Latest YouTube Video
Saturday, January 23, 2016
anonymous posts
from Google Alert - anonymous http://ift.tt/1OOMHt5
via IFTTT
Page still accessible to anonymous users
from Google Alert - anonymous http://ift.tt/1UjdnpG
via IFTTT
Anonymous Gifts Totaling $15 million Fuel Cystic Fibrosis Research at Geisel
from Google Alert - anonymous http://ift.tt/1ZUcPgK
via IFTTT
[FD] HP LaserJet Fax Preview DLL side loading vulnerability
Source: Gmail -> IFTTT-> Blogger
[FD] HP ToComMsg DLL side loading vulnerability
Source: Gmail -> IFTTT-> Blogger
[FD] LEADTOOLS ActiveX control multiple DLL side loading vulnerabilities
Source: Gmail -> IFTTT-> Blogger
International Space Station Transits Saturn

Friday, January 22, 2016
Quand de mon cueur vous ferai part (Anonymous)
from Google Alert - anonymous http://ift.tt/1OMpZSz
via IFTTT
Do not want my blog posts as “anonymous”
from Google Alert - anonymous http://ift.tt/1QqgmNO
via IFTTT
Approaches to anonymous feature engineering?
from Google Alert - anonymous http://ift.tt/1RYPgPx
via IFTTT
ISS Daily Summary Report – 01/21/16
from ISS On-Orbit Status Report http://ift.tt/1PasPnZ
via IFTTT
Samsung Get Sued for Failing to Update its Smartphones
from The Hacker News http://ift.tt/1ndbP5H
via IFTTT
anonymous-sums
from Google Alert - anonymous http://ift.tt/1VdcuPr
via IFTTT
Google to Speed Up Chrome for Fast Internet Browsing
from The Hacker News http://ift.tt/1ZQVN31
via IFTTT
Global Temperature Anomalies from December 2015
from NASA's Scientific Visualization Studio: Most Recent Items http://ift.tt/1Ps2k9c
via IFTTT
Five-Year Global Temperature Anomalies from 1880 to 2015
from NASA's Scientific Visualization Studio: Most Recent Items http://ift.tt/1Jnnc5m
via IFTTT
The View Toward M101
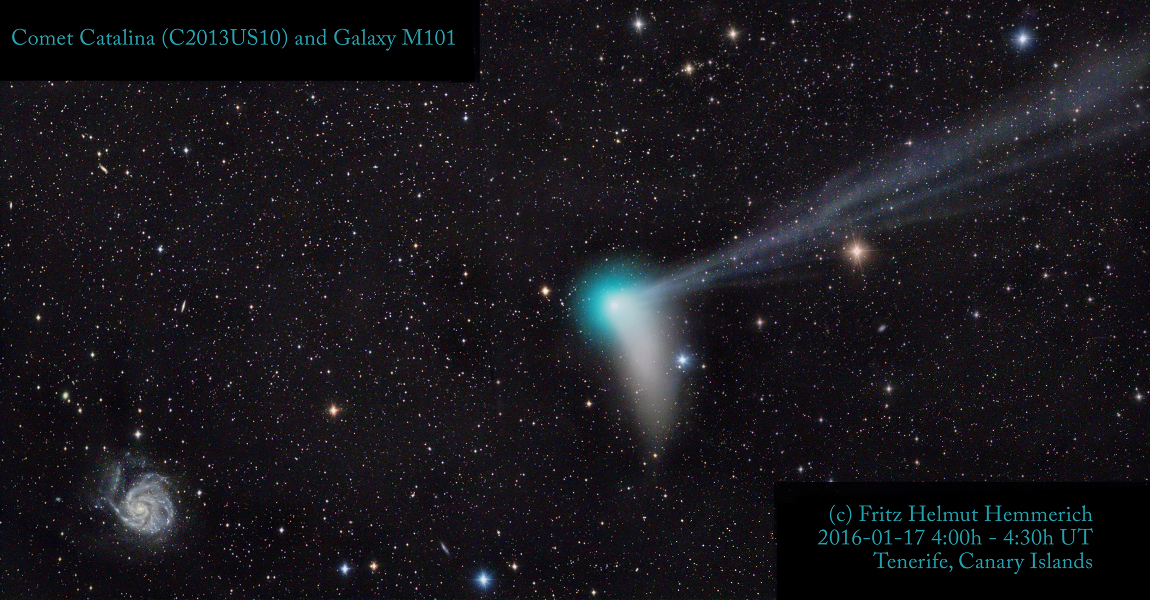
Thursday, January 21, 2016
Anonymous Feedback possible without guest login?
from Google Alert - anonymous http://ift.tt/1ZFX3kd
via IFTTT
Anonymous taking action on Flint water crisis
from Google Alert - anonymous http://ift.tt/1OAHlUy
via IFTTT
Anonymous Access to Remote API
from Google Alert - anonymous http://ift.tt/1RXfoui
via IFTTT
Neural Enquirer: Learning to Query Tables with Natural Language. (arXiv:1512.00965v2 [cs.AI] UPDATED)
We proposed Neural Enquirer as a neural network architecture to execute a natural language (NL) query on a knowledge-base (KB) for answers. Basically, Neural Enquirer finds the distributed representation of a query and then executes it on knowledge-base tables to obtain the answer as one of the values in the tables. Unlike similar efforts in end-to-end training of semantic parsers, Neural Enquirer is fully "neuralized": it not only gives distributional representation of the query and the knowledge-base, but also realizes the execution of compositional queries as a series of differentiable operations, with intermediate results (consisting of annotations of the tables at different levels) saved on multiple layers of memory. Neural Enquirer can be trained with gradient descent, with which not only the parameters of the controlling components and semantic parsing component, but also the embeddings of the tables and query words can be learned from scratch. The training can be done in an end-to-end fashion, but it can take stronger guidance, e.g., the step-by-step supervision for complicated queries, and benefit from it. Neural Enquirer is one step towards building neural network systems which seek to understand language by executing it on real-world. Our experiments show that Neural Enquirer can learn to execute fairly complicated NL queries on tables with rich structures.
from cs.AI updates on arXiv.org http://ift.tt/1jC8VFq
via IFTTT
FullCalendar not displaying color bands for anonymous
from Google Alert - anonymous http://ift.tt/1OABXkh
via IFTTT
Anonymous threats made against police in Philadelphia, NYC
from Google Alert - anonymous http://ift.tt/1PragYf
via IFTTT
You Wouldn't Believe that Too Many People Still Use Terrible Passwords
from The Hacker News http://ift.tt/1ZEquTC
via IFTTT
ISS Daily Summary Report – 01/20/16
from ISS On-Orbit Status Report http://ift.tt/1OzmyAD
via IFTTT
Free Screening of The Anonymous People and Panel Discussion
from Google Alert - anonymous http://ift.tt/1nAk0cx
via IFTTT
Ravens: Joe Flacco tells WBAL Radio his salary ($28.55M) is \"a huge number\"; open to restructuring deal for cap relief (ESPN)
via IFTTT
Anonymous threats made against police in Philadelphia, NYC
from Google Alert - anonymous http://ift.tt/1Szmxk8
via IFTTT
[FD] SEC Consult SA-20160121-0 :: Deliberately hidden backdoor account in AMX (Harman Professional) devices
Source: Gmail -> IFTTT-> Blogger
Ocean City, MD's surf is at least 6.07ft high
Ocean City, MD Summary
At 2:00 AM, surf min of 6.07ft. At 8:00 AM, surf min of 5.1ft. At 2:00 PM, surf min of 3.79ft. At 8:00 PM, surf min of 2.8ft.
