Latest YouTube Video
Saturday, August 26, 2017
Una moza falle yo (Anonymous)
from Google Alert - anonymous http://ift.tt/2xmI7RL
via IFTTT
Anonymous Anonymous
from Google Alert - anonymous http://ift.tt/2wRpQP0
via IFTTT
Ravens: Justin Tucker in concussion protocol after taking hit on kickoff (ESPN)
via IFTTT
▶ Tim Beckham sends 3-run blast over Green Monster in 7-0 win at Red Sox (ESPN)
via IFTTT
Review of Anonymous Beach Hotel, Ayia Napa, Cyprus
from Google Alert - anonymous http://ift.tt/2xATwwK
via IFTTT
Overeaters anonymous chips
from Google Alert - anonymous http://ift.tt/2wdlLlI
via IFTTT
Friday, August 25, 2017
Best anonymous file sharing
from Google Alert - anonymous http://ift.tt/2wOSXT1
via IFTTT
Alcoholics anonymous rochester ny
from Google Alert - anonymous http://ift.tt/2wOmgoI
via IFTTT
Anonymous Single-Round Server-Aided Verification
from Google Alert - anonymous http://ift.tt/2wOffEg
via IFTTT
Ravens: Brandon Williams has become team's Incredible Hulk - Jamison Hensley (ESPN)
via IFTTT
I have a new follower on Twitter
Due
We make it easy to get paid online. Our customers love how we make payments easier, faster and smoother. Support = @DueSupport
Palo Alto, CA
https://t.co/1sOz39spg8
Following: 111623 - Followers: 128858
August 25, 2017 at 01:31PM via Twitter http://twitter.com/due
📷 Here's the full list of nickname jerseys Orioles players are wearing for MLB Players Weekend (ESPN)
via IFTTT
author name for anonymous
from Google Alert - anonymous http://ift.tt/2wEXbx0
via IFTTT
Flix Anonymous - Episode 39 - CKNW Original Podcasts
from Google Alert - anonymous http://ift.tt/2gcoMi4
via IFTTT
Anonymous As Told To Dayna Evans
from Google Alert - anonymous http://ift.tt/2iwDYrk
via IFTTT
ISS Daily Summary Report – 8/24/2017
from ISS On-Orbit Status Report http://ift.tt/2wuelwW
via IFTTT
I have a new follower on Twitter
TCG Network Services
A recognized leader in New England for dynamic #ITConsulting and #ManagedServices for small and mid-sized businesses.
Natick, MA
http://t.co/cTwRWDNVcS
Following: 2866 - Followers: 2770
August 25, 2017 at 07:35AM via Twitter http://twitter.com/TCGNetworks
I have a new follower on Twitter
Chloe Turner
Following: 99 - Followers: 9
August 25, 2017 at 07:35AM via Twitter http://twitter.com/KHssvCXdLbGdhnp
