Latest YouTube Video
Saturday, June 18, 2016
New Marijuana Anonymous Meeting
from Google Alert - anonymous http://ift.tt/1SbLeh5
via IFTTT
Orioles: Buck Showalter says Manny Machado will drop his appeal and begin serving 4-game suspension Sunday vs. Blue Jays (ESPN)
via IFTTT
Orioles Video: Jonathan Schoop crushes R.A. Dickey's knuckleball to right for a solo homer in 4-2 win over the Blue Jays (ESPN)
via IFTTT
An Anonymous Food Company
from Google Alert - anonymous http://ift.tt/1UprDyq
via IFTTT
I have a new follower on Twitter
Anthony Pisapia
#SocEnt, father, husband, building tech, ed, and nonprofit startups. Chief Information and Innovation Officer @ Tower Hill School, Fmr. Head @zipcodewilm
Wilmington, DE
Following: 6350 - Followers: 7657
June 18, 2016 at 04:13PM via Twitter http://twitter.com/anthonypisapia
Orioles reinstate P Yovani Gallardo (shoulder) and SS J.J. Hardy (foot) from DL; P T.J. McFarland optioned to Triple-A (ESPN)
via IFTTT
[FD] CVE-2016-0199 / MS16-063: MSIE 11 garbage collector attribute type confusion
Source: Gmail -> IFTTT-> Blogger
[FD] Multiple vulnerabilities in squid 0.4.16_2 running on pfSense
Source: Gmail -> IFTTT-> Blogger
[FD] [ERPSCAN-16-014] SAP NetWeaver AS Java NavigationURLTester - XSS vulnerability
Source: Gmail -> IFTTT-> Blogger
[FD] [ERPSCAN-16-012] SAP NetWeaver AS JAVA - directory traversal vulnerability
Source: Gmail -> IFTTT-> Blogger
Comet PanSTARRS in the Southern Fish
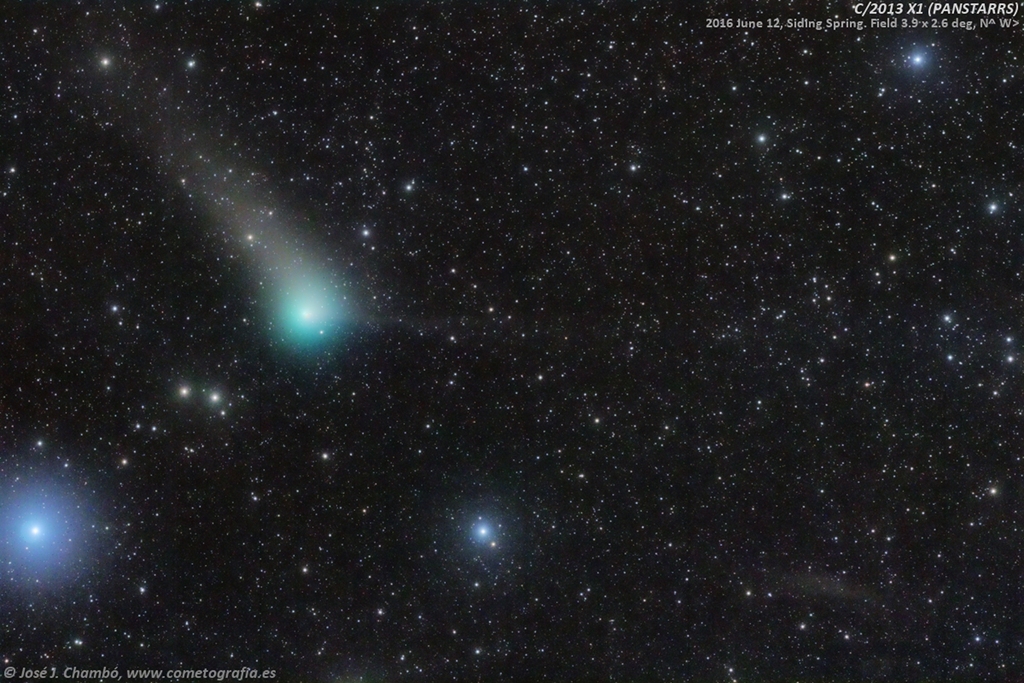
Orioles option P Mike Wright to Triple-A Norfolk; owns a 3-4 record in 12 starts with a 6.12 ERA this season (ESPN)
via IFTTT
Dynamic Earth-A New Beginning
from NASA's Scientific Visualization Studio: Most Recent Items http://ift.tt/1tuFaeR
via IFTTT
Friday, June 17, 2016
I have a new follower on Twitter
AT: Sports US
Part of the FASTEST growing FREE betting/tipping community!! Loads of fantastic OFFERS. AMERICAN SPORTS EXPERT!!
https://t.co/2RyHF1JlEt
Following: 2324 - Followers: 129
June 17, 2016 at 10:59PM via Twitter http://twitter.com/ATSportsUS
MLB: Blue Jays OF Michael Saunders hits his 3rd home run of the game in the 6th inning vs. Orioles; driven in 8 RBIs (ESPN)
via IFTTT
Anonymous user 415cf8
from Google Alert - anonymous http://ift.tt/1V34mzy
via IFTTT
Orioles: SS J.J. Hardy to be activated from the disabled list either Saturday or Sunday according to Buck Showalter (ESPN)
via IFTTT
The Pirate Bay Founder Ordered to Pay $395,000 Fine in Lawsuit he didn't even know about
from The Hacker News http://ift.tt/21qK6g0
via IFTTT
Anonymous Posting in Discussions
from Google Alert - anonymous http://ift.tt/1W3yAHM
via IFTTT
Formal Security-Proved Mobile Anonymous Authentication Protocols with Credit-Based ...
