Latest YouTube Video
Saturday, October 7, 2017
Anonymous user 45ca52
from Google Alert - anonymous http://ift.tt/2yQ1e6Q
via IFTTT
Data analyst in London | Anonymous Recruiter
from Google Alert - anonymous http://ift.tt/2fSB1gu
via IFTTT
I have a new follower on Twitter
Joseph DeFazio
Founder of @OkayRelaxLLC, web developer, tech lover, vegan, helping kids of the Philippines in my spare time with True Manila.
New York, USA
https://t.co/66kGI499gZ
Following: 1633 - Followers: 1429
October 07, 2017 at 02:12PM via Twitter http://twitter.com/JosephDeFazio
Anonymous donor gives University of Oregon $50 million
from Google Alert - anonymous http://ift.tt/2kwe5sH
via IFTTT
dotnet/roslyn
from Google Alert - anonymous http://ift.tt/2g0KZzX
via IFTTT
ALCOHOLICS ANONYMOUS 208-235-1444 AL-ANON 208-232-2692
from Google Alert - anonymous http://ift.tt/2wEaEBI
via IFTTT
I have a new follower on Twitter
Flor Rose
Drawing is the honesty of the art. There is no possibility of cheating. It is either good or bad.
Chicago, IL
Following: 378 - Followers: 213
October 07, 2017 at 04:22AM via Twitter http://twitter.com/flor_ork
[FD] CVE-2017-13706, Lansweeper 6.0.100.29 XXE Vulnerability
Source: Gmail -> IFTTT-> Blogger
Anonymous No active batch
from Google Alert - anonymous http://ift.tt/2wDG1ME
via IFTTT
Hurricane Tracks from 2017 with Precipitation and Cloud Data
from NASA's Scientific Visualization Studio: Most Recent Items http://ift.tt/2fSS7uM
via IFTTT
Disqus Hacked: More than 17.5 Million Users' Details Stolen in 2012 Breach
from The Hacker News http://ift.tt/2xo48U4
via IFTTT
Friday, October 6, 2017
Enabling Anonymous comments
from Google Alert - anonymous http://ift.tt/2yvdBsK
via IFTTT
University of Oregon receives anonymous $50 million donation
from Google Alert - anonymous http://ift.tt/2xmONTU
via IFTTT
ONE LESS GUN
from Google Alert - anonymous http://ift.tt/2y58mPr
via IFTTT
Anonymous Ferrari owner makes dream come true for young cancer survivor
from Google Alert - anonymous http://ift.tt/2krfJvz
via IFTTT
[FD] Nullcon Goa 2018 Call For Papers is Open!
Source: Gmail -> IFTTT-> Blogger
[FD] ESA-2017-111: RSA Archer® GRC Platform Multiple Vulnerabilities
Source: Gmail -> IFTTT-> Blogger
[FD] ESA-2017-112: EMC Network Configuration Manager Reflected Cross-Site Scripting Vulnerability
Source: Gmail -> IFTTT-> Blogger
[FD] SmartBear SoapUI - Remote Code Execution via Deserialization
Source: Gmail -> IFTTT-> Blogger
[FD] DefenseCode Security Advisory: Magento Commerce CSRF, Stored Cross Site Scripting #2
Source: Gmail -> IFTTT-> Blogger
U.S. Believes Russian Spies Used Kaspersky Antivirus to Steal NSA Secrets
from The Hacker News http://ift.tt/2wBbxuB
via IFTTT
Agendas amp Minutes Alpena County Property Tax Search Anonymous Crime Tip Area Maps ...
