
Latest YouTube Video
Saturday, August 13, 2016
Orioles: RP Darren O'Day (rotator cuff strain) placed on the 15-day DL, P Tyler Wilson recalled from Triple-A (ESPN)
via IFTTT
Theme switching issue with "Cache pages for anonymous users"
from Google Alert - anonymous http://ift.tt/2bpmEwU
via IFTTT
Tennessee Area Convention 2016
from Google Alert - anonymous http://ift.tt/2aJjiYH
via IFTTT
Guccifer 2.0 Leaks Personal Info of Nearly 200 Congressional Democrats
from The Hacker News http://ift.tt/2aRXUBT
via IFTTT
The Easterbunny Comes to NGC 4725
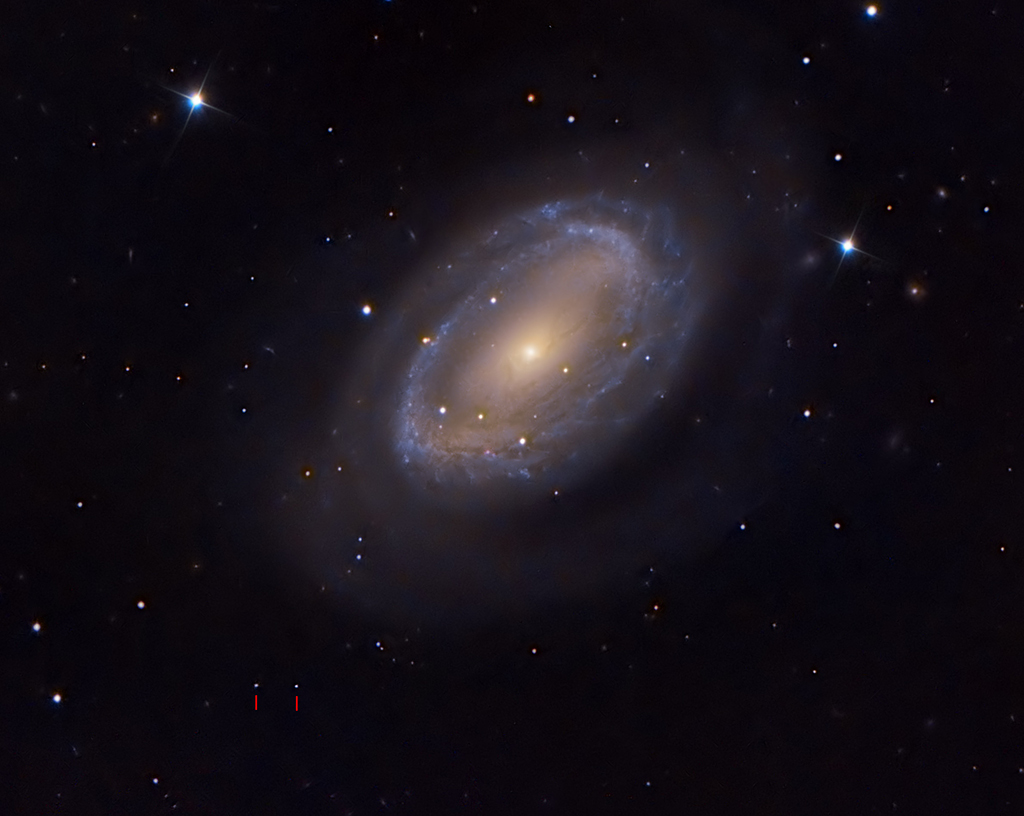
Friday, August 12, 2016
Why am I getting complex values from the following anonymous function?
from Google Alert - anonymous http://ift.tt/2bczocL
via IFTTT
VPN Shield Free - Anonymous Secure Proxy Connection for Private Internet Access and Revoke ...
from Google Alert - anonymous http://ift.tt/2borElD
via IFTTT
[FD] RCE in Teamspeak 3 server
Source: Gmail -> IFTTT-> Blogger
[FD] Zabbix 2.2.x, 3.0.x SQL Injection Vulnerability
Source: Gmail -> IFTTT-> Blogger
[FD] Directory Traversal Vulnerability in ColoradoFTP v1.3 Prime Edition (Build 8)
Source: Gmail -> IFTTT-> Blogger
[FD] Nagios Incident Manager Multiple Vulnerabilities
Source: Gmail -> IFTTT-> Blogger
[FD] Nagios Network Analyzer Multiple Vulnerabilities
Source: Gmail -> IFTTT-> Blogger
[FD] CVE-2016-6483 - vBulletin <= 5.2.2 Preauth Server Side Request Forgery (SSRF)
Source: Gmail -> IFTTT-> Blogger
[FD] Stored XSS in Advanced Custom Fields: Table Field allows authenticated users to do almost anything an admin user can (WordPress plugin)
Source: Gmail -> IFTTT-> Blogger
New Hack Uses Hard Drive's Noise to Transfer Stolen Data from Air-Gapped Computer
from The Hacker News http://ift.tt/2boe6dS
via IFTTT
Anonymous Trump Voters Are Jumping Ship
from Google Alert - anonymous http://ift.tt/2bnWheR
via IFTTT
[FD] NEW VMSA-2016-0011 - vRealize Log Insight update addresses directory traversal vulnerability.