Surf maximum: 7.08ft (2.16m)
Surf minimum: 6.07ft (1.85m)
Tide height: -0.65ft (-0.2m)
Wind direction: NW
Wind speed: 11.47 KTS
from Surfline http://ift.tt/1kVmigH
via IFTTT
Apple testing Ultra-Fast Li-Fi Wireless Technology for Future iPhones
from The Hacker News http://ift.tt/1RUTSGw
via IFTTT
Critical iOS Flaw allowed Hackers to Steal Cookies from Devices
from The Hacker News http://ift.tt/1SyQ3q1
via IFTTT
An Anonymous Donor Helps IEEE Bring Tech History to Life in the Classroom
from Google Alert - anonymous http://ift.tt/1P7llC6
via IFTTT
Stars and Globules in the Running Chicken Nebula
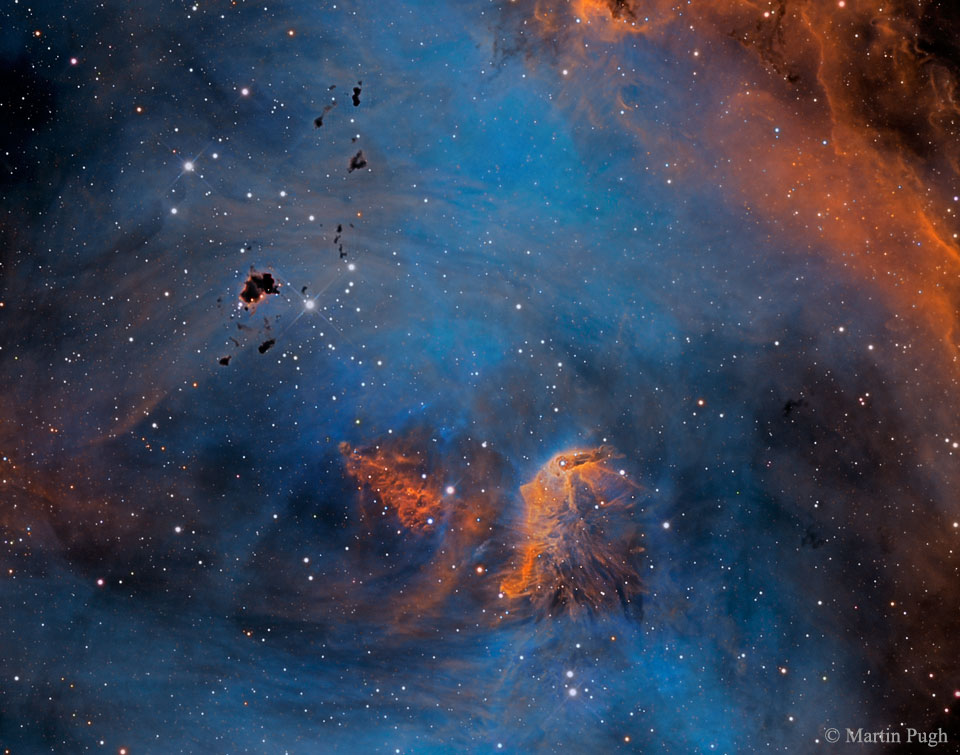
Wednesday, January 20, 2016
I have a new follower on Twitter
Jakub Wachocki
Information Mngmt / BIM Consultant. Digital transformation and process automation advocate. Lecturer. Adventurer. #ukBIMcrew #globalBIMcrew #Innovation Angel
London
https://t.co/ruk4udWTGu
Following: 2335 - Followers: 2637
January 20, 2016 at 11:37PM via Twitter http://twitter.com/_JakubW
The DARPA Twitter Bot Challenge. (arXiv:1601.05140v1 [cs.SI])
A number of organizations ranging from terrorist groups such as ISIS to politicians and nation states reportedly conduct explicit campaigns to influence opinion on social media, posing a risk to democratic processes. There is thus a growing need to identify and eliminate "influence bots" - realistic, automated identities that illicitly shape discussion on sites like Twitter and Facebook - before they get too influential. Spurred by such events, DARPA held a 4-week competition in February/March 2015 in which multiple teams supported by the DARPA Social Media in Strategic Communications program competed to identify a set of previously identified "influence bots" serving as ground truth on a specific topic within Twitter. Past work regarding influence bots often has difficulty supporting claims about accuracy, since there is limited ground truth (though some exceptions do exist [3,7]). However, with the exception of [3], no past work has looked specifically at identifying influence bots on a specific topic. This paper describes the DARPA Challenge and describes the methods used by the three top-ranked teams.
from cs.AI updates on arXiv.org http://ift.tt/1nz0wFb
via IFTTT
Semantic Word Clusters Using Signed Normalized Graph Cuts. (arXiv:1601.05403v1 [cs.CL])
Vector space representations of words capture many aspects of word similarity, but such methods tend to make vector spaces in which antonyms (as well as synonyms) are close to each other. We present a new signed spectral normalized graph cut algorithm, signed clustering, that overlays existing thesauri upon distributionally derived vector representations of words, so that antonym relationships between word pairs are represented by negative weights. Our signed clustering algorithm produces clusters of words which simultaneously capture distributional and synonym relations. We evaluate these clusters against the SimLex-999 dataset (Hill et al.,2014) of human judgments of word pair similarities, and also show the benefit of using our clusters to predict the sentiment of a given text.
from cs.AI updates on arXiv.org http://ift.tt/1OxOnZZ
via IFTTT
Unsupervised Learning of Visual Structure using Predictive Generative Networks. (arXiv:1511.06380v2 [cs.LG] UPDATED)
The ability to predict future states of the environment is a central pillar of intelligence. At its core, effective prediction requires an internal model of the world and an understanding of the rules by which the world changes. Here, we explore the internal models developed by deep neural networks trained using a loss based on predicting future frames in synthetic video sequences, using a CNN-LSTM-deCNN framework. We first show that this architecture can achieve excellent performance in visual sequence prediction tasks, including state-of-the-art performance in a standard 'bouncing balls' dataset (Sutskever et al., 2009). Using a weighted mean-squared error and adversarial loss (Goodfellow et al., 2014), the same architecture successfully extrapolates out-of-the-plane rotations of computer-generated faces. Furthermore, despite being trained end-to-end to predict only pixel-level information, our Predictive Generative Networks learn a representation of the latent structure of the underlying three-dimensional objects themselves. Importantly, we find that this representation is naturally tolerant to object transformations, and generalizes well to new tasks, such as classification of static images. Similar models trained solely with a reconstruction loss fail to generalize as effectively. We argue that prediction can serve as a powerful unsupervised loss for learning rich internal representations of high-level object features.
from cs.AI updates on arXiv.org http://ift.tt/1MwAg69
via IFTTT
How to Discount Deep Reinforcement Learning: Towards New Dynamic Strategies. (arXiv:1512.02011v2 [cs.LG] UPDATED)
Using deep neural nets as function approximator for reinforcement learning tasks have recently been shown to be very powerful for solving problems approaching real-world complexity. Using these results as a benchmark, we discuss the role that the discount factor may play in the quality of the learning process of a deep Q-network (DQN). When the discount factor progressively increases up to its final value, we empirically show that it is possible to significantly reduce the number of learning steps. When used in conjunction with a varying learning rate, we empirically show that it outperforms original DQN on several experiments. We relate this phenomenon with the instabilities of neural networks when they are used in an approximate Dynamic Programming setting. We also describe the possibility to fall within a local optimum during the learning process, thus connecting our discussion with the exploration/exploitation dilemma.
