Latest YouTube Video
Saturday, January 16, 2016
Marcha Fúnebre Saudades (Anonymous)
from Google Alert - anonymous http://ift.tt/1Q61Dpj
via IFTTT
Ravens: Ted Marchibroda, the only man to be head coach of both NFL franchises in Baltimore, dies at age 84 (ESPN)
via IFTTT
Next Hacker to Organize Biggest Java Programming Competition In Germany
from The Hacker News http://ift.tt/1PinsyL
via IFTTT
Ocean City, MD's surf is Good
Ocean City, MD Summary
Surf: waist to shoulder high
Maximum: 1.224m (4.02ft)
Minimum: 0.918m (3.01ft)
Maryland-Delaware Summary
from Surfline http://ift.tt/1kVmigH
via IFTTT
Approaches to anonymous feature engineering?
from Google Alert - anonymous http://ift.tt/1SUdZDB
via IFTTT
SportsCenter Video: Tim Kurkjian says Chris Davis' \"love\" for Baltimore is ultimately what pushed him to re-sign (ESPN)
via IFTTT
[FD] Correct answer Information Disclosure in TCExam <= 12.2.5
Source: Gmail -> IFTTT-> Blogger
Orioles: 1B Chris Davis' deal to return to Baltimore is worth $161 million over 7 years, according to MLB Network (ESPN)
via IFTTT
MLB: 1B Chris Davis to re-sign with the Orioles - MLB Network; led ML in HR (47) for 2nd time in last 3 seasons in 2015 (ESPN)
via IFTTT
Casino Sues Cyber Security Company Over Failure to Stop Hackers
from The Hacker News http://ift.tt/1RrEhxs
via IFTTT
Apple's Mac OS X Still Open to Malware, Thanks Gatekeeper
from The Hacker News http://ift.tt/1QcLkJf
via IFTTT
Wright Mons in Color
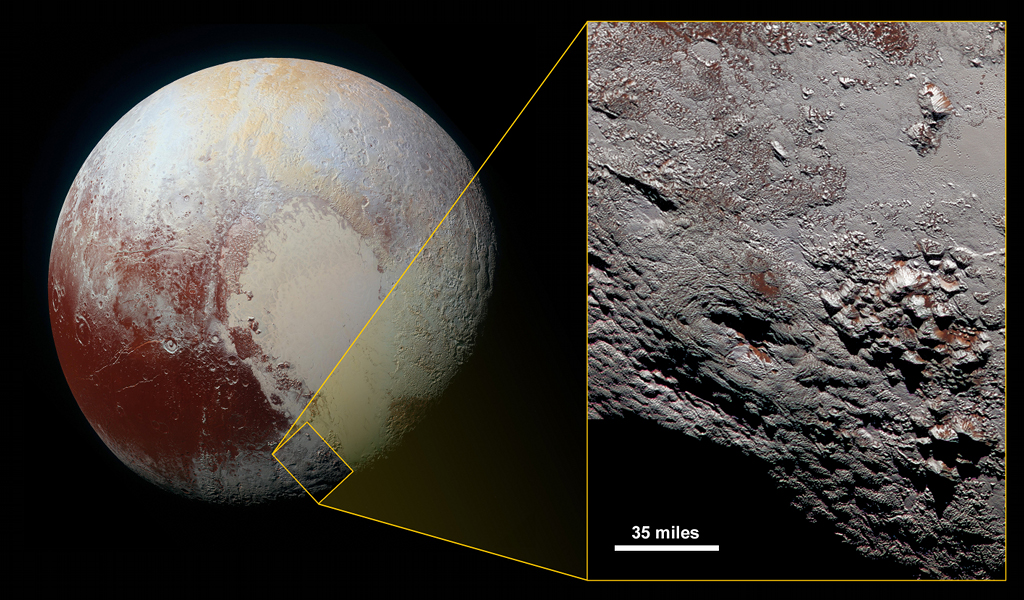
IMERG Rainfall Accumulation over the United States for December 2015
from NASA's Scientific Visualization Studio: Most Recent Items http://ift.tt/1KiDlnz
via IFTTT
Friday, January 15, 2016
Orioles: 3B Manny Machado agrees to a 1-year, $5 million deal to avoid arbitration according to multiple reports (ESPN)
via IFTTT
Re: [FD] Combining DLL hijacking with USB keyboard emulation
Source: Gmail -> IFTTT-> Blogger
[FD] CCA on CoreProc/crypto-guard and an Appeal to PHP Programmers
Source: Gmail -> IFTTT-> Blogger
[FD] Whatever happened with CVE-2015-0072?
Source: Gmail -> IFTTT-> Blogger
[FD] FreeBSD bsnmpd information disclosure
Source: Gmail -> IFTTT-> Blogger
[FD] Qualys Security Advisory - Roaming through the OpenSSH client: CVE-2016-0777 and CVE-2016-0778
Source: Gmail -> IFTTT-> Blogger
[FD] [TOOL] The Metabrik Platform
Source: Gmail -> IFTTT-> Blogger
[FD] [KIS-2016-01] CakePHP <= 3.2.0 "_method" CSRF Protection Bypass Vulnerability
Source: Gmail -> IFTTT-> Blogger
Orioles: Team's offer to Yoenis Cespedes is yet another sign Baltimore is done with 1B Chris Davis, says Eddie Matz (ESPN)
via IFTTT
Anonymous function is from which version existing?
from Google Alert - anonymous http://ift.tt/1OTzxOV
via IFTTT
ISS Daily Summary Report – 1/14/16
from ISS On-Orbit Status Report http://ift.tt/1J6L3Gq
via IFTTT
Creator of MegalodonHTTP DDoS Botnet Arrested
from The Hacker News http://ift.tt/233YAoM
via IFTTT
Re: [psutil] Add basic NetBSD support. (#557)
Source: Gmail -> IFTTT-> Blogger
Critical OpenSSH Flaw Leaks Private Crypto Keys to Hackers
from The Hacker News http://ift.tt/233RXma
via IFTTT
http://ift.tt/fvZjIzanonymous/a14be256861899d01c43 | Parchment - College admissions ...
from Google Alert - anonymous http://ift.tt/1WdM9lw
via IFTTT
Infrared Portrait of the Large Magellanic Cloud
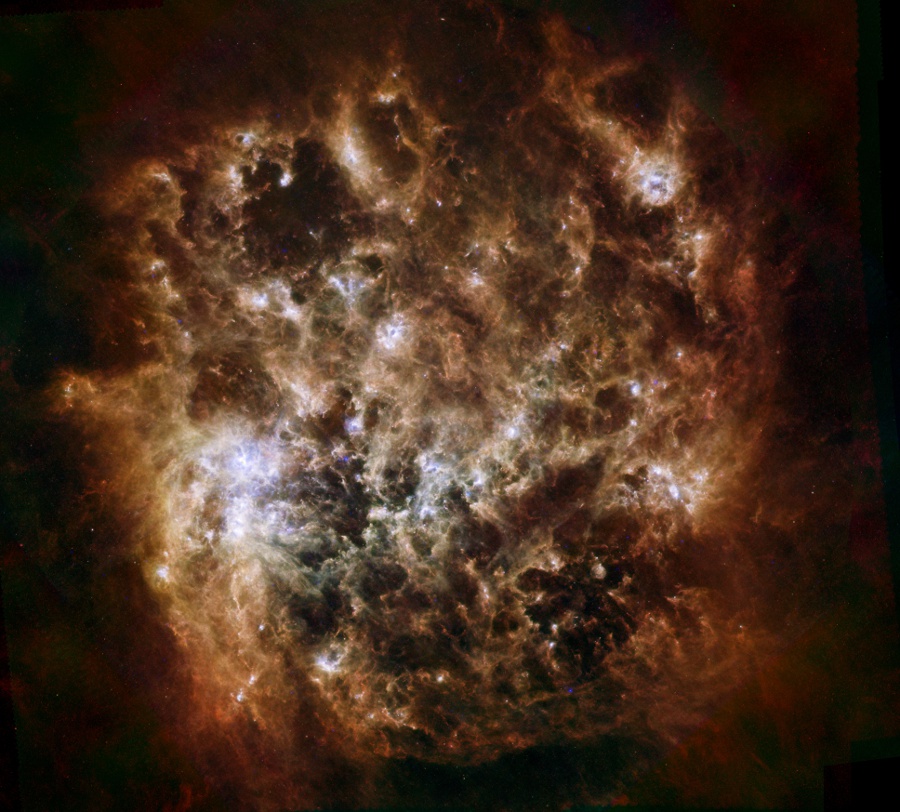
Thursday, January 14, 2016
Analysis of Algorithms and Partial Algorithms. (arXiv:1601.03411v1 [cs.AI])
We present an alternative methodology for the analysis of algorithms, based on the concept of expected discounted reward. This methodology naturally handles algorithms that do not always terminate, so it can (theoretically) be used with partial algorithms for undecidable problems, such as those found in artificial general intelligence (AGI) and automated theorem proving. We mention new approaches to self-improving AGI and logical uncertainty enabled by this methodology.