Easy-to-Use Apps Allow Anyone to Create Android Ransomware Within Seconds
from The Hacker News http://ift.tt/2iwk3J6
via IFTTT
[FD] Trend Micro Hosted Email Security (HES) - Email Interception and Direct Object Reference
Source: Gmail -> IFTTT-> Blogger
FBI Arrests Another Hacker Who Visited United States to Attend a Conference
from The Hacker News http://ift.tt/2gaR5gN
via IFTTT
Diamond Ring in a Cloudy Sky
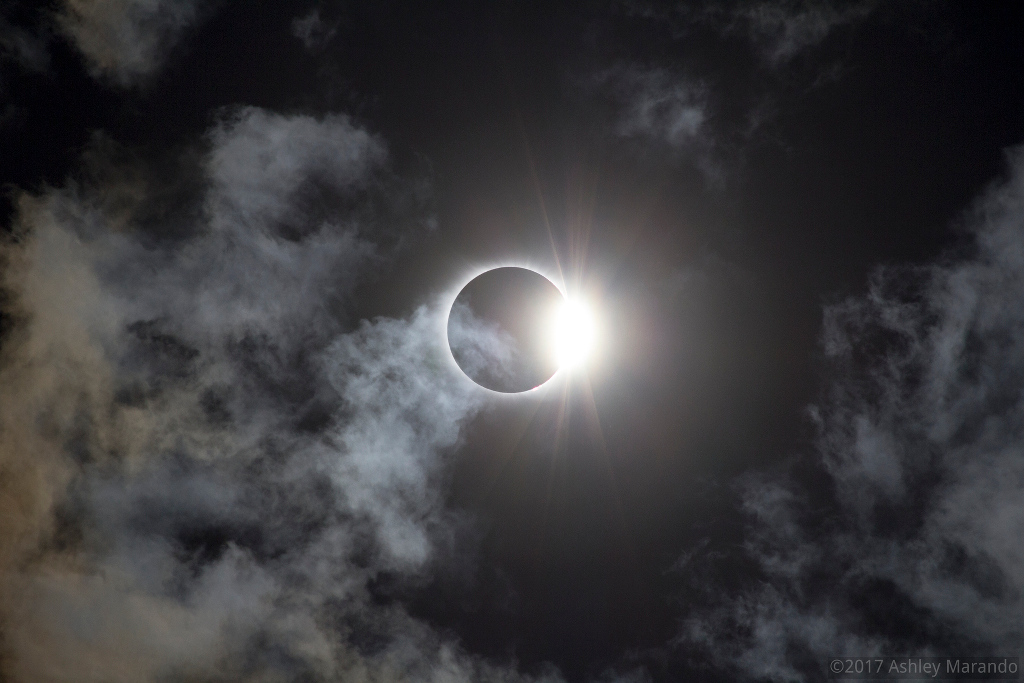
Thursday, August 24, 2017
Anonymous - Executive Chef
from Google Alert - anonymous http://ift.tt/2wKRMUw
via IFTTT
An anonymous psych patient is now acclaimed as a master artist of the immigrant experience
from Google Alert - anonymous http://ift.tt/2gaNQ9a
via IFTTT
Anonymous hacker steals NHS data
from Google Alert - anonymous http://ift.tt/2vudYhq
via IFTTT
Ravens: John Harbaugh defers to Bill Belichick's comments on pressure, "there's stress every week" (ESPN)
via IFTTT
Runtime errors are anonymous unless there is a console
from Google Alert - anonymous http://ift.tt/2w1R3gT
via IFTTT
Anonymous user 5d44d1
from Google Alert - anonymous http://ift.tt/2xfPfPT
via IFTTT
Beware of Windows/MacOS/Linux Virus Spreading Through Facebook Messenger
from The Hacker News http://ift.tt/2w1Kg6W
via IFTTT
ISS Daily Summary Report – 8/23/2017
from ISS On-Orbit Status Report http://ift.tt/2xfgUAF
via IFTTT
I have a new follower on Twitter
The Bodhi Seed
Unique one-of-a-kind art, jewelry and handmade gifts made by artist @LisaKSalerno | Facebook: https://t.co/w066dakutV Instagram:@TheBodhiSeed
Greater Boston
https://t.co/ikXeBEQYr9
Following: 4122 - Followers: 4105
August 24, 2017 at 08:20AM via Twitter http://twitter.com/The_BodhiSeed
Here's How CIA Spies On Its Intelligence Liaison Partners Around the World
from The Hacker News http://ift.tt/2g9B1MF
via IFTTT
I have a new follower on Twitter
Marc R Gagné MAPP 🌐
Senior Privacy and Data Advocate, Cyber Intelligence and Director @ Gagne Legal, Director @PACC_CCAP #Privacy #Fintech #Finserv #DataScience #CyberSecurity
Ottawa, Ontario
https://t.co/anwRZTE7nd
Following: 15494 - Followers: 56192
August 24, 2017 at 05:05AM via Twitter http://twitter.com/OttLegalRebels
8th Street, Ocean City, MD's surf is at least 5.19ft high
8th Street, Ocean City, MD Summary