from Google Alert - anonymous http://ift.tt/2ghonZb
via IFTTT
ISS Daily Summary Report – 10/05/2017
from ISS On-Orbit Status Report http://ift.tt/2xmH4A0
via IFTTT
Apple Allows Uber to Use a Powerful Feature that Lets it Record iPhone Screen
from The Hacker News http://ift.tt/2xXdBRG
via IFTTT
Apple macOS High Sierra Bug Exposes Passwords of Encrypted APFS Volumes As Hint
from The Hacker News http://ift.tt/2ytyTa6
via IFTTT
The Brown Ocean Effect
from NASA's Scientific Visualization Studio: Most Recent Items http://ift.tt/2y3ygmm
via IFTTT
Global Aurora at Mars
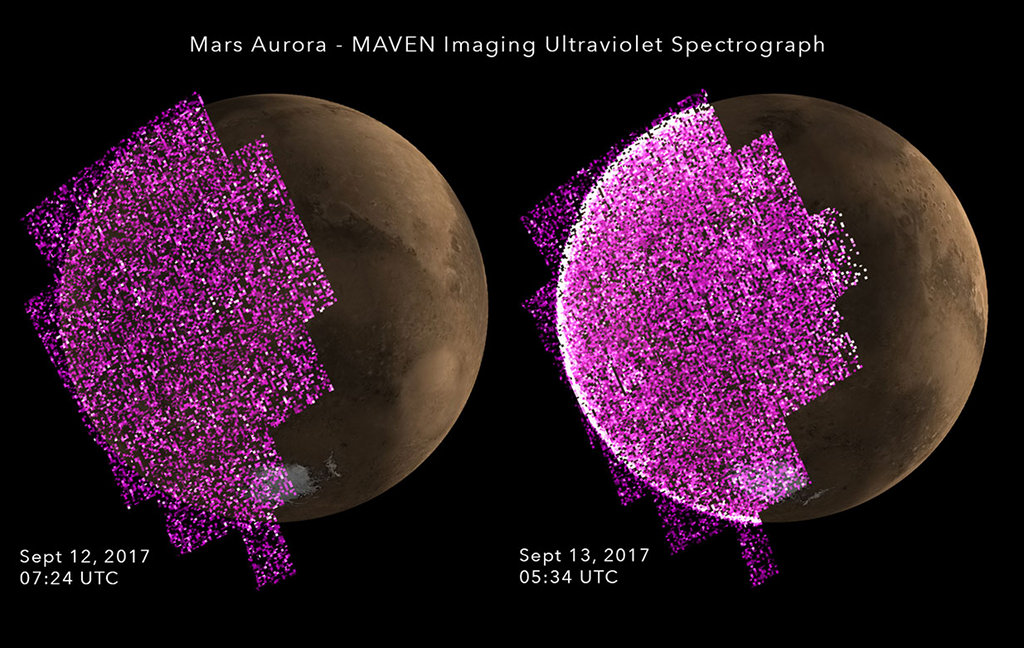
Thursday, October 5, 2017
GrantAdvisor.org: Anonymous Reviews of Foundations
from Google Alert - anonymous http://ift.tt/2xWH9Pk
via IFTTT
FormBook—Cheap Password Stealing Malware Used In Targeted Attacks
from The Hacker News http://ift.tt/2ys0yrZ
via IFTTT
Education Association of Passaic
from Google Alert - anonymous http://ift.tt/2yKOGxz
via IFTTT
Ravens: CB Jimmy Smith, DT Brandon Williams among players missing practice Thursday (ESPN)
via IFTTT
ISS Daily Summary Report – 10/04/2017
from ISS On-Orbit Status Report http://ift.tt/2y2q3PJ
via IFTTT
Anonymous user 64e02f
from Google Alert - anonymous http://ift.tt/2xkv5bi
via IFTTT
Greek Court Approves US Extradition of BTC-e Operator In $4 Billion Money Laundering Case
from The Hacker News http://ift.tt/2z0lxiZ
via IFTTT
Apache Tomcat Patches Important Remote Code Execution Flaw
from The Hacker News http://ift.tt/2y1BlmT
via IFTTT
Spanish Court Agrees to Extradite Russian Spam King to the United States
from The Hacker News http://ift.tt/2hNEUY9
via IFTTT
Pluto s Bladed Terrain
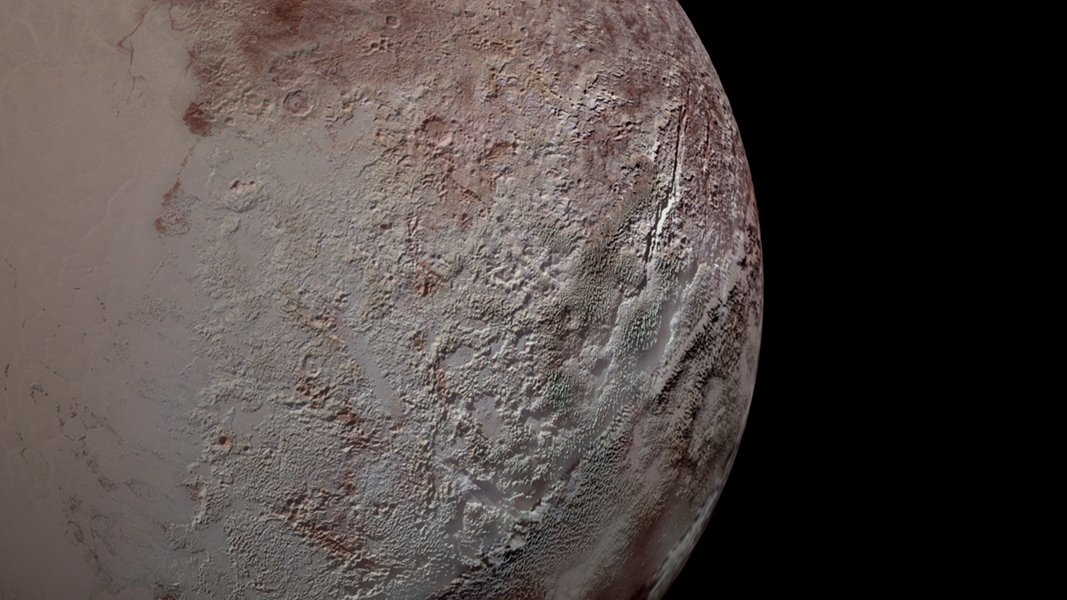
Wednesday, October 4, 2017
I have a new follower on Twitter
Allan McKay
Award winning VFX Supervisor/Director, writer and Entrepreneur, owner of Catastrophic FX film studio and awarded public speaker - http://t.co/Q6BwDU35xI
Los Angeles
http://t.co/Q6BwDU35xI
Following: 7799 - Followers: 12014
October 04, 2017 at 09:45PM via Twitter http://twitter.com/allanftmckay
I have a new follower on Twitter
Kerwin McKenzie
Loyalty Luxury Traveler | Speaker | Author | Visiting every country: 120/194. Airlines flown: 168. https://t.co/PpR6WoIQOL https://t.co/eCcTnEj9B6
