Source: Gmail -> IFTTT-> Blogger
Latest YouTube Video
Saturday, April 9, 2016
[FD] end of useable crypto in browsers?
Source: Gmail -> IFTTT-> Blogger
Lese bok Last ned Archives of Philosophy, Issues 1-14 av Anonymous på norsk id:brwpu0i ap45
from Google Alert - anonymous http://ift.tt/1VHtYG7
via IFTTT
No Password Required! 135 Million Modems Open to Remote Factory Reset
from The Hacker News http://ift.tt/1qDcT59
via IFTTT
Previewing a node as an anonymous user results in a page not found error
from Google Alert - anonymous http://ift.tt/1UPOMfC
via IFTTT
WordPress enables Free HTTPS Encryption for all Blogs with Custom Domain
from The Hacker News http://ift.tt/1qCNus6
via IFTTT
anonymous aceh 2
from Google Alert - anonymous http://ift.tt/1UPoGcJ
via IFTTT
Lese bok Last ned Cinderella av Anonymous på norsk id:7scd46u ap45
from Google Alert - anonymous http://ift.tt/20ovSMx
via IFTTT
[FD] Express Zip <= 2.40 Path Traversal
Source: Gmail -> IFTTT-> Blogger
[FD] [CVE-2016-3971]DotCMS xss vulnerability
Source: Gmail -> IFTTT-> Blogger
[FD] [CVE-2016-3972]DotCMS Directory traversal vulnerability
Source: Gmail -> IFTTT-> Blogger
Lapland Northern Lights
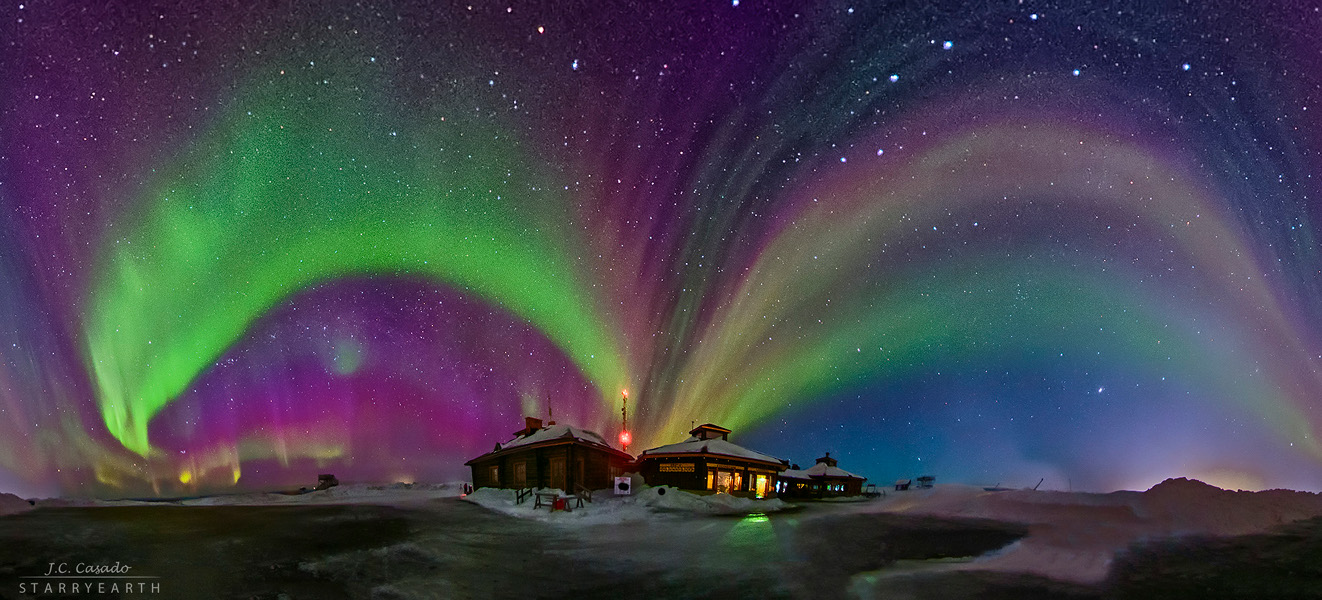
Orioles Video: Jonathan Schoop, Nolan Reimold and Manny Machado all blast homers in the 5th inning of 6-1 win over Rays (ESPN)
via IFTTT
Friday, April 8, 2016
Orioles: CF Adam Jones (sore ribcage) not in Friday's lineup vs. Rays for 2nd straight game; SS J.J. Hardy not starting (ESPN)
via IFTTT
Orioles: CF Adam Jones (sore ribcage) not in Friday's lineup vs. Rays for 2nd straight game; SS J.J. Hardy not starting (ESPN)
via IFTTT
Anonymous Ghost
from Google Alert - anonymous http://ift.tt/1S7HYK4
via IFTTT
[FD] WP Multiple Meta Box v1.0 - SQL Injection Vulnerability
Source: Gmail -> IFTTT-> Blogger
[FD] AccelSite Content Manager v1.0 - SQL Injection Vulnerability
Source: Gmail -> IFTTT-> Blogger
ISS Daily Summary Report – 04/7/16
from ISS On-Orbit Status Report http://ift.tt/1qbwj02
via IFTTT
Google may adopt Apple's Swift Programming Language for Android
from The Hacker News http://ift.tt/1SiLefw
via IFTTT
Lese bok Last ned Deutsches Hausbuch. 1. Band av Anonymous på norsk id:mhx5x50 ap45
from Google Alert - anonymous http://ift.tt/1MjtVP6
via IFTTT
Anonymous Ghost
from Google Alert - anonymous http://ift.tt/1RI1jzo
via IFTTT
Wolf-Lundmark-Melotte
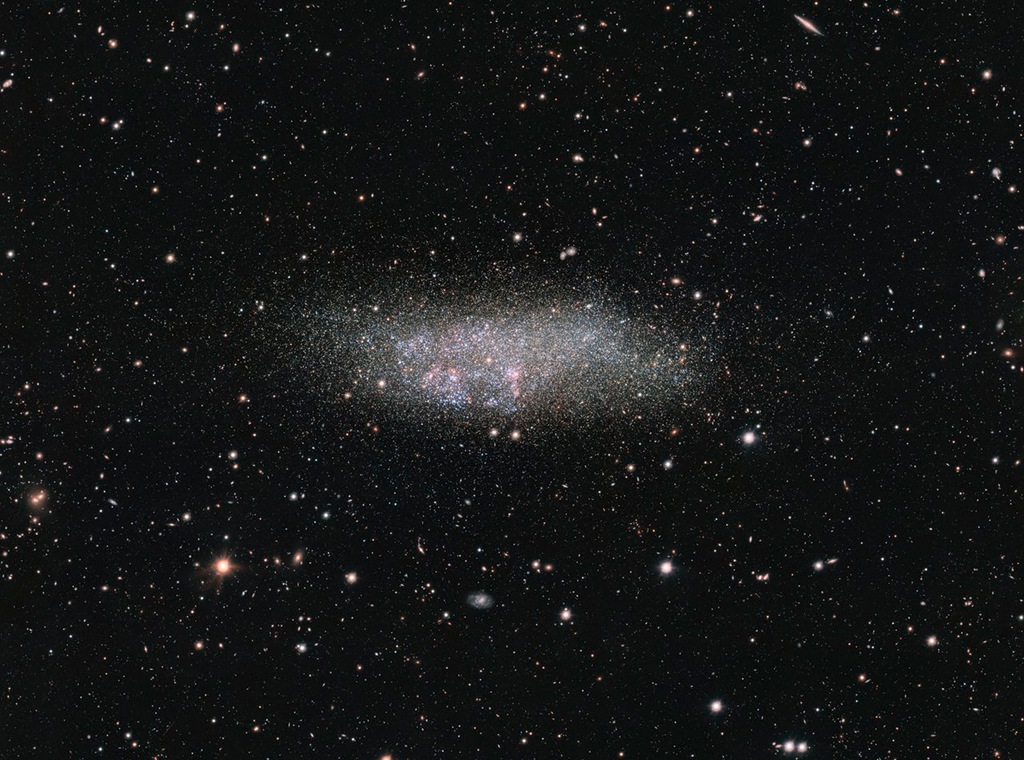
Thursday, April 7, 2016
Anonymous users see "An unrecoverable error occurred..."
