Latest YouTube Video
Saturday, May 28, 2016
English
from Google Alert - anonymous http://ift.tt/25q0K27
via IFTTT
Queen B-day gets anonymous 'donation'
from Google Alert - anonymous http://ift.tt/1siTnLy
via IFTTT
I have a new follower on Twitter
Samimi Yazar
Twitter Çılgını | yozgat | istanbul
Following: 19564 - Followers: 112838
May 28, 2016 at 06:12AM via Twitter http://twitter.com/samimiyazariz
The Great Carina Nebula
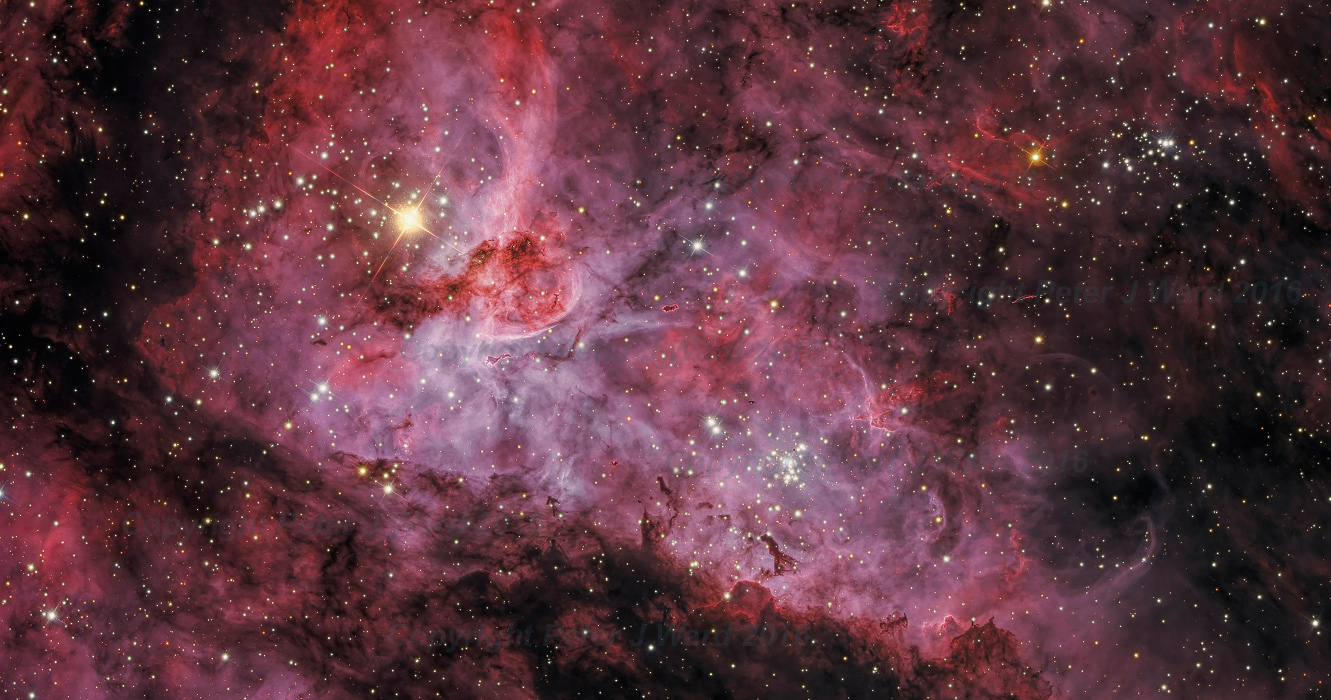
Member Resources
from Google Alert - anonymous http://ift.tt/1qQBFh8
via IFTTT
Friday, May 27, 2016
Unseen Anonymous Photo Sharing
from Google Alert - anonymous http://ift.tt/1P4Nf3N
via IFTTT
An Armenian Wishlist for Anonymous
from Google Alert - anonymous http://ift.tt/1TK6mPD
via IFTTT
Facebook Ads now Tracks you, Even if you don't have an Account
from The Hacker News http://ift.tt/1NSAdpM
via IFTTT
Ravens: Rookie Keenan Reynolds can defer his military service to play in NFL, Sec. of Defense Ashton Carter announces (ESPN)
via IFTTT
How to get Anonymous Ravkav
from Google Alert - anonymous http://ift.tt/1OSd69F
via IFTTT
Google Wins Epic Java Copyright Case Against Oracle
from The Hacker News http://ift.tt/1qP3Hts
via IFTTT
Ravens: DT Timmy Jernigan changes his number to No. 99 to honor Warren Sapp; Hall of Famer tweets \"how do I stop this?\" (ESPN)
via IFTTT
I have a new follower on Twitter
Berat
❤️GÖZTEPE ❤️https://t.co/t15UT6yetL snapchat : beratkamisligol
İZMİR
Following: 2490 - Followers: 16776
May 27, 2016 at 09:51AM via Twitter http://twitter.com/Beraattk
ISS Daily Summary Report – 05/26/16
from ISS On-Orbit Status Report http://ift.tt/1Rwm0th
via IFTTT
Anonymous US Collector Buys 'Holy Grail of Publishing'
from Google Alert - anonymous http://ift.tt/1P2EJlU
via IFTTT
Using an anonymous method to assign a delegate to an action (task 2 of 3).
