Latest YouTube Video
Saturday, March 5, 2016
Anonymous User
from Google Alert - anonymous http://ift.tt/1UJeNfx
via IFTTT
I have a new follower on Twitter
Fractal US
Fractalerts was founded by a group of traders with a passion for math, markets and money. Prepare...don't react. Indices, Commodities and Forex
http://t.co/KHp2AU2oHi
Following: 4855 - Followers: 13262
March 05, 2016 at 04:28PM via Twitter http://twitter.com/FA_USIndices
Reinstall anonymous authentication method
from Google Alert - anonymous http://ift.tt/1YfIOTW
via IFTTT
Sculptor Galaxy NGC 134
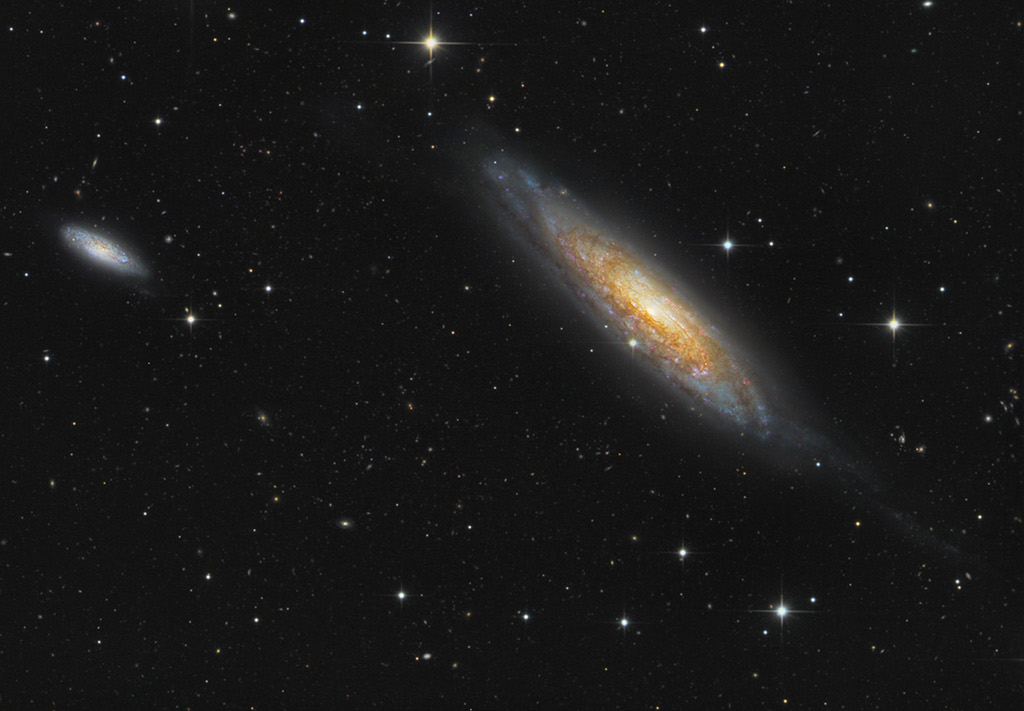
JPSS Multi Mission Concept of Operations
from NASA's Scientific Visualization Studio: Most Recent Items http://ift.tt/1YdDiRQ
via IFTTT
Friday, March 4, 2016
Heart Warmers knit anonymous gifts for newborn babies
from Google Alert - anonymous http://ift.tt/1Ydf2iJ
via IFTTT
Ravens: OL Kelechi Osemele the one free agent Baltimore must sign - Jamison Hensley; already extended \"aggressive\" offer (ESPN)
via IFTTT
How to Steal Secret Encryption Keys from Android and iOS SmartPhones
from The Hacker News http://ift.tt/1LEW0jl
via IFTTT
I have a new follower on Twitter
Eternal Fat Kid
You gonna finish those fries? TV writer
Following: 7594 - Followers: 10761
March 04, 2016 at 11:45AM via Twitter http://twitter.com/EternalFatKid
NFL: Ravens LB Terrell Suggs arrested for a suspended license Friday in Arizona after one-car collision with no injuries (ESPN)
via IFTTT
ISS Daily Summary Report – 03/3/16
from ISS On-Orbit Status Report http://ift.tt/21bnGhE
via IFTTT
Subgraph OS — Secure Linux Operating System for Non-Technical Users
from The Hacker News http://ift.tt/1TXUCJU
via IFTTT
Is there a way to switch off jsdoc enforceExistence for anonymous arrow functions?
from Google Alert - anonymous http://ift.tt/1UDZ8On
via IFTTT
Funds Donors showing as anonymous
from Google Alert - anonymous http://ift.tt/1RMrKlO
via IFTTT
Moons and Jupiter
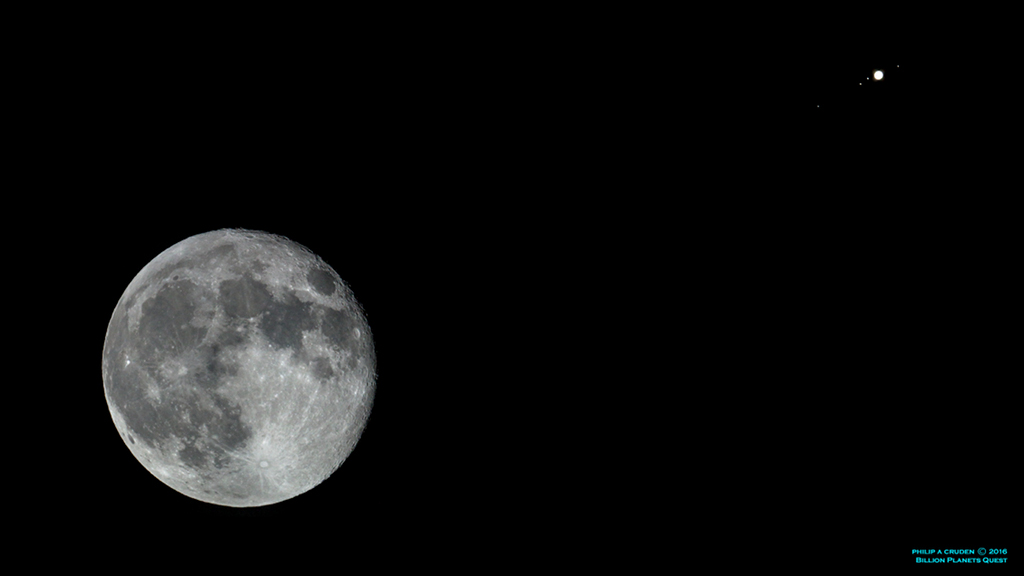
[FD] Hacking Magento eCommerce For Fun And 17.000 USD
Source: Gmail -> IFTTT-> Blogger
Thursday, March 3, 2016
[FD] Hacking Magento eCommerce For Fun And 17.000 USD
Source: Gmail -> IFTTT-> Blogger
Learning Tabletop Object Manipulation by Imitation. (arXiv:1603.00964v1 [cs.RO])
We aim to enable robot to learn tabletop object manipulation by imitation. Given external observations of demonstrations on object manipulations, we believe that two underlying problems to address in learning by imitation is 1) segment a given demonstration into skills that can be individually learned and reused, and 2) formulate the correct RL (Reinforcement Learning) problem that only considers the relevant aspects of each skill so that the policy for each skill can be effectively learned. Previous works made certain progress in this direction, but none has taken private information into account. The public information is the information that is available in the external observations of demonstration, and the private information is the information that are only available to the agent that executes the actions, such as tactile sensations. Our contribution is that we provide a method for the robot to automatically segment the demonstration into multiple skills, and formulate the correct RL problem for each skill, and automatically decide whether the private information is an important aspect of each skill based on interaction with the world. Our motivating example is for a real robot to play the shape sorter game by imitating other's behavior, and we will show the results in a simulated 2D environment that captures the important properties of the shape sorter game. The evaluation is based on whether the demonstration is reasonably segmented, and whether the correct RL problems are formulated. In the end, we will show that robot can imitate the demonstrated behavior based on learned policies.
