Latest YouTube Video
Saturday, February 27, 2016
En l'ombre dung bussinet (Anonymous)
from Google Alert - anonymous http://ift.tt/1VKXSrc'ombre_dung_bussinet_(Anonymous)&ct=ga&cd=CAIyGjgxMzAxNTQ0ZWE3M2NhMmQ6Y29tOmVuOlVT&usg=AFQjCNEqa4BuZ027wRKVPBPKFAGYtgKwSQ
via IFTTT
Helas le poure johan (Anonymous)
from Google Alert - anonymous http://ift.tt/1LMq1bJ
via IFTTT
I have a new follower on Twitter
MuleSoft
Connect anything. Change everything.
HQ: San Francisco, CA
http://t.co/NH6KiJTuMy
Following: 4381 - Followers: 30958
February 27, 2016 at 04:02PM via Twitter http://twitter.com/MuleSoft
Help regarding usage of Anonymous functions
from Google Alert - anonymous http://ift.tt/1UqQQcI
via IFTTT
Wishlist functionality for anonymous users
from Google Alert - anonymous http://ift.tt/1S8qtrs
via IFTTT
CTB-Locker Ransomware Spreading Rapidly, Infects Thousands of Web Servers
from The Hacker News http://ift.tt/1QNyRh4
via IFTTT
Chinese ISPs Caught Injecting Ads and Malware into Web Pages
from The Hacker News http://ift.tt/1LldTUg
via IFTTT
Inside The Anonymous World Of Caffeine
from Google Alert - anonymous http://ift.tt/211tSZB
via IFTTT
Anonymous donation to Goodwill becomes the answer to a couple's prayers
from Google Alert - anonymous http://ift.tt/1UpRyXL
via IFTTT
Anonymous quiz takers see different feedback pages
from Google Alert - anonymous http://ift.tt/1Q9Z2uX
via IFTTT
The Tarantula Nebula
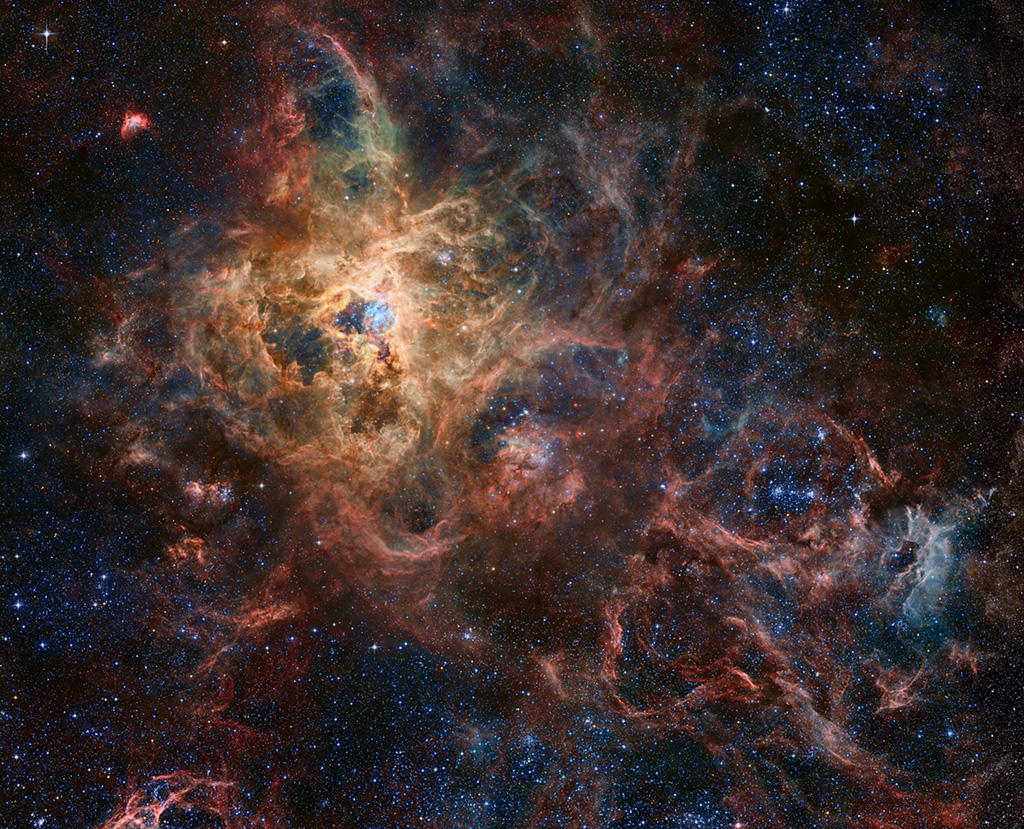
El Niño: GMAO Daily Sea Surface Temperature Anomaly from 1997/1998 and 2015/2016
from NASA's Scientific Visualization Studio: Most Recent Items http://ift.tt/1RuwCvU
via IFTTT
IMERG Rainfall Accumulation from December 2015 through February 2016
from NASA's Scientific Visualization Studio: Most Recent Items http://ift.tt/1oNWQk4
via IFTTT
Friday, February 26, 2016
Anonymous Fred Meyer shopper helps police catch suspect
from Google Alert - anonymous http://ift.tt/1oNUGAO
via IFTTT
Jobs at Anonymous Employer
from Google Alert - anonymous http://ift.tt/1UpBi94
via IFTTT
Config Pages with Paragraphs don't display with Anonymous user.