from Google Alert - anonymous http://ift.tt/1UfOsaN
via IFTTT
Anonymous hacks ISIS Twitter account, turns it into a gay pride parade
from Google Alert - anonymous http://ift.tt/1UmzxbI
via IFTTT
Github accounts Hacked in 'Password reuse attack'
from The Hacker News http://ift.tt/1XsXAJn
via IFTTT
Anonymous tip line created in village
from Google Alert - anonymous http://ift.tt/1UzqpC4
via IFTTT
ISS Daily Summary Report – 06/16/16
from ISS On-Orbit Status Report http://ift.tt/21pNbwJ
via IFTTT
Northern Lights above Lofoten
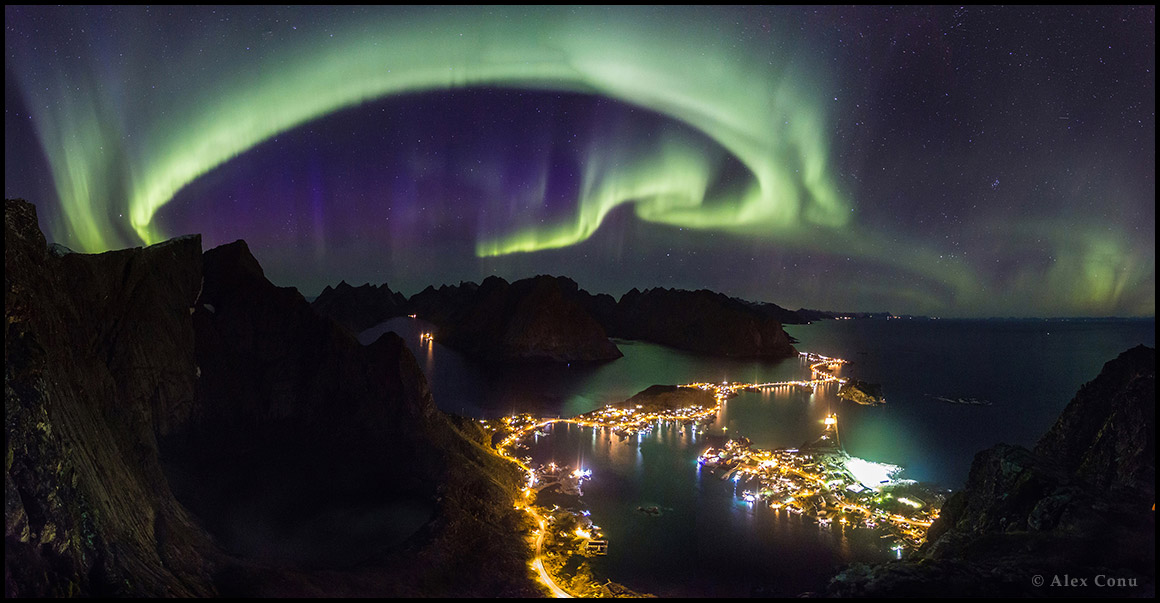
Thursday, June 16, 2016
Orioles Video: Adam Jones clears the Green Monster for a 2-run homer in 5-1 victory over the Red Sox (ESPN)
via IFTTT
Ravens: RB Trent Richardson will have knee scoped after being limited during OTAs on Wednesday (ESPN)
via IFTTT
Assessing Human Error Against a Benchmark of Perfection. (arXiv:1606.04956v1 [cs.AI])
An increasing number of domains are providing us with detailed trace data on human decisions in settings where we can evaluate the quality of these decisions via an algorithm. Motivated by this development, an emerging line of work has begun to consider whether we can characterize and predict the kinds of decisions where people are likely to make errors.
To investigate what a general framework for human error prediction might look like, we focus on a model system with a rich history in the behavioral sciences: the decisions made by chess players as they select moves in a game. We carry out our analysis at a large scale, employing datasets with several million recorded games, and using chess tablebases to acquire a form of ground truth for a subset of chess positions that have been completely solved by computers but remain challenging even for the best players in the world.
We organize our analysis around three categories of features that we argue are present in most settings where the analysis of human error is applicable: the skill of the decision-maker, the time available to make the decision, and the inherent difficulty of the decision. We identify rich structure in all three of these categories of features, and find strong evidence that in our domain, features describing the inherent difficulty of an instance are significantly more powerful than features based on skill or time.
from cs.AI updates on arXiv.org http://ift.tt/1Px4qGf
via IFTTT
Robust Active Perception via Data-association aware Belief Space planning. (arXiv:1606.05124v1 [cs.RO])
We develop a belief space planning (BSP) approach that advances the state of the art by incorporating reasoning about data association (DA) within planning, while considering additional sources of uncertainty. Existing BSP approaches typically assume data association is given and perfect, an assumption that can be harder to justify while operating, in the presence of localization uncertainty, in ambiguous and perceptually aliased environments. In contrast, our data association aware belief space planning (DA-BSP) approach explicitly reasons about DA within belief evolution, and as such can better accommodate these challenging real world scenarios. In particular, we show that due to perceptual aliasing, the posterior belief becomes a mixture of probability distribution functions, and design cost functions that measure the expected level of ambiguity and posterior uncertainty. Using these and standard costs (e.g.~control penalty, distance to goal) within the objective function, yields a general framework that reliably represents action impact, and in particular, capable of active disambiguation. Our approach is thus applicable to robust active perception and autonomous navigation in perceptually aliased environments. We demonstrate key aspects in basic and realistic simulations.