from cs.AI updates on arXiv.org http://ift.tt/1lbZZHF
via IFTTT
Paisley Schottisch (Anonymous)
from Google Alert - anonymous http://ift.tt/1RRzy6Q
via IFTTT
I have a new follower on Twitter
Impellus
The UK's most influential ILM Accredited Leadership & Management Training Centre. Open courses at 15 venues nationwide. Specialist Business Performance Training
London, St Albans, UK Delivery
http://t.co/TgY76SCqau
Following: 1655 - Followers: 1948
January 14, 2016 at 08:07PM via Twitter http://twitter.com/ImpellusUK
Orioles Buzz: OF Yoenis Cespedes receives offer from team; no financial details available - report; 35 HR last season (ESPN)
via IFTTT
Add the option for specify anonymous email and name
from Google Alert - anonymous http://ift.tt/1J5FzvF
via IFTTT
Mel Kiper's Mock Draft 1.0: Ravens select Notre Dame OT Ronnie Stanley No. 6 overall; last picked 1st-round OT in 2009 (ESPN)
via IFTTT
Orioles: Mark Trumbo agrees to $9.15M deal to avoid salary arbitration - Jerry Crasnick; acquired Dec. 2 from Mariners (ESPN)
via IFTTT
Asian Couples Seek Anonymous Asian Donors. Make $7000+ SO Much More!!
from Google Alert - anonymous http://ift.tt/1Skdu6h
via IFTTT
ISS Daily Summary Report – 1/13/16
from ISS On-Orbit Status Report http://ift.tt/1OR6Ou1
via IFTTT
Conan Has Angered "Anonymous"
from Google Alert - anonymous http://ift.tt/1URspmv
via IFTTT
Hacker group Anonymous declares war on Thai police over British backpacker murders
from Google Alert - anonymous http://ift.tt/1RnapSY
via IFTTT
A SNEAK PEEK AT DARK KNIGHT UNIVERSE PRESENTS
from Google Alert - anonymous http://ift.tt/1nkt2dH
via IFTTT
Reflections on the 1970s
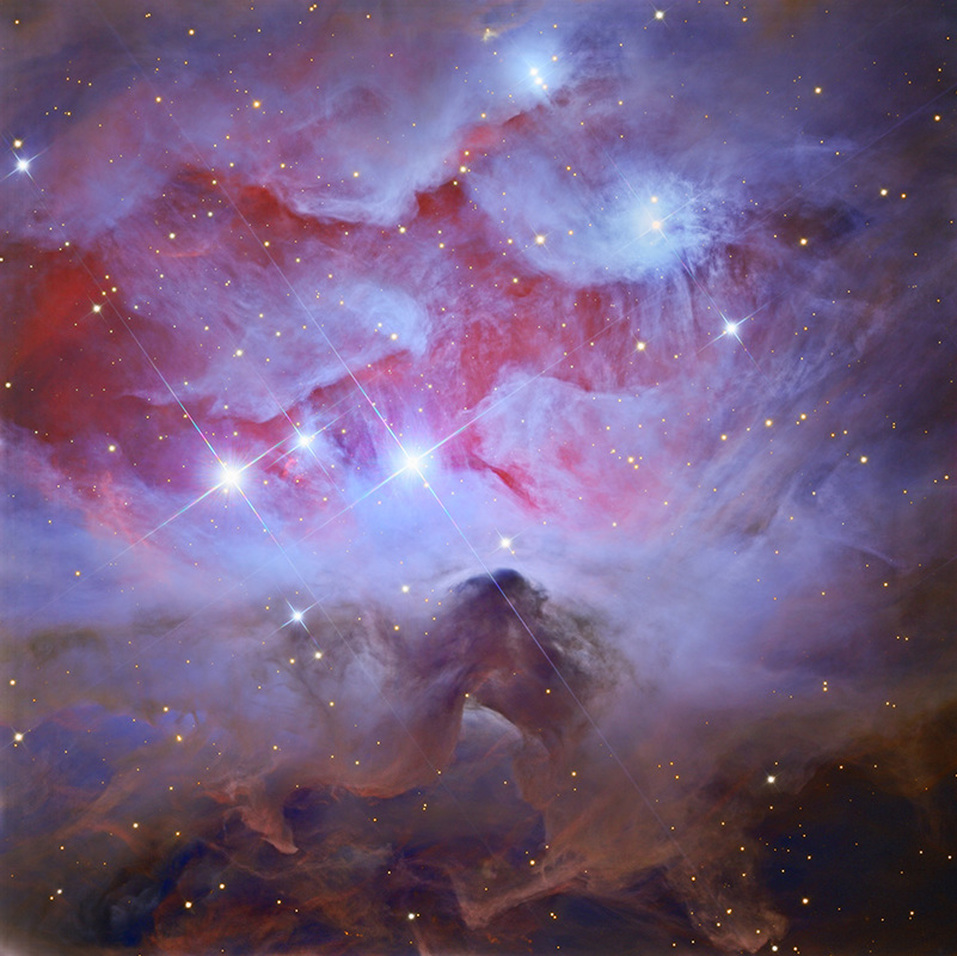
Wednesday, January 13, 2016
Helias tuition costs drop, thanks to anonymous donor
from Google Alert - anonymous http://ift.tt/1JLECsb
via IFTTT
An Application of the Generalized Rectangular Fuzzy Model to Critical Thinking Assessment. (arXiv:1601.03065v1 [cs.AI])
The authors apply the Generalized Rectangular Model to assessing critical thinking skills and its relations with their language competency.
from cs.AI updates on arXiv.org http://ift.tt/1RmsLDB
via IFTTT
Submodular Optimization under Noise. (arXiv:1601.03095v1 [cs.DS])
We consider the problem of maximizing monotone submodular functions under noise, which to the best of our knowledge has not been studied in the past. There has been a great deal of work on optimization of submodular functions under various constraints, with many algorithms that provide desirable approximation guarantees. However, in many applications we do not have access to the submodular function we aim to optimize, but rather to some erroneous or noisy version of it. This raises the question of whether provable guarantees are obtainable in presence of error and noise. We provide initial answers, by focusing on the question of maximizing a monotone submodular function under cardinality constraints when given access to a noisy oracle of the function. We show that:
For a cardinality constraint $k \geq 2$, there is an approximation algorithm whose approximation ratio is arbitrarily close to $1-1/e$;
For $k=1$ there is an approximation algorithm whose approximation ratio is arbitrarily close to $1/2$ in expectation. No randomized algorithm can obtain an approximation ratio in expectation better than $1/2+O(1/\sqrt n)$ and $(2k - 1)/2k + O(1/\sqrt{n})$ for general $k$;
If the noise is adversarial, no non-trivial approximation guarantee can be obtained.
from cs.AI updates on arXiv.org http://ift.tt/1PYTPpp
via IFTTT
Online Prediction of Dyadic Data with Heterogeneous Matrix Factorization. (arXiv:1601.03124v1 [cs.AI])
Dyadic Data Prediction (DDP) is an important problem in many research areas. This paper develops a novel fully Bayesian nonparametric framework which integrates two popular and complementary approaches, discrete mixed membership modeling and continuous latent factor modeling into a unified Heterogeneous Matrix Factorization~(HeMF) model, which can predict the unobserved dyadics accurately. The HeMF can determine the number of communities automatically and exploit the latent linear structure for each bicluster efficiently. We propose a Variational Bayesian method to estimate the parameters and missing data. We further develop a novel online learning approach for Variational inference and use it for the online learning of HeMF, which can efficiently cope with the important large-scale DDP problem. We evaluate the performance of our method on the EachMoive, MovieLens and Netflix Prize collaborative filtering datasets. The experiment shows that, our model outperforms state-of-the-art methods on all benchmarks. Compared with Stochastic Gradient Method (SGD), our online learning approach achieves significant improvement on the estimation accuracy and robustness.