from Google Alert - anonymous http://ift.tt/1QCyiQA
via IFTTT
Food Addicts Anonymous
from Google Alert - anonymous http://ift.tt/1Ru5I7f
via IFTTT
Jobs at Anonymous Employer
from Google Alert - anonymous http://ift.tt/1RtQT4B
via IFTTT
Ravens Buzz: Joe Flacco, team met to discuss contract, source tells Adam Caplan; Flacco has 3 years left on 6-year deal (ESPN)
via IFTTT
Anonymous command just after entering a field throws an error.
from Google Alert - anonymous http://ift.tt/1pdfD85
via IFTTT
Using anonymous modules and prepend to work with generated code
from Google Alert - anonymous http://ift.tt/1RtojjU
via IFTTT
How To Keep Your Android Phone Secure
from The Hacker News http://ift.tt/1T0UbzH
via IFTTT
Windows 10 Started Showing Ads on LockScreen — Here's How to Turn It OFF
from The Hacker News http://ift.tt/1TbUjwN
via IFTTT
Anonymous user 155860
from Google Alert - anonymous http://ift.tt/1QBPDt5
via IFTTT
I have a new follower on Twitter
Mac SEO Tips
Glenn Bearsky Mac SEO-SMM Tools, Social Marketing Tips for Apple OSX Web Designers. Internet Domination ala Macintosh. Link Different! https://t.co/gu7LPFS1ch
USA
http://t.co/dmc7Im4ExN
Following: 1653 - Followers: 3660
February 26, 2016 at 10:26AM via Twitter http://twitter.com/macseotips
Ravens: Franchise tag used on K Justin Tucker the 2nd-most accurate kicker in history; receives $4.5M salary with tag (ESPN)
via IFTTT
Page cache for anonymous users are not cleared when using Views
from Google Alert - anonymous http://ift.tt/1Q7N8S6
via IFTTT
Apple hires developer of World's Most Secure Messaging App
from The Hacker News http://ift.tt/1Qknyan
via IFTTT
ISS Daily Summary Report – 02/25/16
from ISS On-Orbit Status Report http://ift.tt/1Li2kNv
via IFTTT
I have a new follower on Twitter
Rick Sturm
CEO Enterprise Management Assoc., author of 6 IT books Tweet on: #BI, #BigData, #IoT #ITSM, #SLM, Love the outdoors, & world travel. Quelquefois en français.
Boulder, Colorado
http://t.co/aGCHPy3byi
Following: 12843 - Followers: 18418
February 26, 2016 at 04:13AM via Twitter http://twitter.com/Rick345
I have a new follower on Twitter
David Nordella
@BeneficntGuild collates social media posts for physicians | Speak with your doctor about any medical information | IPA | primary care physicians
Southern California
http://t.co/lbsbDupCSo
Following: 4477 - Followers: 6048
February 26, 2016 at 03:37AM via Twitter http://twitter.com/BeneficentGuild
I have a new follower on Twitter
Rebekah Radice
Award Winning #SocialMedia Writer, Strategist, Author, Speaker. Brand Evangelist @PostPlanner. Co-Founder @InfluencerChat Every Tues 5pm PT #InfluencerChat.
Los Angeles, CA
https://t.co/8yhM89JNyp
Following: 27632 - Followers: 67702
February 26, 2016 at 03:17AM via Twitter http://twitter.com/RebekahRadice
I have a new follower on Twitter
VALiNTRY Jobs
VALiNTRY is a National, Values-based IT & Finance/Accounting Consulting and Staffing firm with offices in Orlando, Nashville, and DFW. Also @VALiNTRY
United States
https://t.co/7cUEuOdNNL
Following: 4452 - Followers: 5683
February 26, 2016 at 01:37AM via Twitter http://twitter.com/VALINTRYjobs
I have a new follower on Twitter
Outset
#Data analysis & management software for #startup #accelerators, but will post about any cool data!
Nashville, TN
http://t.co/mvSzMWXhaE
Following: 483 - Followers: 518
February 26, 2016 at 12:37AM via Twitter http://twitter.com/Outset_Data
Highest, Tallest, and Closest to the Stars
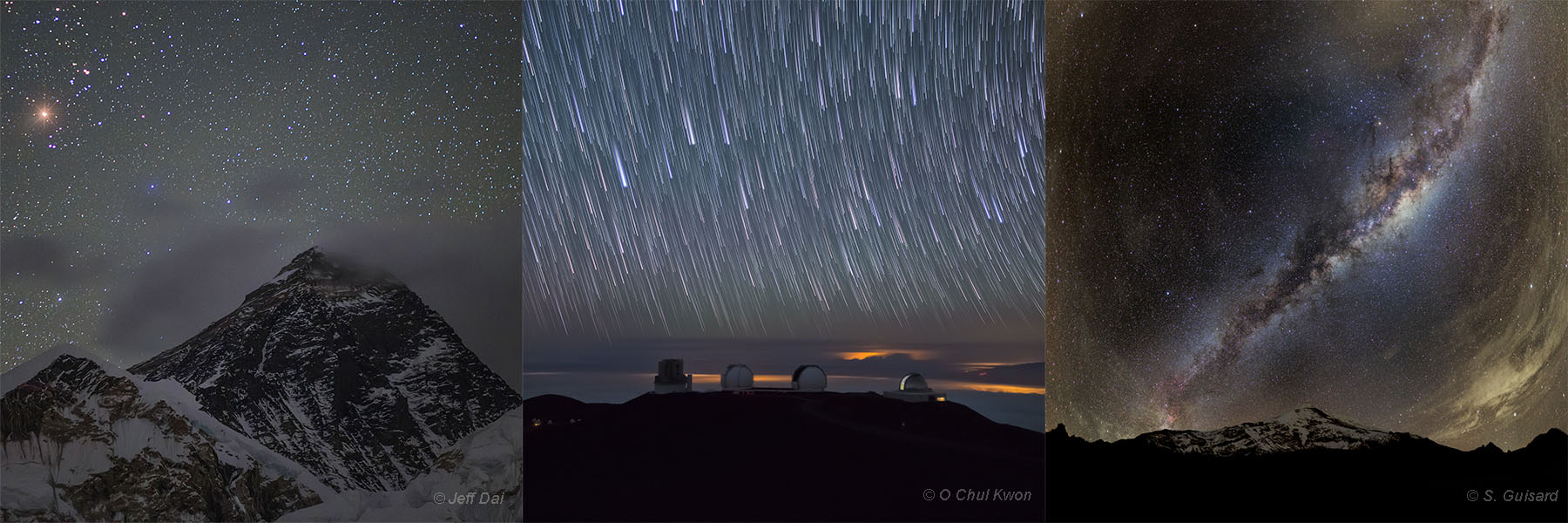
I have a new follower on Twitter
SAM Rizvi
Corporate cog turned entrepreneurial activist who loves Reuben sandwiches.