Source: Gmail -> IFTTT-> Blogger
I have a new follower on Twitter
elearninginfographic
#eLearning Infographics -The No.1 Source for the #Best #Education #Infographics - Submit Yours for Free! http://t.co/AzHzIZ7Tux
USA
http://t.co/Pl5r4Di12A
Following: 44427 - Followers: 56943
August 12, 2016 at 09:17AM via Twitter http://twitter.com/eLearngraphic
Anonymous donors help increase reward for Henry Gutierrez's killer
from Google Alert - anonymous http://ift.tt/2aPR1AQ
via IFTTT
Sex and Love Addicts Anonymous
from Google Alert - anonymous http://ift.tt/2aNXkit
via IFTTT
Perseid, Aurora, and Noctilucent Clouds
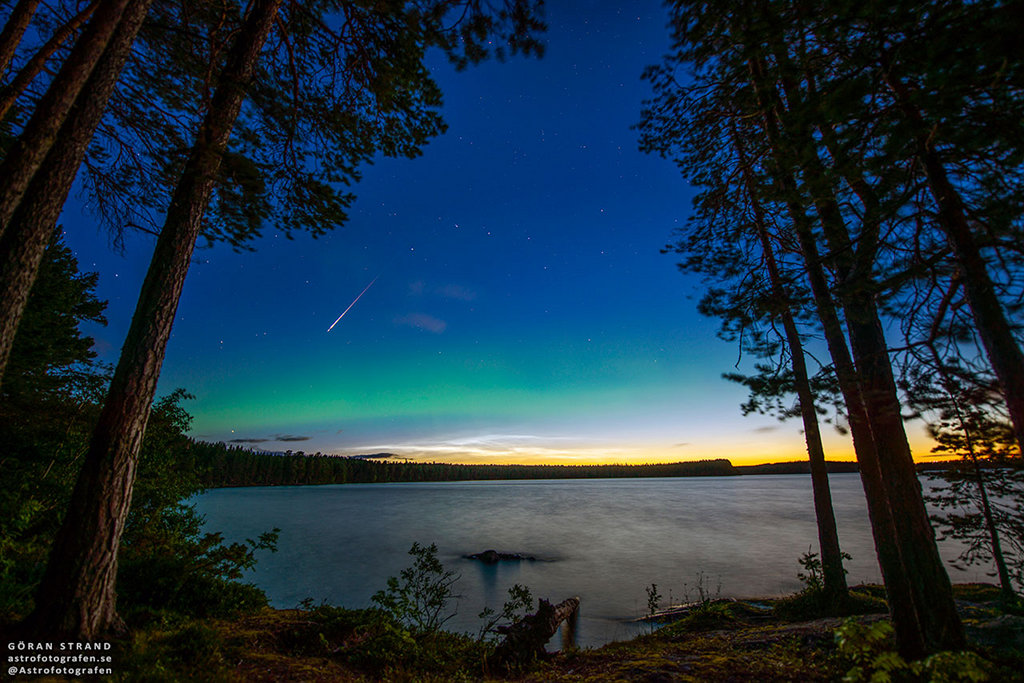
Thursday, August 11, 2016
Banks join the call to end anonymous companies
from Google Alert - anonymous http://ift.tt/2aL0Lqu
via IFTTT
Learning Mobile App Usage Routine through Learning Automata. (arXiv:1608.03507v1 [cs.AI])
Since its conception, smart app market has grown exponentially. Success in the app market depends on many factors among which the quality of the app is a significant contributor, such as energy use. Nevertheless, smartphones, as a subset of mobile computing devices. inherit the limited power resource constraint. Therefore, there is a challenge of maintaining the resource while increasing the target app quality. This paper introduces Learning Automata (LA) as an online learning method to learn and predict the app usage routines of the users. Such prediction can leverage the app cache functionality of the operating system and thus (i) decreases app launch time and (ii) preserve battery. Our algorithm, which is an online learning approach, temporally updates and improves the internal states of itself. In particular, it learns the transition probabilities between app launching. Each App launching instance updates the transition probabilities related to that App, and this will result in improving the prediction. We benefit from a real-world lifelogging dataset and our experimental results show considerable success with respect to the two baseline methods that are used currently for smartphone app prediction approaches.
from cs.AI updates on arXiv.org http://ift.tt/2aPnoQa
via IFTTT
QPass: a Merit-based Evaluation of Soccer Passes. (arXiv:1608.03532v1 [cs.AI])
Quantitative analysis of soccer players' passing ability focuses on descriptive statistics without considering the players' real contribution to the passing and ball possession strategy of their team. Which player is able to help the build-up of an attack, or to maintain the possession of the ball? We introduce a novel methodology called QPass to answer questions like these quantitatively. Based on the analysis of an entire season, we rank the players based on the intrinsic value of their passes using QPass. We derive an album of pass trajectories for different gaming styles. Our methodology reveals a quite counterintuitive paradigm: losing the ball possession could lead to better chances to win a game.
