I don’t cover embedded devices here on PyImageSearch often so it’s a true treat to have Kwabena here today.
Join me in welcoming Kwabena Agyeman to the PyImageSearch blog. And to learn more about embedded computer vision, just keep reading.
An interview with Kwabena Agyeman, co-creator of OpenMV and microcontroller expert
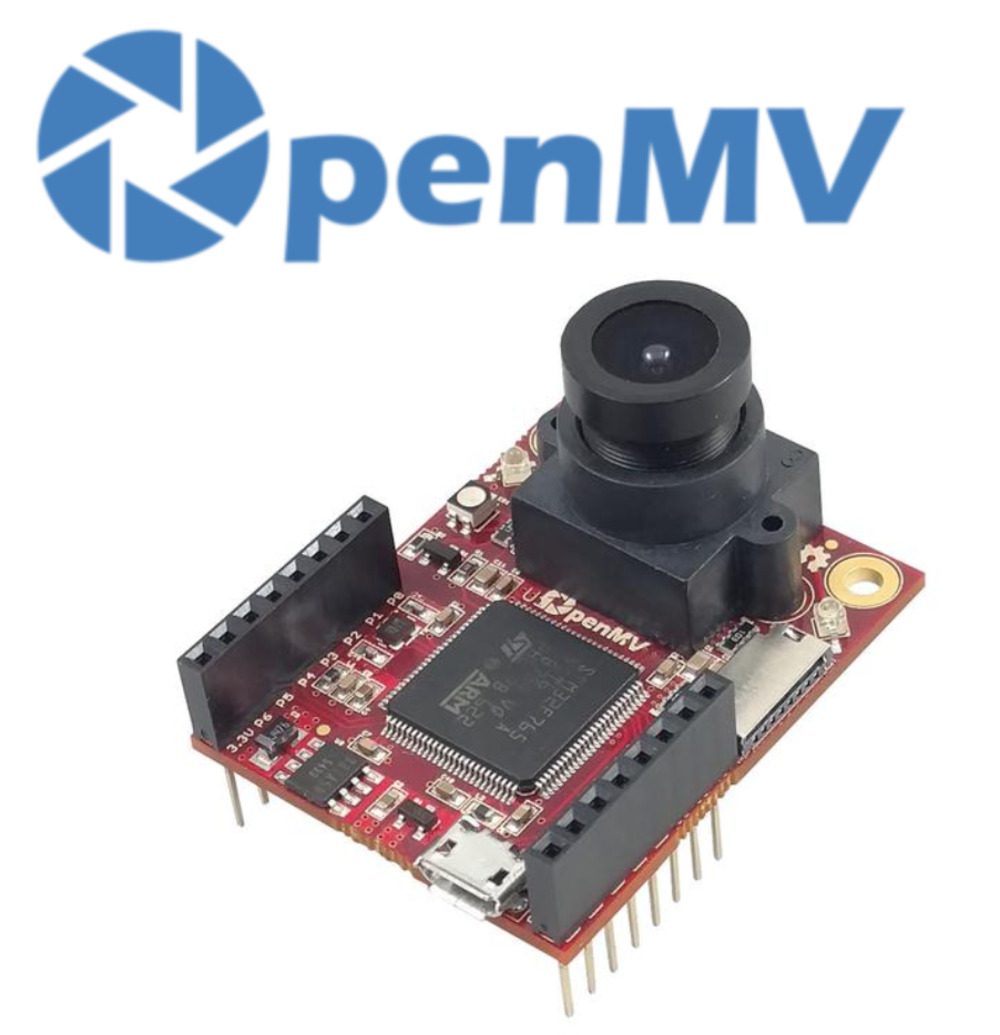
Figure 1: The OpenMV camera is a powerful embedded camera board that runs MicroPython.
Adrian: Hey Kwabena, thanks for doing this interview! It’s great to have you on the PyImageSearch blog. For people who don’t know you and OpenMV, who are you and what do you do?
Kwabena: Hi Adrian, thanks for having me in today. Me and my co-founder Ibrahim created the OpenMV Cam and run the OpenMV project.
OpenMV is a focused effort on making embedded computer/machine vision more accessible. The ultimate goal of the project is to enable machine-vision in more embedded devices than there are today.
For example, let’s say you want to add a face detection sensor to your toaster. This is probably overkill for any application, but, bear with me.
First, you can’t just go out today and buy a $50 face detection sensor. Instead, you’re looking at least setting up a Single-Board-Computer (SBC) Linux system running OpenCV. This means adding face detection to your toaster now just became a whole new project.
If your goal was to just detect if there’s a face in view or not, and then toggle a wire to release the toast when you look at the toaster you don’t necessarily want to go down the SBC path.
Instead, what you really want is a microcontroller that can accomplish the goal of detecting faces out-of-the-box and toggling a wire with minimal setup.
So, the OpenMV project is basically about proving high-level machine-vision functionality out of the box for a variety of tasks to developers who want to add powerful features to their projects without having to focus on all the details.