from cs.AI updates on arXiv.org http://ift.tt/1oTnW8P
via IFTTT
Automatic learning of gait signatures for people identification. (arXiv:1603.01006v1 [cs.CV])
This work targets people identification in video based on the way they walk (i.e. gait). While classical methods typically derive gait signatures from sequences of binary silhouettes, in this work we explore the use of convolutional neural networks (CNN) for learning high-level descriptors from low-level motion features (i.e. optical flow components). We carry out a thorough experimental evaluation of the proposed CNN architecture on the challenging TUM-GAID dataset. The experimental results indicate that using spatio-temporal cuboids of optical flow as input data for CNN allows to obtain state-of-the-art results on the gait task with an image resolution eight times lower than the previously reported results (i.e. 80x60 pixels).
from cs.AI updates on arXiv.org http://ift.tt/1QWDHZm
via IFTTT
Modeling the Sequence of Brain Volumes by Local Mesh Models for Brain Decoding. (arXiv:1603.01067v1 [cs.LG])
We represent the sequence of fMRI (Functional Magnetic Resonance Imaging) brain volumes recorded during a cognitive stimulus by a graph which consists of a set of local meshes. The corresponding cognitive process, encoded in the brain, is then represented by these meshes each of which is estimated assuming a linear relationship among the voxel time series in a predefined locality. First, we define the concept of locality in two neighborhood systems, namely, the spatial and functional neighborhoods. Then, we construct spatially and functionally local meshes around each voxel, called seed voxel, by connecting it either to its spatial or functional p-nearest neighbors. The mesh formed around a voxel is a directed sub-graph with a star topology, where the direction of the edges is taken towards the seed voxel at the center of the mesh. We represent the time series recorded at each seed voxel in terms of linear combination of the time series of its p-nearest neighbors in the mesh. The relationships between a seed voxel and its neighbors are represented by the edge weights of each mesh, and are estimated by solving a linear regression equation. The estimated mesh edge weights lead to a better representation of information in the brain for encoding and decoding of the cognitive tasks. We test our model on a visual object recognition and emotional memory retrieval experiments using Support Vector Machines that are trained using the mesh edge weights as features. In the experimental analysis, we observe that the edge weights of the spatial and functional meshes perform better than the state-of-the-art brain decoding models.
from cs.AI updates on arXiv.org http://ift.tt/1oTnW8G
via IFTTT
Deep Reinforcement Learning from Self-Play in Imperfect-Information Games. (arXiv:1603.01121v1 [cs.LG])
Many real-world applications can be described as large-scale games of imperfect information. To deal with these challenging domains, prior work has focused on computing Nash equilibria in a handcrafted abstraction of the domain. In this paper we introduce the first scalable end-to-end approach to learning approximate Nash equilibria without any prior knowledge. Our method combines fictitious self-play with deep reinforcement learning. When applied to Leduc poker, Neural Fictitious Self-Play (NFSP) approached a Nash equilibrium, whereas common reinforcement learning methods diverged. In Limit Texas Holdem, a poker game of real-world scale, NFSP learnt a competitive strategy that approached the performance of human experts and state-of-the-art methods.
from cs.AI updates on arXiv.org http://ift.tt/1QWDK7A
via IFTTT
Network Unfolding Map by Edge Dynamics Modeling. (arXiv:1603.01182v1 [cs.AI])
The emergence of collective dynamics in neural networks is a mechanism of the animal and human brain for information processing. In this paper, we develop a computational technique of distributed processing elements, which are called particles. We observe the collective dynamics of particles in a complex network for transductive inference on semi-supervised learning problems. Three actions govern the particles' dynamics: walking, absorption, and generation. Labeled vertices generate new particles that compete against rival particles for edge domination. Active particles randomly walk in the network until they are absorbed by either a rival vertex or an edge currently dominated by rival particles. The result from the model simulation consists of sets of edges sorted by the label dominance. Each set tends to form a connected subnetwork to represent a data class. Although the intrinsic dynamics of the model is a stochastic one, we prove there exists a deterministic version with largely reduced computational complexity; specifically, with subquadratic growth. Furthermore, the edge domination process corresponds to an unfolding map. Intuitively, edges "stretch" and "shrink" according to edge dynamics. Consequently, such effect summarizes the relevant relationships between vertices and uncovered data classes. The proposed model captures important details of connectivity patterns over the edge dynamics evolution, which contrasts with previous approaches focused on vertex dynamics. Computer simulations reveal that our model can identify nonlinear features in both real and artificial data, including boundaries between distinct classes and the overlapping structure of data.
from cs.AI updates on arXiv.org http://ift.tt/1oTnVS6
via IFTTT
GeoGebra Tools with Proof Capabilities. (arXiv:1603.01228v1 [cs.AI])
We report about significant enhancements of the complex algebraic geometry theorem proving subsystem in GeoGebra for automated proofs in Euclidean geometry, concerning the extension of numerous GeoGebra tools with proof capabilities. As a result, a number of elementary theorems can be proven by using GeoGebra's intuitive user interface on various computer architectures including native Java and web based systems with JavaScript. We also provide a test suite for benchmarking our results with 200 test cases.