from cs.AI updates on arXiv.org http://ift.tt/2bmiupV
via IFTTT
Online Context-Dependent Clustering in Recommendations based on Exploration-Exploitation Algorithms. (arXiv:1608.03544v1 [cs.LG])
We investigate two context-dependent clustering techniques for content recommendation based on exploration-exploitation strategies in contextual multi-armed bandit settings. Our algorithms dynamically group users based on the items under consideration and, possibly, group items based on the similarity of the clusterings induced over the users. The resulting algorithm thus takes advantage of preference patterns in the data in a way akin to collaborative filtering methods. We provide an empirical analysis on extensive real-world datasets, showing scalability and increased prediction performance over state-of-the-art methods for clustering bandits. For one of the two algorithms we also give a regret analysis within a standard linear stochastic noise setting.
from cs.AI updates on arXiv.org http://ift.tt/2bmj86F
via IFTTT
NESTA, The NICTA Energy System Test Case Archive. (arXiv:1411.0359v5 [cs.AI] UPDATED)
In recent years the power systems research community has seen an explosion of work applying operations research techniques to challenging power network optimization problems. Regardless of the application under consideration, all of these works rely on power system test cases for evaluation and validation. However, many of the well established power system test cases were developed as far back as the 1960s with the aim of testing AC power flow algorithms. It is unclear if these power flow test cases are suitable for power system optimization studies. This report surveys all of the publicly available AC transmission system test cases, to the best of our knowledge, and assess their suitability for optimization tasks. It finds that many of the traditional test cases are missing key network operation constraints, such as line thermal limits and generator capability curves. To incorporate these missing constraints, data driven models are developed from a variety of publicly available data sources. The resulting extended test cases form a compressive archive, NESTA, for the evaluation and validation of power system optimization algorithms.
from cs.AI updates on arXiv.org http://ift.tt/1uoiZX6
via IFTTT
Q($\lambda$) with Off-Policy Corrections. (arXiv:1602.04951v2 [cs.AI] UPDATED)
We propose and analyze an alternate approach to off-policy multi-step temporal difference learning, in which off-policy returns are corrected with the current Q-function in terms of rewards, rather than with the target policy in terms of transition probabilities. We prove that such approximate corrections are sufficient for off-policy convergence both in policy evaluation and control, provided certain conditions. These conditions relate the distance between the target and behavior policies, the eligibility trace parameter and the discount factor, and formalize an underlying tradeoff in off-policy TD($\lambda$). We illustrate this theoretical relationship empirically on a continuous-state control task.
from cs.AI updates on arXiv.org http://ift.tt/249cwhO
via IFTTT
Media members dish on Texas in anonymous survey: Longhorns to win more than eight games?
from Google Alert - anonymous http://ift.tt/2aPT584
via IFTTT
Undefined index: anonymous in content_access_get_role_gid
from Google Alert - anonymous http://ift.tt/2blnejt
via IFTTT
Ravens: Joe Flacco tells Britt McHenry he is not playing in Thursday's preseason opener against the Panthers (ESPN)
via IFTTT
Anonymous Tip Leads to Arrest of Gary Man for Dealing Drugs
from Google Alert - anonymous http://ift.tt/2aF7TJg
via IFTTT
Car Thieves Can Unlock 100 Million Volkswagens With A Simple Wireless Hack
from The Hacker News http://ift.tt/2b8AzqS
via IFTTT
I have a new follower on Twitter
Supergiant
The first container orchestration system that makes it easy to scale stateful, distributed apps. DevOps friendly. Built on Kubernetes.
https://t.co/4ZhxjZAI0N
Following: 1938 - Followers: 201
August 11, 2016 at 12:42PM via Twitter http://twitter.com/supergiantio
Links are rendered without the subdirectory path for anonymous users on multisites hosted in ...
from Google Alert - anonymous http://ift.tt/2blsKyJ
via IFTTT
Links are rendered without the subdirectory path for anonymous users on multisites hosted in ...