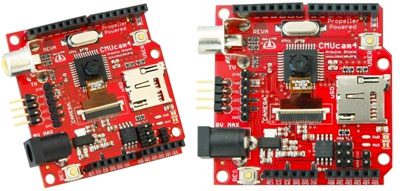
Figure 2: The CMUcam4 is a fully programmable embedded computer vision sensor developed by Kwabena Agyeman while at Carnegie Mellon University.
Adrian: That’s a great point regarding having to set up a SBC Linux system, install OpenCV, and write the code, just to achieve a tiny bit of functionality. I don’t do much work with embedded devices so it’s insightful seeing it from a different perspective. What inspired you to start working in the computer vision, machine learning, and the embedded field?
Kwabena: Thanks for asking Adrian, I got into machine-vision back at Carnegie Mellon University working under Anthony Rowe who created the CMUcam 1, 2, and 3. While I was a student there I created the CMUcam 4 for simple color tracking applications.
While limited, the CMUcams were able to do their jobs of tracking colors quite well (if deployed in a constant lighting environment). I really enjoyed working on the CMUcam4 because it blended board design, microcontroller programming, GUI development, and data-visualization in one project.
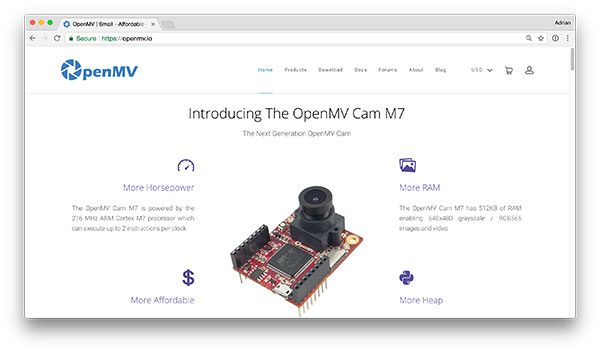
Figure 3: A small, affordable, and expandable embedded computer vision device.
Adrian: Let’s get get into more detail about OpenMV and the OpenMV Cam. What exactly is OpenMV Cam and what is it used for?
Kwabena: So, the OpenMV Cam is a low-powered machine-vision camera. Our current model is the OpenMV Cam M7 which is powered by a 216 MHz Cortex-M7 processor that can execute two-instructions per clock making it about half as fast (single-threaded no-SIMD) compute-wise as the Raspberry Pi zero.
The OpenMV Cam is also a MicroPython board. This means you program it in Python 3. Note that this doesn’t mean desktop python libraries are available. But, if you can program in Python you can program the OpenMV Cam and you’ll feel at home using it.
What’s cool though is that we’ve built a number of high-level machine-vision algorithms into the OpenMV Cam’s firmware (which is written in C — python is just to allow you to glue vision logic together like you do with OpenCV’s python library bindings).
In particular, we’ve got:
- Multi-color blob tracking
- Face detection
- AprilTag tracking
- QR Code, Barcode, Data Matrix detection and decoding
- Template matching
- Phase-correlation
- Optical-flow
- Frame differencing
- and more built-in.
Basically, it’s like OpenCV on a low-power microcontroller (runs off a USB port) with Python bindings
Anyway, our goal is to wrap up as much functionality into an easy-to-use function calls as possible. For example, we have a “find_blobs()” method which returns a list of color blobs objects in the image. Each blob object has an centroid, bounding box, pixel count, rotation angle, and etc. So, the function call automatically segments an image (RGB or Grayscale) by a list of color-thresholds, finds all blobs (connected components), merges overlapping blobs based on their bounding boxes, and additionally calculates each blob’s centroid, rotation angle, etc. Subjectively, using our “find_blobs()” is a lot more straight forwards than finding color blobs with OpenCV if you’re a beginner. That said, our algorithm is also less flexible if you need to do something we didn’t think of. So, there’s a trade-off.
Moving on, sensing is just one part of the problem. Once you detect something you need to act. Because the OpenMV Cam is a microcontroller you can toggle I/O pins, control SPI/I2C buses, send UART data, control servos, and more all from the same script you’ve got your vision logic in. With the OpenMV Cam you sense, plan, and act all from one short python script.
Adrian: Great explanation. Can you elaborate more on the target market for the OpenMV? If you had to describe your ideal end user who absolutely had to have an OpenMV, who would they be?
Kwabena: Right now we’re targeting the hobbyist market with the system. Hobbyist have been our biggest buyers so far and helped us sell over five thousand OpenMV Cam M7s last year. We’ve also got a few companies buying the cameras too.
Anyway, as our firmware gets more mature we hope to sell more cameras to more companies building products.
Right now we’re still rapidly building out our firmware functionality to more or less compliment OpenCV for basic image processing functionality. We’ve already got a lot of stuff on board but we’re trying to make sure you have any tool you need like shadow removal with inpainting for creating a shadow free background frame differencing applications.