from Google Alert - anonymous http://ift.tt/23nkDG6
via IFTTT
Orioles Video: Joey Rickard smashes first career HR in 4-2 victory over Twins that completes 3-game sweep (ESPN)
via IFTTT
Swingers Anonymous
from Google Alert - anonymous http://ift.tt/1V0GbHm
via IFTTT
Safe Probability. (arXiv:1604.01785v1 [stat.ME])
We formalize the idea of probability distributions that lead to reliable predictions about some, but not all aspects of a domain. The resulting notion of `safety' provides a fresh perspective on foundational issues in statistics, providing a middle ground between imprecise probability and multiple-prior models on the one hand and strictly Bayesian approaches on the other. It also allows us to formalize fiducial distributions in terms of the set of random variables that they can safely predict, thus taking some of the sting out of the fiducial idea. By restricting probabilistic inference to safe uses, one also automatically avoids paradoxes such as the Monty Hall problem. Safety comes in a variety of degrees, such as "validity" (the strongest notion), "calibration", "confidence safety" and "unbiasedness" (almost the weakest notion).
from cs.AI updates on arXiv.org http://ift.tt/1WgiaLI
via IFTTT
Learning to Track at 100 FPS with Deep Regression Networks. (arXiv:1604.01802v1 [cs.CV])
Machine learning techniques are often used in computer vision due to their ability to leverage large amounts of training data to improve performance. Unfortunately, most generic object trackers are still trained from scratch online and do not benefit from the large number of videos that are readily available for offline training. We propose a method for using neural networks to track generic objects in a way that allows them to improve performance by training on labeled videos. Previous attempts to use neural networks for tracking are very slow to run and not practical for real-time applications. In contrast, our tracker uses a simple feed-forward network with no online training required, allowing our tracker to run at 100 fps during test time. Our tracker trains from both labeled video as well as a large collection of images, which helps prevent overfitting. The tracker learns generic object motion and can be used to track novel objects that do not appear in the training set. We test our network on a standard tracking benchmark to demonstrate our tracker's state-of-the-art performance. Our network learns to track generic objects in real-time as they move throughout the world.