from cs.AI updates on arXiv.org http://ift.tt/1UYUNBq
via IFTTT
Deep Reinforcement Learning Discovers Internal Models. (arXiv:1606.05174v1 [cs.AI])
Deep Reinforcement Learning (DRL) is a trending field of research, showing great promise in challenging problems such as playing Atari, solving Go and controlling robots. While DRL agents perform well in practice we are still lacking the tools to analayze their performance. In this work we present the Semi-Aggregated MDP (SAMDP) model. A model best suited to describe policies exhibiting both spatial and temporal hierarchies. We describe its advantages for analyzing trained policies over other modeling approaches, and show that under the right state representation, like that of DQN agents, SAMDP can help to identify skills. We detail the automatic process of creating it from recorded trajectories, up to presenting it on t-SNE maps. We explain how to evaluate its fitness and show surprising results indicating high compatibility with the policy at hand. We conclude by showing how using the SAMDP model, an extra performance gain can be squeezed from the agent.
from cs.AI updates on arXiv.org http://ift.tt/1YvOQl1
via IFTTT
SQuAD: 100,000+ Questions for Machine Comprehension of Text. (arXiv:1606.05250v1 [cs.CL])
We present a new reading comprehension dataset, SQuAD, consisting of 100,000+ questions posed by crowdworkers on a set of Wikipedia articles, where the answer to each question is a segment of text from the corresponding reading passage. We analyze the dataset in both manual and automatic ways to understand the types of reasoning required to answer the questions, leaning heavily on dependency and constituency trees. We built a strong logistic regression model, which achieves an F1 score of 51.0%, a significant improvement over a simple baseline (20%). However, human performance (86.8%) is much higher, indicating that the dataset presents a good challenge problem for future research.
from cs.AI updates on arXiv.org http://ift.tt/1UYUfeT
via IFTTT
Successor Features for Transfer in Reinforcement Learning. (arXiv:1606.05312v1 [cs.AI])
Transfer in reinforcement learning refers to the notion that generalization should occur not only within a task but also across tasks. Our focus is on transfer where the reward functions vary across tasks while the environment's dynamics remain the same. The method we propose rests on two key ideas: "successor features," a value function representation that decouples the dynamics of the environment from the rewards, and "generalized policy improvement," a generalization of dynamic programming's policy improvement step that considers a set of policies rather than a single one. Put together, the two ideas lead to an approach that integrates seamlessly within the reinforcement learning framework and allows transfer to take place between tasks without any restriction. The proposed method also provides performance guarantees for the transferred policy even before any learning has taken place. We derive two theorems that set our approach in firm theoretical ground and present experiments that show that it successfully promotes transfer in practice.
from cs.AI updates on arXiv.org http://ift.tt/1YvP63w
via IFTTT
Unsupervised Risk Estimation Using Only Conditional Independence Structure. (arXiv:1606.05313v1 [cs.LG])
We show how to estimate a model's test error from unlabeled data, on distributions very different from the training distribution, while assuming only that certain conditional independencies are preserved between train and test. We do not need to assume that the optimal predictor is the same between train and test, or that the true distribution lies in any parametric family. We can also efficiently differentiate the error estimate to perform unsupervised discriminative learning. Our technical tool is the method of moments, which allows us to exploit conditional independencies in the absence of a fully-specified model. Our framework encompasses a large family of losses including the log and exponential loss, and extends to structured output settings such as hidden Markov models.
from cs.AI updates on arXiv.org http://ift.tt/23f7VsK
via IFTTT
On the expressive power of deep neural networks. (arXiv:1606.05336v1 [stat.ML])
We study the expressivity of deep neural networks with random weights. We provide several results, both theoretical and experimental, precisely characterizing their functional properties in terms of the depth and width of the network. In doing so, we illustrate inherent connections between the length of a latent trajectory, local neuron transitions, and network activation patterns. The latter, a notion defined in this paper, is further studied using properties of hyperplane arrangements, which also help precisely characterize the effect of the neural network on the input space. We further show dualities between changes to the latent state and changes to the network weights, and between the number of achievable activation patterns and the number of achievable labellings over input data. We see that the depth of the network affects all of these quantities exponentially, while the width appears at most as a base. These results also suggest that the remaining depth of a neural network is an important determinant of expressivity, supported by experiments on MNIST and CIFAR-10.
from cs.AI updates on arXiv.org http://ift.tt/1tznj7m
via IFTTT
LSTM Neural Reordering Feature for Statistical Machine Translation. (arXiv:1512.00177v3 [cs.CL] UPDATED)
Artificial neural networks are powerful models, which have been widely applied into many aspects of machine translation, such as language modeling and translation modeling. Though notable improvements have been made in these areas, the reordering problem still remains a challenge in statistical machine translations. In this paper, we present a novel neural reordering model that directly models word pairs and alignment. By utilizing LSTM recurrent neural networks, much longer context could be learned for reordering prediction. Experimental results on NIST OpenMT12 Arabic-English and Chinese-English 1000-best rescoring task show that our LSTM neural reordering feature is robust and achieves significant improvements over various baseline systems.
from cs.AI updates on arXiv.org http://ift.tt/1Tt5y0j
via IFTTT
Auxiliary Deep Generative Models. (arXiv:1602.05473v4 [stat.ML] UPDATED)
Deep generative models parameterized by neural networks have recently achieved state-of-the-art performance in unsupervised and semi-supervised learning. We extend deep generative models with auxiliary variables which improves the variational approximation. The auxiliary variables leave the generative model unchanged but make the variational distribution more expressive. Inspired by the structure of the auxiliary variable we also propose a model with two stochastic layers and skip connections. Our findings suggest that more expressive and properly specified deep generative models converge faster with better results. We show state-of-the-art performance within semi-supervised learning on MNIST, SVHN and NORB datasets.