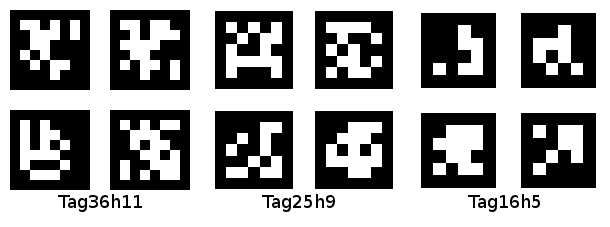
Figure 4: An example of AprilTags (Image credit: MIT).
Adrian: Shadow removal, that’s fun. So, what was the most difficult feature or aspect that you had to wrangle with when putting together OpenMV?
Kwabena: Porting AprilTags to the OpenMV Cam was the most challenging algorithm to get running onboard.
I started with the AprilTag 2 source code meant for the PC. To get it running on the OpenMV Cam M7 which has only 512 KB of RAM versus a desktop PC. I had go through all 15K+ lines of code and redo how memory allocations worked to be more efficient.
Sometimes this was as simple as moving large array allocations from malloc to a dedicated stack. Sometimes I had to change how some algorithms worked to be more efficient.
For example, AprilTags computes a lookup table of every possible hamming code word with 0, 1, 2, etc. bit errors when trying to match detected tag bit patterns with a tag dictionary. This lookup-table (LUT) can be over 30 MBs for some tag dictionaries! Sure, indexing a LUT is fast, but, a linear search through the tag dictionaries for a matching tag can work too.
Anyway, after porting the algorithm the OpenMV Cam M7 it can run AprilTags at 160×120 at 12 FPS. This let’s you detect tags printed on 8”x11” paper from about 8” away with a microcontroller which can run off of your USB port.
Adrian: Wow! Having to manually go through all 15K lines of code and re-implement certain pieces of functionality must have been quite the task. I hear there are going to be some really awesome new OpenMV features in the next release. Can you tell us about them?
Kwabena: Yes, our next product, the OpenMV Cam H7 powered by the STM32H7 processor will double our performance. In fact, it’s coremark score is on par with the 1 GHz Raspberry Pi zero (2020.55 versus 2060.98). That said, the Cortex-M7 core doesn’t have NEON or a GPU. But, we should be able to keep up for CPU limited algorithms.
However, the big feature add is removable camera module support. This allows us to offer the OpenMV Cam H7 with an inexpensive rolling shutter camera module like we do now. But, for more professional users we’ll have a global shutter options for folks who are trying to do machine vision in high speed applications like taking pictures of products moving on a conveyor belt. Better, yet, we’re also planning to support FLIR Lepton Thermal sensors for machine vision too. Best of all, each camera module will use the same “sensor.snapshot()” construct we use to take pictures now allowing you to switch out one module for another without changing your code.
Finally, thanks to ARM, you can now neural networks on the Cortex-M7. Here’s a video of the OpenMV Cam running a CIFAR-10 network onboard:
We’re going to be building out this support for the STM32H7 processor so that you can run NN’s trained on your laptop to do things like detecting when people enter rooms and etc. The STM32H7 should be able to run a variety of simple NN’s for lots of common detection task folks want for an embedded system to do.
We’ll be running a KickStarter for the next generation OpenMV Cam this year. Sign-up on our email list here and follow us on Twitter to stay up-to-date for when we launch the KickStarter.
Adrian: Global shutter and thermal imaging support is awesome! Theoretically, could I turn an OpenMV Cam with a global shutter sensor into a webcam for use with my Raspberry Pi 3? Inexpensive global shutter sensors are hard to find.
Kwabena: Yes, the OpenMV Cam can be used as a webcam. Our USB speed is limited to 12 Mb/s though, so, you’ll want to stream JPEG compressed images. You can also connect the OpenMV Cam to your Raspberry Pi via SPI for a faster 54 Mb/s transfer rate. Since the STM32H7 has a hardware JPEG encoder onboard now the OpenMV Cam H7 should be able to provide a nice high FPS precisely triggered frame stream to your Raspberry Pi.
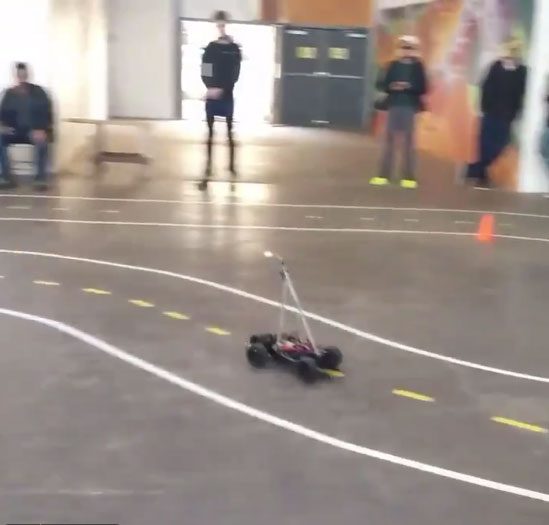
Figure 5: Using OpenMV to build DIY robocar racers.