from cs.AI updates on arXiv.org http://ift.tt/1OMntPK
via IFTTT
Origami: A 803 GOp/s/W Convolutional Network Accelerator. (arXiv:1512.04295v2 [cs.CV] UPDATED)
An ever increasing number of computer vision and image/video processing challenges are being approached using deep convolutional neural networks, obtaining state-of-the-art results in object recognition and detection, semantic segmentation, action recognition, optical flow and superresolution. Hardware acceleration of these algorithms is essential to adopt these improvements in embedded and mobile computer vision systems. We present a new architecture, design and implementation as well as the first reported silicon measurements of such an accelerator, outperforming previous work in terms of power-, area- and I/O-efficiency. The manufactured device provides up to 196 GOp/s on 3.09 mm^2 of silicon in UMC 65nm technology and can achieve a power efficiency of 803 GOp/s/W. The massively reduced bandwidth requirements make it the first architecture scalable to TOp/s performance.
from cs.AI updates on arXiv.org http://ift.tt/1NtM9YW
via IFTTT
Methuen High School Evacuated After Anonymous Email Threat
from Google Alert - anonymous http://ift.tt/1Sy0D0y
via IFTTT
St. Mark's Bookshop receives support from anonymous investor, temporarily avoids closure
from Google Alert - anonymous http://ift.tt/1OH4kLu
via IFTTT
I have a new follower on Twitter
Codementor
Live 1:1 help for software development. In case we miss your message, email us at support[at]codementor.io
https://t.co/fGkcBsfQJ4
Following: 5538 - Followers: 6147
January 20, 2016 at 03:16PM via Twitter http://twitter.com/CodementorIO
Ravens Video: Ex-Baltimore RB Ray Rice describes his time away from football, still \"hopeful\" for 2nd chance in NFL (ESPN)
via IFTTT
[FD] LiteSpeed Web Server - Security Advisory - HTTP Header Injection Vulnerability
Source: Gmail -> IFTTT-> Blogger
[FD] mobile.facebook.com is not on HSTS preload list or sending the Strict-Transport-Security header
Source: Gmail -> IFTTT-> Blogger
SAVE THE DATE! 2017 Spring Retreat
from Google Alert - anonymous http://ift.tt/1nyhKCI
via IFTTT
ISS Daily Summary Report – 01/19/16
from ISS On-Orbit Status Report http://ift.tt/1nlWHUa
via IFTTT
US releases Iranian Hacker as part of Prisoner Exchange Program
from The Hacker News http://ift.tt/1OFn3XR
via IFTTT
A Dark Sand Dune on Mars
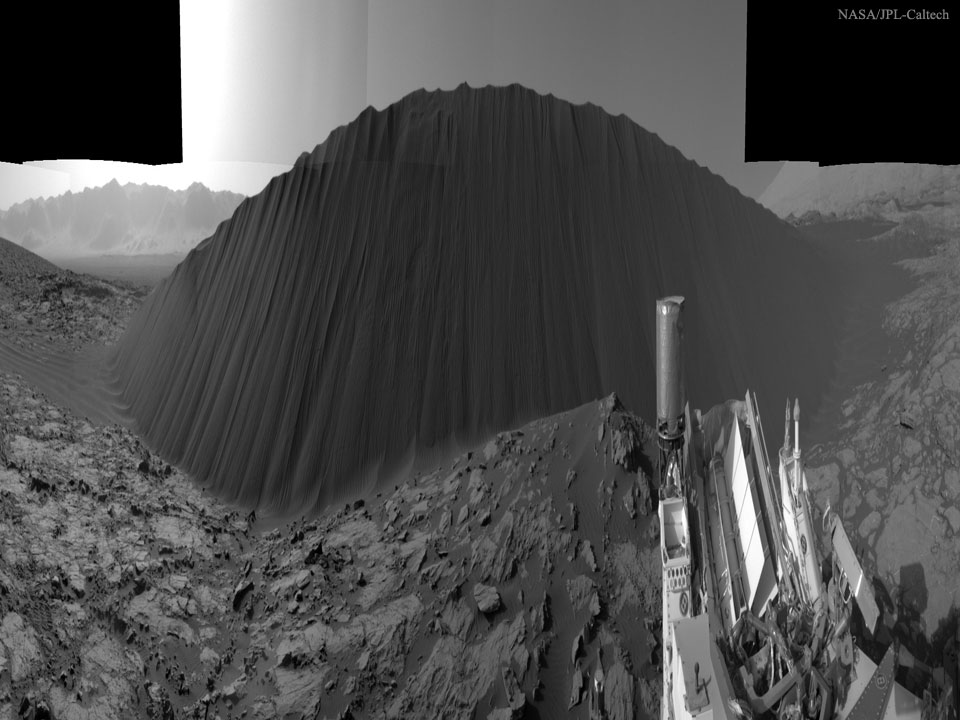
Tuesday, January 19, 2016
Top-N Recommender System via Matrix Completion. (arXiv:1601.04800v1 [cs.IR])
Top-N recommender systems have been investigated widely both in industry and academia. However, the recommendation quality is far from satisfactory. In this paper, we propose a simple yet promising algorithm. We fill the user-item matrix based on a low-rank assumption and simultaneously keep the original information. To do that, a nonconvex rank relaxation rather than the nuclear norm is adopted to provide a better rank approximation and an efficient optimization strategy is designed. A comprehensive set of experiments on real datasets demonstrates that our method pushes the accuracy of Top-N recommendation to a new level.
from cs.AI updates on arXiv.org http://ift.tt/1PE0zpW
via IFTTT
Graded Entailment for Compositional Distributional Semantics. (arXiv:1601.04908v1 [cs.CL])
The categorical compositional distributional model of natural language provides a conceptually motivated procedure to compute the meaning of sentences, given grammatical structure and the meanings of its words. This approach has outperformed other models in mainstream empirical language processing tasks. However, until now it has lacked the crucial feature of lexical entailment -- as do other distributional models of meaning.
In this paper we solve the problem of entailment for categorical compositional distributional semantics. Taking advantage of the abstract categorical framework allows us to vary our choice of model. This enables the introduction of a notion of entailment, exploiting ideas from the categorical semantics of partial knowledge in quantum computation.
The new model of language uses density matrices, on which we introduce a novel robust graded order capturing the entailment strength between concepts. This graded measure emerges from a general framework for approximate entailment, induced by any commutative monoid. Quantum logic embeds in our graded order.
Our main theorem shows that entailment strength lifts compositionally to the sentence level, giving a lower bound on sentence entailment. We describe the essential properties of graded entailment such as continuity, and provide a procedure for calculating entailment strength.
from cs.AI updates on arXiv.org http://ift.tt/1P4hMN7
via IFTTT
Semantics for probabilistic programming: higher-order functions, continuous distributions, and soft constraints. (arXiv:1601.04943v1 [cs.PL])
We study the semantic foundation of expressive probabilistic programming languages, that support higher-order functions, continuous distributions, and soft constraints (such as Anglican, Church, and Venture). We define a metalanguage (an idealised version of Anglican) for probabilistic computation with the above features, develop both operational and denotational semantics, and prove soundness, adequacy, and termination. They involve measure theory, stochastic labelled transition systems, and functor categories, but admit intuitive computational readings, one of which views sampled random variables as dynamically allocated read-only variables. We apply our semantics to validate nontrivial equations underlying the correctness of certain compiler optimisations and inference algorithms such as sequential Monte Carlo simulation. The language enables defining probability distributions on higher-order functions, and we study their properties.