from cs.AI updates on arXiv.org http://ift.tt/1Oh5oUN
via IFTTT
Proving the Incompatibility of Efficiency and Strategyproofness via SMT Solving. (arXiv:1604.05692v3 [cs.GT] UPDATED)
Two important requirements when aggregating the preferences of multiple agents are that the outcome should be economically efficient and the aggregation mechanism should not be manipulable. In this paper, we provide a computer-aided proof of a sweeping impossibility using these two conditions for randomized aggregation mechanisms. More precisely, we show that every efficient aggregation mechanism can be manipulated for all expected utility representations of the agents' preferences. This settles a conjecture by Aziz et al. [2013b] and strengthens a number of existing theorems, including statements that were shown within the special domain of assignment. Our proof is obtained by formulating the claim as a satisfiability problem over predicates from real-valued arithmetic, which is then checked using an SMT (satisfiability modulo theories) solver. To the best of our knowledge, this is the first application of SMT solvers in computational social choice.
from cs.AI updates on arXiv.org http://ift.tt/1SS54hL
via IFTTT
[FD] CVE-2016-5709 - Use of Weak Encryption Algorithm in Solarwinds Virtualization Manager
Source: Gmail -> IFTTT-> Blogger
Sex, Less Death': Anonymous Hacks ISIS Twitter Accounts, Tweets Gay Pride
from Google Alert - anonymous http://ift.tt/266efo5
via IFTTT
Ravens: Joe Flacco believes he will be under center when training camp begins July 28; sidelined since Nov. knee injury (ESPN)
via IFTTT
[FD] Stack Overflow in BLAT
Source: Gmail -> IFTTT-> Blogger
[FD] Papouch TME Temperature & Humidity Thermometers - Multiple Vulnerabilities
Source: Gmail -> IFTTT-> Blogger
[FD] Blindspot Advisory: HTTP Header Injection in Python urllib
Source: Gmail -> IFTTT-> Blogger
VNCserver - with anonymous TLS certificate?
from Google Alert - anonymous http://ift.tt/1Q8ecEq
via IFTTT
Allow for anonymous users to remove csrf token, remove members_only wiget?
from Google Alert - anonymous http://ift.tt/1UQsVCo
via IFTTT
Anonymous spam Isis with gay porn after Orlando attack
from Google Alert - anonymous http://ift.tt/1UAA47c
via IFTTT
Show available plans to anonymous users
from Google Alert - anonymous http://ift.tt/1UWvwrI
via IFTTT
Wednesday, June 15, 2016
International artists overtake Copper Mountain for Altitude Anonymous music festival
from Google Alert - anonymous http://ift.tt/1UPVfET
via IFTTT
ISS Daily Summary Report – 06/15/16
from ISS On-Orbit Status Report http://ift.tt/1ZSyXny
via IFTTT
I have a new follower on Twitter
Elizabeth Fleming
Following: 3689 - Followers: 24
June 15, 2016 at 09:57PM via Twitter http://twitter.com/_harvey34
Impossibility in Belief Merging. (arXiv:1606.04589v1 [cs.AI])
With the aim of studying social properties of belief merging and having a better understanding of impossibility, we extend in three ways the framework of logic-based merging introduced by Konieczny and Pino P\'erez. First, at the level of representation of the information, we pass from belief bases to complex epistemic states. Second, the profiles are represented as functions of finite societies to the set of epistemic states (a sort of vectors) and not as multisets of epistemic states. Third, we extend the set of rational postulates in order to consider the epistemic versions of the classical postulates of Social Choice Theory: Standard Domain, Pareto Property, Independence of Irrelevant Alternatives and Absence of Dictator. These epistemic versions of social postulates are given, essentially, in terms of the finite propositional logic. We state some representation theorems for these operators. These extensions and representation theorems allow us to establish an epistemic and very general version of Arrow's Impossibility Theorem. One of the interesting features of our result, is that it holds for different representations of epistemic states; for instance conditionals, Ordinal Conditional functions and, of course, total preorders.
from cs.AI updates on arXiv.org http://ift.tt/1rqVHPC
via IFTTT
Deep Reinforcement Learning With Macro-Actions. (arXiv:1606.04615v1 [cs.LG])
Deep reinforcement learning has been shown to be a powerful framework for learning policies from complex high-dimensional sensory inputs to actions in complex tasks, such as the Atari domain. In this paper, we explore output representation modeling in the form of temporal abstraction to improve convergence and reliability of deep reinforcement learning approaches. We concentrate on macro-actions, and evaluate these on different Atari 2600 games, where we show that they yield significant improvements in learning speed. Additionally, we show that they can even achieve better scores than DQN. We offer analysis and explanation for both convergence and final results, revealing a problem deep RL approaches have with sparse reward signals.
from cs.AI updates on arXiv.org http://ift.tt/1rqV60t
via IFTTT
Natural Language Generation as Planning under Uncertainty Using Reinforcement Learning. (arXiv:1606.04686v1 [cs.CL])
We present and evaluate a new model for Natural Language Generation (NLG) in Spoken Dialogue Systems, based on statistical planning, given noisy feedback from the current generation context (e.g. a user and a surface realiser). We study its use in a standard NLG problem: how to present information (in this case a set of search results) to users, given the complex trade- offs between utterance length, amount of information conveyed, and cognitive load. We set these trade-offs by analysing existing MATCH data. We then train a NLG pol- icy using Reinforcement Learning (RL), which adapts its behaviour to noisy feed- back from the current generation context. This policy is compared to several base- lines derived from previous work in this area. The learned policy significantly out- performs all the prior approaches.