Adrian: Cool, let’s move on. One of the most exciting aspects of developing a new tool, library, or piece of software is to see how your work is used by others. What are some of the more surprising ways you’ve seen OpenMV used?
Kwabena: For hobbyist our biggest feature has been color tracking. We do that very well at above 50 FPS with our current OpenMV Cam M7. I think this has been the main attraction for a lot of customers. Color tracking has historically been the only thing you were able to do on a microcontroller so it makes sense.
QR Code, Barcode, Datamatrix, and AprilTag support have also been selling points.
For example, we’ve had quadcopter folks start using the OpenMV Cam to point down at giant AprilTags printed out on the ground for precision landing. You can have one AprilTag inside of another one and as the quadcopter gets closer to the ground the control algorithm tries to keep the copter centered on the tag in view.
However, what’s tickled me the most is doing DIY Robocar racing with the OpenMV Cam and having some of my customers beat me in racing with their OpenMV Cams.
Adrian: If a PyImageSearch readers would like to get their own OpenMV camera, where can they purchase one?
Kwabena: We just finished another production run of 2.5K OpenMV Cams and you can buy them online on our webstore now. We’ve also got lens accessories, shields for controlling motors, and more.
Adrian: Most people don’t know this, but you and I ran a Kickstarter campaign at the same time back in 2015! Mine was for the PyImageSearch Gurus course while yours was for the initial release and manufacturing of the OpenMV Camera. OpenMV’s Kickstarter easily beat my own, which just goes to show you how interested the embedded community was in the product — fantastic job and congrats on the success. What was running your first Kickstarter campaign like?
Kwabena: Running that KickStarter campaign was stressful. We’ve come a long, long, long way since then. I gave a talk on this a few years back which more or less summarizes my experience:
Anyway, it’s a lot of work and a lot of stress.
For our next KickStarter we’re trying to prepare as much as possible beforehand so it’s more of a turnkey operation. We’ve got our website, online-shop, forums, documentation, shipping, etc. all setup now so we don’t have to build the business and the product at the same time anymore. This will let us focus on delivering the best experience for our backers.
Adrian: I can attest to the fact that running a Kickstarter campaign is incredibly stressful. It’s easily a full-time job for ~3 months as you prepare the campaign, launch it, and fund it. And once it’s funded you then need to deliver on your promise! It’s a rewarding experience and I wouldn’t discourage others from doing it, but prepared to be really stressed out for 3-4 months. Shifting gears a bit, as an expert in your field, who do you talk to when you get “stuck” on a hard problem?
Kwabena: I wouldn’t say I’m an expert. I’m learning computer vision like everyone else. Developing the OpenMV Cam has actually been a great way to learn how algorithms work. For example, I learned a lot porting the AprilTag code. There’s a lot of magic in that C code. I’m also quite excited actually to now start adding more machine learning features to the OpenMV Cam using the ARM CMSIS NN library for the Cortex-M7.
Anyway, to answer where I go for help… The internet! And research papers! Lots of research papers. I do the reading so my OpenMV Cam users don’t have to.
Adrian: From your LinkedIn I know you have a lot of experience in hardware design languages. What advice do you have for programmers interested in using FPGAs for computer vision?
Kwabena: Hmm… You can definitely get a lot of performance out FPGAs. However, it’s definitely a pay-to-play market. You’re going to need some serious budget to get access to any of the high-end hardware and/or intellectual property. That said, if you’ve got an employer willing to spend there’s a lot of development going on that will allow you to run very large deep neural networks on FPGAs. It’s definitely sweet to get a logic pipeline up and running that’s able to process gigabytes of data a second.
Now, there’s also a growing medium-end FPGA market that’s affordable to play in if you don’t have a large budget. Intel (previously Altera) has an FPGA called the Cyclone for sale that’s more or less affordable if you’re willing pay for the hardware. You can interface the Cyclone to your PC via PCIe using Xillybus IP which exposes FIFOs on your FPGA as linux device files on your PC. This makes it super easy to move data over to the FPGA. Furthermore, Intel offers offers DDR memory controller IP for free so you can get some RAM buffers up and running. Finally, you just need to add a camera module and you can start developing.
But… that said, you’re going to run into a rather unpleasant brick wall on how to write verilog code and having to pay for the tool chains. The hardware design world is not really open source, nor will will you find lots of stack overflow threads about how to do things. Did I mention there’s no vision library for hardware available? Gotta roll everything yourself!
Adrian: When you’re not working at OpenMV, you’re at Planet Labs in San Francisco, CA, which is where PyImageConf, PyImageSearch’s very own computer vision and deep learning conference will be held. Will you be at PyImageConf this year (August 26-28th)? The conference will be a great place the show off OpenMV functionality. I know attendees would enjoy it.