from cs.AI updates on arXiv.org http://ift.tt/1RmsLDx
via IFTTT
How to stay anonymous if you win the $1.5B POWERBALL
from Google Alert - anonymous http://ift.tt/1mWCCTB
via IFTTT
[FD] EasyDNNnews Reflected XSS
Source: Gmail -> IFTTT-> Blogger
How to Hack WiFi Password from Smart Doorbells
from The Hacker News http://ift.tt/1ninjVF
via IFTTT
ISS Daily Summary Report – 1/12/16
from ISS On-Orbit Status Report http://ift.tt/1PWRVWb
via IFTTT
Someone Just Leaked Hard-Coded Password Backdoor for Fortinet Firewalls
from The Hacker News http://ift.tt/1W6FVnj
via IFTTT
US Intelligence Chief Hacked by the Teen Who Hacked CIA Director
from The Hacker News http://ift.tt/1Oqn6Xc
via IFTTT
The California Nebula
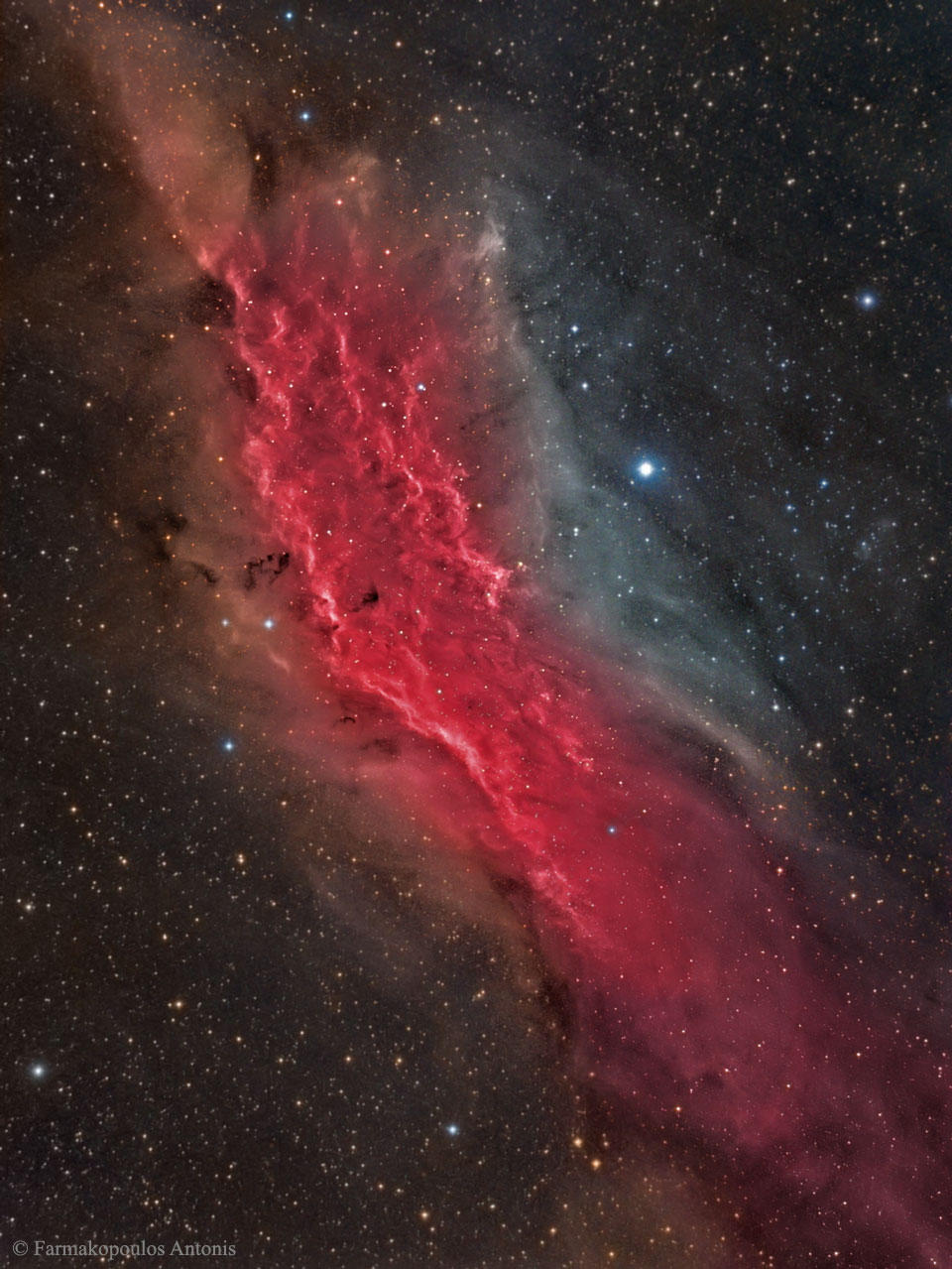
Tuesday, January 12, 2016
Phys. Rev. A 93, 012318
from Google Alert - anonymous http://ift.tt/1PVZxZ6
via IFTTT
Inference rules for RDF(S) and OWL in N3Logic. (arXiv:1601.02650v1 [cs.DB])
This paper presents inference rules for Resource Description Framework (RDF), RDF Schema (RDFS) and Web Ontology Language (OWL). Our formalization is based on Notation 3 Logic, which extended RDF by logical symbols and created Semantic Web logic for deductive RDF graph stores. We also propose OWL-P that is a lightweight formalism of OWL and supports soft inferences by omitting complex language constructs.
from cs.AI updates on arXiv.org http://ift.tt/1mTZset
via IFTTT
Robobarista: Learning to Manipulate Novel Objects via Deep Multimodal Embedding. (arXiv:1601.02705v1 [cs.RO])
There is a large variety of objects and appliances in human environments, such as stoves, coffee dispensers, juice extractors, and so on. It is challenging for a roboticist to program a robot for each of these object types and for each of their instantiations. In this work, we present a novel approach to manipulation planning based on the idea that many household objects share similarly-operated object parts. We formulate the manipulation planning as a structured prediction problem and learn to transfer manipulation strategy across different objects by embedding point-cloud, natural language, and manipulation trajectory data into a shared embedding space using a deep neural network. In order to learn semantically meaningful spaces throughout our network, we introduce a method for pre-training its lower layers for multimodal feature embedding and a method for fine-tuning this embedding space using a loss-based margin. In order to collect a large number of manipulation demonstrations for different objects, we develop a new crowd-sourcing platform called Robobarista. We test our model on our dataset consisting of 116 objects and appliances with 249 parts along with 250 language instructions, for which there are 1225 crowd-sourced manipulation demonstrations. We further show that our robot with our model can even prepare a cup of a latte with appliances it has never seen before.
from cs.AI updates on arXiv.org http://ift.tt/1TTDpPX
via IFTTT
Basic Reasoning with Tensor Product Representations. (arXiv:1601.02745v1 [cs.AI])
In this paper we present the initial development of a general theory for mapping inference in predicate logic to computation over Tensor Product Representations (TPRs; Smolensky (1990), Smolensky & Legendre (2006)). After an initial brief synopsis of TPRs (Section 0), we begin with particular examples of inference with TPRs in the 'bAbI' question-answering task of Weston et al. (2015) (Section 1). We then present a simplification of the general analysis that suffices for the bAbI task (Section 2). Finally, we lay out the general treatment of inference over TPRs (Section 3). We also show the simplification in Section 2 derives the inference methods described in Lee et al. (2016); this shows how the simple methods of Lee et al. (2016) can be formally extended to more general reasoning tasks.
from cs.AI updates on arXiv.org http://ift.tt/1SNvdCv
via IFTTT
Essence' Description. (arXiv:1601.02865v1 [cs.AI])
A description of the Essence' language as used by the tool Savile Row.
from cs.AI updates on arXiv.org http://ift.tt/1mTZpzw
via IFTTT
The minimal hitting set generation problem: algorithms and computation. (arXiv:1601.02939v1 [cs.DS])
Finding inclusion-minimal "hitting sets" for a given collection of sets is a fundamental combinatorial problem with applications in domains as diverse as Boolean algebra, computational biology, and data mining. Much of the algorithmic literature focuses on the problem of *recognizing* the collection of minimal hitting sets; however, in many of the applications, it is more important to *generate* these hitting sets. We survey twenty algorithms from across a variety of domains, considering their history, classification, useful features, and computational performance on a variety of synthetic and real-world inputs. We also provide a suite of implementations of these algorithms with a ready-to-use, platform-agnostic interface based on Docker containers and the AlgoRun framework, so that interested computational scientists can easily perform similar tests with inputs from their own research areas on their own computers or through a convenient Web interface.
from cs.AI updates on arXiv.org http://ift.tt/1SNvfdy
via IFTTT
Indicators of Good Student Performance in Moodle Activity Data. (arXiv:1601.02975v1 [cs.CY])
In this paper we conduct an analysis of Moodle activity data focused on identifying early predictors of good student performance. The analysis shows that three relevant hypotheses are largely supported by the data. These hypotheses are: early submission is a good sign, a high level of activity is predictive of good results and evening activity is even better than daytime activity. We highlight some pathological examples where high levels of activity correlates with bad results.
from cs.AI updates on arXiv.org http://ift.tt/1mTZpiU
via IFTTT
Mapping-equivalence and oid-equivalence of single-function object-creating conjunctive queries. (arXiv:1503.01707v2 [cs.DB] UPDATED)
Conjunctive database queries have been extended with a mechanism for object creation to capture important applications such as data exchange, data integration, and ontology-based data access. Object creation generates new object identifiers in the result, that do not belong to the set of constants in the source database. The new object identifiers can be also seen as Skolem terms. Hence, object-creating conjunctive queries can also be regarded as restricted second-order tuple-generating dependencies (SO tgds), considered in the data exchange literature.