from cs.AI updates on arXiv.org http://ift.tt/1XkoPmu
via IFTTT
Planning with Information-Processing Constraints and Model Uncertainty in Markov Decision Processes. (arXiv:1604.02080v1 [cs.AI])
Information-theoretic principles for learning and acting have been proposed to solve particular classes of Markov Decision Problems. Mathematically, such approaches are governed by a variational free energy principle and allow solving MDP planning problems with information-processing constraints expressed in terms of a Kullback-Leibler divergence with respect to a reference distribution. Here we consider a generalization of such MDP planners by taking model uncertainty into account. As model uncertainty can also be formalized as an information-processing constraint, we can derive a unified solution from a single generalized variational principle. We provide a generalized value iteration scheme together with a convergence proof. As limit cases, this generalized scheme includes standard value iteration with a known model, Bayesian MDP planning, and robust planning. We demonstrate the benefits of this approach in a grid world simulation.
from cs.AI updates on arXiv.org http://ift.tt/1VcmpIF
via IFTTT
On Stochastic Belief Revision and Update and their Combination. (arXiv:1604.02126v1 [cs.AI])
I propose a framework for an agent to change its probabilistic beliefs when a new piece of propositional information $\alpha$ is observed. Traditionally, belief change occurs by either a revision process or by an update process, depending on whether the agent is informed with $\alpha$ in a static world or, respectively, whether $\alpha$ is a 'signal' from the environment due to an event occurring. Boutilier suggested a unified model of qualitative belief change, which "combines aspects of revision and update, providing a more realistic characterization of belief change." In this paper, I propose a unified model of quantitative belief change, where an agent's beliefs are represented as a probability distribution over possible worlds. As does Boutilier, I take a dynamical systems perspective. The proposed approach is evaluated against several rationality postulated, and some properties of the approach are worked out.
from cs.AI updates on arXiv.org http://ift.tt/1XkoRun
via IFTTT
Revising Incompletely Specified Convex Probabilistic Belief Bases. (arXiv:1604.02133v1 [cs.AI])
We propose a method for an agent to revise its incomplete probabilistic beliefs when a new piece of propositional information is observed. In this work, an agent's beliefs are represented by a set of probabilistic formulae -- a belief base. The method involves determining a representative set of 'boundary' probability distributions consistent with the current belief base, revising each of these probability distributions and then translating the revised information into a new belief base. We use a version of Lewis Imaging as the revision operation. The correctness of the approach is proved. The expressivity of the belief bases under consideration are rather restricted, but has some applications. We also discuss methods of belief base revision employing the notion of optimum entropy, and point out some of the benefits and difficulties in those methods. Both the boundary distribution method and the optimum entropy method are reasonable, yet yield different results.
from cs.AI updates on arXiv.org http://ift.tt/1Vcmps2
via IFTTT
A New Oscillating-Error Technique for Classifiers. (arXiv:1505.05312v4 [cs.AI] UPDATED)
This paper describes a new method for reducing the error in a classifier. It uses an error correction update, but includes the very simple rule of either adding or subtracting the adjustment, based on whether the variable value is currently larger or smaller than the desired value. The new neuron can take an input from each variable or column and adjust it by either adding or subtracting the difference, on a variable by variable basis. While a traditional neuron would sum the inputs together and then apply the same function in every instance, this new neuron can change the function for each input variable. This gives added flexibility to the convergence procedure, where through a series of transpositions, variables that are far away can continue towards the desired value, whereas variables that are originally much closer can oscillate from one side to the other. Tests show that the method can successfully classify some benchmark datasets. It can also work in a batch mode, with reduced training times and can be used as part of a neural network architecture. There are also some updates to an earlier wave shape paper.
from cs.AI updates on arXiv.org http://ift.tt/1Bd2M5e
via IFTTT
Building End-To-End Dialogue Systems Using Generative Hierarchical Neural Network Models. (arXiv:1507.04808v3 [cs.CL] UPDATED)
We investigate the task of building open domain, conversational dialogue systems based on large dialogue corpora using generative models. Generative models produce system responses that are autonomously generated word-by-word, opening up the possibility for realistic, flexible interactions. In support of this goal, we extend the recently proposed hierarchical recurrent encoder-decoder neural network to the dialogue domain, and demonstrate that this model is competitive with state-of-the-art neural language models and back-off n-gram models. We investigate the limitations of this and similar approaches, and show how its performance can be improved by bootstrapping the learning from a larger question-answer pair corpus and from pretrained word embeddings.
