Latest YouTube Video
Saturday, February 11, 2017
Disable Form Caching for Anonymous User
from Google Alert - anonymous http://ift.tt/2kj1ljy
via IFTTT
I have a new follower on Twitter
IDIDIT Foundation
Connecting Resources with Recipients! #IDIDIT #1 FREE CrowdFunding Site for ANYTHING FREE https://t.co/4oq4XwNDio
Vancouver
https://t.co/4oq4XwNDio
Following: 36333 - Followers: 56116
February 11, 2017 at 09:01PM via Twitter http://twitter.com/ididitucan
[Suggest] docker volume driver API: Support default-opt for anonymous volume
from Google Alert - anonymous http://ift.tt/2kV1D1Z
via IFTTT
I have a new follower on Twitter
GREGOR SIDERIS
European Marketing Leader @IBM #IOT #Industry40 Top Tech Influencer 🌟Luxury Travel Blogger https://t.co/Bi6A2MY4nS views are my own
Vienna Europe
https://t.co/dE1DUQcFtj
Following: 73862 - Followers: 81430
February 11, 2017 at 05:56AM via Twitter http://twitter.com/GregorSIDERIS
Melotte 15 inthe Heart
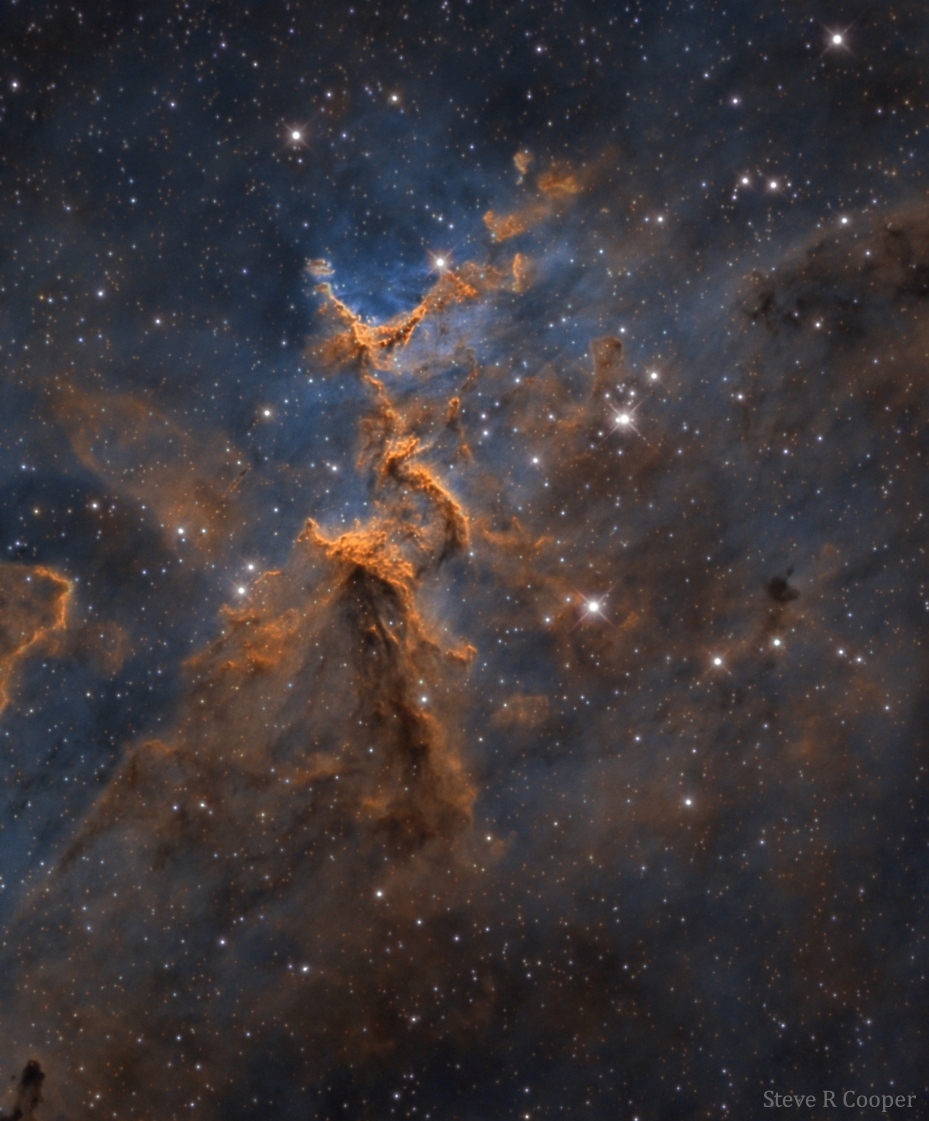
Friday, February 10, 2017
Buddy Montana for Ballers Anonymous
from Google Alert - anonymous http://ift.tt/2kChLqx
via IFTTT
U cautions students after anonymous threat to fraternities
from Google Alert - anonymous http://ift.tt/2kS8VU5
via IFTTT
Anonymous Function vs Class Method vs Eval
from Google Alert - anonymous http://ift.tt/2kcWg1d
via IFTTT
Anonymous Data Service
from Google Alert - anonymous http://ift.tt/2kBSRrm
via IFTTT
One (Anonymous Version)
from Google Alert - anonymous http://ift.tt/2kdYicy
via IFTTT
UM Police unable to contact sender of anonymous email
from Google Alert - anonymous http://ift.tt/2kclVaa
via IFTTT
Orioles acquire P Gabriel Ynoa from Mets for cash considerations; 1-0, 6.38 ERA in 10 games in 2016 (ESPN)
via IFTTT
Hook callback anonymous functions
from Google Alert - anonymous http://ift.tt/2kX7fv7
via IFTTT
Anonymous Interpreter
from Google Alert - anonymous http://ift.tt/2kbnsgJ
via IFTTT
ISS Daily Summary Report – 2/08/2017
from ISS On-Orbit Status Report http://ift.tt/2kX3vd7
via IFTTT
Should Sweden allow anonymous witness testimony?