from cs.AI updates on arXiv.org http://ift.tt/1WO6HV5
via IFTTT
Strategic Attentive Writer for Learning Macro-Actions. (arXiv:1606.04695v1 [cs.AI])
We present a novel deep recurrent neural network architecture that learns to build implicit plans in an end-to-end manner by purely interacting with an environment in reinforcement learning setting. The network builds an internal plan, which is continuously updated upon observation of the next input from the environment. It can also partition this internal representation into contiguous sub- sequences by learning for how long the plan can be committed to - i.e. followed without re-planing. Combining these properties, the proposed model, dubbed STRategic Attentive Writer (STRAW) can learn high-level, temporally abstracted macro- actions of varying lengths that are solely learnt from data without any prior information. These macro-actions enable both structured exploration and economic computation. We experimentally demonstrate that STRAW delivers strong improvements on several ATARI games by employing temporally extended planning strategies (e.g. Ms. Pacman and Frostbite). It is at the same time a general algorithm that can be applied on any sequence data. To that end, we also show that when trained on text prediction task, STRAW naturally predicts frequent n-grams (instead of macro-actions), demonstrating the generality of the approach.
from cs.AI updates on arXiv.org http://ift.tt/1rqVvzZ
via IFTTT
Safe Exploration in Finite Markov Decision Processes with Gaussian Processes. (arXiv:1606.04753v1 [cs.LG])
In classical reinforcement learning, when exploring an environment, agents accept arbitrary short term loss for long term gain. This is infeasible for safety critical applications, such as robotics, where even a single unsafe action may cause system failure. In this paper, we address the problem of safely exploring finite Markov decision processes (MDP). We define safety in terms of an, a priori unknown, safety constraint that depends on states and actions. We aim to explore the MDP under this constraint, assuming that the unknown function satisfies regularity conditions expressed via a Gaussian process prior. We develop a novel algorithm for this task and prove that it is able to completely explore the safely reachable part of the MDP without violating the safety constraint. To achieve this, it cautiously explores safe states and actions in order to gain statistical confidence about the safety of unvisited state-action pairs from noisy observations collected while navigating the environment. Moreover, the algorithm explicitly considers reachability when exploring the MDP, ensuring that it does not get stuck in any state with no safe way out. We demonstrate our method on digital terrain models for the task of exploring an unknown map with a rover.
from cs.AI updates on arXiv.org http://ift.tt/1WO6Pnu
via IFTTT
Category theoretic foundation of single-photon-based decision making. (arXiv:1602.08199v2 [physics.optics] UPDATED)
Decision making is a vital function in the age of machine learning and artificial intelligence; however, its physical realizations and their theoretical fundamentals are not yet known. In our former study, we demonstrated that single photons can be used to make decisions in uncertain, dynamically changing environments. The multi-armed bandit problem was successfully solved using the dual probabilistic and particle attributes of single photons. Herein, we revolutionize how decision making is comprehended via a category theoretic viewpoint; we present the category theoretic foundation of the single-photon-based decision making, including quantitative analysis that agrees well with the experimental results. The category theoretic model unveils complex interdependencies of the entities of the subject matter in the most simplified manner, including a dynamically changing environment. In particular, the octahedral structure and the braid structure in triangulated categories provide clear understandings and quantitative metrics of the underlying mechanisms for the single-photon decision maker. This is the first demonstration of a category theoretic interpretation of decision making, and provides a solid understanding and a design fundamental for machine learning and artificial intelligence.
from cs.AI updates on arXiv.org http://ift.tt/1oIavbK
via IFTTT
Ravens: Elvis Dumervil underwent offseason foot surgery, expects to be ready at some point during training camp (ESPN)
via IFTTT
MLB: Steven Wright (AL-best 2.09 ERA) takes the mound for the Red Sox against the Orioles; watch live in the ESPN App (ESPN)
via IFTTT
MLB: Steven Wright (AL-best 2.09 ERA) takes the mound for the Red Sox against the Orioles; watch live in the ESPN App (ESPN)
via IFTTT
Anonymous Safety Hotline
from Google Alert - anonymous http://ift.tt/1Puy0Mx
via IFTTT
build failure: error: use of deleted function '{anonymous}::Block::Block(const {anonymous}::Block&)'
from Google Alert - anonymous http://ift.tt/1Pur0za
via IFTTT
I have a new follower on Twitter
Delayla Uilkinson
Meow! Let's have some fun? I'm here (my login same in tw) - https://t.co/v0hlMvJryA
Following: 800 - Followers: 4
June 15, 2016 at 03:30PM via Twitter http://twitter.com/pankratiigolub1
How to Hack Someones Facebook Account Just by Knowing their Phone Numbers
from The Hacker News http://ift.tt/24R8U1u
via IFTTT
Orioles: Chris Davis doles out his class superlatives for the roster, including naming Darren O'Day the class clown (ESPN)
via IFTTT
[FD] Microsoft Visio multiple DLL side loading vulnerabilities
Source: Gmail -> IFTTT-> Blogger
help enable accesspress anonymous post through smartphone
from Google Alert - anonymous http://ift.tt/23ajam4
via IFTTT
Anonymous Donor Creates Women's Giving Initiative Challenge
from Google Alert - anonymous http://ift.tt/28EIO6A
via IFTTT
[FD] Siklu EtherHaul Hidden ‘root’ Account
Source: Gmail -> IFTTT-> Blogger
[FD] CVE-2016-3642 - Java Deserialization in Solarwinds Virtualization Manager 6.3.1
Source: Gmail -> IFTTT-> Blogger
Is it suitable for anonymous users notifications
from Google Alert - anonymous http://ift.tt/1UjBHsB
via IFTTT
[FD] CVE-2016-3643 - Misconfiguration of sudo in Solarwinds Virtualization Manager
Source: Gmail -> IFTTT-> Blogger
[FD] CVE-2016-3643 - Misconfiguration of sudo in Solarwinds Virtualization Manager
Source: Gmail -> IFTTT-> Blogger
[FD] Java Deserialization in Solarwinds Virtualization Manager 6.3.1
Source: Gmail -> IFTTT-> Blogger
US charges Chinese ex-IBM employee with Espionage
from The Hacker News http://ift.tt/28EcBw6
via IFTTT
ISS Daily Summary Report – 06/14/16
from ISS On-Orbit Status Report http://ift.tt/1Q40vpV
via IFTTT
Android Ransomware now targets your Smart TV, Too!