from Google Alert - anonymous http://ift.tt/25lICq1
via IFTTT
Adult-Themed Candy with Anonymous Shipping (1, 5, or 10 Bags)
from Google Alert - anonymous http://ift.tt/27UQaT1
via IFTTT
I have a new follower on Twitter
Ahmet Sevim
Avcılar | Beylikdüzü
İstanbul
https://t.co/ReZUtVqraY
Following: 22830 - Followers: 1766370
May 27, 2016 at 01:21AM via Twitter http://twitter.com/AhmetSevimm
IC 5067 in the Pelican Nebula
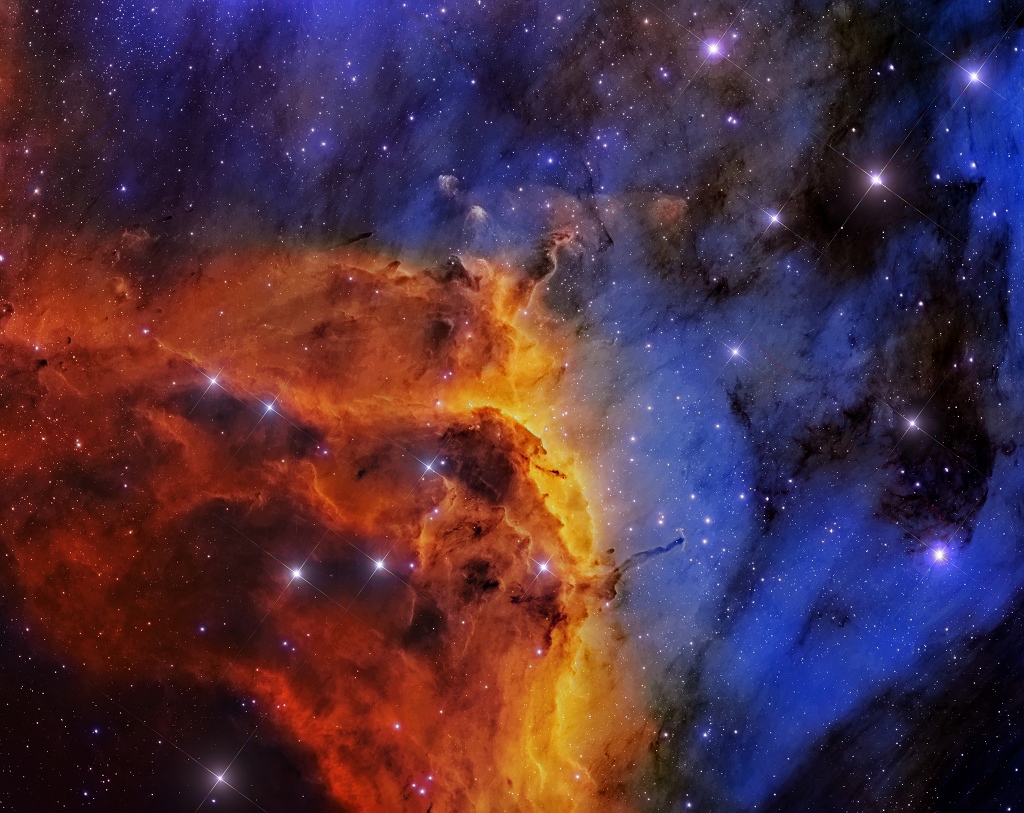
Thursday, May 26, 2016
Philippines Bank hit by SWIFT Hacking Group linked to North Korea
from The Hacker News http://ift.tt/24aw4j0
via IFTTT
A PAC RL Algorithm for Episodic POMDPs. (arXiv:1605.08062v1 [cs.LG])
Many interesting real world domains involve reinforcement learning (RL) in partially observable environments. Efficient learning in such domains is important, but existing sample complexity bounds for partially observable RL are at least exponential in the episode length. We give, to our knowledge, the first partially observable RL algorithm with a polynomial bound on the number of episodes on which the algorithm may not achieve near-optimal performance. Our algorithm is suitable for an important class of episodic POMDPs. Our approach builds on recent advances in method of moments for latent variable model estimation.
from cs.AI updates on arXiv.org http://ift.tt/1Rv5WIo
via IFTTT
Deep Predictive Coding Networks for Video Prediction and Unsupervised Learning. (arXiv:1605.08104v1 [cs.LG])
While great strides have been made in using deep learning algorithms to solve supervised learning tasks, the problem of unsupervised learning - leveraging unlabeled examples to learn about the structure of a domain - remains a difficult unsolved challenge. Here, we explore prediction of future frames in a video sequence as an unsupervised learning rule for learning about the structure of the visual world. We describe a predictive neural network ("PredNet") architecture that is inspired by the concept of "predictive coding" from the neuroscience literature. These networks learn to predict future frames in a video sequence, with each layer in the network making local predictions and only forwarding deviations from those predictions to subsequent network layers. We show that these networks are able to robustly learn to predict the movement of synthetic (rendered) objects, and that in doing so, the networks learn internal representations that are useful for decoding latent object parameters (e.g. pose) that support object recognition with fewer training views. We also show that these networks can scale to complex natural image streams (car-mounted camera videos), capturing key aspects of both egocentric movement and the movement of objects in the visual scene, and generalizing across video datasets. These results suggest that prediction represents a powerful framework for unsupervised learning, allowing for implicit learning of object and scene structure.
from cs.AI updates on arXiv.org http://ift.tt/1sdSBj0
via IFTTT
Cognitive Dynamic Systems: A Technical Review of Cognitive Radar. (arXiv:1605.08150v1 [cs.AI])
We start with the history of cognitive radar, where origins of the PAC, Fuster research on cognition and principals of cognition are provided. Fuster describes five cognitive functions: perception, memory, attention, language, and intelligence. We describe the Perception-Action Cyclec as it applies to cognitive radar, and then discuss long-term memory, memory storage, memory retrieval and working memory. A comparison between memory in human cognition and cognitive radar is given as well. Attention is another function described by Fuster, and we have given the comparison of attention in human cognition and cognitive radar. We talk about the four functional blocks from the PAC: Bayesian filter, feedback information, dynamic programming and state-space model for the radar environment. Then, to show that the PAC improves the tracking accuracy of Cognitive Radar over Traditional Active Radar, we have provided simulation results. In the simulation, three nonlinear filters: Cubature Kalman Filter, Unscented Kalman Filter and Extended Kalman Filter are compared. Based on the results, radars implemented with CKF perform better than the radars implemented with UKF or radars implemented with EKF. Further, radar with EKF has the worst accuracy and has the biggest computation load because of derivation and evaluation of Jacobian matrices. We suggest using the concept of risk management to better control parameters and improve performance in cognitive radar. We believe, spectrum sensing can be seen as a potential interest to be used in cognitive radar and we propose a new approach Probabilistic ICA which will presumably reduce noise based on estimation error in cognitive radar. Parallel computing is a concept based on divide and conquers mechanism, and we suggest using the parallel computing approach in cognitive radar by doing complicated calculations or tasks to reduce processing time.