from cs.AI updates on arXiv.org http://ift.tt/1QWDHZf
via IFTTT
Decision Forests, Convolutional Networks and the Models in-Between. (arXiv:1603.01250v1 [cs.CV])
This paper investigates the connections between two state of the art classifiers: decision forests (DFs, including decision jungles) and convolutional neural networks (CNNs). Decision forests are computationally efficient thanks to their conditional computation property (computation is confined to only a small region of the tree, the nodes along a single branch). CNNs achieve state of the art accuracy, thanks to their representation learning capabilities. We present a systematic analysis of how to fuse conditional computation with representation learning and achieve a continuum of hybrid models with different ratios of accuracy vs. efficiency. We call this new family of hybrid models conditional networks. Conditional networks can be thought of as: i) decision trees augmented with data transformation operators, or ii) CNNs, with block-diagonal sparse weight matrices, and explicit data routing functions. Experimental validation is performed on the common task of image classification on both the CIFAR and Imagenet datasets. Compared to state of the art CNNs, our hybrid models yield the same accuracy with a fraction of the compute cost and much smaller number of parameters.
from cs.AI updates on arXiv.org http://ift.tt/1oTnVBJ
via IFTTT
[FD] [CFP] EuskalHack (San Sebastian / Donostia) 2016
Source: Gmail -> IFTTT-> Blogger
[FD] Vulnerabilities in Mobile Safari
Source: Gmail -> IFTTT-> Blogger
[FD] CVE Request: Fiyo CMS 2.0.6.1 - Multiple XSS Vulnerabilities
Source: Gmail -> IFTTT-> Blogger
[FD] Panda SM Manager iOS Application - MITM SSL Certificate Vulnerability
Source: Gmail -> IFTTT-> Blogger
[FD] Browser Security Tool: HTTPS Only 2.1 (Major Release, Open Source, Python)
Source: Gmail -> IFTTT-> Blogger
Tu me comande (Anonymous)
from Google Alert - anonymous http://ift.tt/1UCRF29
via IFTTT
[FD] Vipps by DNB for Android - cryptographic vulnerabilities
Source: Gmail -> IFTTT-> Blogger
Anonymous tosses sonic bomb in Ain el Helweh camp
from Google Alert - anonymous http://ift.tt/1WXIUyp
via IFTTT
Ravens: Team declines option on DE Chris Canty's contract, making him free agent; spent 3 seasons in Baltimore (ESPN)
via IFTTT
Ravens Video: Skip Bayless calls Joe Flacco \"just pretty good\"; \"outrageous\" contract makes him most overpaid QB in NFL (ESPN)
via IFTTT
I have a new follower on Twitter
Trending Facts
Factoids surrounding the trends of Twitter
United States
Following: 2891 - Followers: 335
March 03, 2016 at 12:36PM via Twitter http://twitter.com/TrendingFactoid
Ravens: LB Daryl Smith released, freeing up $2.625M in cap space - Adam Schefter; 12-year veteran has career 30.5 sacks (ESPN)
via IFTTT
University Takes Steps to Address Anonymous Racial Comments
from Google Alert - anonymous http://ift.tt/1VSDN2f
via IFTTT
Suspected hit-and-run driver arrested following anonymous tip
from Google Alert - anonymous http://ift.tt/1QVnbcb
via IFTTT
Hack the Pentagon — US Government Challenges Hackers to Break its Security
from The Hacker News http://ift.tt/21Jtxg8
via IFTTT
Can Scientists 'Upload Knowledge' Directly into your Brain to Teach New Skills?
from The Hacker News http://ift.tt/1UATkVW
via IFTTT
Unusual Clouds over Hong Kong
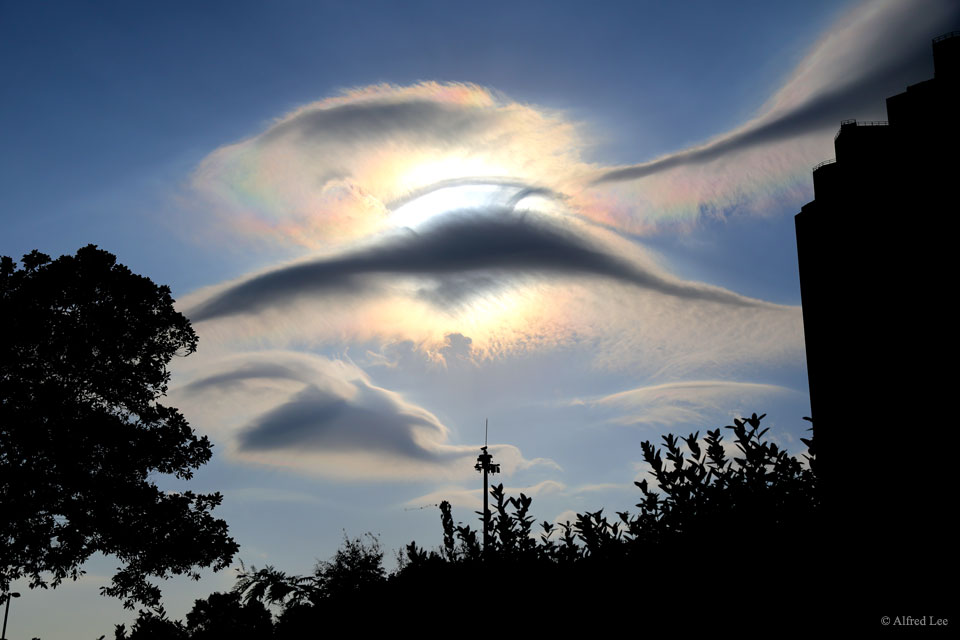
Cyclone Winston Slams Fiji (February 20, 2016)
from NASA's Scientific Visualization Studio: Most Recent Items http://ift.tt/21Fagjm
via IFTTT
Wednesday, March 2, 2016
Mask, Anonymous, Network
from Google Alert - anonymous http://ift.tt/1TRIDyR
via IFTTT
Probabilistic Relational Model Benchmark Generation. (arXiv:1603.00709v1 [cs.LG])
The validation of any database mining methodology goes through an evaluation process where benchmarks availability is essential. In this paper, we aim to randomly generate relational database benchmarks that allow to check probabilistic dependencies among the attributes. We are particularly interested in Probabilistic Relational Models (PRMs), which extend Bayesian Networks (BNs) to a relational data mining context and enable effective and robust reasoning over relational data. Even though a panoply of works have focused, separately , on the generation of random Bayesian networks and relational databases, no work has been identified for PRMs on that track. This paper provides an algorithmic approach for generating random PRMs from scratch to fill this gap. The proposed method allows to generate PRMs as well as synthetic relational data from a randomly generated relational schema and a random set of probabilistic dependencies. This can be of interest not only for machine learning researchers to evaluate their proposals in a common framework, but also for databases designers to evaluate the effectiveness of the components of a database management system.