from cs.AI updates on arXiv.org http://ift.tt/1ea3dHX
via IFTTT
Orioles: OF Adam Jones (ribcage soreness) not in starting lineup Thursday vs. Twins; felt pain after final AB Wednesday (ESPN)
via IFTTT
Ravens open preseason at home vs. Panthers, visit Colts, host Lions and play at Saints to conclude exhibition slate (ESPN)
via IFTTT
Anonymous plans to create new political party in the US, called
from Google Alert - anonymous http://ift.tt/1S6ab3Q
via IFTTT
A Grateful Message To An Anonymous Donor
from Google Alert - anonymous http://ift.tt/1SDtsUy
via IFTTT
Anonymous users cant access webform
from Google Alert - anonymous http://ift.tt/1S6aaNu
via IFTTT
Ravens: RB Terrance West suing a South Florida hotel group where he claims he was robbed twice on the same day in Feb. (ESPN)
via IFTTT
FBI claims its iPhone Hacking Tool can't Unlock iPhone 5S, 6S and 6S Plus
from The Hacker News http://ift.tt/1qwHlxO
via IFTTT
[FD] Monsta Box WebFTP 1.8.2 and below arbitrary file read and path traversal vulnerabilities
Source: Gmail -> IFTTT-> Blogger
How to Run Ubuntu on latest Windows 10 Insider Preview Build 14316
from The Hacker News http://ift.tt/1oEB7Kl
via IFTTT
[FD] Eight Webcom CMS (2016 Q2) - SQL Injection Vulnerability
Source: Gmail -> IFTTT-> Blogger
[FD] Quicksilver HQ VoHo Concept4E CMS v1.0 - Multiple SQL Injection Web Vulnerabilities
Source: Gmail -> IFTTT-> Blogger
[FD] Virtual Freer v1.58 - Client Side Cross Site Scripting Vulnerability
Source: Gmail -> IFTTT-> Blogger
[FD] Techsoft WS CMS (2016 Q2) - SQL Injection Web Vulnerability
Source: Gmail -> IFTTT-> Blogger
'Hacking Team' Loses License to Sell Surveillance Malware Outside Europe
from The Hacker News http://ift.tt/1MTW9A9
via IFTTT
Anonymous Cris
from Google Alert - anonymous http://ift.tt/1SRF88Y
via IFTTT
Auroras and the Magnetosphere of Jupiter
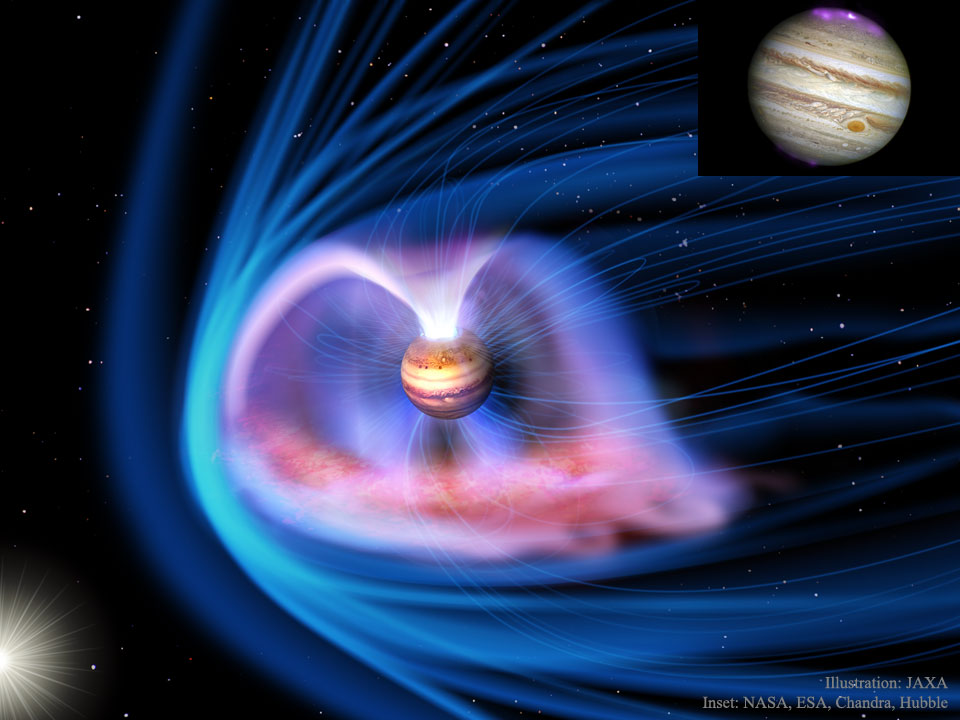
Wednesday, April 6, 2016
Towards Bayesian Deep Learning: A Survey. (arXiv:1604.01662v1 [stat.ML])
While perception tasks such as visual object recognition and text understanding play an important role in human intelligence, the subsequent tasks that involve inference, reasoning and planning require an even higher level of intelligence. The past few years have seen major advances in many perception tasks using deep learning models. For higher-level inference, however, probabilistic graphical models with their Bayesian nature are still more powerful and flexible. To achieve integrated intelligence that involves both perception and inference, it is naturally desirable to tightly integrate deep learning and Bayesian models within a principled probabilistic framework, which we call Bayesian deep learning. In this unified framework, the perception of text or images using deep learning can boost the performance of higher-level inference and in return, the feedback from the inference process is able to enhance the perception of text or images. This survey provides a general introduction to Bayesian deep learning and reviews its recent applications on recommender systems, topic models, and control. In this survey, we also discuss the relationship and differences between Bayesian deep learning and other related topics like Bayesian treatment of neural networks.