At 4:00 AM, surf min of 5.19ft. At 10:00 AM, surf min of 5.38ft. At 4:00 PM, surf min of 3.86ft. At 10:00 PM, surf min of 3.05ft.
Surf maximum: 6.18ft (1.88m)
Surf minimum: 5.19ft (1.58m)
Tide height: 2.5ft (0.76m)
Wind direction: ENE
Wind speed: 11.06 KTS
from Surfline http://ift.tt/1kVmigH
via IFTTT
A Company Offers $500,000 For Secure Messaging Apps Zero-Day Exploits
from The Hacker News http://ift.tt/2vqGQqU
via IFTTT
UK: Anonymous hacker says they stole 1.2 million NHS patients' data
from Google Alert - anonymous http://ift.tt/2vZZSYs
via IFTTT
Anonymous Crafter
from Google Alert - anonymous http://ift.tt/2xuGOj2
via IFTTT
Hadrian Belove Resigns From Cinefamily After Anonymous Email Alleges Sexual Harassment
from Google Alert - anonymous http://ift.tt/2ir0y4k
via IFTTT
The Eagle and The Swan
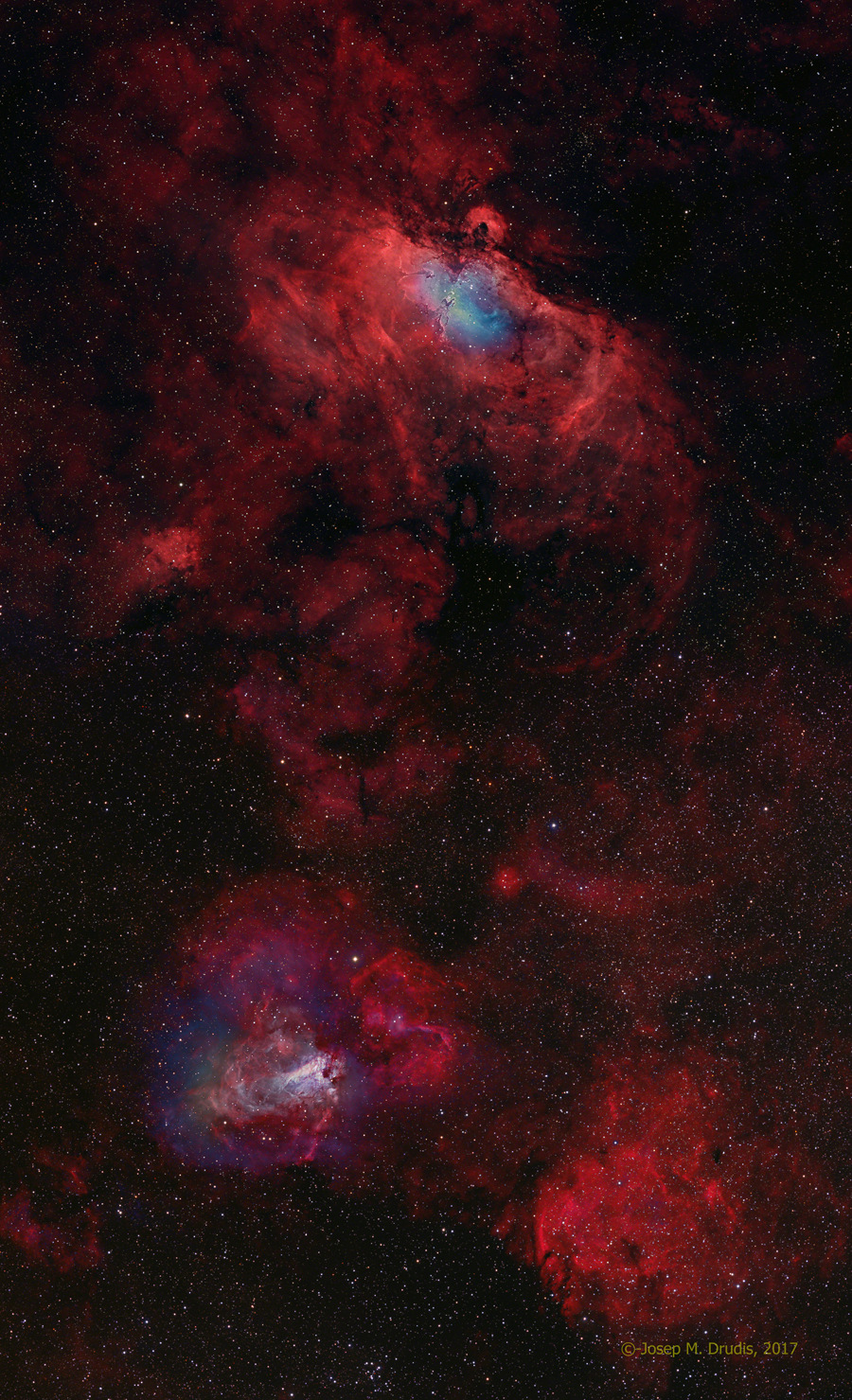
Wednesday, August 23, 2017
8th Street, Ocean City, MD's surf is at least 6.19ft high
8th Street, Ocean City, MD Summary
At 4:00 AM, surf min of 5.43ft. At 10:00 AM, surf min of 5.88ft. At 4:00 PM, surf min of 5.86ft. At 10:00 PM, surf min of 6.19ft.
Surf maximum: 6.53ft (1.99m)
Surf minimum: 6.19ft (1.89m)
Tide height: 1.24ft (0.38m)
Wind direction: ENE
Wind speed: 9.86 KTS
from Surfline http://ift.tt/1kVmigH
via IFTTT
▶ Manny Machado sends fans home happy with walk-off HR for 8-7 win over A's (ESPN)
via IFTTT
Orioles: Zach Britton blows save vs. A's, ending AL-record streak of 60 straight chances converted (ESPN)
via IFTTT
Narcotics Anonymous
from Google Alert - anonymous http://ift.tt/2irH8wx
via IFTTT
Ravens: OC Marty Mornhinweg gives vague answer when asked when Joe Flacco will return (ESPN)
via IFTTT
Anonymous coaches pick college basketball's best players Gallery Slides
from Google Alert - anonymous http://ift.tt/2xt1tE0
via IFTTT
Sarahah is the latest anonymous app under fire
from Google Alert - anonymous http://ift.tt/2wyVIbM
via IFTTT
The Pirate Bay Founders Ordered to Pay Music Labels $477,800 in Compensation
from The Hacker News http://ift.tt/2v6b32U
via IFTTT
Ravens: Patrick Ricard looking to become NFL's sixth two-way player of past decade - Jamison Hensley (ESPN)
via IFTTT
ISS Daily Summary Report – 8/22/2017
from ISS On-Orbit Status Report http://ift.tt/2wyeEHJ
via IFTTT
Simple Exploit Allows Attackers to Modify Email Content — Even After It's Sent!