from cs.AI updates on arXiv.org http://ift.tt/1ltdYJ2
via IFTTT
Sample Complexity of Episodic Fixed-Horizon Reinforcement Learning. (arXiv:1510.08906v2 [stat.ML] UPDATED)
Recently, there has been significant progress in understanding reinforcement learning in discounted infinite-horizon Markov decision processes (MDPs) by deriving tight sample complexity bounds. However, in many real-world applications, an interactive learning agent operates for a fixed or bounded period of time, for example tutoring students for exams or handling customer service requests. Such scenarios can often be better treated as episodic fixed-horizon MDPs, for which only looser bounds on the sample complexity exist. A natural notion of sample complexity in this setting is the number of episodes required to guarantee a certain performance with high probability (PAC guarantee). In this paper, we derive an upper PAC bound $\tilde O(\frac{|\mathcal S|^2 |\mathcal A| H^2}{\epsilon^2} \ln\frac 1 \delta)$ and a lower PAC bound $\tilde \Omega(\frac{|\mathcal S| |\mathcal A| H^2}{\epsilon^2} \ln \frac 1 {\delta + c})$ that match up to log-terms and an additional linear dependency on the number of states $|\mathcal S|$. The lower bound is the first of its kind for this setting. Our upper bound leverages Bernstein's inequality to improve on previous bounds for episodic finite-horizon MDPs which have a time-horizon dependency of at least $H^3$.
from cs.AI updates on arXiv.org http://ift.tt/1GWeRnG
via IFTTT
Ocean City, MD's surf is at least 5.1ft high
Ocean City, MD Summary
At 2:00 AM, surf min of 5.1ft. At 8:00 AM, surf min of 4.05ft. At 2:00 PM, surf min of 3.22ft. At 8:00 PM, surf min of 3.15ft.
Surf maximum: 6.08ft (1.85m)
Surf minimum: 5.1ft (1.55m)
Tide height: -0.5ft (-0.15m)
Wind direction: NNW
Wind speed: 24.61 KTS
from Surfline http://ift.tt/1kVmigH
via IFTTT
First Amendment Protects Anonymous Speech, and for Good Reason
from Google Alert - anonymous http://ift.tt/1PoNOit
via IFTTT
Facebook adds Built-in Tor Support for its Android App
from The Hacker News http://ift.tt/1nwpFAr
via IFTTT
Will this module disable caching for anonymous users?
from Google Alert - anonymous http://ift.tt/1RymOn9
via IFTTT
Zero-Day Flaw Found in 'Linux Kernel' leaves Millions Vulnerable
from The Hacker News http://ift.tt/1Kposjm
via IFTTT
I have a new follower on Twitter
Microsoft Enterprise
Dear followers, @MSFTEnterprise is transitioning to Microsoft in Business, effective 2/01/16. Please follow us on our new handle @MSFT_Business today!
Redmond, WA
http://t.co/kNLYDTdpEP
Following: 10141 - Followers: 30898
January 19, 2016 at 11:57AM via Twitter http://twitter.com/MSFTenterprise
Ravens: Ex-Baltimore RB Ray Rice to coach running backs on Mike Martz's staff at the NFLPA Collegiate Bowl (ESPN)
via IFTTT
Anonymous complaint about Dutch economist is "unfounded": Report
from Google Alert - anonymous http://ift.tt/1ZK9ZuD
via IFTTT
Proxima Centauri: The Closest Star
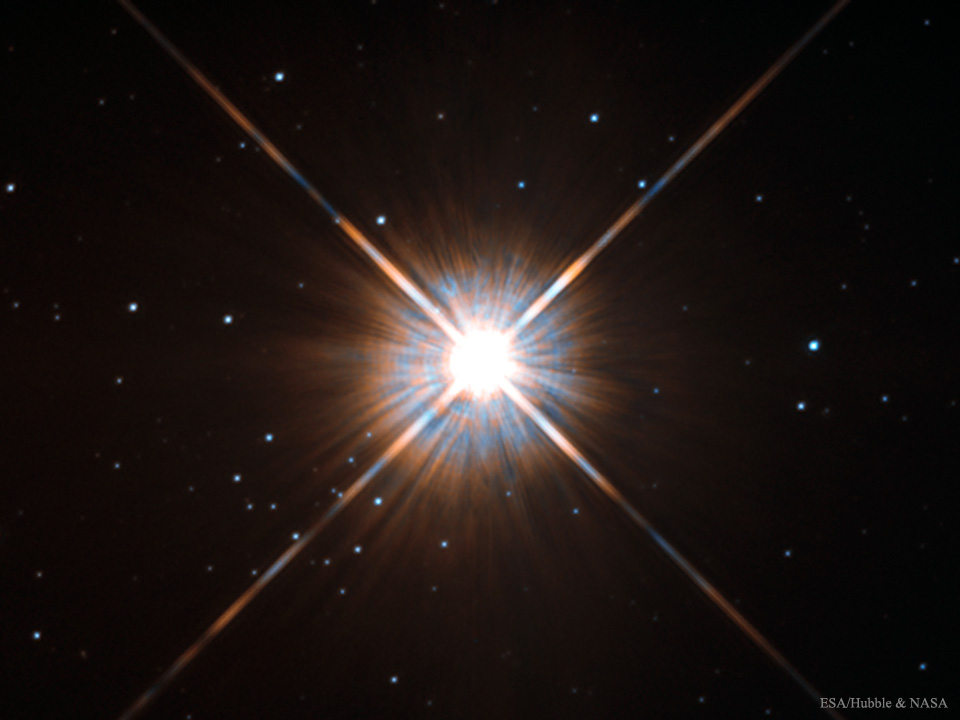
Monday, January 18, 2016
Learning the Semantics of Structured Data Sources. (arXiv:1601.04105v1 [cs.AI])
Information sources such as relational databases, spreadsheets, XML, JSON, and Web APIs contain a tremendous amount of structured data that can be leveraged to build and augment knowledge graphs. However, they rarely provide a semantic model to describe their contents. Semantic models of data sources represent the implicit meaning of the data by specifying the concepts and the relationships within the data. Such models are the key ingredients to automatically publish the data into knowledge graphs. Manually modeling the semantics of data sources requires significant effort and expertise, and although desirable, building these models automatically is a challenging problem. Most of the related work focuses on semantic annotation of the data fields (source attributes). However, constructing a semantic model that explicitly describes the relationships between the attributes in addition to their semantic types is critical.
We present a novel approach that exploits the knowledge from a domain ontology and the semantic models of previously modeled sources to automatically learn a rich semantic model for a new source. This model represents the semantics of the new source in terms of the concepts and relationships defined by the domain ontology. Given some sample data from the new source, we leverage the knowledge in the domain ontology and the known semantic models to construct a weighted graph that represents the space of plausible semantic models for the new source. Then, we compute the top k candidate semantic models and suggest to the user a ranked list of the semantic models for the new source. The approach takes into account user corrections to learn more accurate semantic models on future data sources. Our evaluation shows that our method generates expressive semantic models for data sources and services with minimal user input. ...
from cs.AI updates on arXiv.org http://ift.tt/1NhlLjD
via IFTTT
Engineering Safety in Machine Learning. (arXiv:1601.04126v1 [stat.ML])
Machine learning algorithms are increasingly influencing our decisions and interacting with us in all parts of our daily lives. Therefore, just like for power plants, highways, and myriad other engineered sociotechnical systems, we must consider the safety of systems involving machine learning. In this paper, we first discuss the definition of safety in terms of risk, epistemic uncertainty, and the harm incurred by unwanted outcomes. Then we examine dimensions, such as the choice of cost function and the appropriateness of minimizing the empirical average training cost, along which certain real-world applications may not be completely amenable to the foundational principle of modern statistical machine learning: empirical risk minimization. In particular, we note an emerging dichotomy of applications: ones in which safety is important and risk minimization is not the complete story (we name these Type A applications), and ones in which safety is not so critical and risk minimization is sufficient (we name these Type B applications). Finally, we discuss how four different strategies for achieving safety in engineering (inherently safe design, safety reserves, safe fail, and procedural safeguards) can be mapped to the machine learning context through interpretability and causality of predictive models, objectives beyond expected prediction accuracy, human involvement for labeling difficult or rare examples, and user experience design of software.