from Google Alert - anonymous http://ift.tt/2lyHgYy
via IFTTT
[FD] [Call for Papers] InfoSec2017 in Bratislava, Slovakia | June 29-July 1, 2017
Source: Gmail -> IFTTT-> Blogger
Re: Anonymous access
from Google Alert - anonymous http://ift.tt/2kbq21m
via IFTTT
2017 Eclipse State Maps
from NASA's Scientific Visualization Studio: Most Popular
via IFTTT
Crescent Enceladus
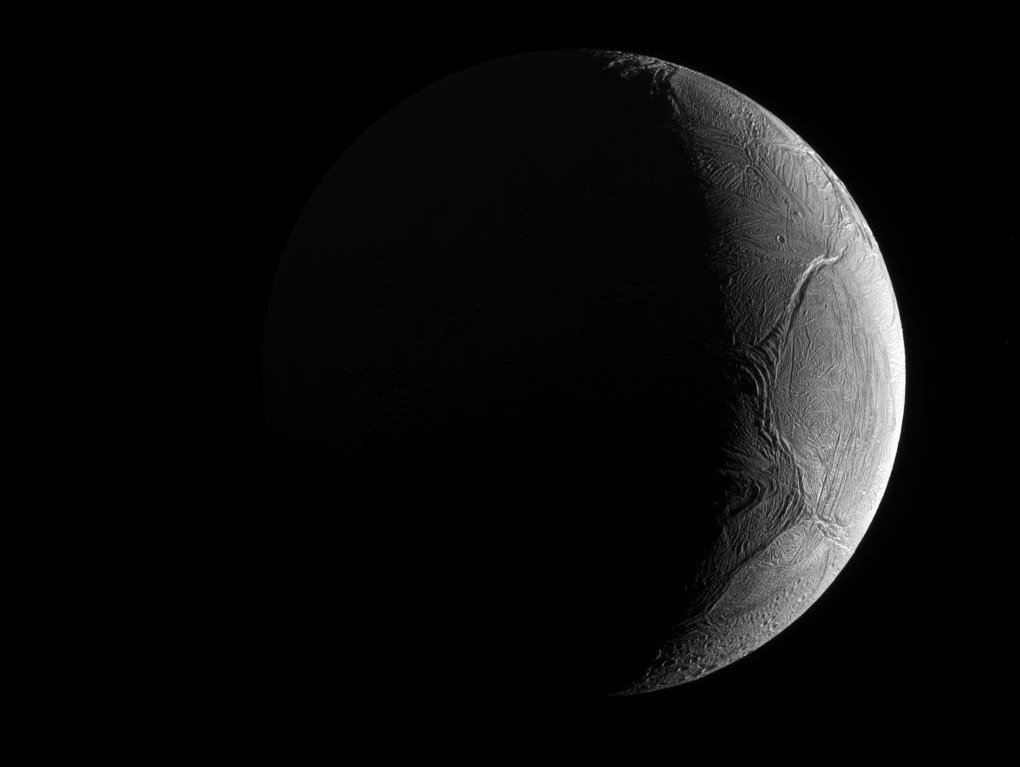
MMS Phase 2b: Transitioning to Magnetosphere Science on the Darkside
from NASA's Scientific Visualization Studio: Most Recent Items http://ift.tt/2kOlJLk
via IFTTT
Thursday, February 9, 2017
Watch Out! First-Ever Word Macro Malware for Apple Mac OS Discovered in the Wild
from The Hacker News http://ift.tt/2kUuSok
via IFTTT
New Windows Trojan Spreads MIRAI Malware To Hack More IoT Devices
from The Hacker News http://ift.tt/2luqs3P
via IFTTT
Neural Causal Regularization under the Independence of Mechanisms Assumption. (arXiv:1702.02604v1 [cs.LG])
Neural networks provide a powerful framework for learning the association between input and response variables and making accurate predictions and offer promise in using the rapidly growing volume of health care data to surface causal relationships that cannot necessarily be tested in randomized clinical trials. In pursuit of models whose predictive power comes maximally from causal variables, we propose a novel causal regularizer based on the assumption about independence of different steps of data generation process. We use the causal regularizer to steer deep neural network architectures towards causally-interpretable solutions. We perform a large-scale analysis of electronic health records. Our causally-regularized algorithm outperforms its L1-regularized counterpart both in predictive performance as well as causal relevance. Finally, we show that the proposed causal regularizer can be used together with representation learning algorithms to yield up to 20% improvement in the causality score of the generated multivariate hypotheses.
from cs.AI updates on arXiv.org http://ift.tt/2kUuaHv
via IFTTT
Optimal Detection of Faulty Traffic Sensors Used in Route Planning. (arXiv:1702.02628v1 [cs.AI])
In a smart city, real-time traffic sensors may be deployed for various applications, such as route planning. Unfortunately, sensors are prone to failures, which result in erroneous traffic data. Erroneous data can adversely affect applications such as route planning, and can cause increased travel time and environmental impact. To minimize the impact of sensor failures, we must detect them promptly and with high accuracy. However, typical detection algorithms may lead to a large number of false positives (i.e., false alarms) and false negatives (i.e., missed detections), which can result in suboptimal route planning. In this paper, we devise an effective detector for identifying faulty traffic sensors using a prediction model based on Gaussian Processes. Further, we present an approach for computing the optimal parameters of the detector which minimize losses due to false-positive and false-negative errors. We also characterize critical sensors, whose failure can have high impact on the route planning application. Finally, we implement our method and evaluate it numerically using a real-world dataset and the route planning platform OpenTripPlanner.
from cs.AI updates on arXiv.org http://ift.tt/2kUgRHa
via IFTTT
Energy Saving Additive Neural Network. (arXiv:1702.02676v1 [cs.NE])
In recent years, machine learning techniques based on neural networks for mobile computing become increasingly popular. Classical multi-layer neural networks require matrix multiplications at each stage. Multiplication operation is not an energy efficient operation and consequently it drains the battery of the mobile device. In this paper, we propose a new energy efficient neural network with the universal approximation property over space of Lebesgue integrable functions. This network, called, additive neural network, is very suitable for mobile computing. The neural structure is based on a novel vector product definition, called ef-operator, that permits a multiplier-free implementation. In ef-operation, the "product" of two real numbers is defined as the sum of their absolute values, with the sign determined by the sign of the product of the numbers. This "product" is used to construct a vector product in $R^N$. The vector product induces the $l_1$ norm. The proposed additive neural network successfully solves the XOR problem. The experiments on MNIST dataset show that the classification performances of the proposed additive neural networks are very similar to the corresponding multi-layer perceptron and convolutional neural networks (LeNet).