Kwabena: Yes, I’ll be in town and present for the conference. I live in SF now.
Adrian: Great to hear! If a PyImageSearch reader wants to chat, what is the best place to connect with you?
Kwabena: Email us at openmv@openmv.io or comment on our forum. Additionally, please follow us on Twitter, our YouTube channel, and sign-up on our mailing list.
Summary
In today’s blog post I interviewed Kwabena Agyeman, co-founder of OpenMV.
If you have any questions for Kwabena, be sure to leave a comment on this post! Kwabena is a regular PyImageSearch reader and is active in the comments.
And if you enjoyed today’s post and want to be notified when future blog posts are published here on PyImageSearch, be sure to enter your email address in the form below!
The post An interview with Kwabena Agyeman, co-creator of OpenMV and microcontroller expert appeared first on PyImageSearch.
from PyImageSearch https://ift.tt/2GhHL2Y
via IFTTT
Tuesday, March 27, 2018
I have a new follower on Twitter

Nimbus XYZ
Follow a cloud-focused accountant for advice on on how to get your #business #online using #cloud #technology and increase #productivity in your business!
Washington, DC
https://t.co/hjJ3GheFfB
Following: 3541 - Followers: 2855
March 27, 2018 at 03:13PM via Twitter http://twitter.com/nimbus_xyz
[FD] DSA-2018-040: RSA® Authentication Agent for Web for IIS and Apache Web Server Multiple Vulnerabilities
Source: Gmail -> IFTTT-> Blogger
[FD] DSA-2018-058: Dell EMC ScaleIO Multiple Security Vulnerabilities
Source: Gmail -> IFTTT-> Blogger
I have a new follower on Twitter

AdvancedMD
Cloud software for independent medical practices that delivers industry-leading patient outcomes & financial performance. #MedicalBilling #EHR #Telemedicine
Salt Lake City
https://t.co/IqOYUqga76
Following: 9158 - Followers: 15279
March 27, 2018 at 01:13PM via Twitter http://twitter.com/advancedmd
📉 NFL Power Rankings: Ravens fall five spots to No. 24 after initial surge of free agency (ESPN)
via IFTTT
ISS Daily Summary Report – 3/26/2018
from ISS On-Orbit Status Report https://ift.tt/2GddQsN
via IFTTT
[FD] Microsoft Skype Mobile v81.2 & v8.13 - Remote Denial of Service Vulnerability
Source: Gmail -> IFTTT-> Blogger
[FD] Sandoba CP:Shop CMS v2016.1 - Multiple Cross Site Scripting Vulnerabilities
Source: Gmail -> IFTTT-> Blogger
[FD] Weblication CMS Core & Grid v12.6.24 - Multiple Cross Site Scripting Vulnerabilities
Source: Gmail -> IFTTT-> Blogger
[FD] AEF CMS v1.0.9 - (PM) Persistent Cross Site Scripting Vulnerability
Source: Gmail -> IFTTT-> Blogger
Monday, March 26, 2018
Ravens president Dick Cass wants to repair "disconnect" in relationship with the fans (ESPN)
via IFTTT
Leader of Hacking Group Who Stole $1 Billion From Banks Arrested In Spain
from The Hacker News https://ift.tt/2IWAQxM
via IFTTT
Anonymous 'Solo' Actor Dishes On Production, Calls Alden Ehrenrich 'Not Good Enough,'
from Google Alert - anonymous https://ift.tt/2pI1iTb
via IFTTT
2018 MLB Goals For All 30 Teams: What the Orioles must accomplish this season - Sam Miller (ESPN)
via IFTTT
Ravens need to prioritize acquiring WR Cameron Meredith from the Bears - Jamison Hensley (ESPN)
via IFTTT
An interview with David Austin: 1st place and $25,000 in Kaggle’s most popular image classification competition
In today’s blog post, I interview David Austin, who, with his teammate, Weimin Wang, took home 1st place (and $25,000) in Kaggle’s Iceberg Classifier Challenge.
David and Weimin’s winning solution can be practically used to allow safer navigation for ships and boats across hazardous waters, resulting in less damages to ships and cargo, and most importantly, reduce accidents, injuries, and deaths.
According to Kaggle, the Iceberg image classification challenge:
- Was the most popular image classification challenge they’ve ever had (measured in terms of competing teams)
- And was the 7th most popular competition of all time (across all challenges types: image, text, etc.)
Soon after the competition ended, David sent me the following message:
Hi Adrian, I’m a PyImageSearch Guru’s member, consumer of all your books, will be at PyImageConf in August, and an overall appreciative student of your teaching.
Just wanted to share a success story with you, as I just finished in first out of 3,343 teams in the Statoil Iceberg Classifier Kaggle competition ($25k first place prize).
A lot of my deep learning and cv knowledge was acquired through your training and a couple of specific techniques I learned through you were used in my winning solution (thresholding and mini-Googlenet specifically). Just wanted to say thanks and to let you know you’re having a great impact.