from cs.AI updates on arXiv.org http://ift.tt/1Z4wWnU
via IFTTT
The Symbolic Interior Point Method. (arXiv:1605.08187v1 [cs.AI])
A recent trend in probabilistic inference emphasizes the codification of models in a formal syntax, with suitable high-level features such as individuals, relations, and connectives, enabling descriptive clarity, succinctness and circumventing the need for the modeler to engineer a custom solver. Unfortunately, bringing these linguistic and pragmatic benefits to numerical optimization has proven surprisingly challenging. In this paper, we turn to these challenges: we introduce a rich modeling language, for which an interior-point method computes approximate solutions in a generic way. While logical features easily complicates the underlying model, often yielding intricate dependencies, we exploit and cache local structure using algebraic decision diagrams (ADDs). Indeed, standard matrix-vector algebra is efficiently realizable in ADDs, but we argue and show that well-known optimization methods are not ideal for ADDs. Our engine, therefore, invokes a sophisticated matrix-free approach. We demonstrate the flexibility of the resulting symbolic-numeric optimizer on decision making and compressed sensing tasks with millions of non-zero entries.
from cs.AI updates on arXiv.org http://ift.tt/1sdSN1F
via IFTTT
Probabilistic Inference Modulo Theories. (arXiv:1605.08367v1 [cs.AI])
We present SGDPLL(T), an algorithm that solves (among many other problems) probabilistic inference modulo theories, that is, inference problems over probabilistic models defined via a logic theory provided as a parameter (currently, propositional, equalities on discrete sorts, and inequalities, more specifically difference arithmetic, on bounded integers).
While many solutions to probabilistic inference over logic representations have been proposed,
SGDPLL(T) is simultaneously (1) lifted, (2) exact and (3) modulo theories, that is, parameterized by a background logic theory.
This offers a foundation for extending it to rich logic languages such as data structures and relational data.
By lifted, we mean algorithms with constant complexity in the domain size (the number of values that variables can take). We also detail a solver for summations with difference arithmetic and show experimental results from a scenario in which SGDPLL(T) is much faster than a state-of-the-art probabilistic solver.
from cs.AI updates on arXiv.org http://ift.tt/1Z4wKFp
via IFTTT
Kronecker Determinantal Point Processes. (arXiv:1605.08374v1 [cs.LG])
Determinantal Point Processes (DPPs) are probabilistic models over all subsets a ground set of $N$ items. They have recently gained prominence in several applications that rely on "diverse" subsets. However, their applicability to large problems is still limited due to the $\mathcal O(N^3)$ complexity of core tasks such as sampling and learning. We enable efficient sampling and learning for DPPs by introducing KronDPP, a DPP model whose kernel matrix decomposes as a tensor product of multiple smaller kernel matrices. This decomposition immediately enables fast exact sampling. But contrary to what one may expect, leveraging the Kronecker product structure for speeding up DPP learning turns out to be more difficult. We overcome this challenge, and derive batch and stochastic optimization algorithms for efficiently learning the parameters of a KronDPP.
from cs.AI updates on arXiv.org http://ift.tt/1Rv6n5t
via IFTTT
Estimation of Passenger Route Choice Pattern Using Smart Card Data for Complex Metro Systems. (arXiv:1605.08390v1 [cs.AI])
Nowadays, metro systems play an important role in meeting the urban transportation demand in large cities. The understanding of passenger route choice is critical for public transit management. The wide deployment of Automated Fare Collection(AFC) systems opens up a new opportunity. However, only each trip's tap-in and tap-out timestamp and stations can be directly obtained from AFC system records; the train and route chosen by a passenger are unknown, which are necessary to solve our problem. While existing methods work well in some specific situations, they don't work for complicated situations. In this paper, we propose a solution that needs no additional equipment or human involvement than the AFC systems. We develop a probabilistic model that can estimate from empirical analysis how the passenger flows are dispatched to different routes and trains. We validate our approach using a large scale data set collected from the Shenzhen metro system. The measured results provide us with useful inputs when building the passenger path choice model.
from cs.AI updates on arXiv.org http://ift.tt/1sdSBzr
via IFTTT
CITlab ARGUS for historical handwritten documents. (arXiv:1605.08412v1 [cs.CV])
We describe CITlab's recognition system for the HTRtS competition attached to the 13. International Conference on Document Analysis and Recognition, ICDAR 2015. The task comprises the recognition of historical handwritten documents. The core algorithms of our system are based on multi-dimensional recurrent neural networks (MDRNN) and connectionist temporal classification (CTC). The software modules behind that as well as the basic utility technologies are essentially powered by PLANET's ARGUS framework for intelligent text recognition and image processing.
from cs.AI updates on arXiv.org http://ift.tt/1Z4wW7o
via IFTTT
Doubly Robust Off-policy Value Evaluation for Reinforcement Learning. (arXiv:1511.03722v3 [cs.LG] UPDATED)
We study the problem of off-policy value evaluation in reinforcement learning (RL), where one aims to estimate the value of a new policy based on data collected by a different policy. This problem is often a critical step when applying RL in real-world problems. Despite its importance, existing general methods either have uncontrolled bias or suffer high variance. In this work, we extend the doubly robust estimator for bandits to sequential decision-making problems, which gets the best of both worlds: it is guaranteed to be unbiased and can have a much lower variance than the popular importance sampling estimators. We demonstrate the estimator's accuracy in several benchmark problems, and illustrate its use as a subroutine in safe policy improvement. We also provide theoretical results on the hardness of the problem, and show that our estimator can match the lower bound in certain scenarios.
from cs.AI updates on arXiv.org http://ift.tt/1QlaMN5
via IFTTT
Auxiliary Deep Generative Models. (arXiv:1602.05473v2 [stat.ML] UPDATED)
Deep generative models parameterized by neural networks have recently achieved state-of-the-art performance in unsupervised and semi-supervised learning. We extend deep generative models with auxiliary variables which improves the variational approximation. The auxiliary variables leave the generative model unchanged but make the variational distribution more expressive. Inspired by the structure of the auxiliary variable we also propose a model with two stochastic layers and skip connections. Our findings suggest that more expressive and properly specified deep generative models converge faster with better results. We show state-of-the-art performance within semi-supervised learning on MNIST, SVHN and NORB datasets.