from cs.AI updates on arXiv.org http://ift.tt/1RqexN5
via IFTTT
Continuous Deep Q-Learning with Model-based Acceleration. (arXiv:1603.00748v1 [cs.LG])
Model-free reinforcement learning has been successfully applied to a range of challenging problems, and has recently been extended to handle large neural network policies and value functions. However, the sample complexity of model-free algorithms, particularly when using high-dimensional function approximators, tends to limit their applicability to physical systems. In this paper, we explore algorithms and representations to reduce the sample complexity of deep reinforcement learning for continuous control tasks. We propose two complementary techniques for improving the efficiency of such algorithms. First, we derive a continuous variant of the Q-learning algorithm, which we call normalized adantage functions (NAF), as an alternative to the more commonly used policy gradient and actor-critic methods. NAF representation allows us to apply Q-learning with experience replay to continuous tasks, and substantially improves performance on a set of simulated robotic control tasks. To further improve the efficiency of our approach, we explore the use of learned models for accelerating model-free reinforcement learning. We show that iteratively refitted local linear models are especially effective for this, and demonstrate substantially faster learning on domains where such models are applicable.
from cs.AI updates on arXiv.org http://ift.tt/24Cv0aD
via IFTTT
Filter based Taxonomy Modification for Improving Hierarchical Classification. (arXiv:1603.00772v1 [cs.AI])
Large scale classification of data organized as a hierarchy of classes has received significant attention in the literature. Top-Down (TD) Hierarchical Classification (HC), which exploits the hierarchical structure during the learning process is an effective method for dealing with problems at scale due to its computational benefits. However, its accuracy suffers due to error propagation i.e., prediction errors made at higher levels in the hierarchy cannot be corrected at lower levels. One of the main reasons behind errors at the higher levels is the presence of inconsistent nodes and links that are introduced due to the arbitrary process of creating these hierarchies by domain experts. In this paper, we propose two efficient data driven filter based approaches for hierarchical structure modification: (i) Flattening (local and global) approach that identifies and removes inconsistent nodes present within the hierarchy and (ii) Rewiring approach modifies parent-child relationships to improve the classification performance of learned models. Our extensive empirical evaluation of the proposed approaches on several image and text datasets shows improved performance over competing approaches.
from cs.AI updates on arXiv.org http://ift.tt/1Rqezo1
via IFTTT
Automatic Differentiation Variational Inference. (arXiv:1603.00788v1 [stat.ML])
Probabilistic modeling is iterative. A scientist posits a simple model, fits it to her data, refines it according to her analysis, and repeats. However, fitting complex models to large data is a bottleneck in this process. Deriving algorithms for new models can be both mathematically and computationally challenging, which makes it difficult to efficiently cycle through the steps. To this end, we develop automatic differentiation variational inference (ADVI). Using our method, the scientist only provides a probabilistic model and a dataset, nothing else. ADVI automatically derives an efficient variational inference algorithm, freeing the scientist to refine and explore many models. ADVI supports a broad class of models-no conjugacy assumptions are required. We study ADVI across ten different models and apply it to a dataset with millions of observations. ADVI is integrated into Stan, a probabilistic programming system; it is available for immediate use.
from cs.AI updates on arXiv.org http://ift.tt/24CuXLZ
via IFTTT
Hybrid Collaborative Filtering with Neural Networks Romaric Gaudel. (arXiv:1603.00806v1 [cs.IR])
Collaborative Filtering aims at exploiting the feedback of users to provide personalised recommendations. Such algorithms look for latent variables in a large sparse matrix of ratings. They can be enhanced by adding side information to tackle the well-known cold start problem. While Neu-ral Networks have tremendous success in image and speech recognition, they have received less attention in Collaborative Filtering. This is all the more surprising that Neural Networks are able to discover latent variables in large and heterogeneous datasets. In this paper, we introduce a Collaborative Filtering Neural network architecture aka CFN which computes a non-linear Matrix Factorization from sparse rating inputs and side information. We show experimentally on the MovieLens and Douban dataset that CFN outper-forms the state of the art and benefits from side information. We provide an implementation of the algorithm as a reusable plugin for Torch, a popular Neural Network framework.
from cs.AI updates on arXiv.org http://ift.tt/1Rqez7D
via IFTTT
Finding Preference Profiles of Condorcet Dimension $k$ via SAT. (arXiv:1402.4303v2 [cs.MA] UPDATED)
Condorcet winning sets are a set-valued generalization of the well-known concept of a Condorcet winner. As supersets of Condorcet winning sets are always Condorcet winning sets themselves, an interesting property of preference profiles is the size of the smallest Condorcet winning set they admit. This smallest size is called the Condorcet dimension of a preference profile. Since little is known about profiles that have a certain Condorcet dimension, we show in this paper how the problem of finding a preference profile that has a given Condorcet dimension can be encoded as a satisfiability problem and solved by a SAT solver. Initial results include a minimal example of a preference profile of Condorcet dimension 3, improving previously known examples both in terms of the number of agents as well as alternatives. Due to the high complexity of such problems it remains open whether a preference profile of Condorcet dimension 4 exists.
from cs.AI updates on arXiv.org http://ift.tt/1m7I3cW
via IFTTT
Belief and Truth in Hypothesised Behaviours. (arXiv:1507.07688v3 [cs.AI] UPDATED)
There is a long history in game theory on the topic of Bayesian or "rational" learning, in which each player maintains beliefs over a set of alternative behaviours, or types, for the other players. This idea has gained increasing interest in the artificial intelligence (AI) community, where it is used as a method to control a single agent in a system composed of multiple agents with unknown behaviours. The idea is to hypothesise a set of types, each specifying a possible behaviour for the other agents, and to plan our own actions with respect to those types which we believe are most likely, given the observed actions of the agents. The game theory literature studies this idea primarily in the context of equilibrium attainment. In contrast, many AI applications have a focus on task completion and payoff maximisation. With this perspective in mind, we identify and address a spectrum of questions pertaining to belief and truth in hypothesised types. We formulate three basic ways to incorporate evidence into posterior beliefs and show when the resulting beliefs are correct, and when they may fail to be correct. Moreover, we demonstrate that prior beliefs can have a significant impact on our ability to maximise payoffs in the long-term, and that they can be computed automatically with consistent performance effects. Furthermore, we analyse the conditions under which we are able complete our task optimally, despite inaccuracies in the hypothesised types. Finally, we show how the correctness of hypothesised types can be ascertained during the interaction via an automated statistical analysis.