Thanks! David
David’s personal message really meant a lot to me, and to be honest, it got me a bit emotional.
As a teacher and educator, there is no better feeling in the world seeing readers:
- Get value out what you’ve taught from your blog posts, books, and courses
- Use their knowledge in ways that enriches their lives and improves the lives of others
Inside today’s post I’ll be interviewing David and discussing:
- What the iceberg image classification challenge is…and why it’s important
- The approach, algorithms, and techniques utilized by David and Weimin in their winning submission
- What the most difficult aspect of the challenge was (and how they overcame it)
- His advice for anyone who wants to compete in a Kaggle competition
I am so incredibly happy for both David and Weimin — they deserve all the congrats and a huge round of applause.
Join me in this interview and discover how David and his teammate Weimin won Kaggle’s most popular image classification competition.
An interview with David Austin: 1st place and $25,000 in Kaggle’s most popular competition
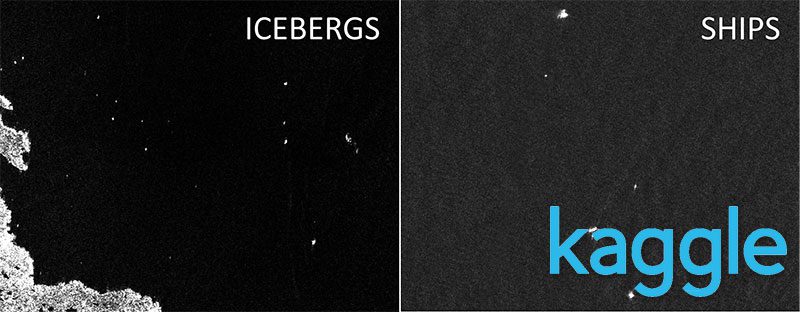
Figure 1: The goal of the Kaggle Iceberg Classifier challenge is to build an image classifier that classifies input regions of a satellite image as either “iceberg” or “ship” (source).
Adrian: Hi David! Thank you for agreeing to do this interview. And congratulations on your 1st place finish in the Kaggle Iceberg Classifier Change, great job!
David: Thanks Adrian, it’s a pleasure to get to speak with you.
Adrian: How did you first become interested in computer vision and deep learning?
David: My interest in deep learning has been growing steadily over the past two years as I’ve seen how people have been using it go gain incredible insights from the data they work with. I have interest in both the active research as well as the practical application sides of deep learning, so I find competing in Kaggle competitions a great place to keep the skills sharp and to try out new techniques as they become available.
Adrian: What was your background in computer vision and machine learning/deep learning before you entered the competition? Did you compete in any previous Kaggle competitions?
David: My first exposure to machine learning goes back about 10 years when I first started learning about gradient boosted trees and random forests and applying them to classification type problems. Over the past couple of years I’ve started focusing more extensively on deep learning and computer vision.
I started competing in Kaggle competitions a little under a year ago in my spare time as a way to sharpen my skills, and this was my third image classification competition.
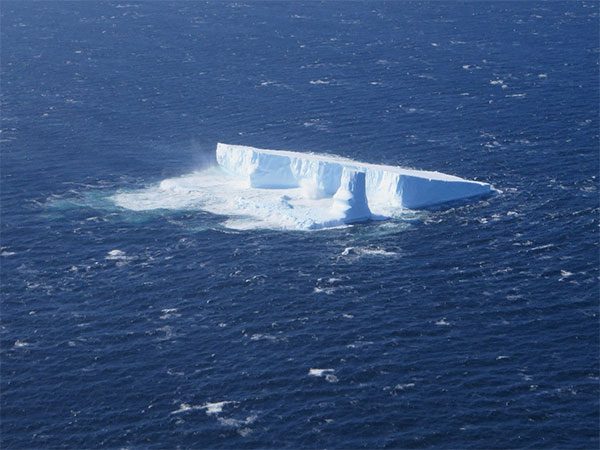
Figure 2: An example of how an iceberg looks. The goal of the Kaggle competition was to recognize such icebergs from satellite imagery (source).
Adrian: Can you tell me a bit more about the Iceberg Classifier Challenge? What motivated you to compete in it?
David: Sure, the Iceberg Classification Challenge was a binary image classification problem in which the participants were asked to classify ships vs. icebergs collected via satellite imagery. It’s especially important in the energy exploration space to be able to identify and avoid threats such as drifting icebergs.
There were a couple interesting aspects to the dataset that made this a fun challenge to work on.
First, the dataset was relatively small with only 1604 images in the training set, so the barrier to entry from a hardware perspective was pretty low, but the difficulty of working with a limited dataset was high.
