Latest YouTube Video
Saturday, September 16, 2017
Ravens promote RB Alex Collins from practice squad, waive RB Jeremy Langford (ESPN)
via IFTTT
ONLINE==LIVE||"""" Eels vs Cowboys 2017 Live Streaming..2017
from Google Alert - anonymous http://ift.tt/2w0ENuO
via IFTTT
Vevo Music Video Service Hacked — 3.12TB of Internal Data Leaked
from The Hacker News http://ift.tt/2wiIXlK
via IFTTT
Live STream: NZ All Blacks Rugby Live Online
from Google Alert - anonymous http://ift.tt/2h9CfIh
via IFTTT
Anonymous Member - Profile
from Google Alert - anonymous http://ift.tt/2h9cCTV
via IFTTT
Racists Anonymous
from Google Alert - anonymous http://ift.tt/2x37QBK
via IFTTT
Book "Order Outline" tab shows for Anonymous users
from Google Alert - anonymous http://ift.tt/2fpyqL0
via IFTTT
Soccer Exec Slams Feds' Anonymous Jury Bid For FIFA Trial
from Google Alert - anonymous http://ift.tt/2xF5EB7
via IFTTT
Cassini s Final Image
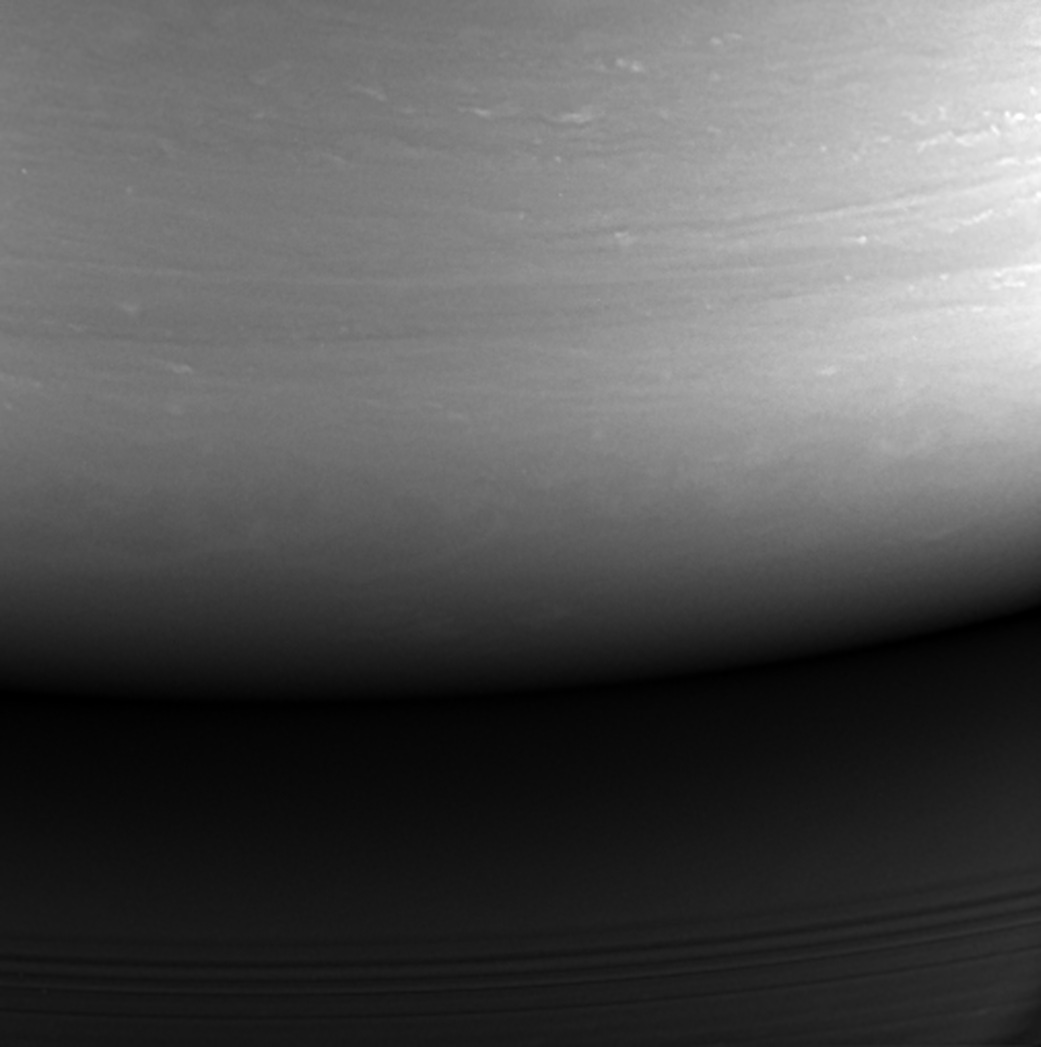
Friday, September 15, 2017
Ravens: RB Kenneth Dixon issued 2nd suspension in 6 months after violating substance abuse policy (ESPN)
via IFTTT
Anonymous user 7eefb0
from Google Alert - anonymous http://ift.tt/2wi5KhN
via IFTTT
I have a new follower on Twitter
Touchdown Trophy
Always looking for the big score! Expect to find NFL news and notes on this account, and a lot of them,
Boston, MA
Following: 199 - Followers: 235
September 15, 2017 at 08:17PM via Twitter http://twitter.com/TrophyTouchdown
White House Staffers May Get Legal Fund For Russia Probe
from Google Alert - anonymous http://ift.tt/2jvUSqv
via IFTTT
I have a new follower on Twitter
Tiffany Monroe
Social Media Enthusiast, Entrepreneur, Blogger, Traveler, Animal Lover and Lover of Life.