from cs.AI updates on arXiv.org http://ift.tt/2k9zySC
via IFTTT
Graph Based Relational Features for Collective Classification. (arXiv:1702.02817v1 [cs.IR])
Statistical Relational Learning (SRL) methods have shown that classification accuracy can be improved by integrating relations between samples. Techniques such as iterative classification or relaxation labeling achieve this by propagating information between related samples during the inference process. When only a few samples are labeled and connections between samples are sparse, collective inference methods have shown large improvements over standard feature-based ML methods. However, in contrast to feature based ML, collective inference methods require complex inference procedures and often depend on the strong assumption of label consistency among related samples. In this paper, we introduce new relational features for standard ML methods by extracting information from direct and indirect relations. We show empirically on three standard benchmark datasets that our relational features yield results comparable to collective inference methods. Finally we show that our proposal outperforms these methods when additional information is available.
from cs.AI updates on arXiv.org http://ift.tt/2kUl1yC
via IFTTT
Phase Transitions of the Typical Algorithmic Complexity of the Random Satisfiability Problem Studied with Linear Programming. (arXiv:1702.02821v1 [cond-mat.dis-nn])
The Boolean Satisfiability problem asks if a Boolean formula is satisfiable by some assignment of the variables or not. It belongs to the NP-complete complexity class and hence no algorithm with polynomial time worst-case complexity is known, i.e., the problem is hard. The K-SAT problem is the subset of the Boolean Satisfiability problem, for which the Boolean formula has the conjunctive normal form with K literals per clause. This problem is still NP-complete for $K \ge 3$. Although the worst case complexity of NP-complete problems is conjectured to be exponential, there might be subsets of the realizations where solutions can typically be found in polynomial time. In fact, random $K$-SAT, with the number of clauses to number of variables ratio $\alpha$ as control parameter, shows a phase transition between a satisfiable phase and an unsatisfiable phase, at which the hardest problems are located. We use here several linear programming approaches to reveal further "easy-hard" transition points at which the typical hardness of the problems increases which means that such algorithms can solve the problem on one side efficiently but not beyond this point. For one of these transitions, we observed a coincidence with a structural transition of the literal factor graphs of the problem instances. We also investigated cutting-plane approaches, which often increase the computational efficiency. Also we tried out a mapping to another NP-complete optimization problem using a specific algorithm for that problem. In both cases, no improvement of the performance was observed, i.e., no shift of the easy-hard transition to higher values of $\alpha$.
from cs.AI updates on arXiv.org http://ift.tt/2kUrZnl
via IFTTT
Answer Set Solving with Bounded Treewidth Revisited. (arXiv:1702.02890v1 [cs.LO])
Parameterized algorithms are a way to solve hard problems more efficiently, given that a specific parameter of the input is small. In this paper, we apply this idea to the field of answer set programming (ASP). To this end, we propose two kinds of graph representations of programs to exploit their treewidth as a parameter. Treewidth roughly measures to which extent the internal structure of a program resembles a tree. Our main contribution is the design of parameterized dynamic programming algorithms, which run in linear time if the treewidth and weights of the given program are bounded. Compared to previous work, our algorithms handle the full syntax of ASP. Finally, we report on an empirical evaluation that shows good runtime behaviour for benchmark instances of low treewidth, especially for counting answer sets.
from cs.AI updates on arXiv.org http://ift.tt/2lucO0T
via IFTTT
I have a new follower on Twitter
Anonymous
DIARY OF AN OXYGEN THIEF CHAMELEON IN A CANDY STORE EUNUCHS AND NYMPHOMANIACS
New York
http://t.co/OmCxgbsrOV
Following: 4888 - Followers: 3948
February 09, 2017 at 06:34PM via Twitter http://twitter.com/02thief
[FD] TP-Link C2 and C20i vulnerable to command injection (authenticated root RCE), DoS, improper firewall rules
Source: Gmail -> IFTTT-> Blogger
Popup when selecting anonymous survey in the Survey tool
from Google Alert - anonymous http://ift.tt/2kxrIFO
via IFTTT
Anonymous lead web activity is not associated with known lead after form submission
from Google Alert - anonymous http://ift.tt/2k8Jtbb
via IFTTT
A Truly Anonymous Quiz?
from Google Alert - anonymous http://ift.tt/2kTEW0H
via IFTTT
Best anonymous image board
from Google Alert - anonymous http://ift.tt/2lsU2Hd
via IFTTT
ISS Daily Summary Report – 2/08/2017
from ISS On-Orbit Status Report http://ift.tt/2kvP7rp
via IFTTT
Wednesday, February 8, 2017
I have a new follower on Twitter
Ed Airey
Director, Solutions Marketing @MicroFocus #VisualCOBOL: #COBOL for next gen #developers #COBOLRocks #DevDay #Java #Mainframe #Skills #DevOps views are my own
Boston
Following: 13857 - Followers: 15735
February 08, 2017 at 10:28PM via Twitter http://twitter.com/EdAirey
MLB: Giancarlo Stanton, Adam Jones among 28-man U.S. roster for World Baseball Classic; includes 18 All-Stars and 2 MVPs (ESPN)
via IFTTT
I have a new follower on Twitter
Synergis Engineering
Providing the best quality Autodesk solutions, professional services, and training to ensure our customers' maximum productivity, competitive advantage, and ROI
Quakertown, PA
http://t.co/hqrhMhJcsH
Following: 3390 - Followers: 3838
February 08, 2017 at 09:48PM via Twitter http://twitter.com/SynergisCAD
I have a new follower on Twitter
Tama Fulton
On-air at 100.7 @Seattlewolf | #IMDb @TrueNorthStory® #podcast co-host | @iTunes @GooglePlay | #socialmedia manager @RationalAgency @Microsoft
Seattle, WA
https://t.co/zvuAzO3L3G
Following: 16203 - Followers: 18351
February 08, 2017 at 09:43PM via Twitter http://twitter.com/tamafulton
Generating Multiple Hypotheses for Human 3D Pose Consistent with 2D Joint Detections. (arXiv:1702.02258v1 [cs.CV])
We propose a method to generate multiple hypotheses for human 3D pose all of them consistent with the 2D detection of joints in a monocular RGB image. To generate these pose hypotheses we use a novel generative model defined in the space of anatomically plausible 3D poses satisfying the joint angle limits and limb length ratios. The proposed generative model is uniform in the space of anatomically valid poses and as a result, does not suffer from the dataset bias in existing motion capture datasets such as Human3.6M (H36M), HumanEva, and CMU MoCap. A good model that spans the full variability of human pose and generalizes to unseen poses must be compositional i.e., produce a pose by combining parts. Our model is flexible and compositional and consequently can generalize to every plausible human 3D pose since it is only limited by physical constraints. We discuss sampling from this model and use these samples to generate multiple diverse human 3D pose hypotheses given the 2D detection of joints. We argue that generating multiple pose hypotheses from a monocular RGB image is more reasonable than generating only a single 3D pose given the depth ambiguity and the uncertainty caused by occlusion and imperfect 2D joint detection. To support this argument, we have performed empirical evaluation on the popular Human3.6M dataset that confirms that most often, at least one of our pose hypotheses is closer to the true 3D pose compared to the estimated pose by other recent baseline methods for 3D pose reconstruction from monocular RGB images. The idea of generating multiple consistent and valid pose hypotheses can give rise to a new line of future work that has not previously been addressed in the literature.