from Google Alert - anonymous http://ift.tt/2blsKyJ
via IFTTT
ISS Daily Summary Report – 08/10/2016
from ISS On-Orbit Status Report http://ift.tt/2aOqyzS
via IFTTT
Linux TCP Flaw allows Hackers to Hijack Internet Traffic and Inject Malware Remotely
from The Hacker News http://ift.tt/2aIJ4rD
via IFTTT
dc entertainment adds another rising star to its talent roster
from Google Alert - anonymous http://ift.tt/2aNvXuL
via IFTTT
[FD] QuickerBB 0.7.0 - Register Cross Site Scripting Vulnerability
[FD] Microsoft Education - Stored Cross Site Web Vulnerability
Source: Gmail -> IFTTT-> Blogger
Blackhat Firm Offers $500,000 for Zero-day iOS Exploit; Double Than Apple’s Highest Bounty
from The Hacker News http://ift.tt/2aNZMHL
via IFTTT
Colliding Galaxies in Stephans Quintet
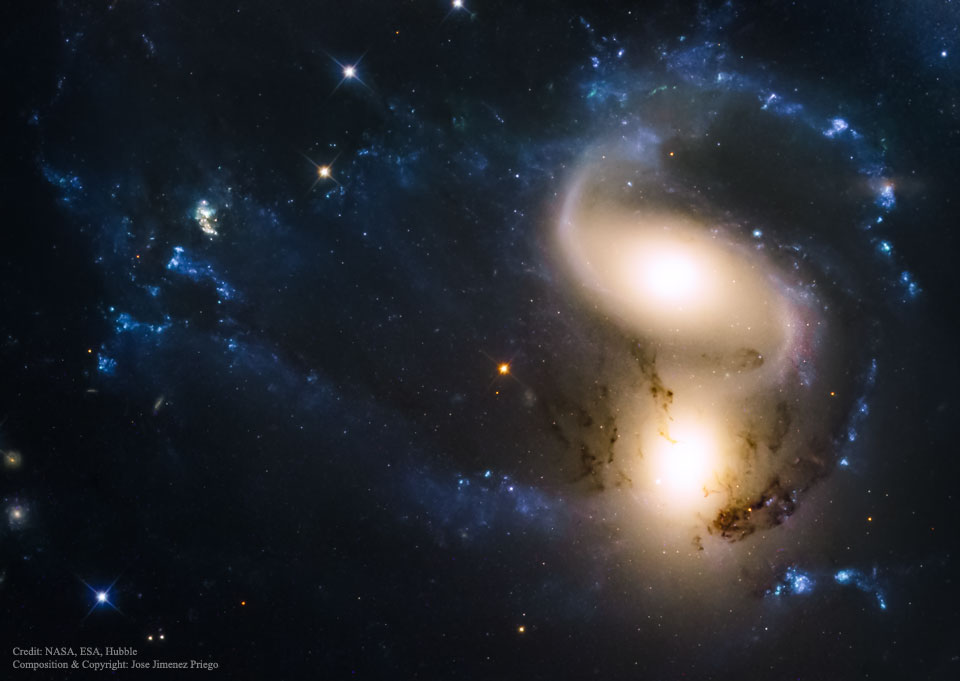
Wednesday, August 10, 2016
Stopping Anonymous Proxy Login Attacks?
from Google Alert - anonymous http://ift.tt/2aU2nRU
via IFTTT
Neuroevolution-Based Inverse Reinforcement Learning. (arXiv:1608.02971v1 [cs.NE])
The problem of Learning from Demonstration is targeted at learning to perform tasks based on observed examples. One approach to Learning from Demonstration is Inverse Reinforcement Learning, in which actions are observed to infer rewards. This work combines a feature based state evaluation approach to Inverse Reinforcement Learning with neuroevolution, a paradigm for modifying neural networks based on their performance on a given task. Neural networks are used to learn from a demonstrated expert policy and are evolved to generate a policy similar to the demonstration. The algorithm is discussed and evaluated against competitive feature-based Inverse Reinforcement Learning approaches. At the cost of execution time, neural networks allow for non-linear combinations of features in state evaluations. These valuations may correspond to state value or state reward. This results in better correspondence to observed examples as opposed to using linear combinations. This work also extends existing work on Bayesian Non-Parametric Feature Construction for Inverse Reinforcement Learning by using non-linear combinations of intermediate data to improve performance. The algorithm is observed to be specifically suitable for a linearly solvable non-deterministic Markov Decision Processes in which multiple rewards are sparsely scattered in state space. A conclusive performance hierarchy between evaluated algorithms is presented.
from cs.AI updates on arXiv.org http://ift.tt/2aC7CXz
via IFTTT
Neural Generation of Regular Expressions from Natural Language with Minimal Domain Knowledge. (arXiv:1608.03000v1 [cs.CL])
This paper explores the task of translating natural language queries into regular expressions which embody their meaning. In contrast to prior work, the proposed neural model does not utilize domain-specific crafting, learning to translate directly from a parallel corpus. To fully explore the potential of neural models, we propose a methodology for collecting a large corpus of regular expression, natural language pairs. Our resulting model achieves a performance gain of 19.6% over previous state-of-the-art models.
from cs.AI updates on arXiv.org http://ift.tt/2aHFX3e
via IFTTT
Towards Visual Type Theory as a Mathematical Tool and Mathematical User Interface. (arXiv:1608.03026v1 [cs.AI])
A visual type theory is a cognitive tool that has much in common with language, and may be regarded as an exceptional form of spatial text adjunct. A mathematical visual type theory, called NPM, has been under development that can be viewed as an early-stage project in mathematical knowledge management and mathematical user interface development. We discuss in greater detail the notion of a visual type theory, report on progress towards a usable mathematical visual type theory, and discuss the outlook for future work on this project.
from cs.AI updates on arXiv.org http://ift.tt/2b5i0Uh
via IFTTT
The Quadripolar Relational Model: an Artificial Intelligence framework for the description of personality disorders. (arXiv:1512.05875v3 [q-bio.NC] UPDATED)
Borderline personality disorder and narcissistic personality disorder are important nosographic entities and have been subject of intensive investigations. The currently prevailing psycodynamic theory for mental disorders is based on the repertoire of defense mechanisms employed. Another fruitful line of research is concerned with the study of psychological traumas and on dissociation as a defensive response. Both theories can be used to shed light on some aspects of pathological mental functioning, and have many points of contact. This work tries to merge these two psychological theories, and conceive a common framework for the description of personality disorders.