Florida, USA
Following: 1477 - Followers: 2559
September 15, 2017 at 04:02PM via Twitter http://twitter.com/Tiffany_Monro72
I have a new follower on Twitter
Jeff Bullas
#1 Content Marketing Influencer,Social Media Marketing Strategist & Speaker,Forbes Top 10 Social Media Influencer,#1 Global Business Blog.
Sydney, Australia
https://t.co/AwDthTHp7P
Following: 238653 - Followers: 552462
September 15, 2017 at 03:47PM via Twitter http://twitter.com/jeffbullas
[FD] ESA-2017-098: EMC Data Protection Advisor Hardcoded Password Vulnerability
Source: Gmail -> IFTTT-> Blogger
[FD] Updated advisory for CVE-2017-8769 - WhatsApp Issues with Media Files
Source: Gmail -> IFTTT-> Blogger
[FD] Mako Web Server v2.5 Multiple Unauthenticated Vulnerabilities
Source: Gmail -> IFTTT-> Blogger
[FD] Exploit toolkit for CVE-2017-8759 - Microsoft .NET Framework RCE (Builder + listener + video tutorial)
Source: Gmail -> IFTTT-> Blogger
[FD] BSides Roma
Source: Gmail -> IFTTT-> Blogger
Ravens can match 72-year-old feat by pitching second straight shutout on Sunday - Jamison Hensley (ESPN)
via IFTTT
Flix Anonymous - Episode 42
from Google Alert - anonymous http://ift.tt/2yd9mi2
via IFTTT
I have a new follower on Twitter
NFL Fandom
Fans of the world's greatest sport. Sundays in the fall; nothing is better.
Richmond, VA
Following: 175 - Followers: 211
September 15, 2017 at 08:42AM via Twitter http://twitter.com/NFL_Fandom
I have a new follower on Twitter
Salt Life
Surfing, Fishing, Diving, or just enjoying a day on the beach, we all have one thing in common - the ocean and all it has to offer. https://t.co/JDz8v9OadF
LIVE THE SALT LIFE
https://t.co/PjcPxzvGhL
Following: 27694 - Followers: 54601
September 15, 2017 at 08:42AM via Twitter http://twitter.com/RealSaltLife
(Answered) Ajax link for anonymous users to delete node they created if $_SESSION exists. Is ...