HKG LAX BOS
http://t.co/6rkyzd0IRx
Following: 2488 - Followers: 4250
February 26, 2016 at 12:08AM via Twitter http://twitter.com/samhkpk
Thursday, February 25, 2016
network-anonymous-i2p
from Google Alert - anonymous http://ift.tt/1Oz60Fs
via IFTTT
I have a new follower on Twitter
Cam Munro
Digital Storyteller #Marketing and #Entrepreneur #blogger & #vlogger, Master of Digital Media (MDM) Toronto.
Toronto, Ontario
https://t.co/y3HCTH0Oj6
Following: 3166 - Followers: 3697
February 25, 2016 at 11:07PM via Twitter http://twitter.com/MunroCameron
Learning functions across many orders of magnitudes. (arXiv:1602.07714v1 [cs.LG])
Learning non-linear functions can be hard when the magnitude of the target function is unknown beforehand, as most learning algorithms are not scale invariant. We propose an algorithm to adaptively normalize these targets. This is complementary to recent advances in input normalization. Importantly, the proposed method preserves the unnormalized outputs whenever the normalization is updated to avoid instability caused by non-stationarity. It can be combined with any learning algorithm and any non-linear function approximation, including the important special case of deep learning. We empirically validate the method in supervised learning and reinforcement learning and apply it to learning how to play Atari 2600 games. Previous work on applying deep learning to this domain relied on clipping the rewards to make learning in different games more homogeneous, but this uses the domain-specific knowledge that in these games counting rewards is often almost as informative as summing these. Using our adaptive normalization we can remove this heuristic without diminishing overall performance, and even improve performance on some games, such as Ms. Pac-Man and Centipede, on which previous methods did not perform well.
from cs.AI updates on arXiv.org http://ift.tt/1Qit6Cj
via IFTTT
Toward Game Level Generation from Gameplay Videos. (arXiv:1602.07721v1 [cs.AI])
Algorithms that generate computer game content require game design knowledge. We present an approach to automatically learn game design knowledge for level design from gameplay videos. We further demonstrate how the acquired design knowledge can be used to generate sections of game levels. Our approach involves parsing video of people playing a game to detect the appearance of patterns of sprites and utilizing machine learning to build a probabilistic model of sprite placement. We show how rich game design information can be automatically parsed from gameplay videos and represented as a set of generative probabilistic models. We use Super Mario Bros. as a proof of concept. We evaluate our approach on a measure of playability and stylistic similarity to the original levels as represented in the gameplay videos.
from cs.AI updates on arXiv.org http://ift.tt/1TaJniP
via IFTTT
Reinforcement Learning of POMDP's using Spectral Methods. (arXiv:1602.07764v1 [cs.AI])
We propose a new reinforcement learning algorithm for partially observable Markov decision processes (POMDP) based on spectral decomposition methods. While spectral methods have been previously employed for consistent learning of (passive) latent variable models such as hidden Markov models, POMDPs are more challenging since the learner interacts with the environment and possibly changes the future observations in the process. We devise a learning algorithm running through episodes, in each episode we employ spectral techniques to learn the POMDP parameters from a trajectory generated by a fixed policy. At the end of the episode, an optimization oracle returns the optimal memoryless planning policy which maximizes the expected reward based on the estimated POMDP model. We prove an order-optimal regret bound w.r.t. the optimal memoryless policy and efficient scaling with respect to the dimensionality of observation and action spaces.
from cs.AI updates on arXiv.org http://ift.tt/1THDTM1
via IFTTT
Top-N Recommendation with Novel Rank Approximation. (arXiv:1602.07783v1 [cs.IR])
The importance of accurate recommender systems has been widely recognized by academia and industry. However, the recommendation quality is still rather low. Recently, a linear sparse and low-rank representation of the user-item matrix has been applied to produce Top-N recommendations. This approach uses the nuclear norm as a convex relaxation for the rank function and has achieved better recommendation accuracy than the state-of-the-art methods. In the past several years, solving rank minimization problems by leveraging nonconvex relaxations has received increasing attention. Some empirical results demonstrate that it can provide a better approximation to original problems than convex relaxation. In this paper, we propose a novel rank approximation to enhance the performance of Top-N recommendation systems, where the approximation error is controllable. Experimental results on real data show that the proposed rank approximation improves the Top-$N$ recommendation accuracy substantially.