from cs.AI updates on arXiv.org http://ift.tt/1QAee7l
via IFTTT
Ravens will air Michael Phelps' 200-meter individual medley final on the video boards during Thursday's preseason game (ESPN)
via IFTTT
Webpack build breaks ability to use npm installed custom components · Issue #1624
from Google Alert - anonymous http://ift.tt/2aTwlpk
via IFTTT
[FD] [CORE-2016-0006] - SAP CAR Multiple Vulnerabilities
Source: Gmail -> IFTTT-> Blogger
Oops! Microsoft Accidentally Leaks Backdoor Keys to Bypass UEFI Secure Boot
from The Hacker News http://ift.tt/2b3Uo2l
via IFTTT
I have a new follower on Twitter
Matt Delgado
LongLiveBryant | #RU20 | sc:dellio757
Newport News, VA
Following: 776 - Followers: 1420
August 10, 2016 at 11:40AM via Twitter http://twitter.com/DelgotHoes
Ravens Video: Ex-Baltimore RB Ray Rice believes he'll get a 2nd chance in NFL; \"In my heart of hearts it will happen\" (ESPN)
via IFTTT
ImageNet classification with Python and Keras
Normally, I only publish blog posts on Monday, but I’m so excited about this one that it couldn’t wait and I decided to hit the publish button early.
You see, just a few days ago, François Chollet pushed three Keras models (VGG16, VGG19, and ResNet50) online — these networks are pre-trained on the ImageNet dataset, meaning that they can recognize 1,000 common object classes out-of-the-box.
To utilize these models in your own applications, all you need to do is:
- Install Keras.
- Clone the deep-learning-models repository.
- Download the weights files for the pre-trained network(s) (which we’ll be done automatically for you when you import and instantiate the respective network architecture).
- Apply the pre-trained ImageNet networks to your own images.
It’s really that simple.
So, why is this so exciting? I mean, we’ve had the weights to popular pre-trained ImageNet classification networks for awhile, right?
The problem is that these weight files are in Caffe format — and while the Caffe library may be the current standard for which many researchers use to construct new network architectures, train them, and evaluate them, Caffe also isn’t the most Python-friendly library in the world, at least in terms of constructing the network architecture itself.
Note: You can do some pretty cool stuff with the Caffe-Python bindings, but I’m mainly focusing on how Caffe architectures and the training process itself is defined via
.prototxtconfiguration files rather than code that logic can be inserted into.
There is also the fact that there isn’t an easy or streamlined method to convert Caffe weights to a Keras-compatible model.
That’s all starting to change now — we can now easily apply VGG16, VGG19, and ResNet50 using Keras and Python to our own applications without having to worry about the Caffe => Keras weight conversion process.
In fact, it’s now as simple as these three lines of code to classify an image using a Convolutional Neural Network pre-trained on the ImageNet dataset with Python and Keras:
model = VGG16(weights="imagenet") preds = model.predict(preprocess_input(image)) print(decode_predictions(preds))
Of course, there are a few other imports and helper functions that need to be utilized — but I think you get the point:
It’s now dead simple to apply ImageNet-level pre-trained networks using Python and Keras.
To find out how, keep reading.
Looking for the source code to this post?
Jump right to the downloads section.
ImageNet classification with Python and Keras
In the remainder of this tutorial, I’ll explain what the ImageNet dataset is, and then provide Python and Keras code to classify images into 1,000 different categories using state-of-the-art network architectures.
What is ImageNet?
Within computer vision and deep learning communities, you might run into a bit of contextual confusion surrounding what ImageNet is and what it isn’t.
You see, ImageNet is actually a project aimed at labeling and categorizing images into almost 22,000 categories based on a defined set of words and phrases. At the time of this writing, there are over 14 million images in the ImageNet project.
So, how is ImageNet organized?
To order such a massive amount of data, ImageNet actually follows the WordNet hierarchy. Each meaningful word/phrase inside WordNet is called a “synonym set” or “synset” for short. Within the ImageNet project, images are organized according to these synsets, with the goal being to have 1,000+ images per synset.
ImageNet Large Scale Recognition Challenge (ILSVRC)
In the context of computer vision and deep learning, whenever you hear people talking about ImageNet, they are very likely referring to the ImageNet Large Scale Recognition Challenge, or simply ILSVRC for short.
The goal of the image classification track in this challenge is to train a model that can classify an image into 1,000 separate categories using over 100,000 test images — the training dataset itself consists of approximately 1.2 million images.
Be sure to keep the context of ImageNet in mind when you’re reading the remainder of this blog post or other tutorials and papers related to ImageNet. While in the context of image classification, object detection, and scene understanding, we often refer to ImageNet as the classification challenge and the dataset associated with the challenge, remember that there is also a more broad project called ImageNet where these images are collected, annotated, and organized.
Configuring your system for Keras and ImageNet
To configure your system to use the state-of-the-art VGG16, VGG19, and ResNet50 networks, make sure you follow my previous tutorial on installing Keras.