from Google Alert - anonymous http://ift.tt/2xph62z
via IFTTT
Yet Another Android Malware Infects Over 4.2 Million Google Play Store Users
from The Hacker News http://ift.tt/2ybfmbb
via IFTTT
75,000 Turks Arrested So Far for Downloading Encrypted Messaging App
from The Hacker News http://ift.tt/2jvc932
via IFTTT
Anonymous os iso download
from Google Alert - anonymous http://ift.tt/2yb4SZk
via IFTTT
Free anonymous surfing
from Google Alert - anonymous http://ift.tt/2weJOUz
via IFTTT
100 Steps Forward
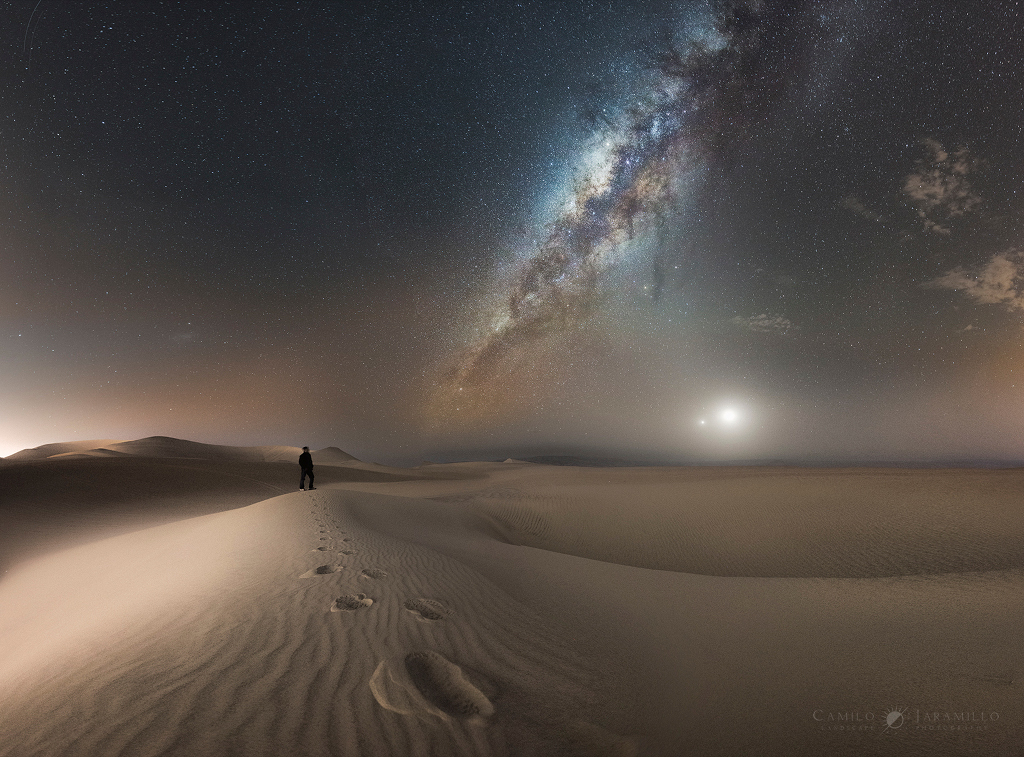
Thursday, September 14, 2017
I have a new follower on Twitter
Pigskin Pride
Love all things football! I cannot get enough of the world's best sport. 25 year old graduate of Alabama #RollTide
Tuscaloosa, AL
Following: 168 - Followers: 190
September 14, 2017 at 09:17PM via Twitter http://twitter.com/PigskinParties
▶ Jeremy Maclin says Joe Flacco "as advertised," the pair has already developed strong chemistry (ESPN)
via IFTTT
I have a new follower on Twitter
ConferTel
ConferTel provides a range of event driven communications applications, including fully managed webinar services, secure audio and web conferences
Carlsbad, California
http://t.co/o1iIAoQ7qY
Following: 1822 - Followers: 1201
September 14, 2017 at 03:57PM via Twitter http://twitter.com/ConferTel
New Book - Anonymous
from Google Alert - anonymous http://ift.tt/2jtnhxv
via IFTTT
Ravens place RB Danny Woodhead (hamstring) on IR, sign RB Jeremy Langford off practice squad (ESPN)
via IFTTT
Windows 10 to Give More Control Over App-level Permissions
from The Hacker News http://ift.tt/2xmxroF
via IFTTT
[FD] SEC Consult SA-20170914-1 :: Persistent Cross-Site Scripting in SilverStripe CMS
Source: Gmail -> IFTTT-> Blogger
[FD] SEC Consult SA-20170914-0 :: Authenticated Command Injection in Ubiquiti Networks UniFi Cloud Key
Source: Gmail -> IFTTT-> Blogger
Equifax Suffered Data Breach After It Failed to Patch Old Apache Struts Flaw
from The Hacker News http://ift.tt/2fkOyxD
via IFTTT
8th Street, Ocean City, MD's surf is at least 5.71ft high
8th Street, Ocean City, MD Summary
At 4:00 AM, surf min of 5.71ft. At 10:00 AM, surf min of 5.17ft. At 4:00 PM, surf min of 4.25ft. At 10:00 PM, surf min of 3.13ft.