from cs.AI updates on arXiv.org http://ift.tt/1SRcEw3
via IFTTT
On the uniform one-dimensional fragment. (arXiv:1604.01673v1 [cs.LO])
The uniform one-dimensional fragment of first-order logic, U1, is a recently introduced formalism that extends two-variable logic in a natural way to contexts with relations of all arities. We survey properties of U1 and investigate its relationship to description logics designed to accommodate higher arity relations, with particular attention given to DLR_reg. We also define a description logic version of a variant of U1 and prove a range of new results concerning the expressivity of U1 and related logics.
from cs.AI updates on arXiv.org http://ift.tt/1VaiqMy
via IFTTT
A Corpus and Evaluation Framework for Deeper Understanding of Commonsense Stories. (arXiv:1604.01696v1 [cs.CL])
Representation and learning of commonsense knowledge is one of the foundational problems in the quest to enable deep language understanding. This issue is particularly challenging for understanding casual and correlational relationships between events. While this topic has received a lot of interest in the NLP community, research has been hindered by the lack of a proper evaluation framework. This paper attempts to address this problem with a new framework for evaluating story understanding and script learning: the 'Story Cloze Test'. This test requires a system to choose the correct ending to a four-sentence story. We created a new corpus of ~50k five-sentence commonsense stories, ROCStories, to enable this evaluation. This corpus is unique in two ways: (1) it captures a rich set of causal and temporal commonsense relations between daily events, and (2) it is a high quality collection of everyday life stories that can also be used for story generation. Experimental evaluation shows that a host of baselines and state-of-the-art models based on shallow language understanding struggle to achieve a high score on the Story Cloze Test. We discuss these implications for script and story learning, and offer suggestions for deeper language understanding.
from cs.AI updates on arXiv.org http://ift.tt/1SRcCEg
via IFTTT
Efficiency and Sequenceability in Fair Division of Indivisible Goods with Additive Preferences. (arXiv:1604.01734v1 [cs.GT])
In fair division of indivisible goods, using sequences of sincere choices (or picking sequences) is a natural way to allocate the objects. The idea is the following: at each stage, a designated agent picks one object among those that remain. This paper, restricted to the case where the agents have numerical additive preferences over objects, revisits to some extent the seminal paper by Brams and King [9] which was specific to ordinal and linear order preferences over items. We point out similarities and differences with this latter context. In particular, we show that any Pareto-optimal allocation (under additive preferences) is sequenceable, but that the converse is not true anymore. This asymmetry leads naturally to the definition of a "scale of efficiency" having three steps: Pareto-optimality, sequenceability without Pareto-optimality, and non-sequenceability. Finally, we investigate the links between these efficiency properties and the "scale of fairness" we have described in an earlier work [7]: we first show that an allocation can be envy-free and non-sequenceable, but that every competitive equilibrium with equal incomes is sequenceable. Then we experimentally explore the links between the scales of efficiency and fairness.
from cs.AI updates on arXiv.org http://ift.tt/1VaisUD
via IFTTT
ASlib: A Benchmark Library for Algorithm Selection. (arXiv:1506.02465v3 [cs.AI] UPDATED)
The task of algorithm selection involves choosing an algorithm from a set of algorithms on a per-instance basis in order to exploit the varying performance of algorithms over a set of instances. The algorithm selection problem is attracting increasing attention from researchers and practitioners in AI. Years of fruitful applications in a number of domains have resulted in a large amount of data, but the community lacks a standard format or repository for this data. This situation makes it difficult to share and compare different approaches effectively, as is done in other, more established fields. It also unnecessarily hinders new researchers who want to work in this area. To address this problem, we introduce a standardized format for representing algorithm selection scenarios and a repository that contains a growing number of data sets from the literature. Our format has been designed to be able to express a wide variety of different scenarios. Demonstrating the breadth and power of our platform, we describe a set of example experiments that build and evaluate algorithm selection models through a common interface. The results display the potential of algorithm selection to achieve significant performance improvements across a broad range of problems and algorithms.
from cs.AI updates on arXiv.org http://ift.tt/1cHsY1G
via IFTTT
Towards a Better Understanding of CAR, CDR, CADR and the Others. (arXiv:1507.05956v5 [cs.AI] UPDATED)
This paper describes the IBM 704 architecture and the genesis of the names for CAR, and CDR, which, as it turns out, probably don't quite make sense. The paper suggests that this may not be all bad, as the names lend themselves to compounding. Indeed that the compound function names , such as CADR, or even CADADR, etc. may be read as little access programs.