from cs.AI updates on arXiv.org http://ift.tt/1THDTvL
via IFTTT
Modeling cumulative biological phenomena with Suppes-Bayes causal networks. (arXiv:1602.07857v1 [cs.AI])
Several statistical techniques have been recently developed for the inference of cancer progression models from the increasingly available NGS cross sectional mutational profiles. A particular algorithm, CAPRI, was proven to be the most efficient with respect to sample size and level of noise in the data. The algorithm combines structural constraints based on Suppes' theory of probabilistic causation and maximum likelihood fit with regularization,and defines constrained Bayesian networks, named Suppes-Bayes Causal Networks(SBCNs), which account for the selective advantage relations among genomic events. In general, SBCNs are effective in modeling any phenomenon driven by cumulative dynamics, as long as the modeled events are persistent. We here discuss on the effectiveness of the SBCN theoretical framework and we investigate the inference of: (i) the priors based on Suppes' theory and (ii) different maximum likelihood regularization parameters on the inference performance estimated on large synthetically generated datasets.
from cs.AI updates on arXiv.org http://ift.tt/24sg8eL
via IFTTT
Probably Approximately Correct Greedy Maximization. (arXiv:1602.07860v1 [cs.AI])
Submodular function maximization finds application in a variety of real-world decision-making problems. However, most existing methods, based on greedy maximization, assume it is computationally feasible to evaluate F, the function being maximized. Unfortunately, in many realistic settings F is too expensive to evaluate exactly even once. We present probably approximately correct greedy maximization, which requires access only to cheap anytime confidence bounds on F and uses them to prune elements. We show that, with high probability, our method returns an approximately optimal set. We propose novel, cheap confidence bounds for conditional entropy, which appears in many common choices of F and for which it is difficult to find unbiased or bounded estimates. Finally, results on a real-world dataset from a multi-camera tracking system in a shopping mall demonstrate that our approach performs comparably to existing methods, but at a fraction of the computational cost.
from cs.AI updates on arXiv.org http://ift.tt/1Qit6lM
via IFTTT
Weight Normalization: A Simple Reparameterization to Accelerate Training of Deep Neural Networks. (arXiv:1602.07868v1 [cs.LG])
We present weight normalization: a reparameterization of the weight vectors in a neural network that decouples the length of those weight vectors from their direction. By reparameterizing the weights in this way we improve the conditioning of the optimization problem and we speed up convergence of stochastic gradient descent. Our reparameterization is inspired by batch normalization but does not introduce any dependencies between the examples in a minibatch. This means that our method can also be applied successfully to recurrent models such as LSTMs and to noise-sensitive applications such as deep reinforcement learning or generative models, for which batch normalization is less well suited. Although our method is much simpler, it still provides much of the speed-up of full batch normalization. In addition, the computational overhead of our method is lower, permitting more optimization steps to be taken in the same amount of time. We demonstrate the usefulness of our method on applications in supervised image recognition, generative modelling, and deep reinforcement learning.
from cs.AI updates on arXiv.org http://ift.tt/1VIg3Oa
via IFTTT
Thompson Sampling is Asymptotically Optimal in General Environments. (arXiv:1602.07905v1 [cs.LG])
We discuss a variant of Thompson sampling for nonparametric reinforcement learning in a countable classes of general stochastic environments. These environments can be non-Markov, non-ergodic, and partially observable. We show that Thompson sampling learns the environment class in the sense that (1) asymptotically its value converges to the optimal value in mean and (2) given a recoverability assumption regret is sublinear.
from cs.AI updates on arXiv.org http://ift.tt/24sga6v
via IFTTT
Causal Discovery from Subsampled Time Series Data by Constraint Optimization. (arXiv:1602.07970v1 [cs.AI])
This paper focuses on causal structure estimation from time series data in which measurements are obtained at a coarser timescale than the causal timescale of the underlying system. Previous work has shown that such sub-sampling can lead to significant errors about the system's causal structure if not properly taken into account. In this paper, we first consider the search for the system timescale causal structures that correspond to a given measurement timescale structure. We provide a constraint satisfaction procedure whose computational performance is several orders of magnitude better than previous approaches, and that has considerably broader applicability. We then consider finite sample data as input, and propose the first constraint optimization approach for recovering the system timescale causal structure. This algorithm optimally recovers from possible conflicts due to statistical errors. More generally, these advances allow for a robust and non-parametric estimation of system timescale causal structures from subsampled time series data.
from cs.AI updates on arXiv.org http://ift.tt/21vgteo
via IFTTT
How effective can simple ordinal peer grading be?. (arXiv:1602.07985v1 [cs.AI])
Ordinal peer grading has been proposed as a simple and scalable solution for computing reliable information about student performance in massive open online courses. The idea is to outsource the grading task to the students themselves as follows. After the end of an exam, each student is asked to rank ---in terms of quality--- a bundle of exam papers by fellow students. An aggregation rule will then combine the individual rankings into a global one that contains all students. We define a broad class of simple aggregation rules and present a theoretical framework for assessing their effectiveness. When statistical information about the grading behaviour of students is available, the framework can be used to compute the optimal rule from this class with respect to a series of performance objectives. For example, a natural rule known as Borda is proved to be optimal when students grade correctly. In addition, we present extensive simulations and a field experiment that validate our theory and prove it to be extremely accurate in predicting the performance of aggregation rules even when only rough information about grading behaviour is available.