Surf maximum: 6.72ft (2.05m)
Surf minimum: 5.71ft (1.74m)
Tide height: 0.77ft (0.23m)
Wind direction: W
Wind speed: 8.71 KTS
from Surfline http://ift.tt/1kVmigH
via IFTTT
Wednesday, September 13, 2017
OEG to allow anonymous donations to Political defense funds
from Google Alert - anonymous http://ift.tt/2wXCBYy
via IFTTT
New Light Shed on Case of Missing Fordyce Mom Thanks to Anonymous
from Google Alert - anonymous http://ift.tt/2jqMI2J
via IFTTT
Ravens: John Harbaugh looks to remain undefeated at home vs. rookie QBs - Jamison Hensley (ESPN)
via IFTTT
Spirit Sand Anonymous
from Google Alert - anonymous http://ift.tt/2f7CCSO
via IFTTT
Feyenoord vs Manchester City Live Streaming
from Google Alert - anonymous http://ift.tt/2xyBu2f
via IFTTT
Ravens: Joe Flacco says he's not 100% but will have "no limitations" Sunday vs. Browns (ESPN)
via IFTTT
Ravens: John Harbaugh says Ray Lewis should be elected to HOF on first ballot; "agenda" if not (ESPN)
via IFTTT
Ravens: Michael Campanaro could fill Danny Woodhead's role - Jamison Hensley (ESPN)
via IFTTT
Ravens: RB Danny Woodhead (hamstring) will miss at least 4 weeks, says John Harbaugh (ESPN)
via IFTTT
Fivestar with comments that need approval removes all anonymous user comments upon approval
from Google Alert - anonymous http://ift.tt/2wZdYZ1
via IFTTT
Ravens put perfect home mark vs. rookie QBs on line against DeShone Kizer - Jamison Hensley (ESPN)
via IFTTT
Zerodium Offers $1 Million for Tor Browser 0-Days That It will Resell to Governments
from The Hacker News http://ift.tt/2f5zxCN
via IFTTT
Immediately Patch Windows 0-Day Flaw That's Being Used to Spread Spyware
from The Hacker News http://ift.tt/2vTG9qV
via IFTTT
8th Street, Ocean City, MD's surf is at least 6.73ft high
8th Street, Ocean City, MD Summary
At 4:00 AM, surf min of 6.73ft. At 10:00 AM, surf min of 5.56ft. At 4:00 PM, surf min of 4.19ft. At 10:00 PM, surf min of 4.45ft.
Surf maximum: 8.04ft (2.45m)
Surf minimum: 6.73ft (2.05m)
Tide height: 1.44ft (0.44m)
Wind direction: NE
Wind speed: 27.8 KTS
from Surfline http://ift.tt/1kVmigH
via IFTTT
[FD] SEC Consult SA-20170913-1 :: Local File Disclosure in VLC media player iOS app
Source: Gmail -> IFTTT-> Blogger
[FD] SEC Consult SA-20170913-0 :: Multiple Vulnerabilities in IBM Infosphere Information Server / Datastage
Source: Gmail -> IFTTT-> Blogger
Linux Subsystem on Windows 10 Allows Malware to Become Fully Undetectable
from The Hacker News http://ift.tt/2woVXBD
via IFTTT
Tuesday, September 12, 2017
To the click handler add an empty anonymous function so that you can add code to it later.
from Google Alert - anonymous http://ift.tt/2f3QjlZ
via IFTTT
Post review for anonymous, save as draft or save unpublished
from Google Alert - anonymous http://ift.tt/2y3W5sm
via IFTTT
I have a new follower on Twitter
Jason Allen
Washington D.C.
Following: 3580 - Followers: 307
September 12, 2017 at 09:17PM via Twitter http://twitter.com/JLHDU
Ravens: Danny Woodhead (hamstring) out 6-8 weeks - Adam Schefter (ESPN)
via IFTTT
Original text
from Google Alert - anonymous http://ift.tt/2wVJ0TZ
via IFTTT
Ravens-Browns recent history could mean closer game than 7.5-point spread - Jamison Hensley (ESPN)
via IFTTT
Merging anonymous activity with a lead created
from Google Alert - anonymous http://ift.tt/2y3evJH
via IFTTT
8th Street, Ocean City, MD's surf is at least 6.73ft high
8th Street, Ocean City, MD Summary
At 4:00 AM, surf min of 4.1ft. At 10:00 AM, surf min of 4.45ft. At 4:00 PM, surf min of 6.73ft. At 10:00 PM, surf min of 8.07ft.