Gather No Moss
https://t.co/Uty9brzR63
Following: 5618 - Followers: 8585
October 04, 2017 at 05:50PM via Twitter http://twitter.com/loyaltytravels
Child Pornography Charges Laid Thanks to Anonymous Tipsters
from Google Alert - anonymous http://ift.tt/2fMUq2g
via IFTTT
Audrey (Century Meeting)
from Google Alert - anonymous http://ift.tt/2yYNrvL
via IFTTT
Ravens to log enough miles in two weeks to travel halfway around the globe - Jamison Hensley (ESPN)
via IFTTT
Motion Graphics artist wanted - full-time Midwest at Anonymous
from Google Alert - anonymous http://ift.tt/2xTyhHM
via IFTTT
▶ Joe Flacco says offense needs to play freely and "let it loose" (ESPN)
via IFTTT
I have a new follower on Twitter
SlashNext
#SlashNext: The world’s first Internet Access Protection System. Protecting users & systems every time they connect to the Internet #CyberDefense #CyberSecurity
Global
https://t.co/g5vcq1ksT3
Following: 2580 - Followers: 2306
October 04, 2017 at 12:35PM via Twitter http://twitter.com/slashnextinc
NY Considers Ban On Anonymous Political Facebook Ads
from Google Alert - anonymous http://ift.tt/2xZAXp7
via IFTTT
ISS Daily Summary Report – 10/03/2017
from ISS On-Orbit Status Report http://ift.tt/2gaOfWf
via IFTTT
High anonymous vpn for free
from Google Alert - anonymous http://ift.tt/2xR5lCF
via IFTTT
Anonymous user e7ff8a
from Google Alert - anonymous http://ift.tt/2yGA3LK
via IFTTT
incoming call show anonymous
from Google Alert - anonymous http://ift.tt/2xZopOU
via IFTTT
Team Anonymous
from Google Alert - anonymous http://ift.tt/2xg1hrx
via IFTTT
I have a new follower on Twitter
Grotez Farnugen
Everything threat and counter intelligence
Sydney, New South Wales, Australia
https://t.co/uEzfg8BrEC
Following: 1774 - Followers: 154
October 04, 2017 at 04:55AM via Twitter http://twitter.com/Grotezinfosec
The Soul Nebula in Infrared from Herschel
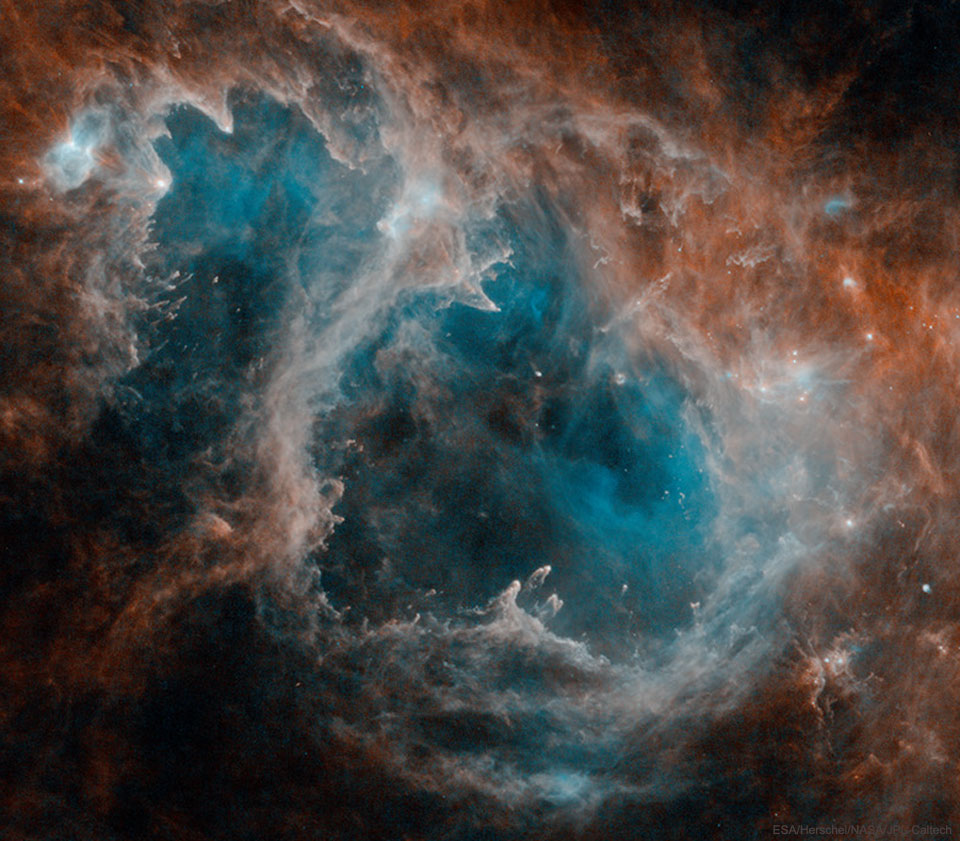
Anonymous - Function Sales Manager
from Google Alert - anonymous http://ift.tt/2y06WGb
via IFTTT
It's 3 Billion! Yes, Every Single Yahoo Account Was Hacked In 2013 Data Breach
from The Hacker News http://ift.tt/2xQN094
via IFTTT
Tuesday, October 3, 2017