from cs.AI updates on arXiv.org http://ift.tt/1RroAE9
via IFTTT
Meta-learning within Projective Simulation. (arXiv:1602.08017v1 [cs.AI])
Learning models of artificial intelligence can nowadays perform very well on a large variety of tasks. However, in practice different task environments are best handled by different learning models, rather than a single, universal, approach. Most non-trivial models thus require the adjustment of several to many learning parameters, which is often done on a case-by-case basis by an external party. Meta-learning refers to the ability of an agent to autonomously and dynamically adjust its own learning parameters, or meta-parameters. In this work we show how projective simulation, a recently developed model of artificial intelligence, can naturally be extended to account for meta-learning in reinforcement learning settings. The projective simulation approach is based on a random walk process over a network of clips. The suggested meta-learning scheme builds upon the same design and employs clip networks to monitor the agent's performance and to adjust its meta-parameters "on the fly". We distinguish between "reflexive adaptation" and "adaptation through learning", and show the utility of both approaches. In addition, a trade-off between flexibility and learning-time is addressed. The extended model is examined on three different kinds of reinforcement learning tasks, in which the agent has different optimal values of the meta-parameters, and is shown to perform well, reaching near-optimal to optimal success rates in all of them, without ever needing to manually adjust any meta-parameter.
from cs.AI updates on arXiv.org http://ift.tt/1THDQQn
via IFTTT
Hip Hop Anonymous
from Google Alert - anonymous http://ift.tt/21veO8D
via IFTTT
SportsCenter Video: Scott Van Pelt's Baltimore accent when discussing Orioles roster has Tim Kurkjian in stitches (ESPN)
via IFTTT
Orioles: OF Dexter Fowler re-signing with the Cubs is an \"absolute gut punch\" for Baltimore, writes Eddie Matz (ESPN)
via IFTTT
[FD] Various Linux Kernel USERNS Issues
Source: Gmail -> IFTTT-> Blogger
[FD] [CVE-2015-5345] Information disclosure vulnerability in Apache Tomcat
Source: Gmail -> IFTTT-> Blogger
[FD] Hacking Passwords, Lesson 11, Available Now!
Source: Gmail -> IFTTT-> Blogger
[FD] XSSer v1.7b: "ZiKA-47 Swarm!" released....
Source: Gmail -> IFTTT-> Blogger
[FD] D-Link, Netgear Router Vulnerabiltiies
Source: Gmail -> IFTTT-> Blogger
[FD] CVE-2015-6541 : Multiple CSRF in Zimbra Mail interface
Source: Gmail -> IFTTT-> Blogger
[FD] CVE ID Request : Proxmox VE Insecure hostname checking (remote root exploit)
Source: Gmail -> IFTTT-> Blogger
[FD] CVE ID Request : Centreon remote code execution
Source: Gmail -> IFTTT-> Blogger
[FD] Ubiquiti Networks UniFi v3.2.10 Generic CSRF Protection Bypass
Source: Gmail -> IFTTT-> Blogger
[FD] CSNC-2016-002 - Open Redirect in OpenAM
Source: Gmail -> IFTTT-> Blogger
[FD] CSNC-2016-001 - XSS in OpenAM
Source: Gmail -> IFTTT-> Blogger
[FD] CVE-2015-0955 - Stored XSS in Adobe Experience Manager (AEM)
Source: Gmail -> IFTTT-> Blogger
NFL Buzz: RB Trent Richardson expected to sign with Ravens - AL.com; No. 3 pick in 2012 draft did not play last season (ESPN)
via IFTTT
I have a new follower on Twitter
HitOpen
Everyone crowdsourcing. #crowdsourcing #marketing
Ottawa, Ontario
https://t.co/cObi7kuuYy
Following: 4791 - Followers: 3538
February 25, 2016 at 09:40AM via Twitter http://twitter.com/htopn
Apple is working on New iPhone Even It Can't Hack
from The Hacker News http://ift.tt/1OwKt08
via IFTTT
Block not appearing for anonymous users
from Google Alert - anonymous http://ift.tt/1S36z11
via IFTTT
Judge Confirms Government Paid CMU Scientists to Hack Tor Users for FBI
from The Hacker News http://ift.tt/1Oww4AZ
via IFTTT
Asus Faces 20 years of Audits Over Poor Wi-Fi Router Security
from The Hacker News http://ift.tt/1TAmcgj
via IFTTT
I have a new follower on Twitter
Andrew Rader
@SpaceX Engineer, @MIT PhD, Know-it-All (TV show) ★ Author: http://t.co/sdWa5mCX9l ★ Youtube: http://t.co/1Z0OfDGtUN ★ Tabletop Card Games: @NewHorizonGames
Planet Earth (for now)
http://t.co/PgljWay80a
Following: 61301 - Followers: 134692
February 25, 2016 at 02:51AM via Twitter http://twitter.com/marsrader
USA's Northeast Megalopolis from Space
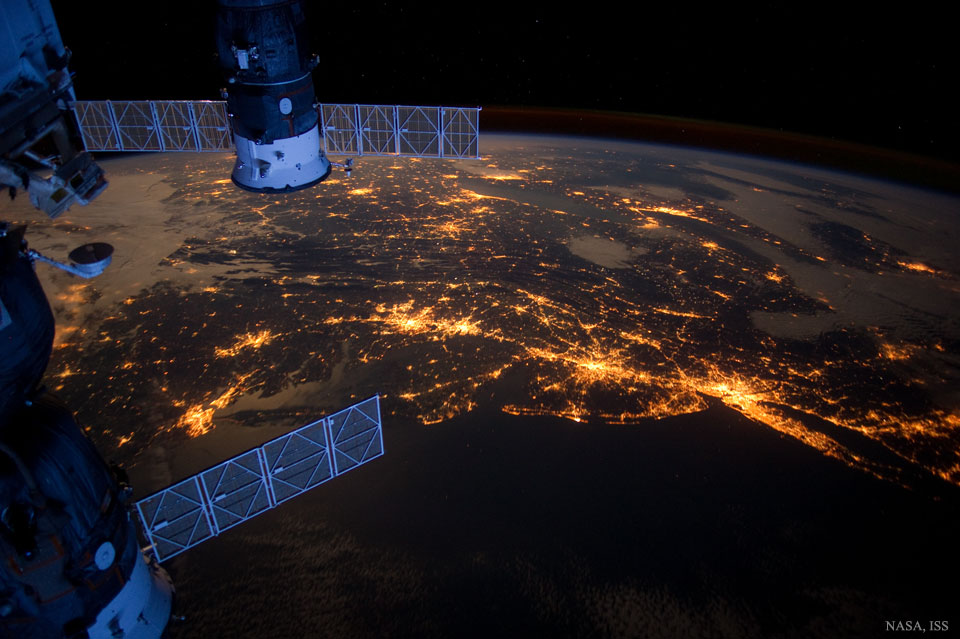
Ozone Transport in the Tropical Western Pacific
from NASA's Scientific Visualization Studio: Most Recent Items http://ift.tt/1Q2yTy7
via IFTTT
Wednesday, February 24, 2016
How to Make Anonymous Payments with Bitcoin
from Google Alert - anonymous http://ift.tt/1T8pqcn
via IFTTT
I have a new follower on Twitter
Steven John
Sagari specialise in providing hosted IT services, with a strong focus of adding value to our customers, by addressing their main frustrations and problems
UK
http://t.co/vGOPYZzERR
Following: 125 - Followers: 90
February 24, 2016 at 11:00PM via Twitter http://twitter.com/SagariLtd
I have a new follower on Twitter
Brian H.
Bellevue
Following: 3240 - Followers: 3305
February 24, 2016 at 09:28PM via Twitter http://twitter.com/TheDataWA
ISS Daily Summary Report – 02/24/16