Surf maximum: 8.04ft (2.45m)
Surf minimum: 6.73ft (2.05m)
Tide height: 2.11ft (0.64m)
Wind direction: NNE
Wind speed: 17.2 KTS
from Surfline http://ift.tt/1kVmigH
via IFTTT
Can I make all attendees anonymous to the presenter and other attendees?
from Google Alert - anonymous http://ift.tt/2w6xeqA
via IFTTT
Clumsy Anonymous, Smallzy in LA, The best thing you've climbed
from Google Alert - anonymous http://ift.tt/2jljrWV
via IFTTT
Ravens: Terrell Suggs showing no signs of age slowing him down - Jamison Hensley (ESPN)
via IFTTT
BlueBorne: Critical Bluetooth Attack Puts Billions of Devices at Risk of Hacking
from The Hacker News http://ift.tt/2eTzFSa
via IFTTT
Ravens climb seven spots to No. 14 in Week 2 NFL Power Rankings (ESPN)
via IFTTT
Anonymous Ass Up Garage Fuck
from Google Alert - anonymous http://ift.tt/2wU14OE
via IFTTT
[FD] SEC Consult SA-20170912-0 :: Email verification bypass in SAP E-Recruiting
Source: Gmail -> IFTTT-> Blogger
Spa manager in Yarm (TS15) | Anonymous Recruiter
from Google Alert - anonymous http://ift.tt/2xuKkhC
via IFTTT
Apache Struts 2 Flaws Affect Multiple Cisco Products
from The Hacker News http://ift.tt/2feZfl9
via IFTTT
Samsung Launches Bug Bounty Program — Offering up to $200,000 in Rewards
from The Hacker News http://ift.tt/2h0lJGB
via IFTTT
[FD] How Apple fixed my 2008's hole in their browser after 9 years
Source: Gmail -> IFTTT-> Blogger
[FD] Access control bypass in Hikvision IP Cameras
Source: Gmail -> IFTTT-> Blogger
Orioles: C Welington Castillo sent to hospital for tests after taking foul ball to groin (ESPN)
via IFTTT
[FD] SSD Advisory – Hanbanggaoke IP Camera Arbitrary Password Change
Source: Gmail -> IFTTT-> Blogger
GPM Examines Hurricane Irma
from NASA's Scientific Visualization Studio: Most Recent Items http://ift.tt/2xh6qTF
via IFTTT
Monday, September 11, 2017
Very dark room, anonymous bj
from Google Alert - anonymous http://ift.tt/2xs3J2D
via IFTTT
📷 Eric Weddle continues tradition of downing lots of ice cream after Ravens win (ESPN)
via IFTTT
Facebook slapped with $1.43 million fine for violating users' privacy in Spain
from The Hacker News http://ift.tt/2gYqi4h
via IFTTT
Object detection with deep learning and OpenCV
A couple weeks ago we learned how to classify images using deep learning and OpenCV 3.3’s deep neural network (
dnn) module.
While this original blog post demonstrated how we can categorize an image into one of ImageNet’s 1,000 separate class labels it could not tell us where an object resides in image.
In order to obtain the bounding box (x, y)-coordinates for an object in a image we need to instead apply object detection.
Object detection can not only tell us what is in an image but also where the object is as well.
In the remainder of today’s blog post we’ll discuss how to apply object detection using deep learning and OpenCV.
Looking for the source code to this post?
Jump right to the downloads section.
Object detection with deep learning and OpenCV
In the first part of today’s post on object detection using deep learning we’ll discuss Single Shot Detectors and MobileNets.
When combined together these methods can be used for super fast, real-time object detection on resource constrained devices (including the Raspberry Pi, smartphones, etc.)
From there we’ll discover how to use OpenCV’s
dnnmodule to load a pre-trained object detection network.
This will enable us to pass input images through the network and obtain the output bounding box (x, y)-coordinates of each object in the image.
Finally we’ll look at the results of applying the MobileNet Single Shot Detector to example input images.
In a future blog post we’ll extend our script to work with real-time video streams as well.
Single Shot Detectors for object detection
When it comes to deep learning-based object detection there are three primary object detection methods that you’ll likely encounter:
- Faster R-CNNs (Girshick et al., 2015)
- You Only Look Once (YOLO) (Redmon and Farhadi, 2015)
- Single Shot Detectors (SSDs) (Liu et al., 2015)
Faster R-CNNs are likely the most “heard of” method for object detection using deep learning; however, the technique can be difficult to understand (especially for beginners in deep learning), hard to implement, and challenging to train.