from cs.AI updates on arXiv.org http://ift.tt/1Oh5oUN
via IFTTT
Hierarchical Compound Poisson Factorization. (arXiv:1604.03853v2 [cs.LG] UPDATED)
Non-negative matrix factorization models based on a hierarchical Gamma-Poisson structure capture user and item behavior effectively in extremely sparse data sets, making them the ideal choice for collaborative filtering applications. Hierarchical Poisson factorization (HPF) in particular has proved successful for scalable recommendation systems with extreme sparsity. HPF, however, suffers from a tight coupling of sparsity model (absence of a rating) and response model (the value of the rating), which limits the expressiveness of the latter. Here, we introduce hierarchical compound Poisson factorization (HCPF) that has the favorable Gamma-Poisson structure and scalability of HPF to high-dimensional extremely sparse matrices. More importantly, HCPF decouples the sparsity model from the response model, allowing us to choose the most suitable distribution for the response. HCPF can capture binary, non-negative discrete, non-negative continuous, and zero-inflated continuous responses. We compare HCPF with HPF on nine discrete and three continuous data sets and conclude that HCPF captures the relationship between sparsity and response better than HPF.
from cs.AI updates on arXiv.org http://ift.tt/23w57Lt
via IFTTT
Ravens: Ray Rice spoke to Baltimore rookies Thursday; believed to be first time he was at facility since being released (ESPN)
via IFTTT
Blan PD receives anonymous $50K donation
from Google Alert - anonymous http://ift.tt/1WnPBxb
via IFTTT
Anonymous $15k Donation to City of Holland
from Google Alert - anonymous http://ift.tt/1RuPMP1
via IFTTT
NFL: Ravens forfeit 1 week of June OTAs, plus team and John Harbaugh fined for violating offseason rules at rookie camp (ESPN)
via IFTTT
Raspberry Pi 3 to get official Android OS support
from The Hacker News http://ift.tt/1OPHLEy
via IFTTT
I have a new follower on Twitter
erpscan
ERPScan. Detect and Prevent Cyberattacks in SAP and Oracle business-critical ERP systems
Palo Alto, CA
http://t.co/eF2IkBDTom
Following: 1953 - Followers: 1767
May 26, 2016 at 11:25AM via Twitter http://twitter.com/erpscan
Anonymous Tip Leads to Drug Arrest
from Google Alert - anonymous http://ift.tt/25jZxJI
via IFTTT
Re: [FD] Teampass v2.1.26 - Stored Cross Site Scripting Vulnerability
Re: [FD] CVE-2015-3854 Battery permission leakage in Android
Source: Gmail -> IFTTT-> Blogger
[FD] CVE-2015-3854 Battery permission leakage in Android
Source: Gmail -> IFTTT-> Blogger
Check anonymous users only if session has started
from Google Alert - anonymous http://ift.tt/1TGj1mt
via IFTTT
ISS Daily Summary Report – 05/25/16
from ISS On-Orbit Status Report http://ift.tt/20FF9zt
via IFTTT
NGC 5078 and Friends
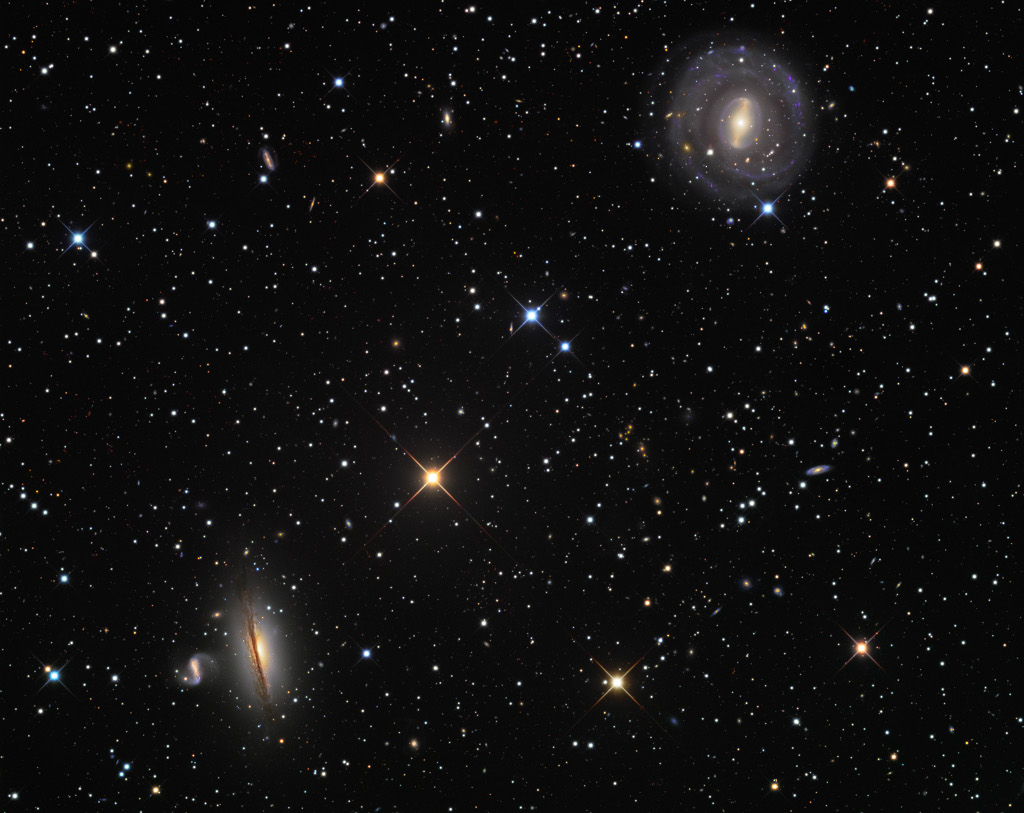
[FD] CVE-2016-4803 dotCMS - Email Header Injection
POST /dotCMS/sendEmail HTTP/1.1 Host: demo2.dotcms.com ... Cookie: _JSESSIONID=998ADA19C99505E75DC6D27A5E84D...; ... Connection: keep-alive Content-Type: application/x-www-form-urlencoded Content-Length: 218 from=myemail&to=youremail&subject=subject%0D%0AX-PoC-of-New-Line%3A+True&returnUrl=%2F1&invalidCaptchaReturnUrl=%2F2&useCaptcha=true&captcha=hwxc5&comments=some+content&send=Send
## Received email source: Message-ID: <1894336506.1449476889789.JavaMail.dotcms@democms1.dotcms.net> From: myemail To: youremail Subject: subject X-PoC-of-New-Line: True MIME-Version: 1.0 Content-Type: multipart/alternative; boundary=
Source: Gmail -> IFTTT-> Blogger
Wednesday, May 25, 2016
I have a new follower on Twitter
Kloete Group, Inc.