[FD] SSD Advisory – Horde Groupware Unauthorized File Download
Source: Gmail -> IFTTT-> Blogger
[FD] SSD Advisory – Mac OS X 10.12 Quarantine Bypass
Source: Gmail -> IFTTT-> Blogger
[FD] SSD Advisory – Netgear ReadyNAS Surveillance Unauthenticated Remote Command Execution
Source: Gmail -> IFTTT-> Blogger
New York bill would ban anonymous political ads on Facebook
from Google Alert - anonymous http://ift.tt/2g9R0Y9
via IFTTT
I have a new follower on Twitter
SwissCognitive
THE Global AI Hub for #ArtificialIntelligence #AI #CognitiveComputing, #Machinelearning #ML #DeepLearning #DL #NLP #Robotic We meet, exchange, connect & debate
Switzerland, World & Universe
https://t.co/DpFFXFnnCo
Following: 3371 - Followers: 12375
October 03, 2017 at 09:05PM via Twitter http://twitter.com/SwissCognitive
📉 Ravens fall 4 spots to No. 22 in Week 5 NFL Power Rankings (ESPN)
via IFTTT
EtherParty Breach: Another Ethereum ICO Gets Hacked
from The Hacker News http://ift.tt/2xdXGKj
via IFTTT
ISS Daily Summary Report – 10/02/2017
from ISS On-Orbit Status Report http://ift.tt/2yG7wFV
via IFTTT
[InsideNothing] toddbschlueter liked your post "[FD] SEC Consult SA-20170914-1 :: Persistent Cross-Site Scripting in SilverStripe CMS"
|
Source: Gmail -> IFTTT-> Blogger
Google Finds 7 Security Flaws in Widely Used Dnsmasq Network Software
from The Hacker News http://ift.tt/2fMrtYa
via IFTTT
Johnston Schools closed after anonymous threats were made
from Google Alert - anonymous http://ift.tt/2xNfWyy
via IFTTT
Anonymous User
from Google Alert - anonymous http://ift.tt/2yUoUrC
via IFTTT
Whoops, Turns Out 2.5 Million More Americans Were Affected By Equifax Breach
from The Hacker News http://ift.tt/2yW7ElM
via IFTTT
Monday, October 2, 2017
October 2017 Webinar Calendar
Source: Gmail -> IFTTT-> Blogger
Bar Person
from Google Alert - anonymous http://ift.tt/2ki6Kgd
via IFTTT
Vinylholics Anonymous (Dazzle Record Exchange)
from Google Alert - anonymous http://ift.tt/2fF2F0h
via IFTTT
Force password protection and private(anonymous)
from Google Alert - anonymous http://ift.tt/2xW6UPp
via IFTTT
Models-Collected works of VOC
from Google Alert - anonymous http://ift.tt/2xakwaC
via IFTTT
Help FreeFrom win $100000 by making a donation before October 31st!