from The Hacker News http://ift.tt/1ZQhk7W
via IFTTT
[FD] FortiManager & FortiAnalyzer - (filename) Persistent Web Vulnerability
Source: Gmail -> IFTTT-> Blogger
<" |
materials manager
from Google Alert - anonymous http://ift.tt/1VYFwWt
via IFTTT
Microsoft releases tons of Security Updates to patch 44 vulnerabilities
from The Hacker News http://ift.tt/1S4Wn2Y
via IFTTT
Marijuana Anonymous
from Google Alert - anonymous http://ift.tt/2392R90
via IFTTT
I have a new follower on Twitter
Sexy Data Science
When data is easy & sexy #datascience #datasexy
Los Angeles, CA
https://t.co/Xt7eN247gH
Following: 2406 - Followers: 1914
June 15, 2016 at 12:32AM via Twitter http://twitter.com/sexydatascience
The North America and Pelican Nebulas
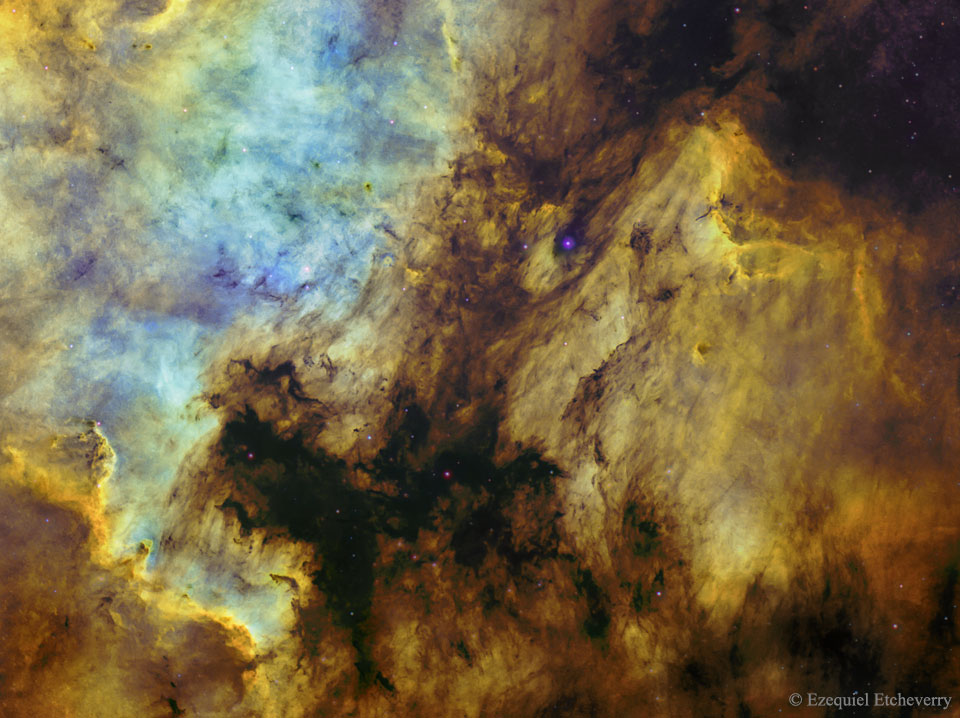
Tuesday, June 14, 2016
Orioles Video: Manny Machado takes David Price deep for his 17th HR of the season, plates 2 runs in 3-2 win over Red Sox (ESPN)
via IFTTT
Micro-interventions in urban transport from pattern discovery on the flow of passengers and on the bus network. (arXiv:1606.04190v1 [cs.AI])
In this paper, we describe a case study in a big metropolis, in which from data collected by digital sensors, we tried to understand mobility patterns of persons using buses and how this can generate knowledge to suggest interventions that are applied incrementally into the transportation network in use. We have first estimated an Origin-Destination matrix of buses users from datasets about the ticket validation and GPS positioning of buses. Then we represent the supply of buses with their routes through bus stops as a complex network, which allowed us to understand the bottlenecks of the current scenario and, in particular, applying community discovery techniques, to identify clusters that the service supply infrastructure has. Finally, from the superimposing of the flow of people represented in the OriginDestination matrix in the supply network, we exemplify how micro-interventions can be prospected by means of an example of the introduction of express routes.
from cs.AI updates on arXiv.org http://ift.tt/261d8WF
via IFTTT
Spreadsheet Probabilistic Programming. (arXiv:1606.04216v1 [cs.AI])
Spreadsheet workbook contents are simple programs. Because of this, probabilistic programming techniques can be used to perform Bayesian inversion of spreadsheet computations. What is more, existing execution engines in spreadsheet applications such as Microsoft Excel can be made to do this using only built-in functionality. We demonstrate this by developing a native Excel implementation of both a particle Markov Chain Monte Carlo variant and black-box variational inference for spreadsheet probabilistic programming. The resulting engine performs probabilistically coherent inference over spreadsheet computations, notably including spreadsheets that include user-defined black-box functions. Spreadsheet engines that choose to integrate the functionality we describe in this paper will give their users the ability to both easily develop probabilistic models and maintain them over time by including actuals via a simple user-interface mechanism. For spreadsheet end-users this would mean having access to efficient and probabilistically coherent probabilistic modeling and inference for use in all kinds of decision making under uncertainty.