from cs.AI updates on arXiv.org http://ift.tt/1ehMF0O
via IFTTT
Learning in Auctions: Regret is Hard, Envy is Easy. (arXiv:1511.01411v6 [cs.GT] UPDATED)
A line of recent work provides welfare guarantees of simple combinatorial auction formats, such as selling m items via simultaneous second price auctions (SiSPAs) (Christodoulou et al. 2008, Bhawalkar and Roughgarden 2011, Feldman et al. 2013). These guarantees hold even when the auctions are repeatedly executed and players use no-regret learning algorithms. Unfortunately, off-the-shelf no-regret algorithms for these auctions are computationally inefficient as the number of actions is exponential. We show that this obstacle is insurmountable: there are no polynomial-time no-regret algorithms for SiSPAs, unless RP$\supseteq$ NP, even when the bidders are unit-demand. Our lower bound raises the question of how good outcomes polynomially-bounded bidders may discover in such auctions.
To answer this question, we propose a novel concept of learning in auctions, termed "no-envy learning." This notion is founded upon Walrasian equilibrium, and we show that it is both efficiently implementable and results in approximately optimal welfare, even when the bidders have fractionally subadditive (XOS) valuations (assuming demand oracles) or coverage valuations (without demand oracles). No-envy learning outcomes are a relaxation of no-regret outcomes, which maintain their approximate welfare optimality while endowing them with computational tractability. Our results extend to other auction formats that have been studied in the literature via the smoothness paradigm.
Our results for XOS valuations are enabled by a novel Follow-The-Perturbed-Leader algorithm for settings where the number of experts is infinite, and the payoff function of the learner is non-linear. This algorithm has applications outside of auction settings, such as in security games. Our result for coverage valuations is based on a novel use of convex rounding schemes and a reduction to online convex optimization.
from cs.AI updates on arXiv.org http://ift.tt/1MB08ux
via IFTTT
Recurrent Instance Segmentation. (arXiv:1511.08250v2 [cs.CV] UPDATED)
Instance segmentation is the problem of detecting and delineating each distinct object of interest appearing in an image. Current instance segmentation approaches consist of ensembles of modules that are trained independently of each other, thus missing learning opportunities. Here we propose a new instance segmentation paradigm consisting in an end-to-end method that learns how to segment instances sequentially. The model is based on a recurrent neural network that sequentially finds objects and their segmentations one at a time. This net is provided with a spatial memory that keeps track of what pixels have been explained and allows handling occlusion. In order to train the model we designed a new principled loss function that accurately represents the properties of the instance segmentation problem. In the experiments carried out, we found that our method outperforms recent approaches on multiple person segmentation, and all state of the art approaches on the Plant Phenotyping dataset for leaf counting.
from cs.AI updates on arXiv.org http://ift.tt/1TfqNCg
via IFTTT
Anonymous Soldiers: the struggle for Israel on Late Night Live
from Google Alert - anonymous http://ift.tt/1q7BOgb
via IFTTT
Ravens: Ohio State RB Ezekiel Elliott goes 6th in Mel Kiper's Mock Draft 4.0; \"an elite RB who can excel on all 3 downs\" (ESPN)
via IFTTT
User references can bypass anonymous restrictions
from Google Alert - anonymous http://ift.tt/1MSQhqL
via IFTTT
General Manager - Fast Food
from Google Alert - anonymous http://ift.tt/1UWvmq1
via IFTTT
[FD] Check out faraday v1.0.18! New CLI mode, Jira support & bug fixes!
Source: Gmail -> IFTTT-> Blogger
[FD] CVE-2016-3672 - Unlimiting the stack not longer disables ASLR
Source: Gmail -> IFTTT-> Blogger
[FD] hardwear.io CFP 2016 - Hardware Security Conference Call for Papers
Source: Gmail -> IFTTT-> Blogger
[FD] Fireware XTM Web UI - Open Redirect
Source: Gmail -> IFTTT-> Blogger
Beautiful/Anonymous - A Real Life Superhero
from Google Alert - anonymous http://ift.tt/1RE5c8t
via IFTTT
ISS Daily Summary Report – 04/5/16
from ISS On-Orbit Status Report http://ift.tt/1TBCBCa
via IFTTT
Adobe to issue Emergency Patch for Critical Flash Player Vulnerability
from The Hacker News http://ift.tt/1oBoaRB
via IFTTT
Efficiently combine anonymous functions?