In this paper, we focus on the class of single-function object-creating conjunctive queries, or sifo CQs for short. We give a new characterization for oid-equivalence of sifo CQs that is simpler than the one given by Hull and Yoshikawa and places the problem in the complexity class NP. Our characterization is based on Cohen's equivalence notions for conjunctive queries with multiplicities. We also solve the logical entailment problem for sifo CQs, showing that also this problem belongs to NP. Results by Pichler et al. have shown that logical equivalence for more general classes of SO tgds is either undecidable or decidable with as yet unknown complexity upper bounds.
from cs.AI updates on arXiv.org http://ift.tt/1FgRrUs
via IFTTT
Anonymous tip leads to drug arrests
from Google Alert - anonymous http://ift.tt/1Sh4RcN
via IFTTT
Confirmation Not Accessible to Anonymous User?
from Google Alert - anonymous http://ift.tt/1mVmLp5
via IFTTT
Quant voi le douz tens bel et cler (Anonymous)
from Google Alert - anonymous http://ift.tt/1mVmLoQ
via IFTTT
'Ridiculous' Bug in Popular Antivirus Allows Hackers to Steal all Your Passwords
from The Hacker News http://ift.tt/1P7NSDl
via IFTTT
Order revision shows Anonymous at order completion phase
from Google Alert - anonymous http://ift.tt/1ZitRPU
via IFTTT
Transformation and Recovery: Spiritual Implications of the Alcoholics Anonymous Twelve-Step ...
from Google Alert - anonymous http://ift.tt/1J11kwA
via IFTTT
ISS Daily Summary Report – 1/11/16
from ISS On-Orbit Status Report http://ift.tt/1ZYfenf
via IFTTT
From Today Onwards, Don't You Even Dare to Use Microsoft Internet Explorer
from The Hacker News http://ift.tt/1RjGXgA
via IFTTT
Simple Yet Effective eBay Bug Allows Hackers to Steal Passwords
from The Hacker News http://ift.tt/1OMRVJk
via IFTTT
[FD] SEC Consult whitepaper: Bypassing McAfee Application Whitelisting for Critical Infrastructure Systems
Source: Gmail -> IFTTT-> Blogger
A Colorful Solar Corona over the Himalayas
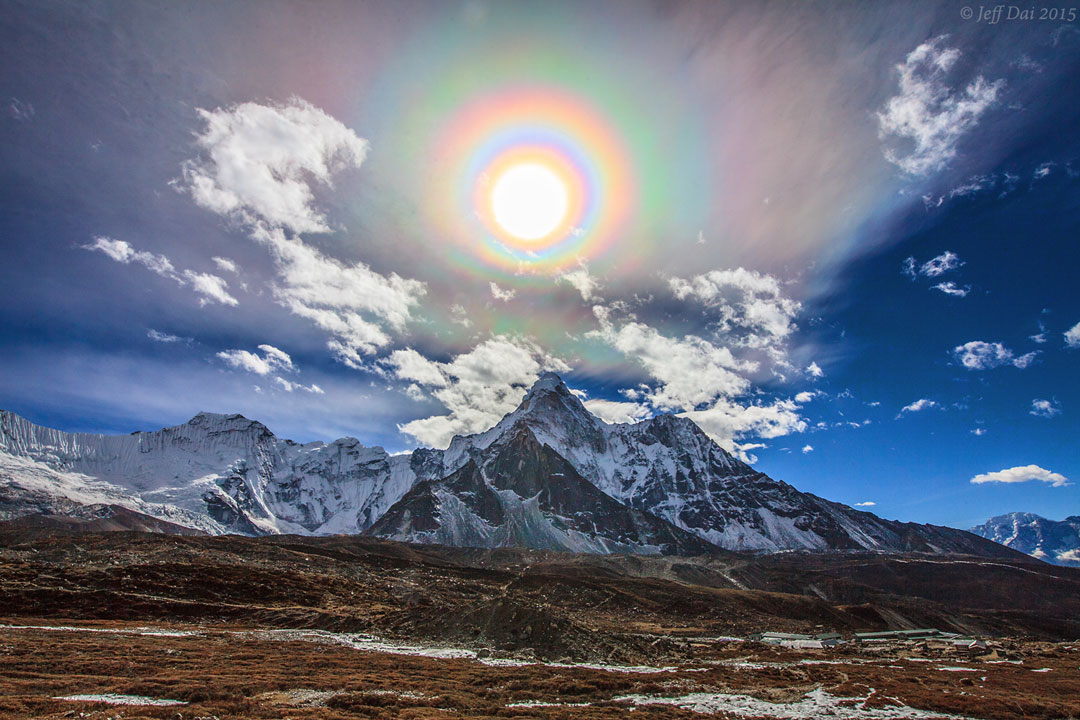
Monday, January 11, 2016
Re: [FD] Executable installers are vulnerable^WEVIL (case 20): TrueCrypt's installers allow arbitrary (remote) code execution and escalation of privilege
Source: Gmail -> IFTTT-> Blogger
same cache for anonymous and authentificated user
from Google Alert - anonymous http://ift.tt/1OeVWVv
via IFTTT
Identifying Stable Patterns over Time for Emotion Recognition from EEG. (arXiv:1601.02197v1 [cs.HC])
In this paper, we investigate stable patterns of electroencephalogram (EEG) over time for emotion recognition using a machine learning approach. Up to now, various findings of activated patterns associated with different emotions have been reported. However, their stability over time has not been fully investigated yet. In this paper, we focus on identifying EEG stability in emotion recognition. To validate the efficiency of the machine learning algorithms used in this study, we systematically evaluate the performance of various popular feature extraction, feature selection, feature smoothing and pattern classification methods with the DEAP dataset and a newly developed dataset for this study. The experimental results indicate that stable patterns exhibit consistency across sessions; the lateral temporal areas activate more for positive emotion than negative one in beta and gamma bands; the neural patterns of neutral emotion have higher alpha responses at parietal and occipital sites; and for negative emotion, the neural patterns have significant higher delta responses at parietal and occipital sites and higher gamma responses at prefrontal sites. The performance of our emotion recognition system shows that the neural patterns are relatively stable within and between sessions.
from cs.AI updates on arXiv.org http://ift.tt/1OeGkkR
via IFTTT
On Clustering Time Series Using Euclidean Distance and Pearson Correlation. (arXiv:1601.02213v1 [cs.LG])
For time series comparisons, it has often been observed that z-score normalized Euclidean distances far outperform the unnormalized variant. In this paper we show that a z-score normalized, squared Euclidean Distance is, in fact, equal to a distance based on Pearson Correlation. This has profound impact on many distance-based classification or clustering methods. In addition to this theoretically sound result we also show that the often used k-Means algorithm formally needs a mod ification to keep the interpretation as Pearson correlation strictly valid. Experimental results demonstrate that in many cases the standard k-Means algorithm generally produces the same results.
from cs.AI updates on arXiv.org http://ift.tt/1Ka9NbA
via IFTTT
A Synthetic Approach for Recommendation: Combining Ratings, Social Relations, and Reviews. (arXiv:1601.02327v1 [cs.IR])
Recommender systems (RSs) provide an effective way of alleviating the information overload problem by selecting personalized choices. Online social networks and user-generated content provide diverse sources for recommendation beyond ratings, which present opportunities as well as challenges for traditional RSs. Although social matrix factorization (Social MF) can integrate ratings with social relations and topic matrix factorization can integrate ratings with item reviews, both of them ignore some useful information. In this paper, we investigate the effective data fusion by combining the two approaches, in two steps. First, we extend Social MF to exploit the graph structure of neighbors. Second, we propose a novel framework MR3 to jointly model these three types of information effectively for rating prediction by aligning latent factors and hidden topics. We achieve more accurate rating prediction on two real-life datasets. Furthermore, we measure the contribution of each data source to the proposed framework.
from cs.AI updates on arXiv.org http://ift.tt/1JGDp5p
via IFTTT
Git4Voc: Git-based Versioning for Collaborative Vocabulary Development. (arXiv:1601.02433v1 [cs.AI])
Collaborative vocabulary development in the context of data integration is the process of finding consensus between the experts of the different systems and domains. The complexity of this process is increased with the number of involved people, the variety of the systems to be integrated and the dynamics of their domain. In this paper we advocate that the realization of a powerful version control system is the heart of the problem. Driven by this idea and the success of Git in the context of software development, we investigate the applicability of Git for collaborative vocabulary development. Even though vocabulary development and software development have much more similarities than differences there are still important differences. These need to be considered within the development of a successful versioning and collaboration system for vocabulary development. Therefore, this paper starts by presenting the challenges we were faced with during the creation of vocabularies collaboratively and discusses its distinction to software development. Based on these insights we propose Git4Voc which comprises guidelines how Git can be adopted to vocabulary development. Finally, we demonstrate how Git hooks can be implemented to go beyond the plain functionality of Git by realizing vocabulary-specific features like syntactic validation and semantic diffs.