from cs.AI updates on arXiv.org http://ift.tt/2k4GVzs
via IFTTT
A Historical Review of Forty Years of Research on CMAC. (arXiv:1702.02277v1 [cs.NE])
The Cerebellar Model Articulation Controller (CMAC) is an influential brain-inspired computing model in many relevant fields. Since its inception in the 1970s, the model has been intensively studied and many variants of the prototype, such as Kernel-CMAC, Self-Organizing Map CMAC, and Linguistic CMAC, have been proposed. This review article focus on how the CMAC model is gradually developed and refined to meet the demand of fast, adaptive, and robust control. Two perspective, CMAC as a neural network and CMAC as a table look-up technique are presented. Three aspects of the model: the architecture, learning algorithms and applications are discussed. In the end, some potential future research directions on this model are suggested.
from cs.AI updates on arXiv.org http://ift.tt/2kUP0pD
via IFTTT
Autonomous Braking System via Deep Reinforcement Learning. (arXiv:1702.02302v1 [cs.AI])
In this paper, we propose a new autonomous braking system based on deep reinforcement learning. The proposed autonomous braking system automatically decides whether to apply the brake at each time step when confronting the risk of collision using the information on the obstacle obtained by the sensors. The problem of designing brake control is formulated as searching for the optimal policy in Markov decision process (MDP) model where the state is given by the relative position of the obstacle and the vehicle's speed, and the action space is defined as whether brake is stepped or not. The policy used for brake control is learned through computer simulations using the deep reinforcement learning method called deep Q-network (DQN). In order to derive desirable braking policy, we propose the reward function which balances the damage imposed to the obstacle in case of accident and the reward achieved when the vehicle runs out of risk as soon as possible. DQN is trained for the scenario where a vehicle is encountered with a pedestrian crossing the urban road. Experiments show that the control agent exhibits desirable control behavior and avoids collision without any mistake in various uncertain environments.
from cs.AI updates on arXiv.org http://ift.tt/2lqbOe5
via IFTTT
Propagation via Kernelization: The Vertex Cover Constraint. (arXiv:1702.02470v1 [cs.AI])
The technique of kernelization consists in extracting, from an instance of a problem, an essentially equivalent instance whose size is bounded in a parameter k. Besides being the basis for efficient param-eterized algorithms, this method also provides a wealth of information to reason about in the context of constraint programming. We study the use of kernelization for designing propagators through the example of the Vertex Cover constraint. Since the classic kernelization rules often correspond to dominance rather than consistency, we introduce the notion of "loss-less" kernel. While our preliminary experimental results show the potential of the approach, they also show some of its limits. In particular, this method is more effective for vertex covers of large and sparse graphs, as they tend to have, relatively, smaller kernels.
from cs.AI updates on arXiv.org http://ift.tt/2kULn3b
via IFTTT
Deep Generalized Canonical Correlation Analysis. (arXiv:1702.02519v1 [cs.LG])
We present Deep Generalized Canonical Correlation Analysis (DGCCA) -- a method for learning nonlinear transformations of arbitrarily many views of data, such that the resulting transformations are maximally informative of each other. While methods for nonlinear two-view representation learning (Deep CCA, (Andrew et al., 2013)) and linear many-view representation learning (Generalized CCA (Horst, 1961)) exist, DGCCA is the first CCA-style multiview representation learning technique that combines the flexibility of nonlinear (deep) representation learning with the statistical power of incorporating information from many independent sources, or views. We present the DGCCA formulation as well as an efficient stochastic optimization algorithm for solving it. We learn DGCCA repre- sentations on two distinct datasets for three downstream tasks: phonetic transcrip- tion from acoustic and articulatory measurements, and recommending hashtags and friends on a dataset of Twitter users. We find that DGCCA representations soundly beat existing methods at phonetic transcription and hashtag recommendation, and in general perform no worse than standard linear many-view techniques.
from cs.AI updates on arXiv.org http://ift.tt/2kol42b
via IFTTT
Automatic Rule Extraction from Long Short Term Memory Networks. (arXiv:1702.02540v1 [cs.CL])
Although deep learning models have proven effective at solving problems in natural language processing, the mechanism by which they come to their conclusions is often unclear. As a result, these models are generally treated as black boxes, yielding no insight of the underlying learned patterns. In this paper we consider Long Short Term Memory networks (LSTMs) and demonstrate a new approach for tracking the importance of a given input to the LSTM for a given output. By identifying consistently important patterns of words, we are able to distill state of the art LSTMs on sentiment analysis and question answering into a set of representative phrases. This representation is then quantitatively validated by using the extracted phrases to construct a simple, rule-based classifier which approximates the output of the LSTM.