from cs.AI updates on arXiv.org http://ift.tt/1ZIxC6U
via IFTTT
$\mathbf{D^3}$: Deep Dual-Domain Based Fast Restoration of JPEG-Compressed Images. (arXiv:1601.04149v1 [cs.CV])
In this paper, we design a Deep Dual-Domain ($\mathbf{D^3}$) based fast restoration model to remove artifacts of JPEG compressed images. It leverages the large learning capacity of deep networks, as well as the problem-specific expertise that was hardly incorporated in the past design of deep architectures. For the latter, we take into consideration both the prior knowledge of the JPEG compression scheme, and the successful practice of the sparsity-based dual-domain approach. We further design the One-Step Sparse Inference (1-SI) module, as an efficient and light-weighted feed-forward approximation of sparse coding. Extensive experiments verify the superiority of the proposed $D^3$ model over several state-of-the-art methods. Specifically, our best model is capable of outperforming the latest deep model for around 1 dB in PSNR, and is 30 times faster.
from cs.AI updates on arXiv.org http://ift.tt/1NhlLjz
via IFTTT
Studying Very Low Resolution Recognition Using Deep Networks. (arXiv:1601.04153v1 [cs.CV])
Visual recognition research often assumes a sufficient resolution of the region of interest (ROI). That is usually violated in practical scenarios, inspiring us to explore the general Very Low Resolution Recognition (VLRR) problem. Typically, the ROI in a VLRR problem can be smaller than $16 \times 16$ pixels, and is challenging to be recognized even by human experts. We attempt to solve the VLRR problem using deep learning methods. Taking advantage of techniques primarily in super resolution, domain adaptation and robust regression, we formulate a dedicated deep learning method and demonstrate how these techniques are incorporated step by step. That leads to a series of well-motivated and powerful models. Any extra complexity due to the introduction of a new model is fully justified by both analysis and simulation results. The resulting \textit{Robust Partially Coupled Networks} achieves feature enhancement and recognition simultaneously, while allowing for both the flexibility to combat the LR-HR domain mismatch and the robustness to outliers. Finally, the effectiveness of the proposed models is evaluated on three different VLRR tasks, including face identification, digit recognition and font recognition, all of which obtain very impressive performances.
from cs.AI updates on arXiv.org http://ift.tt/1ZIxC6K
via IFTTT
SimpleDS: A Simple Deep Reinforcement Learning Dialogue System. (arXiv:1601.04574v1 [cs.AI])
This paper presents 'SimpleDS', a simple and publicly available dialogue system trained with deep reinforcement learning. In contrast to previous reinforcement learning dialogue systems, this system avoids manual feature engineering by performing action selection directly from raw text of the last system and (noisy) user responses. Our initial results, in the restaurant domain, show that it is indeed possible to induce reasonable dialogue behaviour with an approach that aims for high levels of automation in dialogue control for intelligent interactive agents.
from cs.AI updates on arXiv.org http://ift.tt/1NhlL3h
via IFTTT
Proactive Message Passing on Memory Factor Networks. (arXiv:1601.04667v1 [cs.AI])
We introduce a new type of graphical model that we call a "memory factor network" (MFN). We show how to use MFNs to model the structure inherent in many types of data sets. We also introduce an associated message-passing style algorithm called "proactive message passing"' (PMP) that performs inference on MFNs. PMP comes with convergence guarantees and is efficient in comparison to competing algorithms such as variants of belief propagation. We specialize MFNs and PMP to a number of distinct types of data (discrete, continuous, labelled) and inference problems (interpolation, hypothesis testing), provide examples, and discuss approaches for efficient implementation.
from cs.AI updates on arXiv.org http://ift.tt/1ZIxww3
via IFTTT
Spectral Ranking using Seriation. (arXiv:1406.5370v3 [cs.LG] UPDATED)
We describe a seriation algorithm for ranking a set of items given pairwise comparisons between these items. Intuitively, the algorithm assigns similar rankings to items that compare similarly with all others. It does so by constructing a similarity matrix from pairwise comparisons, using seriation methods to reorder this matrix and construct a ranking. We first show that this spectral seriation algorithm recovers the true ranking when all pairwise comparisons are observed and consistent with a total order. We then show that ranking reconstruction is still exact when some pairwise comparisons are corrupted or missing, and that seriation based spectral ranking is more robust to noise than classical scoring methods. Finally, we bound the ranking error when only a random subset of the comparions are observed. An additional benefit of the seriation formulation is that it allows us to solve semi-supervised ranking problems. Experiments on both synthetic and real datasets demonstrate that seriation based spectral ranking achieves competitive and in some cases superior performance compared to classical ranking methods.
from cs.AI updates on arXiv.org http://ift.tt/1p7LaGZ
via IFTTT
Reasoning about Entailment with Neural Attention. (arXiv:1509.06664v3 [cs.CL] UPDATED)
While most approaches to automatically recognizing entailment relations have used classifiers employing hand engineered features derived from complex natural language processing pipelines, in practice their performance has been only slightly better than bag-of-word pair classifiers using only lexical similarity. The only attempt so far to build an end-to-end differentiable neural network for entailment failed to outperform such a simple similarity classifier. In this paper, we propose a neural model that reads two sentences to determine entailment using long short-term memory units. We extend this model with a word-by-word neural attention mechanism that encourages reasoning over entailments of pairs of words and phrases. Furthermore, we present a qualitative analysis of attention weights produced by this model, demonstrating such reasoning capabilities. On a large entailment dataset this model outperforms the previous best neural model and a classifier with engineered features by a substantial margin. It is the first generic end-to-end differentiable system that achieves state-of-the-art accuracy on a textual entailment dataset.
from cs.AI updates on arXiv.org http://ift.tt/1NRZGOz
via IFTTT
Unifying Decision Trees Split Criteria Using Tsallis Entropy. (arXiv:1511.08136v4 [stat.ML] UPDATED)
The construction of efficient and effective decision trees remains a key topic in machine learning because of their simplicity and flexibility. A lot of heuristic algorithms have been proposed to construct near-optimal decision trees. ID3, C4.5 and CART are classical decision tree algorithms and the split criteria they used are Shannon entropy, Gain Ratio and Gini index respectively. All the split criteria seem to be independent, actually, they can be unified in a Tsallis entropy framework. Tsallis entropy is a generalization of Shannon entropy and provides a new approach to enhance decision trees' performance with an adjustable parameter $q$. In this paper, a Tsallis Entropy Criterion (TEC) algorithm is proposed to unify Shannon entropy, Gain Ratio and Gini index, which generalizes the split criteria of decision trees. More importantly, we reveal the relations between Tsallis entropy with different $q$ and other split criteria. Experimental results on UCI data sets indicate that the TEC algorithm achieves statistically significant improvement over the classical algorithms.