from The Hacker News http://ift.tt/2wnFemi
via IFTTT
Mecklenburg Confederate statue still stands after Anonymous announces threat
from Google Alert - anonymous http://ift.tt/2vot9bU
via IFTTT
Over 500 Android Apps On Google Play Store Found Spying On 100 Million Users
from The Hacker News http://ift.tt/2wDUoDv
via IFTTT
The Crown of the Sun
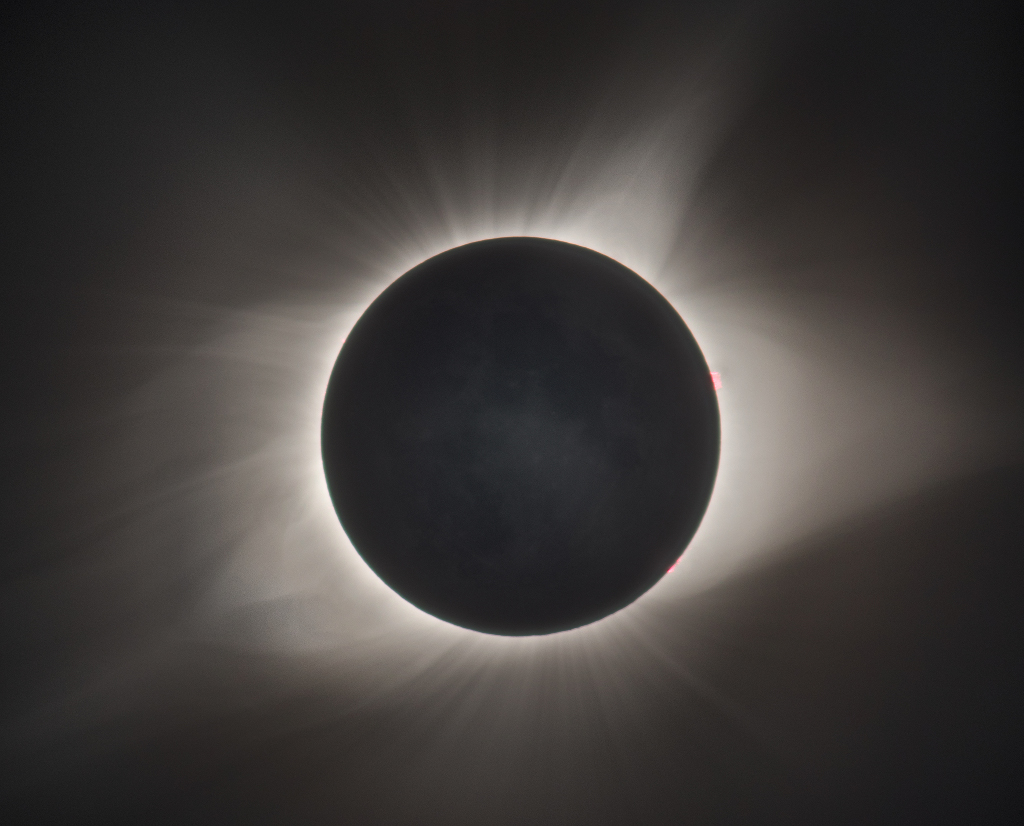
Tuesday, August 22, 2017
Alcohol Anonymous Meetings
from Google Alert - anonymous http://ift.tt/2xbaYZm
via IFTTT
How to disable anonymous user permissions on site build
from Google Alert - anonymous http://ift.tt/2inNAVg
via IFTTT
GitLab.org / GitLab Community Edition
from Google Alert - anonymous http://ift.tt/2g27q7w
via IFTTT
Don't supports anonymous classes from php7
from Google Alert - anonymous http://ift.tt/2iouuOQ
via IFTTT
Frappening 2017: More Celebrity Nude Photos Hacked and Leaked Online
from The Hacker News http://ift.tt/2w0H3D6
via IFTTT
Will Carmen Change Her Name To Anonymous?