Executive Search placing quality Leaders w/ proven 8 step Talent Acquisition process & research helping our clients crush their goals & exceed expectations.
Greater Nashville Area
https://t.co/BDc9gurz0F
Following: 1883 - Followers: 2136
May 25, 2016 at 09:54PM via Twitter http://twitter.com/KloeteGroup
Learning Purposeful Behaviour in the Absence of Rewards. (arXiv:1605.07700v1 [cs.LG])
Artificial intelligence is commonly defined as the ability to achieve goals in the world. In the reinforcement learning framework, goals are encoded as reward functions that guide agent behaviour, and the sum of observed rewards provide a notion of progress. However, some domains have no such reward signal, or have a reward signal so sparse as to appear absent. Without reward feedback, agent behaviour is typically random, often dithering aimlessly and lacking intentionality. In this paper we present an algorithm capable of learning purposeful behaviour in the absence of rewards. The algorithm proceeds by constructing temporally extended actions (options), through the identification of purposes that are "just out of reach" of the agent's current behaviour. These purposes establish intrinsic goals for the agent to learn, ultimately resulting in a suite of behaviours that encourage the agent to visit different parts of the state space. Moreover, the approach is particularly suited for settings where rewards are very sparse, and such behaviours can help in the exploration of the environment until reward is observed.
from cs.AI updates on arXiv.org http://ift.tt/1qKphiH
via IFTTT
Yum-me: Personalized Healthy Meal Recommender System. (arXiv:1605.07722v1 [cs.HC])
Many ubiquitous computing projects have addressed health and wellness behaviors such as healthy eating. Healthy meal recommendations have the potential to help individuals prevent or manage conditions such as diabetes and obesity. However, learning people's food preferences and making healthy recommendations that appeal to their palate is challenging. Existing approaches either only learn high-level preferences or require a prolonged learning period. We propose Yum-me, a personalized healthy meal recommender system designed to meet individuals' health goals, dietary restrictions, and fine-grained food preferences. Marrying ideas from user preference learning and healthy eating promotion, Yum-me enables a simple and accurate food preference profiling procedure via an image-based online learning framework, and projects the learned profile into the domain of healthy food options to find ones that will appeal to the user. We present the design and implementation of Yum-me, and further discuss the most critical component of it: FoodDist, a state-of-the-art food image analysis model. We demonstrate FoodDist's superior performance through careful benchmarking, and discuss its applicability across a wide array of dietary applications. We validate the feasibility and effectiveness of Yum-me through a 60-person user study, in which Yum-me improves the recommendation acceptance rate by 42.63% over the traditional food preference survey.
from cs.AI updates on arXiv.org http://ift.tt/1TFeBMP
via IFTTT
Data Programming: Creating Large Training Sets, Quickly. (arXiv:1605.07723v1 [stat.ML])
Large labeled training sets are the critical building blocks of supervised learning methods and are key enablers of deep learning techniques. For some applications, creating labeled training sets is the most time-consuming and expensive part of applying machine learning. We therefore propose a paradigm for the programmatic creation of training sets called data programming in which users provide a set of labeling functions, which are programs that heuristically label large subsets of data points, albeit noisily. By viewing these labeling functions as implicitly describing a generative model for this noise, we show that we can recover the parameters of this model to "denoise" the training set. Then, we show how to modify a discriminative loss function to make it noise-aware. We demonstrate our method over a range of discriminative models including logistic regression and LSTMs. We establish theoretically that we can recover the parameters of these generative models in a handful of settings. Experimentally, on the 2014 TAC-KBP relation extraction challenge, we show that data programming would have obtained a winning score, and also show that applying data programming to an LSTM model leads to a TAC-KBP score almost 6 F1 points over a supervised LSTM baseline (and into second place in the competition). Additionally, in initial user studies we observed that data programming may be an easier way to create machine learning models for non-experts.
from cs.AI updates on arXiv.org http://ift.tt/1NObZwP
via IFTTT
Small Representations of Big Kidney Exchange Graphs. (arXiv:1605.07728v1 [cs.AI])
Kidney exchanges are organized markets where patients swap willing but incompatible donors. In the last decade, kidney exchanges grew from small and regional to large and national---and soon, international. This growth results in more lives saved, but exacerbates the empirical hardness of the $\mathcal{NP}$-complete problem of optimally matching patients to donors. State-of-the-art matching engines use integer programming techniques to clear fielded kidney exchanges, but these methods must be tailored to specific models and objective functions, and may fail to scale to larger exchanges. In this paper, we observe that if the kidney exchange compatibility graph can be encoded by a constant number of patient and donor attributes, the clearing problem is solvable in polynomial time. We give necessary and sufficient conditions for losslessly shrinking the representation of an arbitrary compatibility graph. Then, using real compatibility graphs from the UNOS nationwide kidney exchange, we show how many attributes are needed to encode real compatibility graphs. The experiments show that, indeed, small numbers of attributes suffice.
from cs.AI updates on arXiv.org http://ift.tt/1WUDacF
via IFTTT
Learning Multiagent Communication with Backpropagation. (arXiv:1605.07736v1 [cs.LG])
Many tasks in AI require the collaboration of multiple agents. Typically, the communication protocol between agents is manually specified and not altered during training. In this paper we explore a simple neural model, called CommNN, that uses continuous communication for fully cooperative tasks. The model consists of multiple agents and the communication between them is learned alongside their policy. We apply this model to a diverse set of tasks, demonstrating the ability of the agents to learn to communicate amongst themselves, yielding improved performance over non-communicative agents and baselines. In some cases, it is possible to interpret the language devised by the agents, revealing simple but effective strategies for solving the task at hand.