from cs.AI updates on arXiv.org http://ift.tt/1JGDoP4
via IFTTT
Evaluating the Performance of a Speech Recognition based System. (arXiv:1601.02543v1 [cs.CL])
Speech based solutions have taken center stage with growth in the services industry where there is a need to cater to a very large number of people from all strata of the society. While natural language speech interfaces are the talk in the research community, yet in practice, menu based speech solutions thrive. Typically in a menu based speech solution the user is required to respond by speaking from a closed set of words when prompted by the system. A sequence of human speech response to the IVR prompts results in the completion of a transaction. A transaction is deemed successful if the speech solution can correctly recognize all the spoken utterances of the user whenever prompted by the system. The usual mechanism to evaluate the performance of a speech solution is to do an extensive test of the system by putting it to actual people use and then evaluating the performance by analyzing the logs for successful transactions. This kind of evaluation could lead to dissatisfied test users especially if the performance of the system were to result in a poor transaction completion rate. To negate this the Wizard of Oz approach is adopted during evaluation of a speech system. Overall this kind of evaluations is an expensive proposition both in terms of time and cost. In this paper, we propose a method to evaluate the performance of a speech solution without actually putting it to people use. We first describe the methodology and then show experimentally that this can be used to identify the performance bottlenecks of the speech solution even before the system is actually used thus saving evaluation time and expenses.
from cs.AI updates on arXiv.org http://ift.tt/1P5b5G3
via IFTTT
Clustering Markov Decision Processes For Continual Transfer. (arXiv:1311.3959v2 [cs.AI] UPDATED)
We present algorithms to effectively represent a set of Markov decision processes (MDPs), whose optimal policies have already been learned, by a smaller source subset for lifelong, policy-reuse-based transfer learning in reinforcement learning. This is necessary when the number of previous tasks is large and the cost of measuring similarity counteracts the benefit of transfer. The source subset forms an `$\epsilon$-net' over the original set of MDPs, in the sense that for each previous MDP $M_p$, there is a source $M^s$ whose optimal policy has $<\epsilon$ regret in $M_p$. Our contributions are as follows. We present EXP-3-Transfer, a principled policy-reuse algorithm that optimally reuses a given source policy set when learning for a new MDP. We present a framework to cluster the previous MDPs to extract a source subset. The framework consists of (i) a distance $d_V$ over MDPs to measure policy-based similarity between MDPs; (ii) a cost function $g(\cdot)$ that uses $d_V$ to measure how good a particular clustering is for generating useful source tasks for EXP-3-Transfer and (iii) a provably convergent algorithm, MHAV, for finding the optimal clustering. We validate our algorithms through experiments in a surveillance domain.
from cs.AI updates on arXiv.org http://ift.tt/1hQHbuA
via IFTTT
What to talk about and how? Selective Generation using LSTMs with Coarse-to-Fine Alignment. (arXiv:1509.00838v2 [cs.CL] UPDATED)
We propose an end-to-end, domain-independent neural encoder-aligner-decoder model for selective generation, i.e., the joint task of content selection and surface realization. Our model first encodes a full set of over-determined database event records via an LSTM-based recurrent neural network, then utilizes a novel coarse-to-fine aligner to identify the small subset of salient records to talk about, and finally employs a decoder to generate free-form descriptions of the aligned, selected records. Our model achieves the best selection and generation results reported to-date (with 59% relative improvement in generation) on the benchmark WeatherGov dataset, despite using no specialized features or linguistic resources. Using an improved k-nearest neighbor beam filter helps further. We also perform a series of ablations and visualizations to elucidate the contributions of our key model components. Lastly, we evaluate the generalizability of our model on the RoboCup dataset, and get results that are competitive with or better than the state-of-the-art, despite being severely data-starved.
from cs.AI updates on arXiv.org http://ift.tt/1LM9FRU
via IFTTT
Client Profiling for an Anti-Money Laundering System. (arXiv:1510.00878v2 [cs.LG] UPDATED)
We present a data mining approach for profiling bank clients in order to support the process of detection of anti-money laundering operations. We first present the overall system architecture, and then focus on the relevant component for this paper. We detail the experiments performed on real world data from a financial institution, which allowed us to group clients in clusters and then generate a set of classification rules. We discuss the relevance of the founded client profiles and of the generated classification rules. According to the defined overall agent-based architecture, these rules will be incorporated in the knowledge base of the intelligent agents responsible for the signaling of suspicious transactions.
from cs.AI updates on arXiv.org http://ift.tt/1VCaK1z
via IFTTT
Highway Long Short-Term Memory RNNs for Distant Speech Recognition. (arXiv:1510.08983v2 [cs.NE] UPDATED)
In this paper, we extend the deep long short-term memory (DLSTM) recurrent neural networks by introducing gated direct connections between memory cells in adjacent layers. These direct links, called highway connections, enable unimpeded information flow across different layers and thus alleviate the gradient vanishing problem when building deeper LSTMs. We further introduce the latency-controlled bidirectional LSTMs (BLSTMs) which can exploit the whole history while keeping the latency under control. Efficient algorithms are proposed to train these novel networks using both frame and sequence discriminative criteria. Experiments on the AMI distant speech recognition (DSR) task indicate that we can train deeper LSTMs and achieve better improvement from sequence training with highway LSTMs (HLSTMs). Our novel model obtains $43.9/47.7\%$ WER on AMI (SDM) dev and eval sets, outperforming all previous works. It beats the strong DNN and DLSTM baselines with $15.7\%$ and $5.3\%$ relative improvement respectively.
from cs.AI updates on arXiv.org http://ift.tt/1k3dOIR
via IFTTT
Deep Reinforcement Learning with an Unbounded Action Space. (arXiv:1511.04636v3 [cs.AI] UPDATED)
In this paper, we propose the deep reinforcement relevance network (DRRN), a novel deep architecture, to design a better model for handling an unbounded action space with applications to language understanding for text-based games. For a particular class of games, a user must choose among a variable number of actions described by text, with the goal of maximizing long-term reward. In these games, the best action is typically that which best fits to the current situation (modeled as a state in the DRRN), also described by text. Because of the exponential complexity of natural language with respect to sentence length, there is typically an unbounded set of unique actions. Therefore, it is difficult to pre-define the action set. To address this challenge, the DRRN extracts separate high-level embedding vectors from the texts that describe states and actions, respectively, using a general interaction function, exploring inner product, bilinear, and DNN interaction, between these embedding vectors to approximate the Q-function. We evaluate the DRRN on two popular text games, showing superior performance over other deep Q-learning architectures.
from cs.AI updates on arXiv.org http://ift.tt/1MiWHMd
via IFTTT
Diego Mejia Ospina (@DiegoMejiaO) retweeted your Tweet!
Source: Gmail -> IFTTT-> Blogger
Javascript Digest (@javascriptd) retweeted your Tweet!
Source: Gmail -> IFTTT-> Blogger
Re: [FD] Combining DLL hijacking with USB keyboard emulation
Source: Gmail -> IFTTT-> Blogger
[FD] New BlackArch Linux ISOs (2016.01.10) released
Source: Gmail -> IFTTT-> Blogger
[FD] CVE-2015-8397: GDCM out-of-bounds read in JPEGLSCodec::DecodeExtent
Source: Gmail -> IFTTT-> Blogger
[FD] CVE-2015-8396: GDCM buffer overflow in ImageRegionReader::ReadIntoBuffer
Source: Gmail -> IFTTT-> Blogger
[FD] Linux user namespaces overlayfs local root
Source: Gmail -> IFTTT-> Blogger
[FD] Exploiting XXE vulnerabilities in AMF libraries
Source: Gmail -> IFTTT-> Blogger
I have a new follower on Twitter
Concussion News
Sharing news and insights on the available treatments for concussions.
USA
http://t.co/jhmdUZjvDu
Following: 3736 - Followers: 3192
January 11, 2016 at 05:20PM via Twitter http://twitter.com/concussion_news
[FD] Google Chrome - Javascript Execution Via Default Search Engines
Source: Gmail -> IFTTT-> Blogger
Passing variables to an anonymous function when using fminbnd
from Google Alert - anonymous http://ift.tt/1RivGNJ
via IFTTT
Collect additional information on vote from anonymous users
from Google Alert - anonymous http://ift.tt/1ZWZPU4
via IFTTT
Anonymous: The Canaries
from Google Alert - anonymous http://ift.tt/1UJGkuB
via IFTTT
OpenCV panorama stitching
In today’s blog post, I’ll demonstrate how to perform image stitching and panorama construction using Python and OpenCV. Given two images, we’ll “stitch” them together to create a simple panorama, as seen in the example above.
To construct our image panorama, we’ll utilize computer vision and image processing techniques such as: keypoint detection and local invariant descriptors; keypoint matching; RANSAC; and perspective warping.