from Google Alert - anonymous http://ift.tt/2xpRpvA
via IFTTT
Ravens: Mike Wallace confident offense "wil be fine" once injured players return (ESPN)
via IFTTT
ISS Daily Summary Report – 8/21/2017
from ISS On-Orbit Status Report http://ift.tt/2vldGco
via IFTTT
Android 8.0 Oreo Released – 11 New Features That Make Android Even Better
from The Hacker News http://ift.tt/2ilVuyj
via IFTTT
Standing Out From the Crowd: Anonymous
from Google Alert - anonymous http://ift.tt/2wjpaBZ
via IFTTT
A Total Solar Eclipse over Wyoming
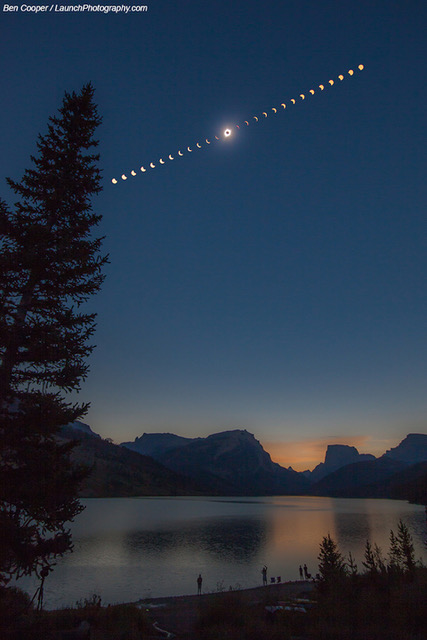
Monday, August 21, 2017
▶ Adam Jones smacks pair of solo homers in 7-3 win over A's (ESPN)
via IFTTT
Anonymous - BOH Positions
from Google Alert - anonymous http://ift.tt/2viNC1I
via IFTTT
Free proxy surf anonymously
from Google Alert - anonymous http://ift.tt/2uZKFIl
via IFTTT
rust-lang/rust
from Google Alert - anonymous http://ift.tt/2vXFV2Y
via IFTTT
The next great sack artist? Where top rookie pass-rushers stand - Dan Graziano (ESPN)
via IFTTT
Sony PlayStation Social Media Accounts Hacked; Claims PSN Database Breach
from The Hacker News http://ift.tt/2virV1R
via IFTTT
I have a new follower on Twitter
Matt L.
Web Developer with React, M.Sc. of Renewable Energies | Improving the world with knowledge and technology 🌿🌎🌞
Olching, Deutschland
https://t.co/ByiGrEgieF
Following: 2000 - Followers: 1874
August 21, 2017 at 01:49PM via Twitter http://twitter.com/ltcmatt
Ravens: Joe Flacco pokes fun at his "eliteness" in Tostitos commercial - Jamison Hensley (ESPN)
via IFTTT
Anonymous Donor Provides School Supplies For Every Student At Texas Elementary School
from Google Alert - anonymous http://ift.tt/2whYmC8
via IFTTT
Ravens: Why Terrell Suggs might've wanted to strangle Justin Tucker - Jamison Hensley (ESPN)
via IFTTT
Deep Learning with OpenCV
Two weeks ago OpenCV 3.3 was officially released, bringing with it a highly improved deep learning (
dnn) module. This module now supports a number of deep learning frameworks, including Caffe, TensorFlow, and Torch/PyTorch.
Furthermore, this API for using pre-trained deep learning models is compatible with both the C++ API and the Python bindings, making it dead simple to:
- Load a model from disk.
- Pre-process an input image.
- Pass the image through the network and obtain the output classifications.
While we cannot train deep learning models using OpenCV (nor should we), this does allow us to take our models trained using dedicated deep learning libraries/tools and then efficiently use them directly inside our OpenCV scripts.
In the remainder of this blog post I’ll demonstrate the fundamentals of how to take a pre-trained deep learning network on the ImageNet dataset and apply it to input images.
To learn more about deep learning with OpenCV, just keep reading.
Looking for the source code to this post?
Jump right to the downloads section.
Deep Learning with OpenCV
In the first part of this post, we’ll discuss the OpenCV 3.3 release and the overhauled
dnnmodule.
We’ll then write a Python script that will use OpenCV and GoogleLeNet (pre-trained on ImageNet) to classify images.
Finally, we’ll explore the results of our classifications.
Deep Learning inside OpenCV 3.3
The dnn module of OpenCV has been part of the
opencv_contribrepository since version v3.1. Now in OpenCV 3.3 it is included in the main repository.
Why should you care?
Deep Learning is a fast growing domain of Machine Learning and if you’re working in the field of computer vision/image processing already (or getting up to speed), it’s a crucial area to explore.
With OpenCV 3.3, we can utilize pre-trained networks with popular deep learning frameworks. The fact that they are pre-trained implies that we don’t need to spend many hours training the network — rather we can complete a forward pass and utilize the output to make a decision within our application.
OpenCV does not (and does not intend to be) to be a tool for training networks — there are already great frameworks available for that purpose. Since a network (such as a CNN) can be used as a classifier, it makes logical sense that OpenCV has a Deep Learning module that we can leverage easily within the OpenCV ecosystem.
Popular network architectures compatible with OpenCV 3.3 include:
- GoogleLeNet (used in this blog post)
- AlexNet
- SqueezeNet
- VGGNet (and associated flavors)
- ResNet
The release notes for this module are available on the OpenCV repository page.
Rynikov Alexander, the main contributor for this module, has ambitious plans for this module so be sure to stay on the lookout and read his release notes (in Russian, so make sure you have Google Translation enabled in your browser if Russian is not your native language).
It’s my opinion that the
dnnmodule will have a big impact on the OpenCV community, so let’s get the word out.