from ISS On-Orbit Status Report http://ift.tt/1LGN3AF
via IFTTT
Visual Genome: Connecting Language and Vision Using Crowdsourced Dense Image Annotations. (arXiv:1602.07332v1 [cs.CV])
Despite progress in perceptual tasks such as image classification, computers still perform poorly on cognitive tasks such as image description and question answering. Cognition is core to tasks that involve not just recognizing, but reasoning about our visual world. However, models used to tackle the rich content in images for cognitive tasks are still being trained using the same datasets designed for perceptual tasks. To achieve success at cognitive tasks, models need to understand the interactions and relationships between objects in an image. When asked "What vehicle is the person riding?", computers will need to identify the objects in an image as well as the relationships riding(man, carriage) and pulling(horse, carriage) in order to answer correctly that "the person is riding a horse-drawn carriage".
In this paper, we present the Visual Genome dataset to enable the modeling of such relationships. We collect dense annotations of objects, attributes, and relationships within each image to learn these models. Specifically, our dataset contains over 100K images where each image has an average of 21 objects, 18 attributes, and 18 pairwise relationships between objects. We canonicalize the objects, attributes, relationships, and noun phrases in region descriptions and questions answer pairs to WordNet synsets. Together, these annotations represent the densest and largest dataset of image descriptions, objects, attributes, relationships, and question answers.
from cs.AI updates on arXiv.org http://ift.tt/1VFBlvZ
via IFTTT
The Possibilities and Limitations of Private Prediction Markets. (arXiv:1602.07362v1 [cs.GT])
We consider the design of private prediction markets, financial markets designed to elicit predictions about uncertain events without revealing too much information about market participants' actions or beliefs. Our goal is to design market mechanisms in which participants' trades or wagers influence the market's behavior in a way that leads to accurate predictions, yet no single participant has too much influence over what others are able to observe. We study the possibilities and limitations of such mechanisms using tools from differential privacy. We begin by designing a private one-shot wagering mechanism in which bettors specify a belief about the likelihood of a future event and a corresponding monetary wager. Wagers are redistributed among bettors in a way that more highly rewards those with accurate predictions. We provide a class of wagering mechanisms that are guaranteed to satisfy truthfulness, budget balance in expectation, and other desirable properties while additionally guaranteeing epsilon-joint differential privacy in the bettors' reported beliefs, and analyze the trade-off between the achievable level of privacy and the sensitivity of a bettor's payment to her own report. We then ask whether it is possible to obtain privacy in dynamic prediction markets, focusing our attention on the popular cost-function framework in which securities with payments linked to future events are bought and sold by an automated market maker. We show that under general conditions, it is impossible for such a market maker to simultaneously achieve bounded worst-case loss and epsilon-differential privacy without allowing the privacy guarantee to degrade extremely quickly as the number of trades grows, making such markets impractical in settings in which privacy is valued. We conclude by suggesting several avenues for potentially circumventing this lower bound.
from cs.AI updates on arXiv.org http://ift.tt/1VFBlvW
via IFTTT
Parametric Prediction from Parametric Agents. (arXiv:1602.07435v1 [cs.GT])
We consider a problem of prediction based on opinions elicited from heterogeneous rational agents with private information. Making an accurate prediction with a minimal cost requires a joint design of the incentive mechanism and the prediction algorithm. Such a problem lies at the nexus of statistical learning theory and game theory, and arises in many domains such as consumer surveys and mobile crowdsourcing. In order to elicit heterogeneous agents' private information and incentivize agents with different capabilities to act in the principal's best interest, we design an optimal joint incentive mechanism and prediction algorithm called COPE (COst and Prediction Elicitation), the analysis of which offers several valuable engineering insights. First, when the costs incurred by the agents are linear in the exerted effort, COPE corresponds to a "crowd contending" mechanism, where the principal only employs the agent with the highest capability. Second, when the costs are quadratic, COPE corresponds to a "crowd-sourcing" mechanism that employs multiple agents with different capabilities at the same time. Numerical simulations show that COPE improves the principal's profit and the network profit significantly (larger than 30% in our simulations), comparing to those mechanisms that assume all agents have equal capabilities.