from cs.AI updates on arXiv.org http://ift.tt/1JQl9k3
via IFTTT
Viagra Anonymous
from Google Alert - anonymous http://ift.tt/24Cgkbs
via IFTTT
Ravens Video: Joe Flacco's extension \"only solution\" to cap issues - Field Yates; says QB has better timing than anyone (ESPN)
via IFTTT
France could Fine Apple $1 Million for each iPhone it Refuses to Unlock
from The Hacker News http://ift.tt/1RoSwht
via IFTTT
Ravens: QB Joe Flacco's 3-year contract extension is worth $66.4M with $44M fully guaranteed, source tells Adam Caplan (ESPN)
via IFTTT
Turing Award — Inventors of Modern Cryptography Win $1 Million Cash Prize
from The Hacker News http://ift.tt/1oPEcaL
via IFTTT
Kanye West, Who wants to destroy ‘The Pirate Bay’, Caught using Torrent Site
from The Hacker News http://ift.tt/1OPVIAN
via IFTTT
Ravens: Notre Dame OT Ronnie Stanley goes No. 6 in Todd McShay's Mock Draft 3.0; RB Ezekiel Elliot is also an option (ESPN)
via IFTTT
NFL: QB Joe Flacco agrees with Ravens to 3-year contract extension through 2021 - Caplan; tore ACL, MCL in 2015 Week 11 (ESPN)
via IFTTT
Pour vous servir belle dame (Anonymous)
from Google Alert - anonymous http://ift.tt/1oYoivy
via IFTTT
Um anjo à Virgem Santa (Anonymous)
from Google Alert - anonymous http://ift.tt/1VQgBSi
via IFTTT
ISS Daily Summary Report – 03/1/16
from ISS On-Orbit Status Report http://ift.tt/1Qr2RK6
via IFTTT
Survive France Network (Anonymous) Suggestion Box
from Google Alert - anonymous http://ift.tt/1RGkpEw
via IFTTT
anonymous,uncategorized,misc,general,other
from Google Alert - anonymous http://ift.tt/1oYhwWt
via IFTTT
FBI Admits — It was a 'Mistake' to Reset Terrorist's iCloud Password
from The Hacker News http://ift.tt/1LwIwpZ
via IFTTT
I have a new follower on Twitter
MelnikovFlorenc1958
Get Handmade word press website Design only in $249. call +1-800-219-0366 visit: https://t.co/3o7Ek7tTLZ
San Jose, CA
https://t.co/3o7Ek7tTLZ
Following: 373 - Followers: 6
March 02, 2016 at 05:59AM via Twitter http://twitter.com/florenc1958
I have a new follower on Twitter
Go Pro Football
Pro Club #footballtrials MARCH 2016!!!ENGLISH & EUROPEAN FOOTBALL LEAGUE SCOUTS! Go Pro today & book YOUR pro club trial @ https://t.co/2sXwxTrzJX #GoPro
United Kingdom
https://t.co/2sXwxTrzJX
Following: 4807 - Followers: 5490
March 02, 2016 at 05:23AM via Twitter http://twitter.com/GoProFootball
Voglio siscà (Anonymous)
from Google Alert - anonymous http://ift.tt/1TRe8I7
via IFTTT
FBI Director — "What If Apple Engineers are Kidnapped and Forced to Write (Exploit) Code?"
from The Hacker News http://ift.tt/1QqHYPb
via IFTTT
Creator alme siderum
from Google Alert - anonymous http://ift.tt/1LUbKtC
via IFTTT
Qui es Ane
from Google Alert - anonymous http://ift.tt/1TQPKXb
via IFTTT
NGC 3310: A Starburst Spiral Galaxy
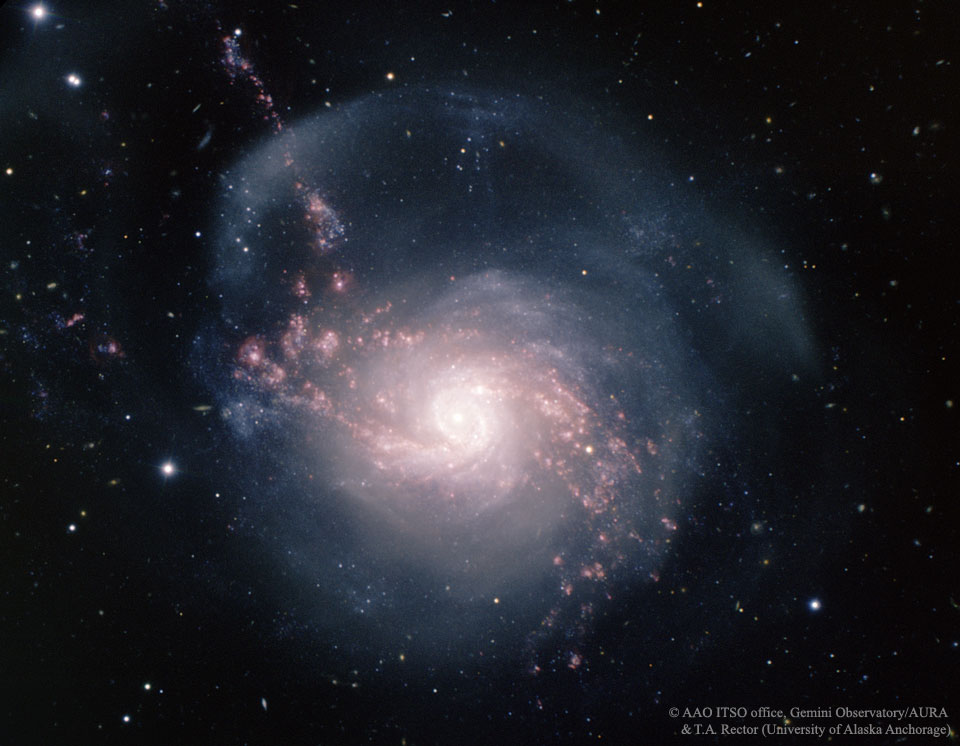
Tuesday, March 1, 2016
Quantifying the vanishing gradient and long distance dependency problem in recursive neural networks and recursive LSTMs. (arXiv:1603.00423v1 [cs.AI])
Recursive neural networks (RNN) and their recently proposed extension recursive long short term memory networks (RLSTM) are models that compute representations for sentences, by recursively combining word embeddings according to an externally provided parse tree. Both models thus, unlike recurrent networks, explicitly make use of the hierarchical structure of a sentence. In this paper, we demonstrate that RNNs nevertheless suffer from the vanishing gradient and long distance dependency problem, and that RLSTMs greatly improve over RNN's on these problems. We present an artificial learning task that allows us to quantify the severity of these problems for both models. We further show that a ratio of gradients (at the root node and a focal leaf node) is highly indicative of the success of backpropagation at optimizing the relevant weights low in the tree. This paper thus provides an explanation for existing, superior results of RLSTMs on tasks such as sentiment analysis, and suggests that the benefits of including hierarchical structure and of including LSTM-style gating are complementary.