from cs.AI updates on arXiv.org http://ift.tt/1tpCr6t
via IFTTT
Experimental and causal view on information integration in autonomous agents. (arXiv:1606.04250v1 [cs.AI])
The amount of digitally available but heterogeneous information about the world is remarkable, and new technologies such as self-driving cars, smart homes or the "internet of things" will further increase it. In this paper we examine certain aspects of the problem of how such heterogeneous information can be harnessed by intelligent agents. We first discuss potentials and limitations of some existing approaches, followed by two investigations. The focus of the first investigation is on using the novel experimentation platform {\em Malmo} to obtain a better understanding of the problem. The focus of the second investigation is on understanding how information about the hardware of different agents (such as self-driving cars), the agents' sensory data, and physical or causal information can be utilized for knowledge transfer between agents and subsequent more data-efficient decision making. Finally, we present some thoughts on what a general theory for the problem could look like, and formulate open questions.
from cs.AI updates on arXiv.org http://ift.tt/1VYei2h
via IFTTT
Context Trees: Augmenting Geospatial Trajectories with Context. (arXiv:1606.04269v1 [cs.AI])
Exposing latent knowledge in geospatial trajectories has the potential to provide a better understanding of the movements of individuals and groups. Motivated by such a desire, this work presents the context tree, a new hierarchical data structure that summarises the context behind user actions in a single model. We propose a method for context tree construction that augments geospatial trajectories with land usage data to identify such contexts. Through evaluation of the construction method and analysis of the properties of generated context trees, we demonstrate the foundation for understanding and modelling behaviour afforded. Summarising user contexts into a single data structure gives easy access to information that would otherwise remain latent, providing the basis for better understanding and predicting the actions and behaviours of individuals and groups. Finally, we also present a method for pruning context trees, for use in applications where it is desirable to reduce the size of the tree while retaining useful information.
from cs.AI updates on arXiv.org http://ift.tt/1tpCRtw
via IFTTT
Entropy/IP: Uncovering Structure in IPv6 Addresses. (arXiv:1606.04327v1 [cs.NI])
In this paper, we introduce Entropy/IP: a system that discovers Internet address structure based on analyses of a subset of IPv6 addresses known to be active, i.e., training data, gleaned by readily available passive and active means. The system is completely automated and employs a combination of information-theoretic and machine learning techniques to probabilistically model IPv6 addresses. We present results showing that our system is effective in exposing structural characteristics of portions of the IPv6 Internet address space populated by active client, service, and router addresses.
In addition to visualizing the address structure for exploration, the system uses its models to generate candidate target addresses for scanning. For each of 15 evaluated datasets, we train on 1K addresses and generate 1M candidates for scanning. We achieve some success in 14 datasets, finding up to 40% of the generated addresses to be active. In 11 of these datasets, we find active network identifiers (e.g., /64 prefixes or `subnets') not seen in training. Thus, we provide the first evidence that it is practical to discover subnets and hosts by scanning probabilistically selected areas of the IPv6 address space not known to contain active hosts a priori.
from cs.AI updates on arXiv.org http://ift.tt/1VYfGlw
via IFTTT
Digits that are not: Generating new types through deep neural nets. (arXiv:1606.04345v1 [cs.AI])
For an artificial creative agent, an essential driver of the search for novelty is a value function which is often provided by the system designer or users. We argue that an important barrier for progress in creativity research is the inability of these systems to develop their own notion of value for novelty. We propose a notion of knowledge-driven creativity that circumvent the need for an externally imposed value function, allowing the system to explore based on what it has learned from a set of referential objects. The concept is illustrated by a specific knowledge model provided by a deep generative autoencoder. Using the described system, we train a knowledge model on a set of digit images and we use the same model to build coherent sets of new digits that do not belong to known digit types.
from cs.AI updates on arXiv.org http://ift.tt/1tpCC1y
via IFTTT
Relating Strong Spatial Cognition to Symbolic Problem Solving --- An Example. (arXiv:1606.04397v1 [cs.AI])
In this note, we discuss and analyse a shortest path finding approach using strong spatial cognition. It is compared with a symbolic graph-based algorithm and it is shown that both approaches are similar with respect to structure and complexity. Nevertheless, the strong spatial cognition solution is easy to understand and even pops up immediately when one has to solve the problem.
from cs.AI updates on arXiv.org http://ift.tt/1VYfGSF
via IFTTT
The Parallel Knowledge Gradient Method for Batch Bayesian Optimization. (arXiv:1606.04414v1 [stat.ML])
In many applications of black-box optimization, one can evaluate multiple points simultaneously, e.g. when evaluating the performances of several different neural network architectures in a parallel computing environment. In this paper, we develop a novel batch Bayesian optimization algorithm --- the parallel knowledge gradient method. By construction, this method provides the one-step Bayes optimal batch of points to sample. We provide an efficient strategy for computing this Bayes-optimal batch of points, and we demonstrate that the parallel knowledge gradient method finds global optima significantly faster than previous batch Bayesian optimization algorithms on both synthetic test functions and when tuning hyperparameters of practical machine learning algorithms, especially when function evaluations are noisy.
from cs.AI updates on arXiv.org http://ift.tt/1UyfjsU
via IFTTT
Logic Tensor Networks: Deep Learning and Logical Reasoning from Data and Knowledge. (arXiv:1606.04422v1 [cs.AI])
We propose real logic: a uniform framework for integrating automatic learning and reasoning. Real logic is defined on a full first-order language where formulas have truth-value in the interval [0,1] and semantics defined concretely on the domain of real numbers. Logical constants are interpreted as (feature) vectors of real numbers. Real logic promotes a well-founded integration of deductive reasoning on knowledge-bases with efficient, data-driven relational machine learning. We show how Real Logic can be implemented in deep Tensor Neural Networks with the use of Google's TensorFlow primitives. The paper concludes with experiments on a simple but representative example of knowledge completion.