from cs.AI updates on arXiv.org http://ift.tt/21hPaYk
via IFTTT
A Bayesian Approach to the Data Description Problem. (arXiv:1602.07507v1 [cs.LG])
In this paper, we address the problem of data description using a Bayesian framework. The goal of data description is to draw a boundary around objects of a certain class of interest to discriminate that class from the rest of the feature space. Data description is also known as one-class learning and has a wide range of applications.
The proposed approach uses a Bayesian framework to precisely compute the class boundary and therefore can utilize domain information in form of prior knowledge in the framework. It can also operate in the kernel space and therefore recognize arbitrary boundary shapes. Moreover, the proposed method can utilize unlabeled data in order to improve accuracy of discrimination.
We evaluate our method using various real-world datasets and compare it with other state of the art approaches of data description. Experiments show promising results and improved performance over other data description and one-class learning algorithms.
from cs.AI updates on arXiv.org http://ift.tt/1T89d77
via IFTTT
Multilingual Twitter Sentiment Classification: The Role of Human Annotators. (arXiv:1602.07563v1 [cs.CL])
What are the limits of automated Twitter sentiment classification? We analyze a large set of manually labeled tweets in different languages, use them as training data, and construct automated classification models. It turns out that the quality of classification models depends much more on the quality and size of training data than on the type of the model trained. Experimental results indicate that there is no statistically significant difference between the performance of the top classification models. We quantify the quality of training data by applying various annotator agreement measures, and identify the weakest points of different datasets. We show that the model performance approaches the inter-annotator agreement when the size of the training set is sufficiently large. However, it is crucial to regularly monitor the self- and inter-annotator agreements since this improves the training datasets and consequently the model performance. Finally, we show that there is strong evidence that humans perceive the sentiment classes (negative, neutral, and positive) as ordered.
from cs.AI updates on arXiv.org http://ift.tt/1VFBkrU
via IFTTT
Stochastic Shortest Path with Energy Constraints in POMDPs. (arXiv:1602.07565v1 [cs.AI])
We consider partially observable Markov decision processes (POMDPs) with a set of target states and positive integer costs associated with every transition. The traditional optimization objective (stochastic shortest path) asks to minimize the expected total cost until the target set is reached. We extend the traditional framework of POMDPs to model energy consumption, which represents a hard constraint. The energy levels may increase and decrease with transitions, and the hard constraint requires that the energy level must remain positive in all steps till the target is reached. First, we present a novel algorithm for solving POMDPs with energy levels, developing on existing POMDP solvers and using RTDP as its main method. Our second contribution is related to policy representation. For larger POMDP instances the policies computed by existing solvers are too large to be understandable. We present an automated procedure based on machine learning techniques that automatically extracts important decisions of the policy allowing us to compute succinct human readable policies. Finally, we show experimentally that our algorithm performs well and computes succinct policies on a number of POMDP instances from the literature that were naturally enhanced with energy levels.
from cs.AI updates on arXiv.org http://ift.tt/21hPaYg
via IFTTT
Time and Activity Sequence Prediction of Business Process Instances. (arXiv:1602.07566v1 [cs.AI])
The ability to know in advance the trend of running process instances, with respect to different features, such as the expected completion time, would allow business managers to timely counteract to undesired situations, in order to prevent losses. Therefore, the ability to accurately predict future features of running business process instances would be a very helpful aid when managing processes, especially under service level agreement constraints. However, making such accurate forecasts is not easy: many factors may influence the predicted features.
Many approaches have been proposed to cope with this problem but all of them assume that the underling process is stationary. However, in real cases this assumption is not always true. In this work we present new methods for predicting the remaining time of running cases. In particular we propose a method, assuming process stationarity, which outperforms the state-of-the-art and two other methods which are able to make predictions even with non-stationary processes. We also describe an approach able to predict the full sequence of activities that a running case is going to take. All these methods are extensively evaluated on two real case studies.
from cs.AI updates on arXiv.org http://ift.tt/1VFBlvU
via IFTTT
A Survey on Domain-Specific Languages for Machine Learning in Big Data. (arXiv:1602.07637v1 [cs.SE])
The amount of data generated in the modern society is increasing rapidly. New problems and novel approaches of data capture, storage, analysis and visualization are responsible for the emergence of the Big Data research field. Machine Learning algorithms can be used in Big Data to make better and more accurate inferences. However, because of the challenges Big Data imposes, these algorithms need to be adapted and optimized to specific applications. One important decision made by software engineers is the choice of the language that is used in the implementation of these algorithms. Therefore, this literature survey identifies and describes domain-specific languages and frameworks used for Machine Learning in Big Data. By doing this, software engineers can then make more informed choices and beginners have an overview of the main languages used in this domain.