Since there are major differences in how OpenCV 2.4.X and OpenCV 3.X handle keypoint detection and local invariant descriptors (such as SIFT and SURF), I’ve taken special care to provide code that is compatible with both versions (provided that you compiled OpenCV 3 with
opencv_contribsupport, of course).
In future blog posts we’ll extend our panorama stitching code to work with multiple images rather than just two.
Read on to find out how panorama stitching with OpenCV is done.
Looking for the source code to this post?
Jump right to the downloads section.
OpenCV panorama stitching
Our panorama stitching algorithm consists of four steps:
- Step #1: Detect keypoints (DoG, Harris, etc.) and extract local invariant descriptors (SIFT, SURF, etc.) from the two input images.
- Step #2: Match the descriptors between the two images.
- Step #3: Use the RANSAC algorithm to estimate a homography matrix using our matched feature vectors.
- Step #4: Apply a warping transformation using the homography matrix obtained from Step #3.
We’ll encapsulate all four of these steps inside
panorama.py, where we’ll define a
Stitcherclass used to construct our panoramas.
The
Stitcherclass will rely on the imutils Python package, so if you don’t already have it installed on your system, you’ll want to go ahead and do that now:
$ pip install imutils
Let’s go ahead and get started by reviewing
panorama.py:
# import the necessary packages import numpy as np import imutils import cv2 class Stitcher: def __init__(self): # determine if we are using OpenCV v3.X self.isv3 = imutils.is_cv3()
We start off on Lines 2-4 by importing our necessary packages. We’ll be using NumPy for matrix/array operations,
imutilsfor a set of OpenCV convenience methods, and finally
cv2for our OpenCV bindings.
From there, we define the
Stitcherclass on Line 6. The constructor to
Stitchersimply checks which version of OpenCV we are using by making a call to the
is_cv3method. Since there are major differences in how OpenCV 2.4 and OpenCV 3 handle keypoint detection and local invariant descriptors, it’s important that we determine the version of OpenCV that we are using.
Next up, let’s start working on the
stitchmethod:
# import the necessary packages import numpy as np import imutils import cv2 class Stitcher: def __init__(self): # determine if we are using OpenCV v3.X self.isv3 = imutils.is_cv3() def stitch(self, images, ratio=0.75, reprojThresh=4.0, showMatches=False): # unpack the images, then detect keypoints and extract # local invariant descriptors from them (imageB, imageA) = images (kpsA, featuresA) = self.detectAndDescribe(imageA) (kpsB, featuresB) = self.detectAndDescribe(imageB) # match features between the two images M = self.matchKeypoints(kpsA, kpsB, featuresA, featuresB, ratio, reprojThresh) # if the match is None, then there aren't enough matched # keypoints to create a panorama if M is None: return None
The
stitchmethod requires only a single parameter,
images, which is the list of (two) images that we are going to stitch together to form the panorama.
We can also optionally supply
ratio, used for David Lowe’s ratio test when matching features (more on this ratio test later in the tutorial),
reprojThreshwhich is the maximum pixel “wiggle room” allowed by the RANSAC algorithm, and finally
showMatches, a boolean used to indicate if the keypoint matches should be visualized or not.
Line 15 unpacks the
imageslist (which again, we presume to contain only two images). The ordering to the
imageslist is important: we expect images to be supplied in left-to-right order. If images are not supplied in this order, then our code will still run — but our output panorama will only contain one image, not both.
Once we have unpacked the
imageslist, we make a call to the
detectAndDescribemethod on Lines 16 and 17. This method simply detects keypoints and extracts local invariant descriptors (i.e., SIFT) from the two images.
Given the keypoints and features, we use
matchKeypoints(Lines 20 and 21) to match the features in the two images. We’ll define this method later in the lesson.
If the returned matches
Mare
None, then not enough keypoints were matched to create a panorama, so we simply return to the calling function (Lines 25 and 26).
Otherwise, we are now ready to apply the perspective transform:
# import the necessary packages import numpy as np import imutils import cv2 class Stitcher: def __init__(self): # determine if we are using OpenCV v3.X self.isv3 = imutils.is_cv3() def stitch(self, images, ratio=0.75, reprojThresh=4.0, showMatches=False): # unpack the images, then detect keypoints and extract # local invariant descriptors from them (imageB, imageA) = images (kpsA, featuresA) = self.detectAndDescribe(imageA) (kpsB, featuresB) = self.detectAndDescribe(imageB) # match features between the two images M = self.matchKeypoints(kpsA, kpsB, featuresA, featuresB, ratio, reprojThresh) # if the match is None, then there aren't enough matched # keypoints to create a panorama if M is None: return None # otherwise, apply a perspective warp to stitch the images # together (matches, H, status) = M result = cv2.warpPerspective(imageA, H, (imageA.shape[1] + imageB.shape[1], imageA.shape[0])) result[0:imageB.shape[0], 0:imageB.shape[1]] = imageB # check to see if the keypoint matches should be visualized if showMatches: vis = self.drawMatches(imageA, imageB, kpsA, kpsB, matches, status) # return a tuple of the stitched image and the # visualization return (result, vis) # return the stitched image return result
Provided that
Mis not
None, we unpack the tuple on Line 30, giving us a list of keypoint
matches, the homography matrix
Hderived from the RANSAC algorithm, and finally
status, a list of indexes to indicate which keypoints in
matcheswere successfully spatially verified using RANSAC.
Given our homography matrix
H, we are now ready to stitch the two images together. First, we make a call to
cv2.warpPerspectivewhich requires three arguments: the image we want to warp (in this case, the right image), the 3 x 3 transformation matrix (
H), and finally the shape out of the output image. We derive the shape out of the output image by taking the sum of the widths of both images and then using the height of the second image.
Line 30 makes a check to see if we should visualize the keypoint matches, and if so, we make a call to
drawMatchesand return a tuple of both the panorama and visualization to the calling method (Lines 37-42).
Otherwise, we simply returned the stitched image (Line 45).
Now that the
stitchmethod has been defined, let’s look into some of the helper methods that it calls. We’ll start with
detectAndDescribe:
# import the necessary packages import numpy as np import imutils import cv2 class Stitcher: def __init__(self): # determine if we are using OpenCV v3.X self.isv3 = imutils.is_cv3() def stitch(self, images, ratio=0.75, reprojThresh=4.0, showMatches=False): # unpack the images, then detect keypoints and extract # local invariant descriptors from them (imageB, imageA) = images (kpsA, featuresA) = self.detectAndDescribe(imageA) (kpsB, featuresB) = self.detectAndDescribe(imageB) # match features between the two images M = self.matchKeypoints(kpsA, kpsB, featuresA, featuresB, ratio, reprojThresh) # if the match is None, then there aren't enough matched # keypoints to create a panorama if M is None: return None # otherwise, apply a perspective warp to stitch the images # together (matches, H, status) = M result = cv2.warpPerspective(imageA, H, (imageA.shape[1] + imageB.shape[1], imageA.shape[0])) result[0:imageB.shape[0], 0:imageB.shape[1]] = imageB # check to see if the keypoint matches should be visualized if showMatches: vis = self.drawMatches(imageA, imageB, kpsA, kpsB, matches, status) # return a tuple of the stitched image and the # visualization return (result, vis) # return the stitched image return result def detectAndDescribe(self, image): # convert the image to grayscale gray = cv2.cvtColor(image, cv2.COLOR_BGR2GRAY) # check to see if we are using OpenCV 3.X if self.isv3: # detect and extract features from the image descriptor = cv2.xfeatures2d.SIFT_create() (kps, features) = descriptor.detectAndCompute(image, None) # otherwise, we are using OpenCV 2.4.X else: # detect keypoints in the image detector = cv2.FeatureDetector_create("SIFT") kps = detector.detect(gray) # extract features from the image extractor = cv2.DescriptorExtractor_create("SIFT") (kps, features) = extractor.compute(gray, kps) # convert the keypoints from KeyPoint objects to NumPy # arrays kps = np.float32([kp.pt for kp in kps]) # return a tuple of keypoints and features return (kps, features)
As the name suggests, the
detectAndDescribemethod accepts an image, then detects keypoints and extracts local invariant descriptors. In our implementation we use the Difference of Gaussian (DoG) keypoint detector and the SIFT feature extractor.
On Line 52 we check to see if we are using OpenCV 3.X. If we are, then we use the
cv2.xfeatures2d.SIFT_createfunction to instantiate both our DoG keypoint detector and SIFT feature extractor. A call to
detectAndComputehandles extracting the keypoints and features (Lines 54 and 55).
It’s important to note that you must have compiled OpenCV 3.X with opencv_contrib support enabled. If you did not, you’ll get an error such as
AttributeError: 'module' object has no attribute 'xfeatures2d'. If that’s the case, head over to my OpenCV 3 tutorials page where I detail how to install OpenCV 3 with
opencv_contribsupport enabled for a variety of operating systems and Python versions.