from cs.AI updates on arXiv.org http://ift.tt/1NObZx1
via IFTTT
Dimension Projection among Languages based on Pseudo-relevant Documents for Query Translation. (arXiv:1605.07844v1 [cs.IR])
Taking advantage of top-ranked documents in response to a query for improving quality of query translation has been shown to be an effective approach for cross-language information retrieval. In this paper, we propose a new method for query translation based on dimension projection of embedded vectors from the pseudo-relevant documents in the source language to their equivalents in the target language. To this end, first we learn low-dimensional representations of the words in the pseudo-relevant collections separately and then aim at finding a query-dependent transformation matrix between the vectors of translation pairs. At the next step, representation of each query term is projected to the target language and then, after using a softmax function, a query-dependent translation model is built. Finally, the model is used for query translation. Our experiments on four CLEF collections in French, Spanish, German, and Persian demonstrate that the proposed method outperforms all competitive baselines in language modelling, particularly when it is combined with a collection-dependent translation model.
from cs.AI updates on arXiv.org http://ift.tt/1TFe8Kp
via IFTTT
Automatic Extraction of Causal Relations from Natural Language Texts: A Comprehensive Survey. (arXiv:1605.07895v1 [cs.AI])
Automatic extraction of cause-effect relationships from natural language texts is a challenging open problem in Artificial Intelligence. Most of the early attempts at its solution used manually constructed linguistic and syntactic rules on small and domain-specific data sets. However, with the advent of big data, the availability of affordable computing power and the recent popularization of machine learning, the paradigm to tackle this problem has slowly shifted. Machines are now expected to learn generic causal extraction rules from labelled data with minimal supervision, in a domain independent-manner. In this paper, we provide a comprehensive survey of causal relation extraction techniques from both paradigms, and analyse their relative strengths and weaknesses, with recommendations for future work.
from cs.AI updates on arXiv.org http://ift.tt/1WUDpo0
via IFTTT
Automatic Open Knowledge Acquisition via Long Short-Term Memory Networks with Feedback Negative Sampling. (arXiv:1605.07918v1 [cs.CL])
Previous studies in Open Information Extraction (Open IE) are mainly based on extraction patterns. They manually define patterns or automatically learn them from a large corpus. However, these approaches are limited when grasping the context of a sentence, and they fail to capture implicit relations. In this paper, we address this problem with the following methods. First, we exploit long short-term memory (LSTM) networks to extract higher-level features along the shortest dependency paths, connecting headwords of relations and arguments. The path-level features from LSTM networks provide useful clues regarding contextual information and the validity of arguments. Second, we constructed samples to train LSTM networks without the need for manual labeling. In particular, feedback negative sampling picks highly negative samples among non-positive samples through a model trained with positive samples. The experimental results show that our approach produces more precise and abundant extractions than state-of-the-art open IE systems. To the best of our knowledge, this is the first work to apply deep learning to Open IE.
from cs.AI updates on arXiv.org http://ift.tt/1TFeAbJ
via IFTTT
Adaptive Neural Compilation. (arXiv:1605.07969v1 [cs.AI])
This paper proposes an adaptive neural-compilation framework to address the problem of efficient program learning. Traditional code optimisation strategies used in compilers are based on applying pre-specified set of transformations that make the code faster to execute without changing its semantics. In contrast, our work involves adapting programs to make them more efficient while considering correctness only on a target input distribution. Our approach is inspired by the recent works on differentiable representations of programs. We show that it is possible to compile programs written in a low-level language to a differentiable representation. We also show how programs in this representation can be optimised to make them efficient on a target distribution of inputs. Experimental results demonstrate that our approach enables learning specifically-tuned algorithms for given data distributions with a high success rate.
from cs.AI updates on arXiv.org http://ift.tt/1NObR0h
via IFTTT
Compliant Conditions for Polynomial Time Approximation of Operator Counts. (arXiv:1605.07989v1 [cs.AI])
In this brief abstract, we develop a computationally simpler version of the operator count heuristic for a particular class of domains. The contribution of this abstract is thus threefold, we (1) propose an efficient closed form approximation to the operator count heuristic; (2) leverage compressed sensing techniques to obtain an integer approximation in polynomial time; and (3) discuss the relationship of the proposed formulation to existing heuristics and investigate properties of domains where such approaches are useful.
from cs.AI updates on arXiv.org http://ift.tt/1WUDmsm
via IFTTT
Toward a general, scaleable framework for Bayesian teaching with applications to topic models. (arXiv:1605.07999v1 [cs.AI])
Machines, not humans, are the world's dominant knowledge accumulators but humans remain the dominant decision makers. Interpreting and disseminating the knowledge accumulated by machines requires expertise, time, and is prone to failure. The problem of how best to convey accumulated knowledge from computers to humans is a critical bottleneck in the broader application of machine learning. We propose an approach based on human teaching where the problem is formalized as selecting a small subset of the data that will, with high probability, lead the human user to the correct inference. This approach, though successful for modeling human learning in simple laboratory experiments, has failed to achieve broader relevance due to challenges in formulating general and scalable algorithms. We propose general-purpose teaching via pseudo-marginal sampling and demonstrate the algorithm by teaching topic models. Simulation results show our sampling-based approach: effectively approximates the probability where ground-truth is possible via enumeration, results in data that are markedly different from those expected by random sampling, and speeds learning especially for small amounts of data. Application to movie synopsis data illustrates differences between teaching and random sampling for teaching distributions and specific topics, and demonstrates gains in scalability and applicability to real-world problems.