Furthermore, even with the “faster” implementation R-CNNs (where the “R” stands for “Region Proposal”) the algorithm can be quite slow, on the order of 7 FPS.
If we are looking for pure speed then we tend to use YOLO as this algorithm is much faster, capable of processing 40-90 FPS on a Titan X GPU. The super fast variant of YOLO can even get up to 155 FPS.
The problem with YOLO is that it leaves much accuracy to be desired.
SSDs, originally developed by Google, are a balance between the two. The algorithm is more straightforward (and I would argue better explained in the original seminal paper) than Faster R-CNNs.
We can also enjoy a much faster FPS throughput than Girshick et al. at 22-46 FPS depending on which variant of the network we use. SSDs also tend to be more accurate than YOLO. To learn more about SSDs, please refer to Liu et al.
MobileNets: Efficient (deep) neural networks
Figure 2: (Left) Standard convolutional layer with batch normalization and ReLU. (Right) Depthwise separable convolution with depthwise and pointwise layers followed by batch normalization and ReLU (figure and caption from Liu et al.).
When building object detection networks we normally use an existing network architecture, such as VGG or ResNet, and then use it inside the object detection pipeline. The problem is that these network architectures can be very large in the order of 200-500MB.
Network architectures such as these are unsuitable for resource constrained devices due to their sheer size and resulting number of computations.
Instead, we can use MobileNets (Howard et al., 2017), another paper by Google researchers. We call these networks “MobileNets” because they are designed for resource constrained devices such as your smartphone. MobileNets differ from traditional CNNs through the usage of depthwise separable convolution (Figure 2 above).
The general idea behind depthwise separable convolution is to split convolution into two stages:
- A 3×3 depthwise convolution.
- Followed by a 1×1 pointwise convolution.
This allows us to actually reduce the number of parameters in our network.
The problem is that we sacrifice accuracy — MobileNets are normally not as accurate as their larger big brothers…
…but they are much more resource efficient.
For more details on MobileNets please see Howard et al.
Combining MobileNets and Single Shot Detectors for fast, efficient deep-learning based object detection
If we combine both the MobileNet architecture and the Single Shot Detector (SSD) framework, we arrive at a fast, efficient deep learning-based method to object detection.
The model we’ll be using in this blog post is a Caffe version of the original TensorFlow implementation by Howard et al. and was trained by chuanqi305 (see GitHub).
The MobileNet SSD was first trained on the COCO dataset (Common Objects in Context) and was then fine-tuned on PASCAL VOC reaching 72.7% mAP (mean average precision).
We can therefore detect 20 objects in images (+1 for the background class), including airplanes, bicycles, birds, boats, bottles, buses, cars, cats, chairs, cows, dining tables, dogs, horses, motorbikes, people, potted plants, sheep, sofas, trains, and tv monitors.
Deep learning-based object detection with OpenCV
In this section we will use the MobileNet SSD + deep neural network (
dnn) module in OpenCV to build our object detector.
I would suggest using the “Downloads” code at the bottom of this blog post to download the source code + trained network + example images so you can test them on your machine.
Let’s go ahead and get started building our deep learning object detector using OpenCV.
Open up a new file, name it
deep_learning_object_detection.py, and insert the following code:
# import the necessary packages import numpy as np import argparse import cv2 # construct the argument parse and parse the arguments ap = argparse.ArgumentParser() ap.add_argument("-i", "--image", required=True, help="path to input image") ap.add_argument("-p", "--prototxt", required=True, help="path to Caffe 'deploy' prototxt file") ap.add_argument("-m", "--model", required=True, help="path to Caffe pre-trained model") ap.add_argument("-c", "--confidence", type=float, default=0.2, help="minimum probability to filter weak detections") args = vars(ap.parse_args())
On Lines 2-4 we import packages required for this script — the
dnnmodule is included in
cv2, again, making hte assumption that you’re using OpenCV 3.3.
Then, we parse our command line arguments (Lines 7-16):
-
--image
: The path to the input image. -
--prototxt
: The path to the Caffe prototxt file. -
--model
: The path to the pre-trained model. -
--confidence
: The minimum probability threshold to filter weak detections. The default is 20%.
Again, example files for the first three arguments are included in the “Downloads” section of this blog post. I urge you to start there while also supplying some query images of your own.