from cs.AI updates on arXiv.org http://ift.tt/2k4IbCF
via IFTTT
What is Wrong with Topic Modeling? (and How to Fix it Using Search-based Software Engineering). (arXiv:1608.08176v2 [cs.SE] UPDATED)
Context: Topic modeling finds human-readable structures in unstructured textual data. A widely used topic modeler is Latent Dirichlet allocation. When run on different datasets, LDA suffers from "order effects" i.e. different topics are generated if the order of training data is shuffled. Such order effects introduce a systematic error for any study. This error can relate to misleading results;specifically, inaccurate topic descriptions and a reduction in the efficacy of text mining classification results. Objective: To provide a method in which distributions generated by LDA are more stable and can be used for further analysis. Method: We use LDADE, a search-based software engineering tool that tunes LDA's parameters using DE (Differential Evolution). LDADE is evaluated on data from a programmer information exchange site (Stackoverflow), title and abstract text of thousands ofSoftware Engineering (SE) papers, and software defect reports from NASA. Results were collected across different implementations of LDA (Python+Scikit-Learn, Scala+Spark); across different platforms (Linux, Macintosh) and for different kinds of LDAs (VEM,or using Gibbs sampling). Results were scored via topic stability and text mining classification accuracy. Results: In all treatments: (i) standard LDA exhibits very large topic instability; (ii) LDADE's tunings dramatically reduce cluster instability; (iii) LDADE also leads to improved performances for supervised as well as unsupervised learning. Conclusion: Due to topic instability, using standard LDA with its "off-the-shelf" settings should now be depreciated. Also, in future, we should require SE papers that use LDA to test and (if needed) mitigate LDA topic instability. Finally, LDADE is a candidate technology for effectively and efficiently reducing that instability.
from cs.AI updates on arXiv.org http://ift.tt/2bvVgSF
via IFTTT
Decoding as Continuous Optimization in Neural Machine Translation. (arXiv:1701.02854v2 [cs.CL] UPDATED)
We propose a novel decoding approach for neural machine translation (NMT) based on continuous optimisation. The resulting optimisation problem is then tackled using constrained gradient optimisation. Our powerful decoding framework, enables decoding intractable models such as the intersection of left-to-right and right-to-left (bidirectional) as well as source-to-target and target-to-source (bilingual) NMT models. Our empirical results show that our decoding framework is effective, and leads to substantial improvements in translations generated from the intersected models where the typical greedy or beam search is infeasible.
from cs.AI updates on arXiv.org http://ift.tt/2j8JFKP
via IFTTT
THN Deal: Online CISSP Certification Training Course (94% Off)
from The Hacker News http://ift.tt/2lm54lo
via IFTTT
Anonymous web proxy java enable
from Google Alert - anonymous http://ift.tt/2kIVWnF
via IFTTT
Anonymous Egg Donors Needed ASAP!
from Google Alert - anonymous http://ift.tt/2kn9FQg
via IFTTT
Anonymous no more: Google's new AI system unscrambles pixelated faces
from Google Alert - anonymous http://ift.tt/2k3WebH
via IFTTT
Dandelion - Turning Bitcoin Into A Fully Anonymous Financial System
from Google Alert - anonymous http://ift.tt/2k4aUCW
via IFTTT
McShay Mock Draft 2.0: Ravens get Michigan State DT Malik McDowell at No. 16; "questions about his motor" - Hensley (ESPN)
via IFTTT
[FD] Authentication bypass vulnerability in Western Digital My Cloud
Source: Gmail -> IFTTT-> Blogger
New “Fileless Malware” Targets Banks and Organizations Spotted in the Wild
from The Hacker News http://ift.tt/2k3Meub
via IFTTT
Tucson Metro Chamber releases results from anonymous survey
from Google Alert - anonymous http://ift.tt/2kSN0hK
via IFTTT
ISS Daily Summary Report – 2/07/2017
from ISS On-Orbit Status Report http://ift.tt/2kSBPWa
via IFTTT
Mr. Anonymous getting better with age
from Google Alert - anonymous http://ift.tt/2k2aiCB
via IFTTT
Thousands of WordPress Sites Hacked Using Recently Disclosed Vulnerability
from The Hacker News http://ift.tt/2k0XVln
via IFTTT
Tuesday, February 7, 2017
ASHACL: Alternative Shapes Constraint Language. (arXiv:1702.01795v1 [cs.AI])
ASHACL, a variant of the W3C Shapes Constraint Language, is designed to determine whether an RDF graph meets some conditions. These conditions are grouped into shapes, which validate whether particular RDF terms each meet the constraints of the shape. Shapes are themselves expressed as RDF triples in an RDF graph, called a shapes graph.
from cs.AI updates on arXiv.org http://ift.tt/2kjRZ82
via IFTTT
Extracting Lifted Mutual Exclusion Invariants from Temporal Planning Domains. (arXiv:1702.01886v1 [cs.AI])
We present a technique for automatically extracting mutual exclusion invariants from temporal planning instances. It first identifies a set of invariant templates by inspecting the lifted representation of the domain and then checks these templates against properties that assure invariance. Our technique builds on other approaches to invariant synthesis presented in the literature, but departs from their limited focus on instantaneous actions by addressing temporal domains. To deal with time, we formulate invariance conditions that account for the entire structure of the actions and the possible concurrent interactions between them. As a result, we construct a significantly more comprehensive technique than previous methods, which is able to find not only invariants for temporal domains, but also a broader set of invariants for non-temporal domains. The experimental results reported in this paper provide evidence that identifying a broader set of invariants results in the generation of fewer multi-valued state variables with larger domains. We show that, in turn, this reduction in the number of variables reflects positively on the performance of a number of temporal planners that use a variable/value representation by significantly reducing their running time.
from cs.AI updates on arXiv.org http://ift.tt/2kpnJLv
via IFTTT
Learning what matters - Sampling interesting patterns. (arXiv:1702.01975v1 [stat.ML])
In the field of exploratory data mining, local structure in data can be described by patterns and discovered by mining algorithms. Although many solutions have been proposed to address the redundancy problems in pattern mining, most of them either provide succinct pattern sets or take the interests of the user into account-but not both. Consequently, the analyst has to invest substantial effort in identifying those patterns that are relevant to her specific interests and goals. To address this problem, we propose a novel approach that combines pattern sampling with interactive data mining. In particular, we introduce the LetSIP algorithm, which builds upon recent advances in 1) weighted sampling in SAT and 2) learning to rank in interactive pattern mining. Specifically, it exploits user feedback to directly learn the parameters of the sampling distribution that represents the user's interests. We compare the performance of the proposed algorithm to the state-of-the-art in interactive pattern mining by emulating the interests of a user. The resulting system allows efficient and interleaved learning and sampling, thus user-specific anytime data exploration. Finally, LetSIP demonstrates favourable trade-offs concerning both quality-diversity and exploitation-exploration when compared to existing methods.