Secondly, when looking at the images, to the human eye many of them look analogous to what a “snowy” TV screen looks like, just a bunch of salt and pepper noise and it was not at all visually clear which images were ships and which ones were icebergs:
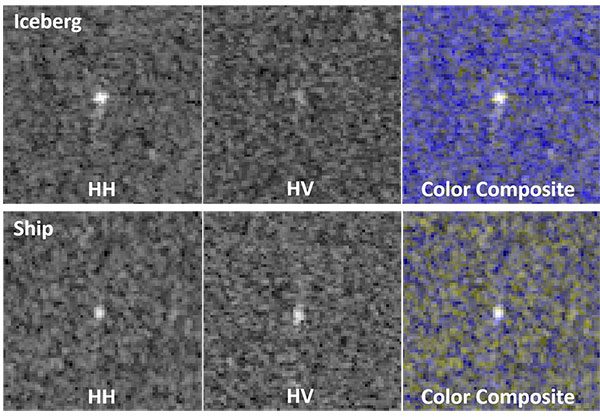
Figure 3: It’s extremely difficult for the human eye to accurately determine if an input region is an “iceberg” or a “ship” (source).
So the fact that it would be particularly difficult for a human to accurately predict the classifications, I thought it would serve as a great test to see what computer vision and deep learning could do.
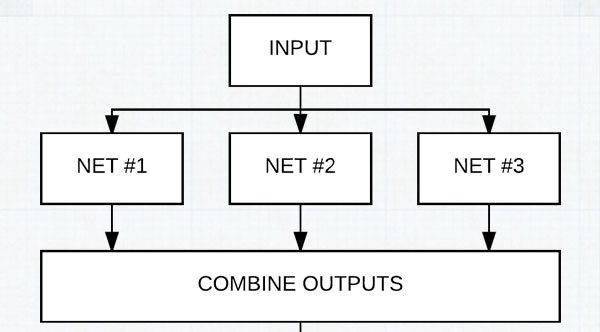
Figure 4: David and Weimin winning solution involved using an ensemble of CNN architectures.
Adrian: Let’s get a bit technical. Can you tell us a bit about the approach, algorithms, and techniques you used in your winning entry?
David: Well, the overall approach was very similar to most typical computer vision problems in that we spent quite a bit of time up front understanding the data.
One of my favorite techniques early on is to use unsupervised learning methods to identify natural patterns in the data, and use that learning to determine what deep learning approaches to take down-stream.
In this case a standard KNN algorithm was able to identify a key signal that helped define our model architecture. From there, we used a pretty extensive CNN architecture that consisted of over 100+ customized CNN’s and VGG like architectures and then combined the results from these models using both greedy blending and two-level stacking with other image features.
Now that may sound like a very complex approach, but remember that the objective function here was to minimize log loss error, and in this case we only added models in so much as they reduced log loss without overfitting, so it was another good example of the power of ensembling many weaker learners.
We ended up training many of the same CNN’s a second time but only using a subset of the data that we identified from the unsupervised learning at the beginning of the process as this also gave us an improvement in performance.
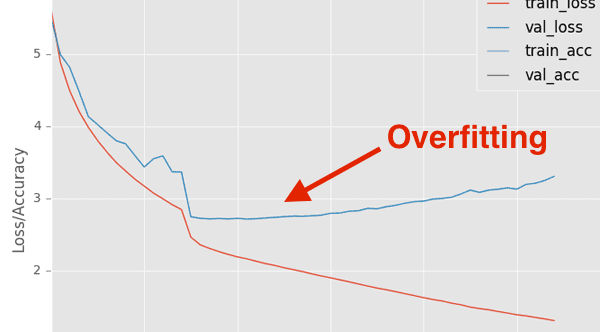
Figure 5: The most difficult aspect of the Kaggle Iceberg challenge for David and his teammate was avoiding overfitting.
Adrian: What was the most difficult aspect of the challenge for you and why?
David: The hardest part of the challenge was in validating that we weren’t overfitting.
The dataset size for an image classification problem was relatively small, so we were always worried that overfitting could be a problem. For this reason we made sure that all of our models were done using 4 fold cross validation, which adds to the computational cost, but reduces the overfitting risk. Especially when you’re dealing with an unforgiving loss function like log loss, you have to be constantly on the lookout for overfitting.
Adrian: How long did it take to train your model(s)?
David: Even with the large number of CNN’s that we chose to use, and even with using 4-fold cross validation on the entire set of models, training only took between 1-2 days. Individual models without cross validation could train in some cases on the order of minutes.
Adrian: If you had to pick the most important technique or trick you applied during the competition, what would it be?
David: Without a doubt, the most important step was the up-front exploratory analysis to give a good understanding of the dataset.
It turns out there was a very important signal in the one other feature other than the image data that helped remove a lot of noise in the data.
In my opinion one of the most overlooked steps in any CV or deep learning problem is the upfront work required to understand the data and use that knowledge to make the best design choices.
As algorithms have become more readily available and easy to import, often times there’s a rush to “throw algorithms” at a problem without really understanding if those algorithms are the right one for the job, or if there’s work that should be done before or after training to handle the data appropriately.