from cs.AI updates on arXiv.org http://ift.tt/1R7ieuG
via IFTTT
A Novel Regularized Principal Graph Learning Framework on Explicit Graph Representation. (arXiv:1512.02752v2 [cs.AI] UPDATED)
Many scientific datasets are of high dimension, and the analysis usually requires visual manipulation by retaining the most important structures of data. Principal curve is a widely used approach for this purpose. However, many existing methods work only for data with structures that are not self-intersected, which is quite restrictive for real applications. A few methods can overcome the above problem, but they either require complicated human-made rules for a specific task with lack of convergence guarantee and adaption flexibility to different tasks, or cannot obtain explicit structures of data. To address these issues, we develop a new regularized principal graph learning framework that captures the local information of the underlying graph structure based on reversed graph embedding. As showcases, models that can learn a spanning tree or a weighted undirected $\ell_1$ graph are proposed, and a new learning algorithm is developed that learns a set of principal points and a graph structure from data, simultaneously. The new algorithm is simple with guaranteed convergence. We then extend the proposed framework to deal with large-scale data. Experimental results on various synthetic and six real world datasets show that the proposed method compares favorably with baselines and can uncover the underlying structure correctly.
from cs.AI updates on arXiv.org http://ift.tt/1jP1URQ
via IFTTT
ISS Daily Summary Report – 1/18/16
from ISS On-Orbit Status Report http://ift.tt/1ngz8fB
via IFTTT
Anonymous VPN Forum, Virtual Services | PIA
from Google Alert - anonymous http://ift.tt/23bab5t
via IFTTT
I have a new follower on Twitter
Animated Loop
We're a digital gallery that retweets looping GIFs directly from artists' twitter profiles. Artists, we'll be hosting weekly themed challenges starting Jan 1st.
U.S.
Following: 1075 - Followers: 1706
January 18, 2016 at 04:42PM via Twitter http://twitter.com/animatedloop
(requirejs) Uncaught Error: Mismatched anonymous define() module
from Google Alert - anonymous http://ift.tt/1WnOvOT
via IFTTT
Orioles: Baltimore restaurant offers Yoenis Cespedes free crab cakes for life if he signs with Orioles (ESPN)
via IFTTT
Multiple cameras with the Raspberry Pi and OpenCV
I’ll keep the introduction to today’s post short, since I think the title of this post and GIF animation above speak for themselves.
Inside this post, I’ll demonstrate how to attach multiple cameras to your Raspberry Pi…and access all of them using a single Python script.
Regardless if your setup includes:
- Multiple USB webcams.
- Or the Raspberry Pi camera module + additional USB cameras…
…the code detailed in this post will allow you to access all of your video streams — and perform motion detection on each of them!
Best of all, our implementation of multiple camera access with the Raspberry Pi and OpenCV is capable of running in real-time (or near real-time, depending on the number of cameras you have attached), making it perfect for creating your own multi-camera home surveillance system.
Keep reading to learn more.
Looking for the source code to this post?
Jump right to the downloads section.
When building a Raspberry Pi setup to leverage multiple cameras, you have two options:
- Simply use multiple USB web cams.
- Or use one Raspberry Pi camera module and at least one USB web camera.
The Raspberry Pi board has only one camera port, so you will not be able to use multiple Raspberry Pi camera boards (unless you want to perform some extensive hacks to your Pi). So in order to attach multiple cameras to your Pi, you’ll need to leverage at least one (if not more) USB cameras.
That said, in order to build my own multi-camera Raspberry Pi setup, I ended up using:
- A Raspberry Pi camera module + camera housing (optional). We can interface with the camera using the
picamera
Python package or (preferably) the threadedVideoStream
class defined in a previous blog post. - A Logitech C920 webcam that is plug-and-play compatible with the Raspberry Pi. We can access this camera using either the
cv2.VideoCapture
function built-in to OpenCV or theVideoStream
class from this lesson.
You can see an example of my setup below:
Here we can see my Raspberry Pi 2, along with the Raspberry Pi camera module (sitting on top of the Pi 2) and my Logitech C920 webcam.
The Raspberry Pi camera module is pointing towards my apartment door to monitor anyone that is entering and leaving, while the USB webcam is pointed towards the kitchen, observing any activity that may be going on:
Figure 2: The Raspberry Pi camera module and USB camera are both hooked up to my Raspberry Pi, but are monitoring different areas of the room.
Ignore the electrical tape and cardboard on the USB camera — this was from a previous experiment which should (hopefully) be published on the PyImageSearch blog soon.
Finally, you can see an example of both video feeds displayed to my Raspberry Pi in the image below:
Figure 3: An example screenshot of monitoring both video feeds from the multiple camera Raspberry Pi setup.
In the remainder of this blog post, we’ll define a simple motion detection class that can detect if a person/object is moving in the field of view of a given camera. We’ll then write a Python driver script that instantiates our two video streams and performs motion detection in both of them.
As we’ll see, by using the threaded video stream capture classes (where one thread per camera is dedicated to perform I/O operations, allowing the main program thread to continue unblocked), we can easily get our motion detectors for multiple cameras to run in real-time on the Raspberry Pi 2.
Let’s go ahead and get started by defining the simple motion detector class.
Defining our simple motion detector
In this section, we’ll build a simple Python class that can be used to detect motion in a field of view of a given camera.
For efficiency, this class will assume there is only one object moving in the camera view at a time — in future blog posts, we’ll look at more advanced motion detection and background subtraction methods to track multiple objects.
In fact, we have already (partially) reviewed this motion detection method in our previous lesson, home surveillance and motion detection with the Raspberry Pi, Python, OpenCV, and Dropbox — we are now formalizing this implementation into a reusable class rather than just inline code.
Let’s get started by opening a new file, naming it
basicmotiondetector.py, and adding in the following code:
# import the necessary packages import imutils import cv2 class BasicMotionDetector: def __init__(self, accumWeight=0.5, deltaThresh=5, minArea=5000): # determine the OpenCV version, followed by storing the # the frame accumulation weight, the fixed threshold for # the delta image, and finally the minimum area required # for "motion" to be reported self.isv2 = imutils.is_cv2() self.accumWeight = accumWeight self.deltaThresh = deltaThresh self.minArea = minArea # initialize the average image for motion detection self.avg = None
Line 6 defines the constructor to our
BasicMotionDetectorclass. The constructor accepts three optional keyword arguments, which include:
-
accumWeight
: The floating point value used for the taking the weighted average between the current frame and the previous set of frames. A largeraccumWeight
will result in the background model having less “memory” and quickly “forgetting” what previous frames looked like. Using a high value ofaccumWeight
is useful if you except lots of motion in a short amount of time. Conversely, smaller values ofaccumWeight
give more weight to the background model than the current frame, allowing you to detect larger changes in the foreground. We’ll use a default value of0.5
in this example, just keep in mind that this is a tunable parameter that you should consider working with. -
deltaThresh
: After computing the difference between the current frame and the background model, we’ll need to apply thresholding to find regions in a frame that contain motion — thisdeltaThresh
value is used for the thresholding. Smaller values ofdeltaThresh
will detect more motion, while larger values will detect less motion. -
minArea
: After applying thresholding, we’ll be left with a binary image that we extract contours from. In order to handle noise and ignore small regions of motion, we can use theminArea
parameter. Any region with> minArea
is labeled as “motion”; otherwise, it is ignored.
Finally, Line 17 initializes
avg, which is simply the running, weighted average of the previous frames the
BasicMotionDetectorhas seen.