from cs.AI updates on arXiv.org http://ift.tt/2loifPC
via IFTTT
Representations of language in a model of visually grounded speech signal. (arXiv:1702.01991v1 [cs.CL])
We present a visually grounded model of speech perception which projects spoken utterances and images to a joint semantic space. We use a multi-layer recurrent highway network to model the temporal nature of spoken speech, and show that it learns to extract both form and meaning-based linguistic knowledge from the input signal. We carry out an in-depth analysis of the representations used by different components of the trained model and show that encoding of semantic aspects tends to become richer as we go up the hierarchy of layers, whereas encoding of form-related aspects of the language input tends to initially increase and then plateau or decrease.
from cs.AI updates on arXiv.org http://ift.tt/2kFyO9S
via IFTTT
Solving the Brachistochrone Problem by an Influence Diagram. (arXiv:1702.02032v1 [math.OC])
Influence diagrams are a decision-theoretic extension of probabilistic graphical models. In this paper we show how they can be used to solve the Brachistochrone problem. We present results of numerical experiments on this problem, compare the solution provided by the influence diagram with the optimal solution. The R code used for the experiments is presented in the Appendix.
from cs.AI updates on arXiv.org http://ift.tt/2kFftFS
via IFTTT
Landmark-Based Plan Recognition. (arXiv:1604.01277v3 [cs.AI] UPDATED)
Recognition of goals and plans using incomplete evidence from action execution can be done efficiently by using planning techniques. In many applications it is important to recognize goals and plans not only accurately, but also quickly. In this paper, we develop a heuristic approach for recognizing plans based on planning techniques that rely on ordering constraints to filter candidate goals from observations. These ordering constraints are called landmarks in the planning literature, which are facts or actions that cannot be avoided to achieve a goal. We show the applicability of planning landmarks in two settings: first, we use it directly to develop a heuristic-based plan recognition approach; second, we refine an existing planning-based plan recognition approach by pre-filtering its candidate goals. Our empirical evaluation shows that our approach is not only substantially more accurate than the state-of-the-art in all available datasets, it is also an order of magnitude faster.
from cs.AI updates on arXiv.org http://ift.tt/1SOnFhC
via IFTTT
Playing SNES in the Retro Learning Environment. (arXiv:1611.02205v2 [cs.LG] UPDATED)
Mastering a video game requires skill, tactics and strategy. While these attributes may be acquired naturally by human players, teaching them to a computer program is a far more challenging task. In recent years, extensive research was carried out in the field of reinforcement learning and numerous algorithms were introduced, aiming to learn how to perform human tasks such as playing video games. As a result, the Arcade Learning Environment (ALE) (Bellemare et al., 2013) has become a commonly used benchmark environment allowing algorithms to train on various Atari 2600 games. In many games the state-of-the-art algorithms outperform humans. In this paper we introduce a new learning environment, the Retro Learning Environment --- RLE, that can run games on the Super Nintendo Entertainment System (SNES), Sega Genesis and several other gaming consoles. The environment is expandable, allowing for more video games and consoles to be easily added to the environment, while maintaining the same interface as ALE. Moreover, RLE is compatible with Python and Torch. SNES games pose a significant challenge to current algorithms due to their higher level of complexity and versatility.
from cs.AI updates on arXiv.org http://ift.tt/2fxNDcb
via IFTTT
GuessWhat?! Visual object discovery through multi-modal dialogue. (arXiv:1611.08481v2 [cs.AI] UPDATED)
We introduce GuessWhat?!, a two-player guessing game as a testbed for research on the interplay of computer vision and dialogue systems. The goal of the game is to locate an unknown object in a rich image scene by asking a sequence of questions. Higher-level image understanding, like spatial reasoning and language grounding, is required to solve the proposed task. Our key contribution is the collection of a large-scale dataset consisting of 150K human-played games with a total of 800K visual question-answer pairs on 66K images. We explain our design decisions in collecting the dataset and introduce the oracle and questioner tasks that are associated with the two players of the game. We prototyped deep learning models to establish initial baselines of the introduced tasks.
from cs.AI updates on arXiv.org http://ift.tt/2gwMwZi
via IFTTT
NewsQA: A Machine Comprehension Dataset. (arXiv:1611.09830v3 [cs.CL] UPDATED)
We present NewsQA, a challenging machine comprehension dataset of over 100,000 human-generated question-answer pairs. Crowdworkers supply questions and answers based on a set of over 10,000 news articles from CNN, with answers consisting of spans of text from the corresponding articles. We collect this dataset through a four-stage process designed to solicit exploratory questions that require reasoning. A thorough analysis confirms that NewsQA demands abilities beyond simple word matching and recognizing textual entailment. We measure human performance on the dataset and compare it to several strong neural models. The performance gap between humans and machines (0.198 in F1) indicates that significant progress can be made on NewsQA through future research. The dataset is freely available at http://ift.tt/2li9b1K.