Configure your machine with OpenCV 3.3
Installing OpenCV 3.3 is on par with installing other versions. The same install tutorials can be utilized — just make sure you download and use the correct release.
Simply follow these instructions for MacOS or Ubuntu while making sure to use the opencv and opencv_contrib releases for OpenCV 3.3. If you opt for the MacOS + homebrew install instructions, be sure to use the
--HEADswitch (among the others mentioned) to get the bleeding edge version of OpenCV.
If you’re using virtual environments (highly recommended), you can easily install OpenCV 3.3 alongside a previous version. Just create a brand new virtual environment (and name it appropriately) as you follow the tutorial corresponding to your system.
OpenCV deep learning functions and frameworks
OpenCV 3.3 supports the Caffe, TensorFlow, and Torch/PyTorch frameworks.
Keras is currently not supported (since Keras is actually a wrapper around backends such as TensorFlow and Theano), although I imagine it’s only a matter of time until Keras is directly supported given the popularity of the deep learning library.
Using OpenCV 3.3 we can load images from disk using the following functions inside
dnn:
-
cv2.dnn.blobFromImage
-
cv2.dnn.blobFromImages
We can directly import models from various frameworks via the “create” methods:
-
cv2.dnn.createCaffeImporter
-
cv2.dnn.createTensorFlowImporter
-
cv2.dnn.createTorchImporter
Although I think it’s easier to simply use the “read” methods and load a serialized model from disk directly:
-
cv2.dnn.readNetFromCaffe
-
cv2.dnn.readNetFromTensorFlow
-
cv2.dnn.readNetFromTorch
-
cv2.dnn.readhTorchBlob
Once we have loaded a model from disk, the .forward
method is used to forward-propagate our image and obtain the actual classification.
To learn how all these OpenCV deep learning pieces fit together, let’s move on to the next section.
Classifying images using deep learning and OpenCV
In this section, we’ll be creating a Python script that can be used to classify input images using OpenCV and GoogLeNet (pre-trained on ImageNet) using the Caffe framework.
The GoogLeNet architecture (now known as “Inception” after the novel micro-architecture) was introduced by Szegedy et al. in their 2014 paper, Going deeper with convolutions.
Other architectures are also supported with OpenCV 3.3 including AlexNet, ResNet, and SqueezeNet — we’ll be examining these architectures for deep learning with OpenCV in a future blog post.
In the meantime, let’s learn how we can load a pre-trained Caffe model and use it to classify an image using OpenCV.
To begin, open up a new file, name it
deep_learning_with_opencv.py, and insert the following code:
# import the necessary packages import numpy as np import argparse import time import cv2
On Lines 2-5 we import our necessary packages.
Then we parse command line arguments:
# construct the argument parse and parse the arguments ap = argparse.ArgumentParser() ap.add_argument("-i", "--image", required=True, help="path to input image") ap.add_argument("-p", "--prototxt", required=True, help="path to Caffe 'deploy' prototxt file") ap.add_argument("-m", "--model", required=True, help="path to Caffe pre-trained model") ap.add_argument("-l", "--labels", required=True, help="path to ImageNet labels (i.e., syn-sets)") args = vars(ap.parse_args())
On Line 8 we create an argument parser followed by establishing four required command line arguments (Lines 9-16):
-
--image
: The path to the input image. -
--prototxt
: The path to the Caffe “deploy” prototxt file. -
--model
: The pre-trained Caffe model (i.e,. the network weights themselves). -
--labels
: The path to ImageNet labels (i.e., “syn-sets”).
Now that we’ve established our arguments, we parse them and store them in a variable,
args, for easy access later.
Let’s load the input image and class labels:
# load the input image from disk image = cv2.imread(args["image"]) # load the class labels from disk rows = open(args["labels"]).read().strip().split("\n") classes = [r[r.find(" ") + 1:].split(",")[0] for r in rows]
On Line 20, we load the
imagefrom disk via
cv2.imread.
Let’s take a closer look at the class label data which we load on Lines 23 and 24:
n01440764 tench, Tinca tinca n01443537 goldfish, Carassius auratus n01484850 great white shark, white shark, man-eater, man-eating shark, Carcharodon carcharias n01491361 tiger shark, Galeocerdo cuvieri n01494475 hammerhead, hammerhead shark n01496331 electric ray, crampfish, numbfish, torpedo n01498041 stingray ...
As you can see, we have a unique identifier followed by a space, some class labels, and a new-line. Parsing this file line-by-line is straightforward and efficient using Python.
First, we load the class label
rowsfrom disk into a list. To do this we strip whitespace from the beginning and end of each line while using the new-line (‘
\n‘) as the row delimiter (Line 23). The result is a list of IDs and labels:
['n01440764 tench, Tinca tinca', 'n01443537 goldfish, Carassius auratus', 'n01484850 great white shark, white shark, man-eater, man-eating shark, Carcharodon carcharias', 'n01491361 tiger shark, Galeocerdo cuvieri', 'n01494475 hammerhead, hammerhead shark', 'n01496331 electric ray, crampfish, numbfish, torpedo', 'n01498041 stingray', ...]