Lines 58-65 handle if we are using OpenCV 2.4. The
cv2.FeatureDetector_createfunction instantiates our keypoint detector (DoG). A call to
detectreturns our set of keypoints.
From there, we need to initialize
cv2.DescriptorExtractor_createusing the
SIFTkeyword to setup our SIFT feature
extractor. Calling the
computemethod of the
extractorreturns a set of feature vectors which quantify the region surrounding each of the detected keypoints in the image.
Finally, our keypoints are converted from
KeyPointobjects to a NumPy array (Line 69) and returned to the calling method (Line 72).
Next up, let’s look at the
matchKeypointsmethod:
# import the necessary packages import numpy as np import imutils import cv2 class Stitcher: def __init__(self): # determine if we are using OpenCV v3.X self.isv3 = imutils.is_cv3() def stitch(self, images, ratio=0.75, reprojThresh=4.0, showMatches=False): # unpack the images, then detect keypoints and extract # local invariant descriptors from them (imageB, imageA) = images (kpsA, featuresA) = self.detectAndDescribe(imageA) (kpsB, featuresB) = self.detectAndDescribe(imageB) # match features between the two images M = self.matchKeypoints(kpsA, kpsB, featuresA, featuresB, ratio, reprojThresh) # if the match is None, then there aren't enough matched # keypoints to create a panorama if M is None: return None # otherwise, apply a perspective warp to stitch the images # together (matches, H, status) = M result = cv2.warpPerspective(imageA, H, (imageA.shape[1] + imageB.shape[1], imageA.shape[0])) result[0:imageB.shape[0], 0:imageB.shape[1]] = imageB # check to see if the keypoint matches should be visualized if showMatches: vis = self.drawMatches(imageA, imageB, kpsA, kpsB, matches, status) # return a tuple of the stitched image and the # visualization return (result, vis) # return the stitched image return result def detectAndDescribe(self, image): # convert the image to grayscale gray = cv2.cvtColor(image, cv2.COLOR_BGR2GRAY) # check to see if we are using OpenCV 3.X if self.isv3: # detect and extract features from the image descriptor = cv2.xfeatures2d.SIFT_create() (kps, features) = descriptor.detectAndCompute(image, None) # otherwise, we are using OpenCV 2.4.X else: # detect keypoints in the image detector = cv2.FeatureDetector_create("SIFT") kps = detector.detect(gray) # extract features from the image extractor = cv2.DescriptorExtractor_create("SIFT") (kps, features) = extractor.compute(gray, kps) # convert the keypoints from KeyPoint objects to NumPy # arrays kps = np.float32([kp.pt for kp in kps]) # return a tuple of keypoints and features return (kps, features) def matchKeypoints(self, kpsA, kpsB, featuresA, featuresB, ratio, reprojThresh): # compute the raw matches and initialize the list of actual # matches matcher = cv2.DescriptorMatcher_create("BruteForce") rawMatches = matcher.knnMatch(featuresA, featuresB, 2) matches = [] # loop over the raw matches for m in rawMatches: # ensure the distance is within a certain ratio of each # other (i.e. Lowe's ratio test) if len(m) == 2 and m[0].distance < m[1].distance * ratio: matches.append((m[0].trainIdx, m[0].queryIdx))
The
matchKeypointsfunction requires four arguments: the keypoints and feature vectors associated with the first image, followed by the keypoints and feature vectors associated with the second image. David Lowe’s
ratiotest variable and RANSAC re-projection threshold are also be supplied.
Matching features together is actually a fairly straightforward process. We simply loop over the descriptors from both images, compute the distances, and find the smallest distance for each pair of descriptors. Since this is a very common practice in computer vision, OpenCV has a built-in function called
cv2.DescriptorMatcher_createthat constructs the feature matcher for us. The
BruteForcevalue indicates that we are going to exhaustively compute the Euclidean distance between all feature vectors from both images and find the pairs of descriptors that have the smallest distance.
A call to
knnMatchon Line 79 performs k-NN matching between the two feature vector sets using k=2 (indicating the top two matches for each feature vector are returned).
The reason we want the top two matches rather than just the top one match is because we need to apply David Lowe’s ratio test for false-positive match pruning.
Again, Line 79 computes the
rawMatchesfor each pair of descriptors — but there is a chance that some of these pairs are false positives, meaning that the image patches are not actually true matches. In an attempt to prune these false-positive matches, we can loop over each of the
rawMatchesindividually (Line 83) and apply Lowe’s ratio test, which is used to determine high-quality feature matches. Typical values for Lowe’s ratio are normally in the range [0.7, 0.8].
Once we have obtained the
matchesusing Lowe’s ratio test, we can compute the homography between the two sets of keypoints:
# import the necessary packages import numpy as np import imutils import cv2 class Stitcher: def __init__(self): # determine if we are using OpenCV v3.X self.isv3 = imutils.is_cv3() def stitch(self, images, ratio=0.75, reprojThresh=4.0, showMatches=False): # unpack the images, then detect keypoints and extract # local invariant descriptors from them (imageB, imageA) = images (kpsA, featuresA) = self.detectAndDescribe(imageA) (kpsB, featuresB) = self.detectAndDescribe(imageB) # match features between the two images M = self.matchKeypoints(kpsA, kpsB, featuresA, featuresB, ratio, reprojThresh) # if the match is None, then there aren't enough matched # keypoints to create a panorama if M is None: return None # otherwise, apply a perspective warp to stitch the images # together (matches, H, status) = M result = cv2.warpPerspective(imageA, H, (imageA.shape[1] + imageB.shape[1], imageA.shape[0])) result[0:imageB.shape[0], 0:imageB.shape[1]] = imageB # check to see if the keypoint matches should be visualized if showMatches: vis = self.drawMatches(imageA, imageB, kpsA, kpsB, matches, status) # return a tuple of the stitched image and the # visualization return (result, vis) # return the stitched image return result def detectAndDescribe(self, image): # convert the image to grayscale gray = cv2.cvtColor(image, cv2.COLOR_BGR2GRAY) # check to see if we are using OpenCV 3.X if self.isv3: # detect and extract features from the image descriptor = cv2.xfeatures2d.SIFT_create() (kps, features) = descriptor.detectAndCompute(image, None) # otherwise, we are using OpenCV 2.4.X else: # detect keypoints in the image detector = cv2.FeatureDetector_create("SIFT") kps = detector.detect(gray) # extract features from the image extractor = cv2.DescriptorExtractor_create("SIFT") (kps, features) = extractor.compute(gray, kps) # convert the keypoints from KeyPoint objects to NumPy # arrays kps = np.float32([kp.pt for kp in kps]) # return a tuple of keypoints and features return (kps, features) def matchKeypoints(self, kpsA, kpsB, featuresA, featuresB, ratio, reprojThresh): # compute the raw matches and initialize the list of actual # matches matcher = cv2.DescriptorMatcher_create("BruteForce") rawMatches = matcher.knnMatch(featuresA, featuresB, 2) matches = [] # loop over the raw matches for m in rawMatches: # ensure the distance is within a certain ratio of each # other (i.e. Lowe's ratio test) if len(m) == 2 and m[0].distance < m[1].distance * ratio: matches.append((m[0].trainIdx, m[0].queryIdx)) # computing a homography requires at least 4 matches if len(matches) > 4: # construct the two sets of points ptsA = np.float32([kpsA[i] for (_, i) in matches]) ptsB = np.float32([kpsB[i] for (i, _) in matches]) # compute the homography between the two sets of points (H, status) = cv2.findHomography(ptsA, ptsB, cv2.RANSAC, reprojThresh) # return the matches along with the homograpy matrix # and status of each matched point return (matches, H, status) # otherwise, no homograpy could be computed return None
Computing a homography between two sets of points requires at a bare minimum an initial set of four matches. For a more reliable homography estimation, we should have substantially more than just four matched points.