from cs.AI updates on arXiv.org http://ift.tt/1YpYmpM
via IFTTT
DeepMath - Deep Sequence Models for Premise Selection. (arXiv:1606.04442v1 [cs.AI])
We study the effectiveness of neural sequence models for premise selection in automated theorem proving, one of the main bottlenecks in the formalization of mathematics. We propose a two stage approach for this task that yields good results for the premise selection task on the Mizar corpus while avoiding the hand-engineered features of existing state-of-the-art models. To our knowledge, this is the first time deep learning has been applied to theorem proving.
from cs.AI updates on arXiv.org http://ift.tt/1tpCT4B
via IFTTT
Lifted Convex Quadratic Programming. (arXiv:1606.04486v1 [cs.AI])
Symmetry is the essential element of lifted inference that has recently demon- strated the possibility to perform very efficient inference in highly-connected, but symmetric probabilistic models models. This raises the question, whether this holds for optimisation problems in general. Here we show that for a large class of optimisation methods this is actually the case. More precisely, we introduce the concept of fractional symmetries of convex quadratic programs (QPs), which lie at the heart of many machine learning approaches, and exploit it to lift, i.e., to compress QPs. These lifted QPs can then be tackled with the usual optimization toolbox (off-the-shelf solvers, cutting plane algorithms, stochastic gradients etc.). If the original QP exhibits symmetry, then the lifted one will generally be more compact, and hence their optimization is likely to be more efficient.
from cs.AI updates on arXiv.org http://ift.tt/1tpD4gc
via IFTTT
Why is Compiling Lifted Inference into a Low-Level Language so Effective?. (arXiv:1606.04512v1 [cs.AI])
First-order knowledge compilation techniques have proven efficient for lifted inference. They compile a relational probability model into a target circuit on which many inference queries can be answered efficiently. Early methods used data structures as their target circuit. In our KR-2016 paper, we showed that compiling to a low-level program instead of a data structure offers orders of magnitude speedup, resulting in the state-of-the-art lifted inference technique. In this paper, we conduct experiments to address two questions regarding our KR-2016 results: 1- does the speedup come from more efficient compilation or more efficient reasoning with the target circuit?, and 2- why are low-level programs more efficient target circuits than data structures?
from cs.AI updates on arXiv.org http://ift.tt/1VYeWwO
via IFTTT
Sparsely Connected and Disjointly Trained Deep Neural Networks for Low Resource Behavioral Annotation: Acoustic Classification in Couples' Therapy. (arXiv:1606.04518v1 [cs.LG])
Observational studies are based on accurate assessment of human state. A behavior recognition system that models interlocutors' state in real-time can significantly aid the mental health domain. However, behavior recognition from speech remains a challenging task since it is difficult to find generalizable and representative features because of noisy and high-dimensional data, especially when data is limited and annotated coarsely and subjectively. Deep Neural Networks (DNN) have shown promise in a wide range of machine learning tasks, but for Behavioral Signal Processing (BSP) tasks their application has been constrained due to limited quantity of data. We propose a Sparsely-Connected and Disjointly-Trained DNN (SD-DNN) framework to deal with limited data. First, we break the acoustic feature set into subsets and train multiple distinct classifiers. Then, the hidden layers of these classifiers become parts of a deeper network that integrates all feature streams. The overall system allows for full connectivity while limiting the number of parameters trained at any time and allows convergence possible with even limited data. We present results on multiple behavior codes in the couples' therapy domain and demonstrate the benefits in behavior classification accuracy. We also show the viability of this system towards live behavior annotations.
from cs.AI updates on arXiv.org http://ift.tt/1tpCorj
via IFTTT
Dissociation and Propagation for Approximate Lifted Inference with Standard Relational Database Management Systems. (arXiv:1310.6257v4 [cs.DB] UPDATED)
Probabilistic inference over large data sets is a challenging data management problem since exact inference is generally #P-hard and is most often solved approximately with sampling-based methods today. This paper proposes an alternative approach for approximate evaluation of conjunctive queries with standard relational databases: In our approach, every query is evaluated entirely in the database engine by evaluating a fixed number of query plans, each providing an upper bound on the true probability, then taking their minimum. We provide an algorithm that takes into account important schema information to enumerate only the minimal necessary plans among all possible plans. Importantly, this algorithm is a strict generalization of all known PTIME self-join-free conjunctive queries: A query is in PTIME if and only if our algorithm returns one single plan. Furthermore, our approach is a generalization of a family of efficient ranking methods from graphs to hypergraphs. We also adapt three relational query optimization techniques to evaluate all necessary plans very fast. We give a detailed experimental evaluation of our approach and, in the process, provide a new way of thinking about the value of probabilistic methods over non-probabilistic methods for ranking query answers. We also note that the techniques developed in this paper apply immediately to lifted inference from statistical relational models since lifted inference corresponds to PTIME plans in probabilistic databases.
from cs.AI updates on arXiv.org http://ift.tt/18MQRQf
via IFTTT
Automatic learning of gait signatures for people identification. (arXiv:1603.01006v2 [cs.CV] UPDATED)
This work targets people identification in video based on the way they walk (i.e. gait). While classical methods typically derive gait signatures from sequences of binary silhouettes, in this work we explore the use of convolutional neural networks (CNN) for learning high-level descriptors from low-level motion features (i.e. optical flow components). We carry out a thorough experimental evaluation of the proposed CNN architecture on the challenging TUM-GAID dataset. The experimental results indicate that using spatio-temporal cuboids of optical flow as input data for CNN allows to obtain state-of-the-art results on the gait task with an image resolution eight times lower than the previously reported results (i.e. 80x60 pixels).
from cs.AI updates on arXiv.org http://ift.tt/1QWDHZm
via IFTTT