Second, we use list comprehension to extract the relevant class labels from
rowsby looking for the space (‘ ‘) after the ID, followed by delimiting class labels with a comma (‘
,‘). The result is simply a list of class labels:
['tench', 'goldfish', 'great white shark', 'tiger shark', 'hammerhead', 'electric ray', 'stingray', ...]
Now that we’ve taken care of the labels, let’s dig into the
dnnmodule of OpenCV 3.3:
# our CNN requires fixed spatial dimensions for our input image(s) # so we need to ensure it is resized to 224x224 pixels while # performing mean subtraction (104, 117, 123) to normalize the input; # after executing this command our "blob" now has the shape: # (1, 3, 224, 224) blob = cv2.dnn.blobFromImage(image, 1, (224, 224), (104, 117, 123))
Taking note of the comment in the block above, we use
cv2.dnn.blobFromImageto perform mean subtraction to normalize the input image which results in a known blob shape (Line 31).
We then load our model from disk:
# load our serialized model from disk print("[INFO] loading model...") net = cv2.dnn.readNetFromCaffe(args["prototxt"], args["model"])
Since we’ve opted to use Caffe, we utilize
cv2.dnn.readNetFromCaffeto load our Caffe model definition
prototxtand pre-trained
modelfrom disk (Line 35).
If you are familiar with Caffe, you’ll recognize the
prototxtfile as a plain text configuration which follows a JSON-like structure — I recommend that you open
bvlc_googlenet.prototxtfrom the “Downloads” section in a text editor to inspect it.
Note: If you are unfamiliar with configuring Caffe CNNs, then this is a great time to consider the PyImageSearch Gurus course — inside the course you’ll get an in depth look at using deep nets for computer vision and image classification.
Now let’s complete a forward pass through the network with
blobas the input:
# set the blob as input to the network and perform a forward-pass to # obtain our output classification net.setInput(blob) start = time.time() preds = net.forward() end = time.time() print("[INFO] classification took {:.5} seconds".format(end - start))
It is important to note at this step that we aren’t training a CNN — rather, we are making use of a pre-trained network. Therefore we are just passing the blob through the network (i.e., forward propagation) to obtain the result (no back-propagation).
First, we specify
blobas our input (Line 39). Second, we make a
starttimestamp (Line 40), followed by passing our input image through the network and storing the predictions. Finally, we set an
endtimestamp (Line 42) so we can calculate the difference and print the elapsed time (Line 43).
Let’s finish up by determining the top five predictions for our input image:
# sort the indexes of the probabilities in descending order (higher # probabilitiy first) and grab the top-5 predictions idxs = np.argsort(preds[0])[::-1][:5]
Using NumPy, we can easily sort and extract the top five predictions on Line 47.
Next, we will display the top five class predictions:
# loop over the top-5 predictions and display them for (i, idx) in enumerate(idxs): # draw the top prediction on the input image if i == 0: text = "Label: {}, {:.2f}%".format(classes[idx], preds[0][idx] * 100) cv2.putText(image, text, (5, 25), cv2.FONT_HERSHEY_SIMPLEX, 0.7, (0, 0, 255), 2) # display the predicted label + associated probability to the # console print("[INFO] {}. label: {}, probability: {:.5}".format(i + 1, classes[idx], preds[0][idx])) # display the output image cv2.imshow("Image", image) cv2.waitKey(0)
The idea for this loop is to (1) draw the top prediction label on the image itself and (2) print the associated class label probabilities to the terminal.
Lastly, we display the image to the screen (Line 64) and wait for the user to press a key before exiting (Line 65).
Deep learning and OpenCV classification results
Now that we have implemented our Python script to utilize deep learning with OpenCV, let’s go ahead and apply it to a few example images.
Make sure you use the “Downloads” section of this blog post to download the source code + pre-trained GoogLeNet architecture + example images.
From there, open up a terminal and execute the following command:
$ python deep_learning_with_opencv.py --image images/jemma.png --prototxt bvlc_googlenet.prototxt \ --model bvlc_googlenet.caffemodel --labels synset_words.txt [INFO] loading model... [INFO] classification took 0.075035 seconds [INFO] 1. label: beagle, probability: 0.81137 [INFO] 2. label: Labrador retriever, probability: 0.031416 [INFO] 3. label: bluetick, probability: 0.023929 [INFO] 4. label: EntleBucher, probability: 0.017507 [INFO] 5. label: Greater Swiss Mountain dog, probability: 0.01444
In the above example, we have Jemma, the family beagle. Using OpenCV and GoogLeNet we have correctly classified this image as “beagle”.
Furthermore, inspecting the top-5 results we can see that the other top predictions are also relevant, all of them of which are dogs that have similar physical appearances as beagles.
Taking a look at the timing we also see that the forward pass took < 1 second, even though we are using our CPU.