from cs.AI updates on arXiv.org http://ift.tt/1QK3xJO
via IFTTT
Guided Cost Learning: Deep Inverse Optimal Control via Policy Optimization. (arXiv:1603.00448v1 [cs.LG])
Reinforcement learning can acquire complex behaviors from high-level specifications. However, defining a cost function that can be optimized effectively and encodes the correct task is challenging in practice. We explore how inverse optimal control (IOC) can be used to learn behaviors from demonstrations, with applications to torque control of high-dimensional robotic systems. Our method addresses two key challenges in inverse optimal control: first, the need for informative features and effective regularization to impose structure on the cost, and second, the difficulty of learning the cost function under unknown dynamics for high-dimensional continuous systems. To address the former challenge, we present an algorithm capable of learning arbitrary nonlinear cost functions, such as neural networks, without meticulous feature engineering. To address the latter challenge, we formulate an efficient sample-based approximation for MaxEnt IOC. We evaluate our method on a series of simulated tasks and real-world robotic manipulation problems, demonstrating substantial improvement over prior methods both in terms of task complexity and sample efficiency.
from cs.AI updates on arXiv.org http://ift.tt/1UxsLB5
via IFTTT
Reasoning about Entailment with Neural Attention. (arXiv:1509.06664v4 [cs.CL] UPDATED)
While most approaches to automatically recognizing entailment relations have used classifiers employing hand engineered features derived from complex natural language processing pipelines, in practice their performance has been only slightly better than bag-of-word pair classifiers using only lexical similarity. The only attempt so far to build an end-to-end differentiable neural network for entailment failed to outperform such a simple similarity classifier. In this paper, we propose a neural model that reads two sentences to determine entailment using long short-term memory units. We extend this model with a word-by-word neural attention mechanism that encourages reasoning over entailments of pairs of words and phrases. Furthermore, we present a qualitative analysis of attention weights produced by this model, demonstrating such reasoning capabilities. On a large entailment dataset this model outperforms the previous best neural model and a classifier with engineered features by a substantial margin. It is the first generic end-to-end differentiable system that achieves state-of-the-art accuracy on a textual entailment dataset.
from cs.AI updates on arXiv.org http://ift.tt/1NRZGOz
via IFTTT
Facebook's Vice President Arrested in Brazil for Refusing to Share WhatsApp Data
from The Hacker News http://ift.tt/1QqqWE4
via IFTTT
DROWN Attack — More than 11 Million OpenSSL HTTPS Websites at Risk
from The Hacker News http://ift.tt/1OMroXK
via IFTTT
When an anonymous user submits a form with an un-uploaded file that
from Google Alert - anonymous http://ift.tt/1oMyKFu
via IFTTT
Rorate, coeli, desuper
from Google Alert - anonymous http://ift.tt/1UwwUoM
via IFTTT
New York Judge Rules FBI Can't Force Apple to Unlock iPhone
from The Hacker News http://ift.tt/1QISeS0
via IFTTT
Ravens: WR Steve Smith (torn Achilles) confident he will be ready for start of season; \"No setbacks. No real pain\" (ESPN)
via IFTTT
ISS Daily Summary Report – 02/29/16
from ISS On-Orbit Status Report http://ift.tt/1TO7EcZ
via IFTTT
I have a new follower on Twitter
Tara Reed
Founder @Kollecto. I like to build software without writing any code. Former Googler, Foursquarer & Microsoftie.
Detroit
https://t.co/oUf60ea0Uu
Following: 657 - Followers: 5345
March 01, 2016 at 04:10AM via Twitter http://twitter.com/TaraReed_
I have a new follower on Twitter
Issac Avila
Research Associate @internetresearc #internetresearch #marketresearch #research #academicresearch #onlineresearch #researchmethods
United States
https://t.co/kWRfUZ6xBV
Following: 712 - Followers: 76
March 01, 2016 at 02:47AM via Twitter http://twitter.com/researchassocit
Julius Caesar and Leap Days
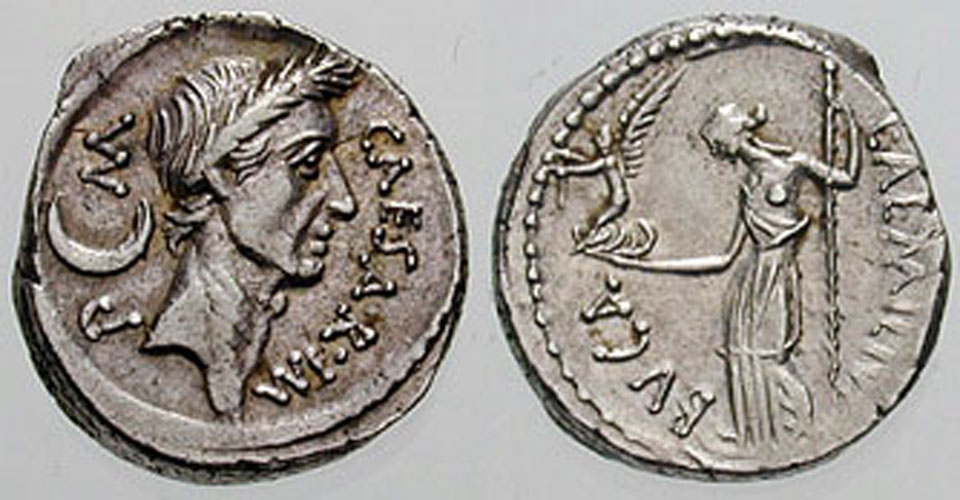
Monday, February 29, 2016
Towards Neural Knowledge DNA. (arXiv:1602.08571v1 [cs.AI])
In this paper, we propose the Neural Knowledge DNA, a framework that tailors the ideas underlying the success of neural networks to the scope of knowledge representation. Knowledge representation is a fundamental field that dedicate to representing information about the world in a form that computer systems can utilize to solve complex tasks. The proposed Neural Knowledge DNA is designed to support discovering, storing, reusing, improving, and sharing knowledge among machines and organisation. It is constructed in a similar fashion of how DNA formed: built up by four essential elements. As the DNA produces phenotypes, the Neural Knowledge DNA carries information and knowledge via its four essential elements, namely, Networks, Experiences, States, and Actions.