Next, let’s initialize class labels and bounding box colors:
# initialize the list of class labels MobileNet SSD was trained to # detect, then generate a set of bounding box colors for each class CLASSES = ["background", "aeroplane", "bicycle", "bird", "boat", "bottle", "bus", "car", "cat", "chair", "cow", "diningtable", "dog", "horse", "motorbike", "person", "pottedplant", "sheep", "sofa", "train", "tvmonitor"] COLORS = np.random.uniform(0, 255, size=(len(CLASSES), 3))
Lines 20-23 build a list called
CLASSEScontaining our labels. This is followed by a list,
COLORSwhich contains corresponding random colors for bounding boxes (Line 24).
Now we need to load our model:
# load our serialized model from disk print("[INFO] loading model...") net = cv2.dnn.readNetFromCaffe(args["prototxt"], args["model"])
The above lines are self-explanatory, we simply print a message and load our
model(Lines 27 and 28).
Next, we will load our query image and prepare our
blob, which we will feed-forward through the network:
# load the input image and construct an input blob for the image # by resizing to a fixed 300x300 pixels and then normalizing it # (note: normalization is done via the authors of the MobileNet SSD # implementation) image = cv2.imread(args["image"]) (h, w) = image.shape[:2] blob = cv2.dnn.blobFromImage(image, 0.007843, (300, 300), 127.5)
Taking note of the comment in this block, we load our
image(Line 34), extract the height and width (Line 35), and calculate a 300 by 300 pixel
blobfrom our image (Line 36).
Now we’re ready to do the heavy lifting — we’ll pass this blog through the neural network:
# pass the blob through the network and obtain the detections and # predictions print("[INFO] computing object detections...") net.setInput(blob) detections = net.forward()
On Lines 41 and 42 we set the input to the network and compute the forward pass for the input, storing the result as
detections. Computing the forward pass and associated detections could take awhile depending on your model and input size, but for this example it will be relatively quick on most CPUs.
Let’s loop through our
detectionsand determine what and where the objects are in the image:
# loop over the detections for i in np.arange(0, detections.shape[2]): # extract the confidence (i.e., probability) associated with the # prediction confidence = detections[0, 0, i, 2] # filter out weak detections by ensuring the `confidence` is # greater than the minimum confidence if confidence > args["confidence"]: # extract the index of the class label from the `detections`, # then compute the (x, y)-coordinates of the bounding box for # the object idx = int(detections[0, 0, i, 1]) box = detections[0, 0, i, 3:7] * np.array([w, h, w, h]) (startX, startY, endX, endY) = box.astype("int") # display the prediction label = "{}: {:.2f}%".format(CLASSES[idx], confidence * 100) print("[INFO] {}".format(label)) cv2.rectangle(image, (startX, startY), (endX, endY), COLORS[idx], 2) y = startY - 15 if startY - 15 > 15 else startY + 15 cv2.putText(image, label, (startX, y), cv2.FONT_HERSHEY_SIMPLEX, 0.5, COLORS[idx], 2)
We start by looping over our detections, keeping in mind that multiple objects can be detected in a single image. We also apply a check to the confidence (i.e., probability) associated with each detection. If the confidence is high enough (i.e. above the threshold), then we’ll display the prediction in the terminal as well as draw the prediction on the image with text and a colored bounding box. Let’s break it down line-by-line:
Looping through our
detections, first we extract the
confidencevalue (Line 48).
If the
confidenceis above our minimum threshold (Line 52), we extract the class label index (Line 56) and compute the bounding box around the detected object (Line 57).
Then, we extract the (x, y)-coordinates of the box (Line 58) which we will will use shortly for drawing a rectangle and displaying text.
Next, we build a text
labelcontaining the
CLASSname and the
confidence(Line 61).
Using the label, we print it to the terminal (Line 62), followed by drawing a colored rectangle around the object using our previously extracted (x, y)-coordinates (Lines 63 and 64).
In general, we want the label to be displayed above the rectangle, but if there isn’t room, we’ll display it just below the top of the rectangle (Line 65).
Finally, we overlay the colored text onto the
imageusing the y-value that we just calculated (Lines 66 and 67).
The only remaining step is to display the result:
# show the output image cv2.imshow("Output", image) cv2.waitKey(0)
We display the resulting output image to the screen until a key is pressed (Lines 70 and 71).
OpenCV and deep learning object detection results
To download the code + pre-trained network + example images, be sure to use the “Downloads” section at the bottom of this blog post.