from cs.AI updates on arXiv.org http://ift.tt/1NObK56
via IFTTT
Causal inference for cloud computing. (arXiv:1603.01581v2 [cs.AI] UPDATED)
Cloud computing involves complex technical and economical systems and interactions. This brings about various challenges, two of which are: (1) debugging and control of computing systems, based on heterogeneous data, and (2) prediction of performance and price of "spot" resources, allocated via auctions. In this paper, we first establish two theoretical results on approximate causal inference. We then use the first one, approximate counterfactuals, along with established causal methodology, to outline a general framework to address (1). To address (2), we show how the second one, approximate integration of causal knowledge, can in principle provide a tool for cloud clients to trade off privacy against predictability of cloud costs. We report experiments on simulated and real data.
from cs.AI updates on arXiv.org http://ift.tt/1p0WgPt
via IFTTT
One-Shot Generalization in Deep Generative Models. (arXiv:1603.05106v2 [stat.ML] UPDATED)
Humans have an impressive ability to reason about new concepts and experiences from just a single example. In particular, humans have an ability for one-shot generalization: an ability to encounter a new concept, understand its structure, and then be able to generate compelling alternative variations of the concept. We develop machine learning systems with this important capacity by developing new deep generative models, models that combine the representational power of deep learning with the inferential power of Bayesian reasoning. We develop a class of sequential generative models that are built on the principles of feedback and attention. These two characteristics lead to generative models that are among the state-of-the art in density estimation and image generation. We demonstrate the one-shot generalization ability of our models using three tasks: unconditional sampling, generating new exemplars of a given concept, and generating new exemplars of a family of concepts. In all cases our models are able to generate compelling and diverse samples---having seen new examples just once---providing an important class of general-purpose models for one-shot machine learning.
from cs.AI updates on arXiv.org http://ift.tt/1nPBWiT
via IFTTT
COCO: A Platform for Comparing Continuous Optimizers in a Black-Box Setting. (arXiv:1603.08785v2 [cs.AI] UPDATED)
COCO is a platform for Comparing Continuous Optimizers in a black-box setting. It aims at automatizing the tedious and repetitive task of benchmarking numerical optimization algorithms to the greatest possible extent. We present the rationals behind the development of the platform as a general proposition for a guideline towards better benchmarking. We detail underlying fundamental concepts of COCO such as its definition of a problem, the idea of instances, the relevance of target values, and runtime as central performance measure. Finally, we give a quick overview of the basic code structure and the available test suites.
from cs.AI updates on arXiv.org http://ift.tt/1MzUfo5
via IFTTT
COCO: The Bi-objective Black Box Optimization Benchmarking (bbob-biobj) Test Suite. (arXiv:1604.00359v2 [cs.AI] UPDATED)
The bbob-biobj test suite contains 55 bi-objective functions in continuous domain which are derived from combining functions of the well-known single-objective noiseless bbob test suite. Besides giving the actual function definitions and presenting their (known) properties, this documentation also aims at giving the rationale behind our approach in terms of function groups, instances, and potential objective space normalization.
from cs.AI updates on arXiv.org http://ift.tt/1RHmwWP
via IFTTT
Distributed Clustering of Linear Bandits in Peer to Peer Networks. (arXiv:1604.07706v2 [cs.LG] UPDATED)
We provide two distributed confidence ball algorithms for solving linear bandit problems in peer to peer networks with limited communication capabilities. For the first, we assume that all the peers are solving the same linear bandit problem, and prove that our algorithm achieves the optimal asymptotic regret rate of any centralised algorithm that can instantly communicate information between the peers. For the second, we assume that there are clusters of peers solving the same bandit problem within each cluster, and we prove that our algorithm discovers these clusters, while achieving the optimal asymptotic regret rate within each one. Through experiments on several real-world datasets, we demonstrate the performance of proposed algorithms compared to the state-of-the-art.
from cs.AI updates on arXiv.org http://ift.tt/1WoMi7e
via IFTTT
When Anonymous Grading is on, hide Student Name in Gradebook
from Google Alert - anonymous http://ift.tt/1saMa02
via IFTTT
Anonymous user cart not clearing - Exposing sensitive user info
from Google Alert - anonymous http://ift.tt/1UdZg5N
via IFTTT
Ravens: OL John Urschel tweets that he got straight A's in the four Ph.D. classes he took in his first semester at MIT (ESPN)
via IFTTT
Question about OV Anonymous Chip card - North Holland Province Forum
from Google Alert - anonymous http://ift.tt/1TEm6Um
via IFTTT
Oregon Veteran Surprised by Anonymous Gift
from Google Alert - anonymous http://ift.tt/1OX2ITs
via IFTTT
I have a new follower on Twitter
ComSec
Global Counterespionage Specialists | Cyber TSCM
United States of America
https://t.co/Bf9FOt0RbG
Following: 597 - Followers: 1717
May 25, 2016 at 11:29AM via Twitter http://twitter.com/comsec
Apple hires Encryption Expert to Beef Up Security on its Devices
from The Hacker News http://ift.tt/1Tyjfzc
via IFTTT
ISS Daily Summary Report – 05/24/16
from ISS On-Orbit Status Report http://ift.tt/247pnOF
via IFTTT
Hacker Group Anonymous Targets Governor Rick Scott
from Google Alert - anonymous http://ift.tt/1YYyPCs
via IFTTT
[FD] Bashi v1.6 iOS - Persistent Mail Encoding Vulnerability
#Note:The template file will be copied to a new file. When you change the code of the template file you can create new file with& nbsp;this base code.