Let’s move on to our
updatemethod:
# import the necessary packages import imutils import cv2 class BasicMotionDetector: def __init__(self, accumWeight=0.5, deltaThresh=5, minArea=5000): # determine the OpenCV version, followed by storing the # the frame accumulation weight, the fixed threshold for # the delta image, and finally the minimum area required # for "motion" to be reported self.isv2 = imutils.is_cv2() self.accumWeight = accumWeight self.deltaThresh = deltaThresh self.minArea = minArea # initialize the average image for motion detection self.avg = None def update(self, image): # initialize the list of locations containing motion locs = [] # if the average image is None, initialize it if self.avg is None: self.avg = image.astype("float") return locs # otherwise, accumulate the weighted average between # the current frame and the previous frames, then compute # the pixel-wise differences between the current frame # and running average cv2.accumulateWeighted(image, self.avg, self.accumWeight) frameDelta = cv2.absdiff(image, cv2.convertScaleAbs(self.avg)
The
updatefunction requires a single parameter — the image we want to detect motion in.
Line 21 initializes
locs, the list of contours that correspond to motion locations in the image. However, if the
avghas not been initialized (Lines 24-26), we set
avgto the current frame and return from the method.
Otherwise, the
avghas already been initialized so we accumulate the running, weighted average between the previous frames and the current frames, using the
accumWeightvalue supplied to the constructor (Line 32). Taking the absolute value difference between the current frame and the running average yields regions of the image that contain motion — we call this our delta image.
However, in order to actually detect regions in our delta image that contain motion, we first need to apply thresholding and contour detection:
# import the necessary packages import imutils import cv2 class BasicMotionDetector: def __init__(self, accumWeight=0.5, deltaThresh=5, minArea=5000): # determine the OpenCV version, followed by storing the # the frame accumulation weight, the fixed threshold for # the delta image, and finally the minimum area required # for "motion" to be reported self.isv2 = imutils.is_cv2() self.accumWeight = accumWeight self.deltaThresh = deltaThresh self.minArea = minArea # initialize the average image for motion detection self.avg = None def update(self, image): # initialize the list of locations containing motion locs = [] # if the average image is None, initialize it if self.avg is None: self.avg = image.astype("float") return locs # otherwise, accumulate the weighted average between # the current frame and the previous frames, then compute # the pixel-wise differences between the current frame # and running average cv2.accumulateWeighted(image, self.avg, self.accumWeight) frameDelta = cv2.absdiff(image, cv2.convertScaleAbs(self.avg)) # threshold the delta image and apply a series of dilations # to help fill in holes thresh = cv2.threshold(frameDelta, self.deltaThresh, 255, cv2.THRESH_BINARY)[1] thresh = cv2.dilate(thresh, None, iterations=2) # find contours in the thresholded image, taking care to # use the appropriate version of OpenCV cnts = cv2.findContours(thresh, cv2.RETR_EXTERNAL, cv2.CHAIN_APPROX_SIMPLE) cnts = cnts[0] if self.isv2 else cnts[1] # loop over the contours for c in cnts: # only add the contour to the locations list if it # exceeds the minimum area if cv2.contourArea(c) > self.minArea: locs.append(c) # return the set of locations return locs
Calling
cv2.thresholdusing the supplied value of
deltaThreshallows us to binarize the delta image, which we then find contours in (Lines 37-45).
Note: Take special care when examining Lines 43-45. As we know, the
cv2.findContoursmethod return signature changed between OpenCV 2.4 and 3. This codeblock allows us to use
cv2.findContoursin both OpenCV 2.4 and 3 without having to change a line of code (or worry about versioning issues).
Finally, Lines 48-52 loop over the detected contours, check to see if their area is greater than the supplied
minArea, and if so, updates the
locslist.
The list of contours containing motion are then returned to calling method on Line 55.
Note: Again, for a more detailed review of the motion detection algorithm, please see the home surveillance tutorial.
Accessing multiple cameras on the Raspberry Pi
Now that our
BasicMotionDetectorclass has been defined, we are now ready to create the
multi_cam_motion.pydriver script to access the multiple cameras with the Raspberry Pi — and apply motion detection to each of the video streams.
Let’s go ahead and get started defining our driver script:
# import the necessary packages from __future__ import print_function from pyimagesearch.basicmotiondetector import BasicMotionDetector from imutils.video import VideoStream import numpy as np import datetime import imutils import time import cv2 # initialize the video streams and allow them to warmup print("[INFO] starting cameras...") webcam = VideoStream(src=0).start() picam = VideoStream(usePiCamera=True).start() time.sleep(2.0) # initialize the two motion detectors, along with the total # number of frames read camMotion = BasicMotionDetector() piMotion = BasicMotionDetector() total = 0
We start off on Lines 2-9 by importing our required Python packages. Notice how we have placed the
BasicMotionDetectorclass inside the
pyimagesearchmodule for organizational purposes. We also import
VideoStream, our threaded video stream class that is capable of accessing both the Raspberry Pi camera module and built-in/USB web cameras.
The
VideoStreamclass is part of the imutils package, so if you do not already have it installed, just execute the following command:
$ pip install imutils
Line 13 initializes our USB webcam
VideoStreamclass while Line 14 initializes our Raspberry Pi camera module
VideoStreamclass (by specifying
usePiCamera=True).
In the case that you do not want to use the Raspberry Pi camera module and instead want to leverage two USB cameras, simply changes Lines 13 and 14 to:
webcam1 = VideoStream(src=0).start() webcam2 = VideoStream(src=1).start()
Where the
srcparameter controls the index of the camera on your machine. Also note that you’ll have to replace
webcamand
picamwith
webcam1and
webcam2, respectively throughout the rest of this script as well.
Finally, Lines 19 and 20 instantiate two
BasicMotionDetector‘s, one for the USB camera and a second for the Raspberry Pi camera module.
We are now ready to perform motion detection in both video feeds:
# import the necessary packages from __future__ import print_function from pyimagesearch.basicmotiondetector import BasicMotionDetector from imutils.video import VideoStream import numpy as np import datetime import imutils import time import cv2 # initialize the video streams and allow them to warmup print("[INFO] starting cameras...") webcam = VideoStream(src=0).start() picam = VideoStream(usePiCamera=True).start() time.sleep(2.0) # initialize the two motion detectors, along with the total # number of frames read camMotion = BasicMotionDetector() piMotion = BasicMotionDetector() total = 0 # loop over frames from the video streams while True: # initialize the list of frames that have been processed frames = [] # loop over the frames and their respective motion detectors for (stream, motion) in zip((webcam, picam), (camMotion, piMotion)): # read the next frame from the video stream and resize # it to have a maximum width of 400 pixels frame = stream.read() frame = imutils.resize(frame, width=400) # convert the frame to grayscale, blur it slightly, update # the motion detector gray = cv2.cvtColor(frame, cv2.COLOR_BGR2GRAY) gray = cv2.GaussianBlur(gray, (21, 21), 0) locs = motion.update(gray) # we should allow the motion detector to "run" for a bit # and accumulate a set of frames to form a nice average if total < 32: frames.append(frame) continue
On Line 24 we start an infinite loop that is used to constantly poll frames from our (two) camera sensors. We initialize a list of such
frameson Line 26.
Then, Line 29 defines a
forloop that loops over each of the video stream and motion detectors, respectively. We use the
streamto read a
framefrom our camera sensor and then resize the frame to have a fixed width of 400 pixels.
Further pre-processing is performed on Lines 37 and 38 by converting the frame to grayscale and applying a Gaussian smoothing operation to reduce high frequency noise. Finally, the processed frame is passed to our
motiondetector where the actual motion detection is performed (Line 39).
However, it’s important to let our motion detector “run” for a bit so that it can obtain an accurate running average of what our background “looks like”. We’ll allow 32 frames to be used in the average background computation before applying any motion detection (Lines 43-45).