From there, unzip the archive and execute the following command:
$ python deep_learning_object_detection.py \ --prototxt MobileNetSSD_deploy.prototxt.txt \ --model MobileNetSSD_deploy.caffemodel --image images/example_01.jpg [INFO] loading model... [INFO] computing object detections... [INFO] loading model... [INFO] computing object detections... [INFO] car: 99.78% [INFO] car: 99.25%
Figure 3: Two Toyotas on the highway recognized with near-100% confidence using OpenCV, deep learning, and object detection.
Our first result shows cars recognized and detected with near-100% confidence.
In this example we detect an airplane using deep learning-based object detection:
$ python deep_learning_object_detection.py \ --prototxt MobileNetSSD_deploy.prototxt.txt \ --model MobileNetSSD_deploy.caffemodel --image images/example_02.jpg [INFO] loading model... [INFO] computing object detections... [INFO] loading model... [INFO] computing object detections... [INFO] aeroplane: 98.42%
Figure 4: An airplane successfully detected with high confidence via Python, OpenCV, and deep learning.
The ability for deep learning to detect and localize obscured objects is demonstrated in the following image, where we see a horse (and it’s rider) jumping a fence flanked by two potted plants:
$ python deep_learning_object_detection.py \ --prototxt MobileNetSSD_deploy.prototxt.txt \ --model MobileNetSSD_deploy.caffemodel --image images/example_03.jpg [INFO] loading model... [INFO] computing object detections... [INFO] horse: 96.67% [INFO] person: 92.58% [INFO] pottedplant: 96.87% [INFO] pottedplant: 34.42%
Figure 5: A person riding a horse and two potted plants are successfully identified despite a lot of objects in the image via deep learning-based object detection.
In this example we can see a beer bottle is detected with an impressive 100% confidence:
$ python deep_learning_object_detection.py --prototxt MobileNetSSD_deploy.prototxt.txt \ --model MobileNetSSD_deploy.caffemodel --image images/example_04.jpg [INFO] loading model... [INFO] computing object detections... [INFO] bottle: 100.00%
Followed by another horse image which also contains a dog, car, and person:
$ python deep_learning_object_detection.py \ --prototxt MobileNetSSD_deploy.prototxt.txt \ --model MobileNetSSD_deploy.caffemodel --image images/example_05.jpg [INFO] loading model... [INFO] computing object detections... [INFO] car: 99.87% [INFO] dog: 94.88% [INFO] horse: 99.97% [INFO] person: 99.88%
Finally, a picture of me and Jemma, the family beagle:
$ python deep_learning_object_detection.py \ --prototxt MobileNetSSD_deploy.prototxt.txt \ --model MobileNetSSD_deploy.caffemodel --image images/example_06.jpg [INFO] loading model... [INFO] computing object detections... [INFO] dog: 95.88% [INFO] person: 99.95%
Figure 8: Me and the family beagle are corrected as a “person” and a “dog” via deep learning, object detection, and OpenCV. The TV monitor is not recognized.
Unfortunately the TV monitor isn’t recognized in this image which is likely due to (1) me blocking it and (2) poor contrast around the TV. That being said, we have demonstrated excellent object detection results using OpenCV’s
dnnmodule.
Summary
In today’s blog post we learned how to perform object detection using deep learning and OpenCV.
Specifically, we used both MobileNets + Single Shot Detectors along with OpenCV 3.3’s brand new (totally overhauled)
dnnmodule to detect objects in images.
As a computer vision and deep learning community we owe a lot to the contributions of Aleksandr Rybnikov, the main contributor to the
dnnmodule for making deep learning so accessible from within the OpenCV library. You can find Aleksandr’s original OpenCV example script here — I have modified it for the purposes of this blog post.
In a future blog post I’ll be demonstrating how we can modify today’s tutorial to work with real-time video streams, thus enabling us to perform deep learning-based object detection to videos. We’ll be sure to leverage efficient frame I/O to increase the FPS throughout our pipeline as well.
To be notified when future blog posts (such as the real-time object detection tutorial) are published here on PyImageSearch, simply enter your email address in the form below.
Downloads:
The post Object detection with deep learning and OpenCV appeared first on PyImageSearch.
from PyImageSearch http://ift.tt/2ji8up5
via IFTTT
Raspberry Pi: Learn How to Build Amazing IoT & Robotics Projects at Home
from The Hacker News http://ift.tt/2xqyOUj
via IFTTT
ISS Daily Summary Report – 9/08/2017
from ISS On-Orbit Status Report http://ift.tt/2wkjUd5
via IFTTT
Researcher Discloses 10 Zero-Day Flaws in D-Link 850L Wireless Routers
from The Hacker News http://ift.tt/2w11jHW
via IFTTT