echo
Source: Gmail -> IFTTT-> Blogger
[FD] Bugcrowd Bug Bounty #7 - Persistent Web Vulnerability
Hi "><[PERSISTENT INJECTED SCRIPT CODE EXECUTION!]")" <="" "=""><iframe src=a onload=alert("PENTEST") <,</p> <p style="margin-bottom: 1em; -webkit-text-size-adjust:100%; -ms-text-size-adjust:100%">As you may have&nbsp;already heard, this morning we <a href="http://ift.tt/1TDBMXY" style="-webkit-text-size-adjust:100%; -ms-text-size-adjust:100%" data-hs-link-id="0">announced our $15M Series B funding</a>. We're proud to have many of our previous investors joining us again, as well as pleased to welcome new investors to the family. <br><br>We couldn't have done this without you. With your help we've built a massive&nbsp;community of hackers, made up of diverse and talented folks from all over the world.<br><br>We've got lots of plans for what's next and I've shared those details on our blog. Please check it out to learn more.<br><br><strong><a href="http://ift.tt/1OW0AeP" style="-webkit-text-size-adjust:100%; -ms-text-size-adjust:100%" data-hs-link-id="1">Read about our plans for Bugcrowd's next chapter</a>.</strong> </p><p style="margin-bottom: 1em; -webkit-text-size-adjust:100%; -ms-text-size-adjust:100%">Thank you joining us on this journey. There's so much more to come! We hope you'll <a href="https://twitter.com/Bugcrowd/status/722904657366884353" style="-webkit-text-size-adjust:100%; -ms-text-size-adjust:100%" data-hs-link-id="0">join us today in our celebration</a> of this Bugcrowd community milestone.</p> <p style="margin-bottom: 1em; -webkit-text-size-adjust:100%; -ms-text-size-adjust:100%">Sincerely,</p> <p style="margin-bottom: 1em; -webkit-text-size-adjust:100%; -ms-text-size-adjust:100%">Casey Ellis<br>Bugcrowd Founder &amp; CEO</p></div> Solution - Fix & Patch: ======================= The vulnerability can be patched by a secure parse and encode of the vulnerable firstname, lastname and company values in the add POST method request. Disallow the usage of special chars in the name values, encode the inputs as well to prevent further exploitation. Encode as well outgoing emails to ensure that no malicious script code injection can take place with application-side attack vector. Security Risk: ============== The security risk of the filter bypass issue and application-side arbitrary script code injection web vulnerability is estimated as medium. (CVSS 3.8) Credits & Authors: ================== Vulnerability Laboratory [Research Team] - Benjamin Kunz Mejri (research@vulnerability-lab.com) [http://ift.tt/1jnqRwA] [http://ift.tt/1TDrAB7.] Disclaimer & Information: ========================= The information provided in this advisory is provided as it is without any warranty. Vulnerability Lab disclaims all warranties, either expressed or implied, including the warranties of merchantability and capability for a particular purpose. Vulnerability-Lab or its suppliers are not liable in any case of damage, including direct, indirect, incidental, consequential loss of business profits or special damages, even if Vulnerability-Lab or its suppliers have been advised of the possibility of such damages. Some states do not allow the exclusion or limitation of liability for consequential or incidental damages so the foregoing limitation may not apply. We do not approve or encourage anybody to break any licenses, policies, deface websites, hack into databases or trade with stolen data. Domains: http://ift.tt/1jnqRwA - www.vuln-lab.com - http://ift.tt/1kouTut Contact: admin@vulnerability-lab.com - research@vulnerability-lab.com - admin@evolution-sec.com Section: magazine.vulnerability-lab.com - http://ift.tt/1zNuo47 - http://ift.tt/1wo6y8x Social: twitter.com/vuln_lab - http://ift.tt/1kouSqa - http://youtube.com/user/vulnerability0lab Feeds: http://ift.tt/1iS1DH0 - http://ift.tt/1kouSqh - http://ift.tt/1kouTKS Programs: http://ift.tt/1iS1GCs - http://ift.tt/1iS1FyF - http://ift.tt/1oSBx0A Any modified copy or reproduction, including partially usages, of this file requires authorization from Vulnerability Laboratory. Permission to electronically redistribute this alert in its unmodified form is granted. All other rights, including the use of other media, are reserved by Vulnerability-Lab Research Team or its suppliers. All pictures, texts, advisories, source code, videos and other information on this website is trademark of vulnerability-lab team & the specific authors or managers. To record, list, modify, use or edit our material contact (admin@ or research@vulnerability-lab.com) to get a ask permission. Copyright © 2016 | Vulnerability Laboratory - [Evolution Security GmbH]™
Source: Gmail -> IFTTT-> Blogger
[FD] Teampass v2.1.25 - Arbitrary File Download Vulnerability
Source: Gmail -> IFTTT-> Blogger
Milky Way Over the Spanish Peaks
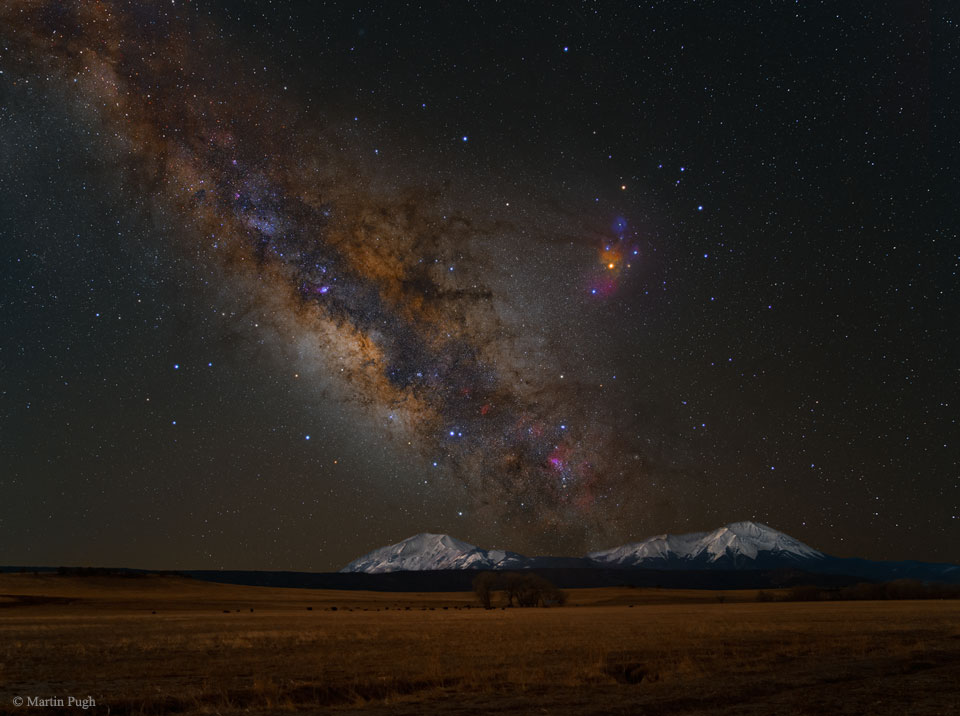