from cs.AI updates on arXiv.org http://ift.tt/1XWzl3G
via IFTTT
Scalable Bayesian Rule Lists. (arXiv:1602.08610v1 [cs.AI])
We present an algorithm for building rule lists that is two orders of magnitude faster than previous work. Rule list algorithms are competitors for decision tree algorithms. They are associative classifiers, in that they are built from pre-mined association rules. They have a logical structure that is a sequence of IF-THEN rules, identical to a decision list or one-sided decision tree. Instead of using greedy splitting and pruning like decision tree algorithms, we fully optimize over rule lists, striking a practical balance between accuracy, interpretability, and computational speed. The algorithm presented here uses a mixture of theoretical bounds (tight enough to have practical implications as a screening or bounding procedure), computational reuse, and highly tuned language libraries to achieve computational efficiency. Currently, for many practical problems, this method achieves better accuracy and sparsity than decision trees; further, in many cases, the computational time is practical and often less than that of decision trees.
from cs.AI updates on arXiv.org http://ift.tt/1oUm0xs
via IFTTT
Lie Access Neural Turing Machine. (arXiv:1602.08671v1 [cs.NE])
Recently, Neural Turing Machine and Memory Networks have shown that adding an external memory can greatly ameliorate a traditional recurrent neural network's tendency to forget after a long period of time. Here we present a new design of an external memory, wherein memories are stored in an Euclidean key space $\mathbb R^n$. An LSTM controller performs read and write via specialized structures called read and write heads, following the design of Neural Turing Machine. It can move a head by either providing a new address in the key space (aka random access) or moving from its previous position via a Lie group action (aka Lie access). In this way, the "L" and "R" instructions of a traditional Turing Machine is generalized to arbitrary elements of a fixed Lie group action. For this reason, we name this new model the Lie Access Neural Turing Machine, or LANTM.
We tested two different configurations of LANTM against an LSTM baseline in several basic experiments. As LANTM is differentiable end-to-end, training was done with RMSProp. We found the right configuration of LANTM to be capable of learning different permutation and arithmetic tasks and extrapolating to at least twice the input size, all with the number of parameters 2 orders of magnitude below that for the LSTM baseline. In particular, we trained LANTM on addition of $k$-digit numbers for $2 \le k \le 16$, but it was able to generalize almost perfectly to $17 \le k \le 32$.
from cs.AI updates on arXiv.org http://ift.tt/1XWzjsL
via IFTTT
Investigating practical, linear temporal difference learning. (arXiv:1602.08771v1 [cs.LG])
Off-policy reinforcement learning has many applications including: learning from demonstration, learning multiple goal seeking policies in parallel, and representing predictive knowledge. Recently there has been an proliferation of new policy-evaluation algorithms that fill a longstanding algorithmic void in reinforcement learning: combining robustness to off-policy sampling, function approximation, linear complexity, and temporal difference (TD) updates. This paper contains two main contributions. First, we derive two new hybrid TD policy-evaluation algorithms, which fill a gap in this collection of algorithms. Second, we perform an empirical comparison to elicit which of these new linear TD methods should be preferred in different situations, and make concrete suggestions about practical use.
from cs.AI updates on arXiv.org http://ift.tt/1TMGfIj
via IFTTT
Range-based argumentation semantics as 2-valued models. (arXiv:1602.08903v1 [cs.LO])
Characterizations of semi-stable and stage extensions in terms of 2-valued logical models are presented. To this end, the so-called GL-supported and GL-stage models are defined. These two classes of logical models are logic programming counterparts of the notion of range which is an established concept in argumentation semantics.
from cs.AI updates on arXiv.org http://ift.tt/1oUlYWl
via IFTTT
Personalized and situation-aware multimodal route recommendations: the FAVOUR algorithm. (arXiv:1602.09076v1 [cs.AI])
Route choice in multimodal networks shows a considerable variation between different individuals as well as the current situational context. Personalization of recommendation algorithms are already common in many areas, e.g., online retail. However, most online routing applications still provide shortest distance or shortest travel-time routes only, neglecting individual preferences as well as the current situation. Both aspects are of particular importance in a multimodal setting as attractivity of some transportation modes such as biking crucially depends on personal characteristics and exogenous factors like the weather. This paper introduces the FAVourite rOUte Recommendation (FAVOUR) approach to provide personalized, situation-aware route proposals based on three steps: first, at the initialization stage, the user provides limited information (home location, work place, mobility options, sociodemographics) used to select one out of a small number of initial profiles. Second, based on this information, a stated preference survey is designed in order to sharpen the profile. In this step a mass preference prior is used to encode the prior knowledge on preferences from the class identified in step one. And third, subsequently the profile is continuously updated during usage of the routing services. The last two steps use Bayesian learning techniques in order to incorporate information from all contributing individuals. The FAVOUR approach is presented in detail and tested on a small number of survey participants. The experimental results on this real-world dataset show that FAVOUR generates better-quality recommendations w.r.t. alternative learning algorithms from the literature. In particular the definition of the mass preference prior for initialization of step two is shown to provide better predictions than a number of alternatives from the literature.
from cs.AI updates on arXiv.org http://ift.tt/1XWzjsE
via IFTTT
Easy Monotonic Policy Iteration. (arXiv:1602.09118v1 [cs.LG])
A key problem in reinforcement learning for control with general function approximators (such as deep neural networks and other nonlinear functions) is that, for many algorithms employed in practice, updates to the policy or $Q$-function may fail to improve performance---or worse, actually cause the policy performance to degrade. Prior work has addressed this for policy iteration by deriving tight policy improvement bounds; by optimizing the lower bound on policy improvement, a better policy is guaranteed. However, existing approaches suffer from bounds that are hard to optimize in practice because they include sup norm terms which cannot be efficiently estimated or differentiated. In this work, we derive a better policy improvement bound where the sup norm of the policy divergence has been replaced with an average divergence; this leads to an algorithm, Easy Monotonic Policy Iteration, that generates sequences of policies with guaranteed non-decreasing returns and is easy to implement in a sample-based framework.
from cs.AI updates on arXiv.org http://ift.tt/1OK3bl0
via IFTTT
Illustrating a neural model of logic computations: The case of Sherlock Holmes' old maxim. (arXiv:1210.7495v3 [q-bio.NC] UPDATED)
Natural languages can express some logical propositions that humans are able to understand. We illustrate this fact with a famous text that Conan Doyle attributed to Holmes: 'It is an old maxim of mine that when you have excluded the impossible, whatever remains, however improbable, must be the truth'. This is a subtle logical statement usually felt as an evident truth. The problem we are trying to solve is the cognitive reason for such a feeling. We postulate here that we accept Holmes' maxim as true because our adult brains are equipped with neural modules that naturally perform modal logical computations.