from Google Alert - anonymous http://ift.tt/1SzWrsk
via IFTTT
Facebook uses Artificial Intelligence to Describe Photos to Blind Users
from The Hacker News http://ift.tt/1SPf91K
via IFTTT
Hacker reveals How to Bypass iPhone 6s Lock Screen Passcode [Video]
from The Hacker News http://ift.tt/1oB3itq
via IFTTT
Adult Video (you will remain anonymous)
from Google Alert - anonymous http://ift.tt/1V8tcTF
via IFTTT
Tuesday, April 5, 2016
An Efficient Algorithm for Mining Frequent Sequence with Constraint Programming. (arXiv:1604.01166v1 [cs.DB])
The main advantage of Constraint Programming (CP) approaches for sequential pattern mining (SPM) is their modularity, which includes the ability to add new constraints (regular expressions, length restrictions, etc). The current best CP approach for SPM uses a global constraint (module) that computes the projected database and enforces the minimum frequency; it does this with a filtering algorithm similar to the PrefixSpan method. However, the resulting system is not as scalable as some of the most advanced mining systems like Zaki's cSPADE. We show how, using techniques from both data mining and CP, one can use a generic constraint solver and yet outperform existing specialized systems. This is mainly due to two improvements in the module that computes the projected frequencies: first, computing the projected database can be sped up by pre-computing the positions at which an symbol can become unsupported by a sequence, thereby avoiding to scan the full sequence each time; and second by taking inspiration from the trailing used in CP solvers to devise a backtracking-aware data structure that allows fast incremental storing and restoring of the projected database. Detailed experiments show how this approach outperforms existing CP as well as specialized systems for SPM, and that the gain in efficiency translates directly into increased efficiency for other settings such as mining with regular expressions.
from cs.AI updates on arXiv.org http://ift.tt/1SOnDpV
via IFTTT
Learning to Generate Posters of Scientific Papers. (arXiv:1604.01219v1 [cs.AI])
Researchers often summarize their work in the form of posters. Posters provide a coherent and efficient way to convey core ideas from scientific papers. Generating a good scientific poster, however, is a complex and time consuming cognitive task, since such posters need to be readable, informative, and visually aesthetic. In this paper, for the first time, we study the challenging problem of learning to generate posters from scientific papers. To this end, a data-driven framework, that utilizes graphical models, is proposed. Specifically, given content to display, the key elements of a good poster, including panel layout and attributes of each panel, are learned and inferred from data. Then, given inferred layout and attributes, composition of graphical elements within each panel is synthesized. To learn and validate our model, we collect and make public a Poster-Paper dataset, which consists of scientific papers and corresponding posters with exhaustively labelled panels and attributes. Qualitative and quantitative results indicate the effectiveness of our approach.
from cs.AI updates on arXiv.org http://ift.tt/239GYdH
via IFTTT
Feature extraction using Latent Dirichlet Allocation and Neural Networks: A case study on movie synopses. (arXiv:1604.01272v1 [cs.CL])
Feature extraction has gained increasing attention in the field of machine learning, as in order to detect patterns, extract information, or predict future observations from big data, the urge of informative features is crucial. The process of extracting features is highly linked to dimensionality reduction as it implies the transformation of the data from a sparse high-dimensional space, to higher level meaningful abstractions. This dissertation employs Neural Networks for distributed paragraph representations, and Latent Dirichlet Allocation to capture higher level features of paragraph vectors. Although Neural Networks for distributed paragraph representations are considered the state of the art for extracting paragraph vectors, we show that a quick topic analysis model such as Latent Dirichlet Allocation can provide meaningful features too. We evaluate the two methods on the CMU Movie Summary Corpus, a collection of 25,203 movie plot summaries extracted from Wikipedia. Finally, for both approaches, we use K-Nearest Neighbors to discover similar movies, and plot the projected representations using T-Distributed Stochastic Neighbor Embedding to depict the context similarities. These similarities, expressed as movie distances, can be used for movies recommendation. The recommended movies of this approach are compared with the recommended movies from IMDB, which use a collaborative filtering recommendation approach, to show that our two models could constitute either an alternative or a supplementary recommendation approach.
from cs.AI updates on arXiv.org http://ift.tt/22aSy2l
via IFTTT
Landmark-based Plan Recognition. (arXiv:1604.01277v1 [cs.AI])
Recognition of goals and plans using incomplete evidence from action execution can be done efficiently by using planning techniques. In many applications it is important to recognize goals and plans not only accurately, but also quickly. In this paper, we develop a heuristic approach for recognizing plans based on planning techniques that rely on ordering constraints to filter candidate goals from observations. In the planning literature, these ordering constraints are called landmarks, which are facts or actions that cannot be avoided to achieve a goal. We show the applicability of planning landmarks in two settings: first, we use it directly to develop a heuristic-based plan recognition approach; second, we refine an existing planning-based plan recognition approach. Our empirical evaluation shows that our approach is not only substantially more accurate than the state-of-the-art in all available datasets, it is also an order of magnitude faster.
from cs.AI updates on arXiv.org http://ift.tt/1SOnFhC
via IFTTT
Deep Cross Residual Learning for Multitask Visual Recognition. (arXiv:1604.01335v1 [cs.CV])
Residual learning has recently surfaced as an effective means of constructing very deep neural networks for object recognition. However, current incarnations of residual networks do not allow for the modeling and integration of complex relations between closely coupled recognition tasks or across domains. Such problems are often encountered in multimedia and vision applications involving large-scale content recognition. We propose a novel extension of residual learning for deep networks that enables intuitive learning across multiple related tasks using cross-connections called cross-residuals. These cross-residuals connections can be viewed as a form of in-network regularization and enables greater network generalization. We show how cross-residual learning (CRL) can be integrated in multitask networks to jointly train and detect visual concepts across several tasks. We present a single multitask cross-residual network with >40% less parameters that is able to achieve competitive, or even better, detection performance on a visual sentiment concept detection problem normally requiring multiple specialized single-task networks. The resulting multitask cross-residual network also achieves better detection performance by about 10.4% over a standard multitask residual network without cross-residuals with even a small amount of cross-task weighting.