from cs.AI updates on arXiv.org http://ift.tt/2g3UxFf
via IFTTT
Webform: Not accessible by anonymous user
from Google Alert - anonymous http://ift.tt/2jZPqH9
via IFTTT
ISS Daily Summary Report – 2/06/2017
from ISS On-Orbit Status Report http://ift.tt/2kohRSG
via IFTTT
[FD] Responsive Filemanger <= 9.11.0 - Arbitrary File Disclosure/Deletion
Source: Gmail -> IFTTT-> Blogger
Smart TV Maker Fined $2.2 Million For Spying on Its 11 Million Users
from The Hacker News http://ift.tt/2jYZLYM
via IFTTT
[FD] SEC Consult SA-20170207 :: Path Traversal, Backdoor accounts & KNX group address password bypass in JUNG Smart Visu server
Source: Gmail -> IFTTT-> Blogger
Ravens: Pierre Garcon would fit type of receiver the team has targeted in free agency in the past - Jamison Hensley (ESPN)
via IFTTT
[FD] Call for Papers: FIRST Amsterdam Technical Colloquium (TC) April 2017
Source: Gmail -> IFTTT-> Blogger
[FD] interpreter bugs
Source: Gmail -> IFTTT-> Blogger
2017 Eclipse State Maps
from NASA's Scientific Visualization Studio: Most Recent Items http://ift.tt/2jWL03O
via IFTTT
Monday, February 6, 2017
I have a new follower on Twitter
Peter Mahoney
Recovering CMO of @NuanceInc and GM of @DragonTweets. Posting my musings on all the critical topics: #marketing, #math, #AI, and #bigdata. Oh, and #robots too
Newton, MA
https://t.co/fFj31hR4jx
Following: 295 - Followers: 510
February 06, 2017 at 11:59PM via Twitter http://twitter.com/nerdCMO
Polish Banks Hacked using Malware Planted on their own Government Site
from The Hacker News http://ift.tt/2ldyeTM
via IFTTT
US Judge Ordered Google to Hand Over Emails Stored On Foreign Servers to FBI
from The Hacker News http://ift.tt/2jWny6T
via IFTTT
I have a new follower on Twitter
Nadya Khoja
Director of Marketing at @Venngage and Creator of https://t.co/tSopzLvTsg. Contributor at @HuffingtonPost @Entrepreneur @TheNextWeb @Businessdotcom
Toronto, Ontario
https://t.co/tnQlCazw8c
Following: 10574 - Followers: 13628
February 06, 2017 at 09:14PM via Twitter http://twitter.com/NadyaKhoja
Reluplex: An Efficient SMT Solver for Verifying Deep Neural Networks. (arXiv:1702.01135v1 [cs.AI])
Deep neural networks have emerged as a widely used and effective means for tackling complex, real-world problems. However, a major obstacle in applying them to safety-critical systems is the great difficulty in providing formal guarantees about their behavior. We present a novel, scalable, and efficient technique for verifying properties of deep neural networks (or providing counter-examples). The technique is based on the simplex method, extended to handle the non-convex Rectified Linear Unit (ReLU) activation function, which is a crucial ingredient in many modern neural networks. The verification procedure tackles neural networks as a whole, without making any simplifying assumptions. We evaluated our technique on a prototype deep neural network implementation of the next-generation Airborne Collision Avoidance System for unmanned aircraft (ACAS Xu). Results show that our technique can successfully prove properties of networks that are an order of magnitude larger than the largest networks verified using existing methods.
from cs.AI updates on arXiv.org http://ift.tt/2kl4Vgd
via IFTTT
Traffic Lights with Auction-Based Controllers: Algorithms and Real-World Data. (arXiv:1702.01205v1 [cs.AI])
Real-time optimization of traffic flow addresses important practical problems: reducing a driver's wasted time, improving city-wide efficiency, reducing gas emissions and improving air quality. Much of the current research in traffic-light optimization relies on extending the capabilities of traffic lights to either communicate with each other or communicate with vehicles. However, before such capabilities become ubiquitous, opportunities exist to improve traffic lights by being more responsive to current traffic situations within the current, already deployed, infrastructure. In this paper, we introduce a traffic light controller that employs bidding within micro-auctions to efficiently incorporate traffic sensor information; no other outside sources of information are assumed. We train and test traffic light controllers on large-scale data collected from opted-in Android cell-phone users over a period of several months in Mountain View, California and the River North neighborhood of Chicago, Illinois. The learned auction-based controllers surpass (in both the relevant metrics of road-capacity and mean travel time) the currently deployed lights, optimized static-program lights, and longer-term planning approaches, in both cities, measured using real user driving data.
from cs.AI updates on arXiv.org http://ift.tt/2kMtYth
via IFTTT
A Theoretical Analysis of First Heuristics of Crowdsourced Entity Resolution. (arXiv:1702.01208v1 [cs.DB])
Entity resolution (ER) is the task of identifying all records in a database that refer to the same underlying entity, and are therefore duplicates of each other. Due to inherent ambiguity of data representation and poor data quality, ER is a challenging task for any automated process. As a remedy, human-powered ER via crowdsourcing has become popular in recent years. Using crowd to answer queries is costly and time consuming. Furthermore, crowd-answers can often be faulty. Therefore, crowd-based ER methods aim to minimize human participation without sacrificing the quality and use a computer generated similarity matrix actively. While, some of these methods perform well in practice, no theoretical analysis exists for them, and further their worst case performances do not reflect the experimental findings. This creates a disparity in the understanding of the popular heuristics for this problem. In this paper, we make the first attempt to close this gap. We provide a thorough analysis of the prominent heuristic algorithms for crowd-based ER. We justify experimental observations with our analysis and information theoretic lower bounds.
from cs.AI updates on arXiv.org http://ift.tt/2lhLwdZ
via IFTTT
Cluster-based Kriging Approximation Algorithms for Complexity Reduction. (arXiv:1702.01313v1 [cs.LG])
Kriging or Gaussian Process Regression is applied in many fields as a non-linear regression model as well as a surrogate model in the field of evolutionary computation. However, the computational and space complexity of Kriging, that is cubic and quadratic in the number of data points respectively, becomes a major bottleneck with more and more data available nowadays. In this paper, we propose a general methodology for the complexity reduction, called cluster Kriging, where the whole data set is partitioned into smaller clusters and multiple Kriging models are built on top of them. In addition, four Kriging approximation algorithms are proposed as candidate algorithms within the new framework. Each of these algorithms can be applied to much larger data sets while maintaining the advantages and power of Kriging. The proposed algorithms are explained in detail and compared empirically against a broad set of existing state-of-the-art Kriging approximation methods on a well-defined testing framework. According to the empirical study, the proposed algorithms consistently outperform the existing algorithms. Moreover, some practical suggestions are provided for using the proposed algorithms.
from cs.AI updates on arXiv.org http://ift.tt/2lhWEYh
via IFTTT
Manyopt: An Extensible Tool for Mixed, Non-Linear Optimization Through SMT Solving. (arXiv:1702.01332v1 [cs.AI])
Optimization of Mixed-Integer Non-Linear Programming (MINLP) supports important decisions in applications such as Chemical Process Engineering. But current solvers have limited ability for deductive reasoning or the use of domain-specific theories, and the management of integrality constraints does not yet exploit automated reasoning tools such as SMT solvers. This seems to limit both scalability and reach of such tools in practice. We therefore present a tool, ManyOpt, for MINLP optimization that enables experimentation with reduction techniques which transform a MINLP problem to feasibility checking realized by an SMT solver. ManyOpt is similar to the SAT solver ManySAT in that it runs a specified number of such reduction techniques in parallel to get the strongest result on a given MINLP problem. The tool is implemented in layers, which we may see as features and where reduction techniques are feature vectors. Some of these features are inspired by known MINLP techniques whereas others are novel and specific to SMT. Our experimental results on standard benchmarks demonstrate the benefits of this approach. The tool supports a variety of SMT solvers and is easily extensible with new features, courtesy of its layered structure. For example, logical formulas for deductive reasoning are easily added to constrain further the optimization of a MINLP problem of interest.