The Keras library will use PIL/Pillow for some helper functions (such as loading an image from disk). You can install Pillow, the more Python friendly fork of PIL, by using this command:
$ pip install pillow
To run the networks pre-trained on the ImageNet dataset with Python, you’ll need to make sure you have the latest version of Keras installed. At the time of this writing, the latest version of Keras is
1.0.6, the minimum requirement for utilizing the pre-trained models.
You can check your version of Keras by executing the following commands:
$ python >>> import keras Using Theano backend. Using gpu device 1: GeForce GTX TITAN X (CNMeM is disabled, cuDNN 4007) >>> keras.__version__ '1.0.6' >>>
Alternatively, you can use
pip freezeto list the out the packages installed in your environment:
Figure 1: Listing the set of Python packages installed in your environment.
If you are using an earlier version of Keras prior to
1.0.6, uninstall it, and then use my previous tutorial to install the latest version.
Next, to gain access to VGG16, VGG19, and the ResNet50 architectures and pre-trained weights, you need to clone the deep-learning-models repository from GitHub:
$ git clone http://ift.tt/2ajePvK
From there, change into the
deep-learning-modelsdirectory and
lsthe contents:
$ cd deep-learning-models $ ls -l total 40 -rw-rw-r-- 1 adrian adrian 1233 Aug 6 11:20 imagenet_utils.py -rw-rw-r-- 1 adrian adrian 1074 Aug 6 11:20 LICENSE -rw-rw-r-- 1 adrian adrian 2569 Aug 6 11:20 README.md -rw-rw-r-- 1 adrian adrian 10258 Aug 6 11:20 resnet50.py -rw-rw-r-- 1 adrian adrian 7225 Aug 6 11:20 vgg16.py -rw-rw-r-- 1 adrian adrian 7508 Aug 6 11:20 vgg19.py
Notice how we have four Python files. The
resnet50.py,
vgg16.py, and
vgg19.pyfiles correspond to their respective network architecture definitions.
The
imagenet_utilsfile, as the name suggests, contains a couple helper functions that allow us to prepare images for classification as well as obtain the final class label predictions from the network.
Keras and Python code for ImageNet CNNs
We are now ready to write some Python code to classify image contents utilizing Convolutional Neural Networks (CNNs) pre-trained on the ImageNet dataset.
To start, open up a new file, name it
test_imagenet.py, and insert the following code:
# import the necessary packages from keras.preprocessing import image as image_utils from imagenet_utils import decode_predictions from imagenet_utils import preprocess_input from vgg16 import VGG16 import numpy as np import argparse import cv2 # construct the argument parse and parse the arguments ap = argparse.ArgumentParser() ap.add_argument("-i", "--image", required=True, help="path to the input image") args = vars(ap.parse_args()) # load the original image via OpenCV so we can draw on it and display # it to our screen later orig = cv2.imread(args["image"])
We start on Lines 2-8 by importing our required Python packages. Line 2 imports the
imagepre-processing module directly from the Keras library. However, Lines 3-5 import functions and network architectures from within the
deep-learning-modelsdirectory. Because of this, you’ll want to make sure your
test_imagenet.pyfile is inside the
deep-learning-modelsdirectory (or your
PYTHONPATHis updated accordingly), otherwise your script will fail to import these functions.
Alternatively, you can use the “Downloads” section at the bottom of this tutorial to download the source code + example images. This download ensures the code is configured correctly and that your directory structure is setup properly.
Lines 11-14 parse our command line arguments. We only need a single switch here,
--image, which is the path to our input image.
We then load our image in OpenCV format on Line 18. This step isn’t strictly required since Keras provides helper functions to load images (which I’ll demonstrate in the next code block), but there are differences in how both these functions work, so if you intend on applying any type of OpenCV functions to your images, I suggest loading your image via
cv2.imreadand then again via the Keras helpers. Once you get a bit more experience manipulating NumPy arrays and swapping channels, you can avoid the extra I/O overhead, but for the time being, let’s keep things simple.
# import the necessary packages from keras.preprocessing import image as image_utils from imagenet_utils import decode_predictions from imagenet_utils import preprocess_input from vgg16 import VGG16 import numpy as np import argparse import cv2 # construct the argument parse and parse the arguments ap = argparse.ArgumentParser() ap.add_argument("-i", "--image", required=True, help="path to the input image") args = vars(ap.parse_args()) # load the original image via OpenCV so we can draw on it and display # it to our screen later orig = cv2.imread(args["image"]) # load the input image using the Keras helper utility while ensuring # that the image is resized to 224x224 pxiels, the required input # dimensions for the network -- then convert the PIL image to a # NumPy array print("[INFO] loading and preprocessing image...") image = image_utils.load_img(args["image"], target_size=(224, 224)) image = image_utils.img_to_array(image)
Line 25 applies the
.load_imgKeras helper function to load our image from disk. We supply a
target_sizeof 224 x 224 pixels, the required spatial input image dimensions for the VGG16, VGG19, and ResNet50 network architectures.
After calling
.load_img, our
imageis actually in PIL/Pillow format, so we need to apply the
.img_to_arrayfunction to convert the
imageto a NumPy format.