from cs.AI updates on arXiv.org http://ift.tt/1WaTrZ9
via IFTTT
Bounded Optimal Exploration in MDP. (arXiv:1604.01350v1 [cs.AI])
Within the framework of probably approximately correct Markov decision processes (PAC-MDP), much theoretical work has focused on methods to attain near optimality after a relatively long period of learning and exploration. However, practical concerns require the attainment of satisfactory behavior within a short period of time. In this paper, we relax the PAC-MDP conditions to reconcile theoretically driven exploration methods and practical needs. We propose simple algorithms for discrete and continuous state spaces, and illustrate the benefits of our proposed relaxation via theoretical analyses and numerical examples. Our algorithms also maintain anytime error bounds and average loss bounds. Our approach accommodates both Bayesian and non-Bayesian methods.
from cs.AI updates on arXiv.org http://ift.tt/1Mc85Nx
via IFTTT
The Curious Robot: Learning Visual Representations via Physical Interactions. (arXiv:1604.01360v1 [cs.CV])
What is the right supervisory signal to train visual representations? Current approaches in computer vision use category labels from datasets such as ImageNet to train ConvNets. However, in case of biological agents, visual representation learning does not require semantic labels. In fact, we argue that biological agents use active exploration and physical interactions with the world to learn visual representations unlike current vision systems which just use passive observations (images and videos downloaded from web). For example, babies push objects, poke them, put them in their mouth and throw them to learn representations. Towards this goal, we build one of the first systems on a Baxter platform that pushes, pokes, grasps and actively observes objects in a tabletop environment. It uses four different types of physical interactions to collect more than 130K datapoints, with each datapoint providing backprops to a shared ConvNet architecture allowing us to learn visual representations. We show the quality of learned representations by observing neuron activations and performing nearest neighbor retrieval on this learned representation. Finally, we evaluate our learned ConvNet on different image classification tasks and show improvements compared to learning without external data.
from cs.AI updates on arXiv.org http://ift.tt/1WaTpk2
via IFTTT
A Brain-like Cognitive Process with Shared Methods. (arXiv:1507.04928v2 [cs.AI] UPDATED)
This paper describes a detailed cognitive structure and related processes. It also suggests some specific methods for carrying out those processes, where the methods interact well with each other. The main purpose of this paper is to reaffirm earlier research on different knowledge-based and experience-based clustering techniques, by describing in more detail how they can work as part of the same system. While an earlier paper defined three levels of functional requirement, this paper re-defines the levels in a more human vernacular. The intended higher-level goals are made clearer, in terms of action-result pairs. The system is therefore modelled on the human brain, where pattern creation and activation would be automatic and distributed, and the information can flow seamlessly between the main constructs. The overall architecture has stayed essentially the same and so it is the localised processes or smaller details that have been updated. For example, a counting mechanism is used slightly differently, to measure a level of 'cohesion' instead of a 'correct' classification, over pattern instances. The Concept Trees structure can perform more than one type of role and the introduction of features has enhanced the architecture again. There is also some new information and theory.
from cs.AI updates on arXiv.org http://ift.tt/1LlCiaY
via IFTTT
Science Question Answering using Instructional Materials. (arXiv:1602.04375v2 [cs.CL] UPDATED)
We provide a solution for elementary science test using instructional materials. We posit that there is a hidden structure that explains the correctness of an answer given the question and instructional materials and present a unified max-margin framework that learns to find these hidden structures (given a corpus of question-answer pairs and instructional materials), and uses what it learns to answer novel elementary science questions. Our evaluation shows that our framework outperforms several strong baselines.
from cs.AI updates on arXiv.org http://ift.tt/1TkaiqI
via IFTTT
FIRE, First Amendment Allies Ask OCR to Reject Calls to Ban Anonymous Social Media Applications
from Google Alert - anonymous http://ift.tt/1N7pHoD
via IFTTT
Re: [FD] [SE-2012-01] Broken security fix in IBM Java 7/8
Source: Gmail -> IFTTT-> Blogger
Ravens: WR Steve Smith Sr. joins Russillo and Kanell to address his tweets on Greg Hardy; listen live in the ESPN app (ESPN)
via IFTTT
Orioles Video: Adam Jones disregards team's no-pie celebration rules and slams dessert into Matt Wieters' face (ESPN)
via IFTTT
Got my second star, this wraps up a large part of my life, now to focus on what comes next!

[FD] Apple iOS 9.3.1 (iPhone 6S & iPhone Plus) - (3D Touch) Passcode Bypass Vulnerability
Source: Gmail -> IFTTT-> Blogger