from cs.AI updates on arXiv.org http://ift.tt/2jWdQ9m
via IFTTT
Survey of modern Fault Diagnosis methods in networks. (arXiv:1702.01510v1 [cs.AI])
With the advent of modern computer networks, fault diagnosis has been a focus of research activity. This paper reviews the history of fault diagnosis in networks and discusses the main methods in information gathering section, information analyzing section and diagnosing and revolving section of fault diagnosis in networks. Emphasis will be placed upon knowledge-based methods with discussing the advantages and shortcomings of the different methods. The survey is concluded with a description of some open problems.
from cs.AI updates on arXiv.org http://ift.tt/2kfG4Ii
via IFTTT
Exploring the bidimensional space: A dynamic logic point of view. (arXiv:1702.01601v1 [cs.AI])
We present a family of logics for reasoning about agents' positions and motion in the plane which have several potential applications in the area of multi-agent systems (MAS), such as multi-agent planning and robotics. The most general logic includes (i) atomic formulas for representing the truth of a given fact or the presence of a given agent at a certain position of the plane, (ii) atomic programs corresponding to the four basic orientations in the plane (up, down, left, right) as well as the four program constructs of propositional dynamic logic (sequential composition, nondeterministic composition, iteration and test). As this logic is not computably enumerable, we study some interesting decidable and axiomatizable fragments of it. We also present a decidable extension of the iteration-free fragment of the logic by special programs representing motion of agents in the plane.
from cs.AI updates on arXiv.org http://ift.tt/2kBkBdM
via IFTTT
View Independent Vehicle Make, Model and Color Recognition Using Convolutional Neural Network. (arXiv:1702.01721v1 [cs.CV])
This paper describes the details of Sighthound's fully automated vehicle make, model and color recognition system. The backbone of our system is a deep convolutional neural network that is not only computationally inexpensive, but also provides state-of-the-art results on several competitive benchmarks. Additionally, our deep network is trained on a large dataset of several million images which are labeled through a semi-automated process. Finally we test our system on several public datasets as well as our own internal test dataset. Our results show that we outperform other methods on all benchmarks by significant margins. Our model is available to developers through the Sighthound Cloud API at http://ift.tt/1MlcpaD
from cs.AI updates on arXiv.org http://ift.tt/2jWhOin
via IFTTT
A New Oscillating-Error Technique for Classifiers. (arXiv:1505.05312v6 [cs.AI] UPDATED)
This paper describes a new method for reducing the error in a classifier. It uses an error correction update that includes the very simple rule of either adding or subtracting the error adjustment, based on whether the variable value is currently larger or smaller than the desired value. While a traditional neuron would sum the inputs together and then apply a function to the total, this new method can change the function decision for each input value. This gives added flexibility to the convergence procedure, where through a series of transpositions, variables that are far away can continue towards the desired value, whereas variables that are originally much closer can oscillate from one side to the other. Tests show that the method can successfully classify some benchmark datasets. It can also work in a batch mode, with reduced training times and can be used as part of a neural network architecture. Some comparisons with an earlier wave shape paper are also made.
from cs.AI updates on arXiv.org http://ift.tt/1Bd2M5e
via IFTTT
Value Iteration Networks. (arXiv:1602.02867v3 [cs.AI] UPDATED)
We introduce the value iteration network (VIN): a fully differentiable neural network with a `planning module' embedded within. VINs can learn to plan, and are suitable for predicting outcomes that involve planning-based reasoning, such as policies for reinforcement learning. Key to our approach is a novel differentiable approximation of the value-iteration algorithm, which can be represented as a convolutional neural network, and trained end-to-end using standard backpropagation. We evaluate VIN based policies on discrete and continuous path-planning domains, and on a natural-language based search task. We show that by learning an explicit planning computation, VIN policies generalize better to new, unseen domains.
from cs.AI updates on arXiv.org http://ift.tt/1XhcmQD
via IFTTT
Network of Bandits insure Privacy of end-users. (arXiv:1602.03779v12 [cs.AI] UPDATED)
In order to distribute the best arm identification task as close as possible to the user's devices, on the edge of the Radio Access Network, we propose a new problem setting, where distributed players collaborate to find the best arm. This architecture guarantees privacy to end-users since no events are stored. The only thing that can be observed by an adversary through the core network is aggregated information across users. We provide a first algorithm, Distributed Median Elimination, which is near optimal both in terms of number of transmitted bits in terms of number of pulls per player. This first algorithm requires the distribution of players to be known. Extended Distributed Median Elimination overcomes this limitation, by playing in parallel different instances of Distributed Median Elimination and selecting the best one. Experiments illustrate and complete the analysis. According to the analysis, in comparison to Median Elimination performed on each player, the proposed algorithm shows significant practical improvements.
from cs.AI updates on arXiv.org http://ift.tt/1XmBDce
via IFTTT
An Information-Theoretic Framework for Fast and Robust Unsupervised Learning via Neural Population Infomax. (arXiv:1611.01886v3 [cs.LG] UPDATED)
A framework is presented for unsupervised learning of representations based on infomax principle for large-scale neural populations. We use an asymptotic approximation to the Shannon's mutual information for a large neural population to demonstrate that a good initial approximation to the global information-theoretic optimum can be obtained by a hierarchical infomax method. Starting from the initial solution, an efficient algorithm based on gradient descent of the final objective function is proposed to learn representations from the input datasets, and the method works for complete, overcomplete, and undercomplete bases. As confirmed by numerical experiments, our method is robust and highly efficient for extracting salient features from input datasets. Compared with the main existing methods, our algorithm has a distinct advantage in both the training speed and the robustness of unsupervised representation learning. Moreover, the method is readily extended to train deep structure networks by unsupervised or supervised learning.
from cs.AI updates on arXiv.org http://ift.tt/2fx5xxG
via IFTTT