Keep in mind that the forward pass is substantially faster than the backward pass as we do not need to compute the gradient and backpropagate through the network.
Let’s classify another image using OpenCV and deep learning:
$ python deep_learning_with_opencv.py --image images/traffic_light.png --prototxt bvlc_googlenet.prototxt \ --model bvlc_googlenet.caffemodel --labels synset_words.txt [INFO] loading model... [INFO] classification took 0.080521 seconds [INFO] 1. label: traffic light, probability: 1.0 [INFO] 2. label: pole, probability: 4.9961e-07 [INFO] 3. label: spotlight, probability: 3.4974e-08 [INFO] 4. label: street sign, probability: 3.3623e-08 [INFO] 5. label: loudspeaker, probability: 2.0235e-08
OpenCV and GoogLeNet correctly label this image as “traffic light” with 100% certainty.
In this example we have a “bald eagle”:
$ python deep_learning_with_opencv.py --image images/eagle.png --prototxt bvlc_googlenet.prototxt \ --model bvlc_googlenet.caffemodel --labels synset_words.txt [INFO] loading model... [INFO] classification took 0.087207 seconds [INFO] 1. label: bald eagle, probability: 0.96768 [INFO] 2. label: kite, probability: 0.031964 [INFO] 3. label: vulture, probability: 0.00023595 [INFO] 4. label: albatross, probability: 6.3653e-05 [INFO] 5. label: black grouse, probability: 1.6147e-05
Figure 3: The “deep neural network” (dnn) module inside OpenCV 3.3 can be used to classify images using pre-trained models.
We are once again able to correctly classify the input image.
Our final example is a “vending machine”:
$ python deep_learning_with_opencv.py --image images/vending_machine.png --prototxt bvlc_googlenet.prototxt \ --model bvlc_googlenet.caffemodel --labels synset_words.txt [INFO] loading model... [INFO] classification took 0.099602 seconds [INFO] 1. label: vending machine, probability: 0.99269 [INFO] 2. label: cash machine, probability: 0.0023691 [INFO] 3. label: pay-phone, probability: 0.00097005 [INFO] 4. label: ashcan, probability: 0.00092097 [INFO] 5. label: mailbox, probability: 0.00061188
Figure 4: Since our GoogLeNet model is pre-trained on ImageNet, we can classify each of the 1,000 labels inside the dataset using OpenCV + deep learning.
OpenCV + deep learning once again correctly classifes the image.
Summary
In today’s blog post we learned how to use OpenCV for deep learning.
With the release of OpenCV 3.3 the deep neural network (
dnn) library has been substantially overhauled, allowing us to load pre-trained networks via the Caffe, TensorFlow, and Torch/PyTorch frameworks and then use them to classify input images.
I imagine Keras support will also be coming soon, given how popular the framework is. This will likely take be a non-trivial implementation as Keras itself can support multiple numeric computation backends.
Over the next few weeks we’ll:
- Take a deeper dive into the
dnn
module and how it can be used inside our Python + OpenCV scripts. - Learn how to modify Caffe
.prototxt
files to be compatible with OpenCV. - Discover how we can apply deep learning using OpenCV to the Raspberry Pi.
This is a can’t-miss series of blog posts, so be before you go, make sure you enter your email address in the form below to be notified when these posts go live!
Downloads:
The post Deep Learning with OpenCV appeared first on PyImageSearch.
from PyImageSearch http://ift.tt/2wgXJbP
via IFTTT
Ravens cancel Monday's practice to do team-building activity, also have planned day off Tuesday (ESPN)
via IFTTT
ISS Daily Summary Report – 8/18/2017
from ISS On-Orbit Status Report http://ift.tt/2wwnaGa
via IFTTT
Sarahah: Know About Anonymous Messaging App
from Google Alert - anonymous http://ift.tt/2wgAKOf
via IFTTT
Warning: Enigma Hacked; Over $470,000 in Ethereum Stolen So Far
from The Hacker News http://ift.tt/2ijSFxT
via IFTTT
Doctor Implanted 6 MicroChips Under His Skin to Unlock Doors and Secure Data
from The Hacker News http://ift.tt/2vRwx2y
via IFTTT
Sunday, August 20, 2017
Anonymous Donor Gives $2000 to Oklahoma School in Honor of Beloved Teacher
from Google Alert - anonymous http://ift.tt/2vfyRge
via IFTTT
Menu icon does not render correctly for anonymous users
from Google Alert - anonymous http://ift.tt/2fXebrd
via IFTTT
New Snowden Leak Exposes Secret NSA Spying Hub in Australian
from The Hacker News http://ift.tt/2xge39w
via IFTTT
Anonymous request can't be found with search filter 'open requests to others'
from Google Alert - anonymous http://ift.tt/2v3rSrn
via IFTTT
Smart Devices Can Be Hijacked to Track Your Body Movements And Activities Remotely
from The Hacker News http://ift.tt/2wlt4dQ
via IFTTT