from cs.AI updates on arXiv.org http://ift.tt/21hPdDv
via IFTTT
New Results on Equilibria in Strategic Candidacy. (arXiv:1306.1849v2 [cs.GT] UPDATED)
We consider a voting setting where candidates have preferences about the outcome of the election and are free to join or leave the election. The corresponding candidacy game, where candidates choose strategically to participate or not, has been studied %initially by Dutta et al., who showed that no non-dictatorial voting procedure satisfying unanimity is candidacy-strategyproof, that is, is such that the joint action where all candidates enter the election is always a pure strategy Nash equilibrium. Dutta et al. also showed that for some voting tree procedures, there are candidacy games with no pure Nash equilibria, and that for the rule that outputs the sophisticated winner of voting by successive elimination, all games have a pure Nash equilibrium. No results were known about other voting rules. Here we prove several such results. For four candidates, the message is, roughly, that most scoring rules (with the exception of Borda) do not guarantee the existence of a pure Nash equilibrium but that Condorcet-consistent rules, for an odd number of voters, do. For five candidates, most rules we study no longer have this guarantee. Finally, we identify one prominent rule that guarantees the existence of a pure Nash equilibrium for any number of candidates (and for an odd number of voters): the Copeland rule. We also show that under mild assumptions on the voting rule, the existence of strong equilibria cannot be guaranteed.
from cs.AI updates on arXiv.org http://ift.tt/14QStD1
via IFTTT
ASlib: A Benchmark Library for Algorithm Selection. (arXiv:1506.02465v2 [cs.AI] UPDATED)
The task of algorithm selection involves choosing an algorithm from a set of algorithms on a per-instance basis in order to exploit the varying performance of algorithms over a set of instances. The algorithm selection problem is attracting increasing attention from researchers and practitioners in AI. Years of fruitful applications in a number of domains have resulted in a large amount of data, but the community lacks a standard format or repository for this data. This situation makes it difficult to share and compare different approaches effectively, as is done in other, more established fields. It also unnecessarily hinders new researchers who want to work in this area. To address this problem, we introduce a standardized format for representing algorithm selection scenarios and a repository that contains a growing number of data sets from the literature. Our format has been designed to be able to express a wide variety of different scenarios. Demonstrating the breadth and power of our platform, we describe a set of example experiments that build and evaluate algorithm selection models through a common interface. The results display the potential of algorithm selection to achieve significant performance improvements across a broad range of problems and algorithms.
from cs.AI updates on arXiv.org http://ift.tt/1cHsY1G
via IFTTT
Identification and classification of TCM syndrome types among patients with vascular mild cognitive impairment using latent tree analysis. (arXiv:1601.06923v2 [cs.AI] UPDATED)
Objective: To treat patients with vascular mild cognitive impairment (VMCI) using TCM, it is necessary to classify the patients into TCM syndrome types and to apply different treatments to different types. We investigate how to properly carry out the classification using a novel data-driven method known as latent tree analysis.
Method: A cross-sectional survey on VMCI was carried out in several regions in northern China from 2008 to 2011, which resulted in a data set that involves 803 patients and 93 symptoms. Latent tree analysis was performed on the data to reveal symptom co-occurrence patterns, and the patients were partitioned into clusters in multiple ways based on the patterns. The patient clusters were matched up with syndrome types, and population statistics of the clusters are used to quantify the syndrome types and to establish classification rules.
Results: Eight syndrome types are identified: Qi Deficiency, Qi Stagnation, Blood Deficiency, Blood Stasis, Phlegm-Dampness, Fire-Heat, Yang Deficiency, and Yin Deficiency. The prevalence and symptom occurrence characteristics of each syndrome type are determined. Quantitative classification rules are established for determining whether a patient belongs to each of the syndrome types.
Conclusions: A solution for the TCM syndrome classification problem associated with VMCI is established based on the latent tree analysis of unlabeled symptom survey data. The results can be used as a reference in clinic practice to improve the quality of syndrome differentiation and to reduce diagnosis variances across physicians. They can also be used for patient selection in research projects aimed at finding biomarkers for the syndrome types and in randomized control trials aimed at determining the efficacy of TCM treatments of VMCI.
from cs.AI updates on arXiv.org http://ift.tt/1KDJtHb
via IFTTT
Orioles: SP Yovani Gallardo agrees to 2-year, $22 million contract; originally had agreed to 3-year, $35 million deal (ESPN)
via IFTTT
Ravens: K Justin Tucker will receive franchise tag if team is unable to reach long-term contract, GM Ozzie Newsome said (ESPN)
via IFTTT
Anonymous user 41069a
from Google Alert - anonymous http://ift.tt/1p5sBF3
via IFTTT
Status message for anonymous users.
from Google Alert - anonymous http://ift.tt/1oByitT
via IFTTT
Anonymous partnerships among MSM and transgender women (TW)
from Google Alert - anonymous http://ift.tt/1Q0WsHk
via IFTTT
'Anonymous' releases police officers' personal information
from Google Alert - anonymous http://ift.tt/1T7q75Z
via IFTTT
Ravens: Ohio St. DE Joey Bosa, Florida CB Vernon Hargreaves are players team could focus on at combine - Jamison Hensley (ESPN)
via IFTTT
Top 5 Facts about Anonymous
from Google Alert - anonymous http://ift.tt/1LcEnax
via IFTTT
'Anonymous' releases police officers' personal information
from Google Alert - anonymous http://ift.tt/1LFSS1t
via IFTTT
ISS Daily Summary Report – 02/23/16
from ISS On-Orbit Status Report http://ift.tt/1R0VjNN
via IFTTT
Just One Device? No, Government wants Apple to Unlock 12 More iPhones
from The Hacker News http://ift.tt/1PZfpdJ
via IFTTT
[FD] GTA Firewall GB-OS v6.2.02 - Filter Bypass & Persistent Vulnerability
Source: Gmail -> IFTTT-> Blogger