from cs.AI updates on arXiv.org http://ift.tt/TkYGAI
via IFTTT
Discovering Beaten Paths in Collaborative Ontology-Engineering Projects using Markov Chains. (arXiv:1407.2002v2 [cs.SI] UPDATED)
Biomedical taxonomies, thesauri and ontologies in the form of the International Classification of Diseases (ICD) as a taxonomy or the National Cancer Institute Thesaurus as an OWL-based ontology, play a critical role in acquiring, representing and processing information about human health. With increasing adoption and relevance, biomedical ontologies have also significantly increased in size. For example, the 11th revision of the ICD, which is currently under active development by the WHO contains nearly 50,000 classes representing a vast variety of different diseases and causes of death. This evolution in terms of size was accompanied by an evolution in the way ontologies are engineered. Because no single individual has the expertise to develop such large-scale ontologies, ontology-engineering projects have evolved from small-scale efforts involving just a few domain experts to large-scale projects that require effective collaboration between dozens or even hundreds of experts, practitioners and other stakeholders. Understanding how these stakeholders collaborate will enable us to improve editing environments that support such collaborations. We uncover how large ontology-engineering projects, such as the ICD in its 11th revision, unfold by analyzing usage logs of five different biomedical ontology-engineering projects of varying sizes and scopes using Markov chains. We discover intriguing interaction patterns (e.g., which properties users subsequently change) that suggest that large collaborative ontology-engineering projects are governed by a few general principles that determine and drive development. From our analysis, we identify commonalities and differences between different projects that have implications for project managers, ontology editors, developers and contributors working on collaborative ontology-engineering projects and tools in the biomedical domain.
from cs.AI updates on arXiv.org http://ift.tt/VHixTV
via IFTTT
Better Computer Go Player with Neural Network and Long-term Prediction. (arXiv:1511.06410v3 [cs.LG] UPDATED)
Competing with top human players in the ancient game of Go has been a long-term goal of artificial intelligence. Go's high branching factor makes traditional search techniques ineffective, even on leading-edge hardware, and Go's evaluation function could change drastically with one stone change. Recent works [Maddison et al. (2015); Clark & Storkey (2015)] show that search is not strictly necessary for machine Go players. A pure pattern-matching approach, based on a Deep Convolutional Neural Network (DCNN) that predicts the next move, can perform as well as Monte Carlo Tree Search (MCTS)-based open source Go engines such as Pachi [Baudis & Gailly (2012)] if its search budget is limited. We extend this idea in our bot named darkforest, which relies on a DCNN designed for long-term predictions. Darkforest substantially improves the win rate for pattern-matching approaches against MCTS-based approaches, even with looser search budgets. Against human players, the newest versions, darkfores2, achieve a stable 3d level on KGS Go Server as a ranked bot, a substantial improvement upon the estimated 4k-5k ranks for DCNN reported in Clark & Storkey (2015) based on games against other machine players. Adding MCTS to darkfores2 creates a much stronger player named darkfmcts3: with 5000 rollouts, it beats Pachi with 10k rollouts in all 250 games; with 75k rollouts it achieves a stable 5d level in KGS server, on par with state-of-the-art Go AIs (e.g., Zen, DolBaram, CrazyStone) except for AlphaGo [Silver et al. (2016)]; with 110k rollouts, it won the 3rd place in January KGS Go Tournament.
from cs.AI updates on arXiv.org http://ift.tt/1QCLVEE
via IFTTT
Analysis of Algorithms and Partial Algorithms. (arXiv:1601.03411v2 [cs.AI] UPDATED)
We present an alternative methodology for the analysis of algorithms, based on the concept of expected discounted reward. This methodology naturally handles algorithms that do not always terminate, so it can (theoretically) be used with partial algorithms for undecidable problems, such as those found in artificial general intelligence (AGI) and automated theorem proving. We mention new approaches to self-improving AGI and logical uncertainty enabled by this methodology.
from cs.AI updates on arXiv.org http://ift.tt/1lbZZHF
via IFTTT
Ocean City, MD's surf is at least 5.02ft high
Ocean City, MD Summary
At 2:00 AM, surf min of 0.3ft. At 8:00 AM, surf min of 2.41ft. At 2:00 PM, surf min of 4.1ft. At 8:00 PM, surf min of 5.02ft.
Surf maximum: 6.02ft (1.84m)
Surf minimum: 5.02ft (1.53m)
Tide height: 0.7ft (0.21m)
Wind direction: N
Wind speed: 9.49 KTS
from Surfline http://ift.tt/1kVmigH
via IFTTT
Ocean City, MD's surf is at least 5.44ft high
Ocean City, MD Summary
At 2:00 AM, surf min of 5.44ft. At 8:00 AM, surf min of 5.02ft. At 2:00 PM, surf min of 4.42ft. At 8:00 PM, surf min of 3.71ft.
Surf maximum: 6.45ft (1.96m)
Surf minimum: 5.44ft (1.66m)
Tide height: 2.72ft (0.83m)
Wind direction: N
Wind speed: 4.51 KTS
from Surfline http://ift.tt/1kVmigH
via IFTTT
Je m'en vois (Anonymous)
from Google Alert - anonymous http://ift.tt/1TNcjgH'en_vois_(Anonymous)&ct=ga&cd=CAIyGjgxMzAxNTQ0ZWE3M2NhMmQ6Y29tOmVuOlVT&usg=AFQjCNEgaFf96D42lZwRoIwa8eRCr4N-cQ
via IFTTT
Better anonymous user support
from Google Alert - anonymous http://ift.tt/1QgWdbq
via IFTTT
I have a new follower on Twitter
Flybrix
Play with #robotics & #engineering using Flybrix. It's a programable toy LEGO #drone kit. Comes with a cool app for flight controls and airframe ideas #makerED
https://t.co/tyOpVGU90u
Following: 1804 - Followers: 3469
February 29, 2016 at 11:25AM via Twitter http://twitter.com/Flybrix
[FD] Fing v3.3.0 iOS - Persistent Mail Encoding Vulnerability
Betreff: Fing discovery report for "><img>%20<iframe>%20<iframe src="x"> (No address) |
Von: Benjamin Mejri Kunz <vulnerabilitylab@icloud.com> |
Datum: 28.02.2016 18:38 |
An: bkm@evolution-sec.com |

Von meinem iPad gesendet
Source: Gmail -> IFTTT-> Blogger