Next, let’s preprocess our image:
# import the necessary packages from keras.preprocessing import image as image_utils from imagenet_utils import decode_predictions from imagenet_utils import preprocess_input from vgg16 import VGG16 import numpy as np import argparse import cv2 # construct the argument parse and parse the arguments ap = argparse.ArgumentParser() ap.add_argument("-i", "--image", required=True, help="path to the input image") args = vars(ap.parse_args()) # load the original image via OpenCV so we can draw on it and display # it to our screen later orig = cv2.imread(args["image"]) # load the input image using the Keras helper utility while ensuring # that the image is resized to 224x224 pxiels, the required input # dimensions for the network -- then convert the PIL image to a # NumPy array print("[INFO] loading and preprocessing image...") image = image_utils.load_img(args["image"], target_size=(224, 224)) image = image_utils.img_to_array(image) # our image is now represented by a NumPy array of shape (3, 224, 224), # but we need to expand the dimensions to be (1, 3, 224, 224) so we can # pass it through the network -- we'll also preprocess the image by # subtracting the mean RGB pixel intensity from the ImageNet dataset image = np.expand_dims(image, axis=0) image = preprocess_input(image)
If at this stage we inspect the
.shapeof our
image, you’ll notice the shape of the NumPy array is (3, 224, 224) — each image is 224 pixels wide, 224 pixels tall, and has 3 channels (one for each of the Red, Green, and Blue channels, respectively).
However, before we can pass our
imagethrough our CNN for classification, we need to expand the dimensions to be (1, 3, 224, 224).
Why do we do this?
When classifying images using Deep Learning and Convolutional Neural Networks, we often send images through the network in “batches” for efficiency. Thus, it’s actually quite rare to pass only one image at a time through the network — unless of course, you only have one image to classify (like we do).
We then preprocess the
imageon Line 33 by subtracting the mean RGB pixel intensity computed from the ImageNet dataset.
Finally, we can load our Keras network and classify the image:
# import the necessary packages from keras.preprocessing import image as image_utils from imagenet_utils import decode_predictions from imagenet_utils import preprocess_input from vgg16 import VGG16 import numpy as np import argparse import cv2 # construct the argument parse and parse the arguments ap = argparse.ArgumentParser() ap.add_argument("-i", "--image", required=True, help="path to the input image") args = vars(ap.parse_args()) # load the original image via OpenCV so we can draw on it and display # it to our screen later orig = cv2.imread(args["image"]) # load the input image using the Keras helper utility while ensuring # that the image is resized to 224x224 pxiels, the required input # dimensions for the network -- then convert the PIL image to a # NumPy array print("[INFO] loading and preprocessing image...") image = image_utils.load_img(args["image"], target_size=(224, 224)) image = image_utils.img_to_array(image) # our image is now represented by a NumPy array of shape (3, 224, 224), # but we need to expand the dimensions to be (1, 3, 224, 224) so we can # pass it through the network -- we'll also preprocess the image by # subtracting the mean RGB pixel intensity from the ImageNet dataset image = np.expand_dims(image, axis=0) image = preprocess_input(image) # load the VGG16 network print("[INFO] loading network...") model = VGG16(weights="imagenet") # classify the image print("[INFO] classifying image...") preds = model.predict(image) (inID, label) = decode_predictions(preds)[0] # display the predictions to our screen print("ImageNet ID: {}, Label: {}".format(inID, label)) cv2.putText(orig, "Label: {}".format(label), (10, 30), cv2.FONT_HERSHEY_SIMPLEX, 0.9, (0, 255, 0), 2) cv2.imshow("Classification", orig) cv2.waitKey(0)
On Line 37 we initialize our
VGG16class. We could also substitute in
VGG19or
ResNet50here, but for the sake of this tutorial, we’ll use
VGG16.
Supplying
weights="imagenet"indicates that we want to use the pre-trained ImageNet weights for the respective model.
Once the network has been loaded and initialized, we can predict class labels by making a call to the
.predictmethod of the
model. These predictions are actually a NumPy array with 1,000 entries — the predicted probabilities associated with each class in the ImageNet dataset.
Calling
decode_predictionson these predictions gives us the ImageNet Unique ID of the label, along with a human-readable text version of the label.
Finally, Lines 45-49 print the predicted
labelto our terminal and display the output image to our screen.
ImageNet + Keras image classification results
To apply the Keras models pre-trained on the ImageNet dataset to your own images, make sure you use the “Downloads” form at the bottom of this blog post to download the source code and example images. This will ensure your code is properly formatted (without errors) and your directory structure is correct.
But before we can apply our pre-trained Keras models to our own images, let’s first discuss how the model weights are (automatically) downloaded.
Downloading the model weights
The first time you execute the
test_imagenet.pyscript, Keras will automatically download and cache the architecture weights to your disk in the
~/.keras/modelsdirectory.
Subsequent runs of
test_imagenet.pywill be substantially faster (since the network weights will already be downloaded) — but that first run will be quite slow (comparatively), due to the download process.
That said, keep in mind that these weights are fairly large HDF5 files and might take awhile to download if you do not have a fast internet connection. For convenience, I have listed out the size of the weights files for each respective network architecture:
- ResNet50: 102MB
- VGG16: 553MB
- VGG19: 574MB
ImageNet and Keras results
We are now ready to classify images using the pre-trained Keras models! To test out the models, I downloaded a couple images from Wikipedia (“brown bear” and “space shuttle”) — the rest are from my personal library.