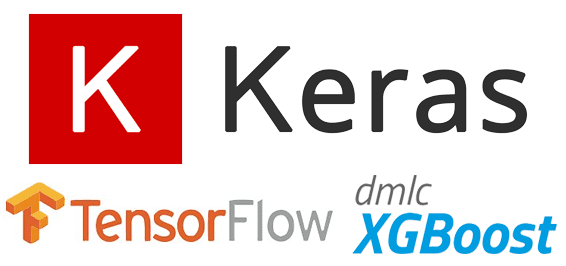
Figure 6: David used TensorFlow, Keras, and xgboost in the winning Kaggle submission.
Adrian: What are your tools and libraries of choice?
David: Personally I find Tensorflow and Keras to be amongst the most usable so when working on deep learning problems, I tend to stick to them.
For stacking and boosting, I use xgboost, again primarily due to familiarity and it’s proven results.
In this competition I used my
dl4cvvirtualenv (a Python virtual environment used inside Deep Learning for Computer Vision with Python) and added xgboost to it.
Adrian: What advice would you give to someone who wants to compete in their first Kaggle competition?
David: One of the great things about Kaggle competitions is the community nature of how the competitions work.
There’s a very rich discussion forum and way for participants to share their code if they choose to do so which is really invaluable when you’re trying to learn both general approaches as well as ways to apply code to a specific problem.
When I started on my first competition I spent hours reading through the forums and other high quality code and found it to be one of the best ways to learn.
Adrian: How did the PyImageSearch Gurus course and Deep Learning for Computer Vision with Python book prepare you for the Kaggle competition?
David: Very similar to competing in a Kaggle competition, PyImageSearch Gurus is a learn-by-doing formatted course.
To me there’s nothing that can prepare you for working on problems like actually working on problems and following high quality solutions and code, and one of the things I appreciate most about the PyImageSearch material is the way it walks you through practical solutions with production level code.
I also believe that one of the best ways to really learn and understand deep learning architectures is to read a paper and then go try to implement it.
This strategy is implemented in practice throughout the ImageNet Bundle book, and it’s the same strategy that can be used to modify and adapt architectures like we did in this competition.
I also learned about MiniGoogleNet from the Practitioner Bundle book which I hadn’t come across before and was a model that performed well in this competition.
Adrian: Would you recommend PyImageSearch Gurus or Deep Learning for Computer Vision with Python to other developers, researchers, and students trying to learn computer vision + deep learning?
David: Absolutely. I would recommend it to anyone who’s looking to establish a strong foundation in CV and deep learning because you’re not only going to learn the principals, but you’re going to learn how to quickly apply your learning to real-world problems using the most popular and up to date tools and SW.
Adrian: What’s next?
David: Well, I’ve got a pretty big pipeline of projects I want to work on lined up so I’m going to be busy for a while. There are a couple other Kaggle competitions that look like really fun challenges to work on so there’s a good chance I’ll jump back into those too.
Adrian: If a PyImageSearch reader wants to chat, what is the best place to connect with you?
David: The best way to reach me is my LinkedIn profile. You can connect with Weimin Wang on LinkedIn as well. I’ll also be attending PyImageConf 2018 in August if you want to chat in person.
What about you? Are you ready to follow in the footsteps of David?
Are you ready start your journey to computer vision + deep learning mastery and follow in the footsteps of David Austin?
David is a long-time PyImageSearch reader and has worked through both:
- The PyImageSearch Gurus course, an in-depth treatment of computer vision and image processing
- Deep Learning for Computer Vision with Python, the most comprehensive computer vision + deep learning book available today
I can’t promise you’ll win a Kaggle competition like David has, but I can guarantee that these are the two best resources available today to master computer vision and deep learning.
To quote Stephen Caldara, a Sr. Systems Engineer at Amazon Robotics:
I am very pleased with the [PyImageSearch Gurus] content you have created. I would rate it easily at a university ‘masters program’ level. And better organized.
Along with Adam Geitgey, author of the popular Machine Learning is Fun! blog series:
I highly recommend grabbing a copy of Deep Learning for Computer Vision with Python. It goes into a lot of detail and has tons of detailed examples. It’s the only book I’ve seen so far that covers both how things work and how to actually use them in the real world to solve difficult problems. Check it out!
Give the course and book a try — I’ll be there to help you every step of the way.
Summary
In today’s blog post, I interviewed David Austin, who, with his teammate, Weimin Wang, won first place (and $25,000) in Kaggle’s Iceberg Classifier Challenge.
David and Weimin’s hard work will help ensure safer, less hazardous travel through iceberg-prone waters.
I am so incredibly happy (and proud) for David and Weimin. Please join me and congratulate them in the comments section of this blog post.
The post An interview with David Austin: 1st place and $25,000 in Kaggle’s most popular image classification competition appeared first on PyImageSearch.
from PyImageSearch https://ift.tt/2G9cejF
via IFTTT