Finally, the last method in our
Stitchermethod,
drawMatchesis used to visualize keypoint correspondences between two images:
# import the necessary packages import numpy as np import imutils import cv2 class Stitcher: def __init__(self): # determine if we are using OpenCV v3.X self.isv3 = imutils.is_cv3() def stitch(self, images, ratio=0.75, reprojThresh=4.0, showMatches=False): # unpack the images, then detect keypoints and extract # local invariant descriptors from them (imageB, imageA) = images (kpsA, featuresA) = self.detectAndDescribe(imageA) (kpsB, featuresB) = self.detectAndDescribe(imageB) # match features between the two images M = self.matchKeypoints(kpsA, kpsB, featuresA, featuresB, ratio, reprojThresh) # if the match is None, then there aren't enough matched # keypoints to create a panorama if M is None: return None # otherwise, apply a perspective warp to stitch the images # together (matches, H, status) = M result = cv2.warpPerspective(imageA, H, (imageA.shape[1] + imageB.shape[1], imageA.shape[0])) result[0:imageB.shape[0], 0:imageB.shape[1]] = imageB # check to see if the keypoint matches should be visualized if showMatches: vis = self.drawMatches(imageA, imageB, kpsA, kpsB, matches, status) # return a tuple of the stitched image and the # visualization return (result, vis) # return the stitched image return result def detectAndDescribe(self, image): # convert the image to grayscale gray = cv2.cvtColor(image, cv2.COLOR_BGR2GRAY) # check to see if we are using OpenCV 3.X if self.isv3: # detect and extract features from the image descriptor = cv2.xfeatures2d.SIFT_create() (kps, features) = descriptor.detectAndCompute(image, None) # otherwise, we are using OpenCV 2.4.X else: # detect keypoints in the image detector = cv2.FeatureDetector_create("SIFT") kps = detector.detect(gray) # extract features from the image extractor = cv2.DescriptorExtractor_create("SIFT") (kps, features) = extractor.compute(gray, kps) # convert the keypoints from KeyPoint objects to NumPy # arrays kps = np.float32([kp.pt for kp in kps]) # return a tuple of keypoints and features return (kps, features) def matchKeypoints(self, kpsA, kpsB, featuresA, featuresB, ratio, reprojThresh): # compute the raw matches and initialize the list of actual # matches matcher = cv2.DescriptorMatcher_create("BruteForce") rawMatches = matcher.knnMatch(featuresA, featuresB, 2) matches = [] # loop over the raw matches for m in rawMatches: # ensure the distance is within a certain ratio of each # other (i.e. Lowe's ratio test) if len(m) == 2 and m[0].distance < m[1].distance * ratio: matches.append((m[0].trainIdx, m[0].queryIdx)) # computing a homography requires at least 4 matches if len(matches) > 4: # construct the two sets of points ptsA = np.float32([kpsA[i] for (_, i) in matches]) ptsB = np.float32([kpsB[i] for (i, _) in matches]) # compute the homography between the two sets of points (H, status) = cv2.findHomography(ptsA, ptsB, cv2.RANSAC, reprojThresh) # return the matches along with the homograpy matrix # and status of each matched point return (matches, H, status) # otherwise, no homograpy could be computed return None def drawMatches(self, imageA, imageB, kpsA, kpsB, matches, status): # initialize the output visualization image (hA, wA) = imageA.shape[:2] (hB, wB) = imageB.shape[:2] vis = np.zeros((max(hA, hB), wA + wB, 3), dtype="uint8") vis[0:hA, 0:wA] = imageA vis[0:hB, wA:] = imageB # loop over the matches for ((trainIdx, queryIdx), s) in zip(matches, status): # only process the match if the keypoint was successfully # matched if s == 1: # draw the match ptA = (int(kpsA[queryIdx][0]), int(kpsA[queryIdx][1])) ptB = (int(kpsB[trainIdx][0]) + wA, int(kpsB[trainIdx][1])) cv2.line(vis, ptA, ptB, (0, 255, 0), 1) # return the visualization return vis
This method requires that we pass in the two original images, the set of keypoints associated with each image, the initial matches after applying Lowe’s ratio test, and finally the
statuslist provided by the homography calculation. Using these variables, we can visualize the “inlier” keypoints by drawing a straight line from keypoint N in the first image to keypoint M in the second image.
Now that we have our
Stitcherclass defined, let’s move on to creating the
stitch.pydriver script:
# import the necessary packages from pyimagesearch.panorama import Stitcher import argparse import imutils import cv2 # construct the argument parse and parse the arguments ap = argparse.ArgumentParser() ap.add_argument("-f", "--first", required=True, help="path to the first image") ap.add_argument("-s", "--second", required=True, help="path to the second image") args = vars(ap.parse_args())
We start off by importing our required packages on Lines 2-5. Notice how we’ve placed the
panorama.pyand
Stitcherclass into the
pyimagesearchmodule just to keep our code tidy.
Note: If you are following along with this post and having trouble organizing your code, please be sure to download the source code using the form at the bottom of this post. The .zip of the code download will run out of the box without any errors.
From there, Lines 8-14 parse our command line arguments:
--first, which is the path to the first image in our panorama (the left-most image), and
--second, the path to the second image in the panorama (the right-most image).
Remember, these image paths need to be suppled in left-to-right order!
The rest of the
stitch.pydriver script simply handles loading our images, resizing them (so they can fit on our screen), and constructing our panorama:
# import the necessary packages from pyimagesearch.panorama import Stitcher import argparse import imutils import cv2 # construct the argument parse and parse the arguments ap = argparse.ArgumentParser() ap.add_argument("-f", "--first", required=True, help="path to the first image") ap.add_argument("-s", "--second", required=True, help="path to the second image") args = vars(ap.parse_args()) # load the two images and resize them to have a width of 400 pixels # (for faster processing) imageA = cv2.imread(args["first"]) imageB = cv2.imread(args["second"]) imageA = imutils.resize(imageA, width=400) imageB = imutils.resize(imageB, width=400) # stitch the images together to create a panorama stitcher = Stitcher() (result, vis) = stitcher.stitch([imageA, imageB], showMatches=True) # show the images cv2.imshow("Image A", imageA) cv2.imshow("Image B", imageB) cv2.imshow("Keypoint Matches", vis) cv2.imshow("Result", result) cv2.waitKey(0)
Once our images are loaded and resized, we initialize our
Stitcherclass on Line 23. We then call the
stitchmethod, passing in our two images (again, in left-to-right order) and indicate that we would like to visualize the keypoint matches between the two images.
Finally, Lines 27-31 display our output images to our screen.
Panorama stitching results
In mid-2014 I took a trip out to Arizona and Utah to enjoy the national parks. Along the way I stopped at many locations, including Bryce Canyon, Grand Canyon, and Sedona. Given that these areas contain beautiful scenic views, I naturally took a bunch of photos — some of which are perfect for constructing panoramas. I’ve included a sample of these images in today’s blog to demonstrate panorama stitching.
All that said, let’s give our OpenCV panorama stitcher a try. Open up a terminal and issue the following command:
$ python stitch.py --first images/bryce_left_01.png \ --second images/bryce_right_01.png
Figure 1: (Top) The two input images from Bryce canyon (in left-to-right order). (Bottom) The matched keypoint correspondences between the two images.
At the top of this figure, we can see two input images (resized to fit on my screen, the raw .jpg files are a much higher resolution). And on the bottom, we can see the matched keypoints between the two images.
Using these matched keypoints, we can apply a perspective transform and obtain the final panorama:
As we can see, the two images have been successfully stitched together!
Note: On many of these example images, you’ll often see a visible “seam” running through the center of the stitched images. This is because I shot many of photos using either my iPhone or a digital camera with autofocus turned on, thus the focus is slightly different between each shot. Image stitching and panorama construction work best when you use the same focus for every photo. I never intended to use these vacation photos for image stitching, otherwise I would have taken care to adjust the camera sensors. In either case, just keep in mind the seam is due to varying sensor properties at the time I took the photo and was not intentional.
Let’s give another set of images a try:
$ python stitch.py --first images/bryce_left_02.png \ --second images/bryce_right_02.png
Again, our
Stitcherclass was able to construct a panorama from the two input images.
Now, let’s move on to the Grand Canyon:
$ python stitch.py --first images/grand_canyon_left_01.png \ --second images/grand_canyon_right_01.png
In the above input images we can see heavy overlap between the two input images. The main addition to the panorama is towards the right side of the stitched images where we can see more of the “ledge” is added to the output.
Here’s another example from the Grand Canyon:
$ python stitch.py --first images/grand_canyon_left_02.png \ --second images/grand_canyon_right_02.png
From this example, we can see that more of the huge expanse of the Grand Canyon has been added to the panorama.
Finally, let’s wrap up this blog post with an example image stitching from Sedona, AZ:
$ python stitch.py --first images/sedona_left_01.png \ --second images/sedona_right_01.png
Personally, I find the red rock country of Sedona to be one of the most beautiful areas I’ve ever visited. If you ever have a chance, definitely stop by — you won’t be disappointed.
So there you have it, image stitching and panorama construction using Python and OpenCV!
Summary
In this blog post we learned how to perform image stitching and panorama construction using OpenCV. Source code was provided for image stitching for both OpenCV 2.4 and OpenCV 3.
Our image stitching algorithm requires four steps: (1) detecting keypoints and extracting local invariant descriptors; (2) matching descriptors between images; (3) applying RANSAC to estimate the homography matrix; and (4) applying a warping transformation using the homography matrix.
While simple, this algorithm works well in practice when constructing panoramas for two images. In a future blog post, we’ll review how to construct panoramas and perform image stitching for more than two images.
Anyway, I hope you enjoyed this post! Be sure to use the form below to download the source code and give it a try.
Downloads:
The post OpenCV panorama stitching appeared first on PyImageSearch.
from PyImageSearch http://ift.tt/1OdNHZB
via IFTTT