To start, execute the following command:
$ python test_imagenet.py --image images/dog_beagle.png
Notice that since this is my first run of
test_imagenet.py, the weights associated with the VGG16 ImageNet model need to be downloaded:
Figure 2: Downloading the pre-trained ImageNet weights for VGG16.
Once our weights are downloaded, the VGG16 network is initialized, the ImageNet weights loaded, and the final classification is obtained:
Figure 3: Utilizing the VGG16 network trained on ImageNet to recognize a beagle (dog) in an image.
Let’s give another image a try, this one of a beer glass:
$ python test_imagenet.py --image images/beer.png
Figure 4: Recognizing a beer glass using a Convolutional Neural Network trained on ImageNet.
The following image is of a brown bear:
$ python test_imagenet.py --image images/brown_bear.png
Figure 5: Utilizing VGG16, Keras, and Python to recognize the brown bear in an image.
I took the following photo of my keyboard to test out the ImageNet network using Python and Keras:
$ python test_imagenet.py --image images/keyboard.png
Figure 6: Utilizing Python, Keras, and a Convolutional Neural Network trained on ImageNet to recognize image contents.
I then took a photo of my monitor as I was writing the code for this blog post. Interestingly, the network classified this image as “desktop computer”, which makes sense given that the monitor is the primary subject of the image:
$ python test_imagenet.py --image images/monitor.png
Figure 7: Image classification via Python, Keras, and CNNs.
This next image is of a space shuttle:
$ python test_imagenet.py --image images/space_shuttle.png
Figure 8: Recognizing image contents using a Convolutional Neural Network trained on ImageNet via Keras + Python.
The final image is of a steamed crab, a blue crab, to be specific:
$ python test_imagenet.py --image images/steamed_crab.png
Figure 9: Convolutional Neural Networks and ImageNet for image classification with Python and Keras.
What I find interesting about this particular example is that VGG16 classified this image as “Dungeness crab”, which may be technically incorrect. However, keep in mind that blue crabs are called blue crabs for a reason — their outer shell is blue. It is not until you steam them for eating do their shells turn red. The Dungeness crab on the other hand has a slightly dark orange tint to it, even before steaming. The fact that the network was even able to label this image as “crab” is very impressive.
A note on model timing
From start to finish (not including the downloading of the network weights files), classifying an image using VGG16 took approximately 11 seconds on my Titan X GPU. This includes the process of actually loading both the image and network from disk, performing any initializations, passing the image through the network, and obtaining the final predictions.
However, once the network is actually loaded into memory, classification takes only 1.8 seconds, which goes to show you how much overhead is involved in actually loading an initializing a large Convolutional Neural Network. Furthermore, since images can be presented to the network in batches, this same time for classification will hold for multiple images.
If you’re classifying images on your CPU, then you should obtain a similar classification time. This is mainly because there is substantial overhead in copying the image from memory over to the GPU. When you pass multiple images via batches, it makes the I/O overhead for using the GPU more acceptable.
Summary
In this blog post, I demonstrated how to use the newly released deep-learning-models repository to classify image contents using state-of-the-art Convolutional Neural Networks trained on the ImageNet dataset.
To accomplish this, we leveraged the Keras library, which is maintained by François Chollet — be sure to reach out to him and say thanks for maintaining such an incredible library. Without Keras, deep learning with Python wouldn’t be half as easy (or as fun).
Of course, you might be wondering how to train your own Convolutional Neural Network from scratch using ImageNet. Don’t worry, we’re getting there — we just need to understand the basics of neural networks, machine learning, and deep learning first. Walk before you run, so to speak.
I’ll be back next week with a tutorial on hyperparameter tuning, a key step to maximizing your model’s accuracy.
To be notified when future blog posts are published on the PyImageSearch blog, be sure to enter your email address in the form below — se you next week!
Downloads:
The post ImageNet classification with Python and Keras appeared first on PyImageSearch.
from PyImageSearch http://ift.tt/2bf1Usw
via IFTTT
Anonymous Consequences
from Google Alert - anonymous http://ift.tt/2aZ4ROu
via IFTTT
ISS Daily Summary Report – 08/09/2016
from ISS On-Orbit Status Report http://ift.tt/2aYTjun
via IFTTT
[FD] SEC Consult SA-20160810-0 :: Multiple vulnerabilities in LINE instant messenger platform
Source: Gmail -> IFTTT-> Blogger
Microsoft Releases 9 Security Updates to Patch 38 Vulnerabilities
from The Hacker News http://ift.tt/2b2BEjK
via IFTTT
Tuesday, August 9, 2016
I have a new follower on Twitter
Dr. Liz Hardy
At https://t.co/ib7zlO9dbS, I show online teachers how to work smarter. These #elearning tactics mean you can do a stunning job – without working 24/7.
New Zealand
https://t.co/9gfxgiJYll
Following: 9702 - Followers: 10993
August 09, 2016 at 11:39PM via Twitter http://twitter.com/SimpliTeach
Appendix B: Collection of Anonymous Data
from Google Alert - anonymous http://ift.tt/2aJ5Lvu
via IFTTT