After we have allowed 32 frames to be passed into our
BasicMotionDetector’s, we can check to see if any motion was detected:
# import the necessary packages from __future__ import print_function from pyimagesearch.basicmotiondetector import BasicMotionDetector from imutils.video import VideoStream import numpy as np import datetime import imutils import time import cv2 # initialize the video streams and allow them to warmup print("[INFO] starting cameras...") webcam = VideoStream(src=0).start() picam = VideoStream(usePiCamera=True).start() time.sleep(2.0) # initialize the two motion detectors, along with the total # number of frames read camMotion = BasicMotionDetector() piMotion = BasicMotionDetector() total = 0 # loop over frames from the video streams while True: # initialize the list of frames that have been processed frames = [] # loop over the frames and their respective motion detectors for (stream, motion) in zip((webcam, picam), (camMotion, piMotion)): # read the next frame from the video stream and resize # it to have a maximum width of 400 pixels frame = stream.read() frame = imutils.resize(frame, width=400) # convert the frame to grayscale, blur it slightly, update # the motion detector gray = cv2.cvtColor(frame, cv2.COLOR_BGR2GRAY) gray = cv2.GaussianBlur(gray, (21, 21), 0) locs = motion.update(gray) # we should allow the motion detector to "run" for a bit # and accumulate a set of frames to form a nice average if total < 32: frames.append(frame) continue # otherwise, check to see if motion was detected if len(locs) > 0: # initialize the minimum and maximum (x, y)-coordinates, # respectively (minX, minY) = (np.inf, np.inf) (maxX, maxY) = (-np.inf, -np.inf) # loop over the locations of motion and accumulate the # minimum and maximum locations of the bounding boxes for l in locs: (x, y, w, h) = cv2.boundingRect(l) (minX, maxX) = (min(minX, x), max(maxX, x + w)) (minY, maxY) = (min(minY, y), max(maxY, y + h)) # draw the bounding box cv2.rectangle(frame, (minX, minY), (maxX, maxY), (0, 0, 255), 3) # update the frames list frames.append(frame)
Line 48 checks to see if motion was detected in the
frameof the current video
stream.
Provided that motion was detected, we initialize the minimum and maximum (x, y)-coordinates associated with the contours (i.e.,
locs). We then loop over the contours individually and use them to determine the smallest bounding box that encompasses all contours (Lines 51-59).
The bounding box is then drawn surrounding the motion region on Lines 62 and 63, followed by our list of
framesupdated on Line 66.
Again, the code detailed in this blog post assumes that there is only one object/person moving at a time in the given frame, hence this approach will obtain the desired result. However, if there are multiple moving objects, then we’ll need to use more advanced background subtraction and tracking methods — future blog posts on PyImageSearch will cover how to perform multi-object tracking.
The last step is to display our
framesto our screen:
# import the necessary packages from __future__ import print_function from pyimagesearch.basicmotiondetector import BasicMotionDetector from imutils.video import VideoStream import numpy as np import datetime import imutils import time import cv2 # initialize the video streams and allow them to warmup print("[INFO] starting cameras...") webcam = VideoStream(src=0).start() picam = VideoStream(usePiCamera=True).start() time.sleep(2.0) # initialize the two motion detectors, along with the total # number of frames read camMotion = BasicMotionDetector() piMotion = BasicMotionDetector() total = 0 # loop over frames from the video streams while True: # initialize the list of frames that have been processed frames = [] # loop over the frames and their respective motion detectors for (stream, motion) in zip((webcam, picam), (camMotion, piMotion)): # read the next frame from the video stream and resize # it to have a maximum width of 400 pixels frame = stream.read() frame = imutils.resize(frame, width=400) # convert the frame to grayscale, blur it slightly, update # the motion detector gray = cv2.cvtColor(frame, cv2.COLOR_BGR2GRAY) gray = cv2.GaussianBlur(gray, (21, 21), 0) locs = motion.update(gray) # we should allow the motion detector to "run" for a bit # and accumulate a set of frames to form a nice average if total < 32: frames.append(frame) continue # otherwise, check to see if motion was detected if len(locs) > 0: # initialize the minimum and maximum (x, y)-coordinates, # respectively (minX, minY) = (np.inf, np.inf) (maxX, maxY) = (-np.inf, -np.inf) # loop over the locations of motion and accumulate the # minimum and maximum locations of the bounding boxes for l in locs: (x, y, w, h) = cv2.boundingRect(l) (minX, maxX) = (min(minX, x), max(maxX, x + w)) (minY, maxY) = (min(minY, y), max(maxY, y + h)) # draw the bounding box cv2.rectangle(frame, (minX, minY), (maxX, maxY), (0, 0, 255), 3) # update the frames list frames.append(frame) # increment the total number of frames read and grab the # current timestamp total += 1 timestamp = datetime.datetime.now() ts = timestamp.strftime("%A %d %B %Y %I:%M:%S%p") # loop over the frames a second time for (frame, name) in zip(frames, ("Webcam", "Picamera")): # draw the timestamp on the frame and display it cv2.putText(frame, ts, (10, frame.shape[0] - 10), cv2.FONT_HERSHEY_SIMPLEX, 0.35, (0, 0, 255), 1) cv2.imshow(name, frame) # check to see if a key was pressed key = cv2.waitKey(1) & 0xFF # if the `q` key was pressed, break from the loop if key == ord("q"): break # do a bit of cleanup print("[INFO] cleaning up...") cv2.destroyAllWindows() webcam.stop() picam.stop()
Liens 70-72 increments the
totalnumber of frames processed, followed by grabbing and formatting the current timestamp.
We then loop over each of the
frameswe have processed for motion on Line 75 and display them to our screen.
Finally, Lines 82-86 check to see if the
qkey is pressed, indicating that we should break from the frame reading loop. Lines 89-92 then perform a bit of cleanup.
Motion detection on the Raspberry Pi with multiple cameras
To see our multiple camera motion detector run on the Raspberry Pi, just execute the following command:
$ python multi_cam_motion.py
I have included a series of “highlight frames” in the following GIF that demonstrate our multi-camera motion detector in action:
Figure 4: An example of applying motion detection to multiple cameras using the Raspberry Pi, OpenCV, and Python.
Notice how I start in the kitchen, open a cabinet, reach for a mug, and head to the sink to fill the mug up with water — this series of actions and motion are detected on the first camera.
Finally, I head to the trash can to throw out a paper towel before exiting the frame view of the second camera.
A full video demo of multiple camera access using the Raspberry Pi can be seen below:
Summary
In this blog post, we learned how to access multiple cameras using the Raspberry Pi 2, OpenCV, and Python.
When accessing multiple cameras on the Raspberry Pi, you have two choices when constructing your setup:
- Either use multiple USB webcams.
- Or using a single Raspberry Pi camera module and at least one USB webcam.
Since the Raspberry Pi board has only one camera input, you cannot leverage multiple Pi camera boards — atleast without extensive hacks to your Pi.
In order to provide an interesting implementation of multiple camera access with the Raspberry Pi, we created a simple motion detection class that can be used to detect motion in the frame views of each camera connected to the Pi.
While basic, this motion detector demonstrated that multiple camera access is capable of being executed in real-time on the Raspberry Pi — especially with the help of our threaded
PiVideoStreamand
VideoStreamclasses implemented in blog posts a few weeks ago.
If you are interested in learning more about using the Raspberry Pi for computer vision, along with other tips, tricks, and hacks related to OpenCV, be sure to signup for the PyImageSearch Newsletter using the form at the bottom of this post.
See you next week!
Downloads:
The post Multiple cameras with the Raspberry Pi and OpenCV appeared first on PyImageSearch.
from PyImageSearch http://ift.tt/1OAU739
via IFTTT