from Google Alert - anonymous http://ift.tt/2xazWMe
via IFTTT
▶ #ICYMI: Le'Veon Bell (186 total yards, 2 TDs) helps Steelers run past Ravens 26-9 (ESPN)
via IFTTT
Deep learning on the Raspberry Pi with OpenCV
I’ve received a number of emails from PyImageSearch readers who are interested in performing deep learning in their Raspberry Pi. Most of the questions go something like this:
Hey Adrian, thanks for all the tutorials on deep learning. You’ve really made deep learning accessible and easy to understand. I have a question: Can I do deep learning on the Raspberry Pi? What are the steps?
And almost always, I have the same response:
The question really depends on what you mean by “do”. You should never be training a neural network on the Raspberry Pi — it’s far too underpowered. You’re much better off training the network on your laptop, desktop, or even GPU (if you have one available).
That said, you can deploy efficient, shallow neural networks to the Raspberry Pi and use them to classify input images.
Again, I cannot stress this point enough:
You should not be training neural networks on the Raspberry Pi (unless you’re using the Pi to do the “Hello, World” equivalent of neural networks — but again, I would still argue that your laptop/desktop is a better fit).
With the Raspberry Pi there just isn’t enough RAM.
The processor is too slow.
And in general it’s not the right hardware for heavy computational processes.
Instead, you should first train your network on your laptop, desktop, or deep learning environment.
Once the network is trained, you can then deploy the neural network to your Raspberry Pi.
In the remainder of this blog post I’ll demonstrate how we can use the Raspberry Pi and pre- trained deep learning neural networks to classify input images.
Looking for the source code to this post?
Jump right to the downloads section.
Deep learning on the Raspberry Pi with OpenCV
When using the Raspberry Pi for deep learning we have two major pitfalls working against us:
- Restricted memory (only 1GB on the Raspberry Pi 3).
- Limited processor speed.
This makes it near impossible to use larger, deeper neural networks.
Instead, we need to use more computationally efficient networks with a smaller memory/processing footprint such as MobileNet and SqueezeNet. These networks are more appropriate for the Raspberry Pi; however, you need to set your expectations accordingly — you should not expect blazing fast speed.
In this tutorial we’ll specifically be using SqueezeNet.
What is SqueezeNet?
Figure 1: The “fire” module in SqueezeNet, consisting of a “squeeze” and an “expand” (Iandola et al., 2016).
SqueezeNet was first introduced by Iandola et al. in their 2016 paper, SqueezeNet: AlexNet-level accuracy with 50x few parameters and <0.5MB model size.
The title alone of this paper should pique your interest.
State-of-the-art architectures such as ResNet have model sizes that are >100MB. VGGNet is over 550MB. AlexNet sits in the middle of this size range with a model size of ~250MB.
In fact, one of the smaller Convolutional Neural Networks used for image classification is GoogLeNet at ~25-50MB (depending on which version of the architecture is implemented).
The real question is: Can we go smaller?
As the work of Iandola et al. demonstrates, the answer is: Yes, we can decrease model size by applying a novel usage of 1×1 and 3×3 convolutions, along with no fully-connected layers. The end result is a model weighing in at 4.9MB, which can be further reduced to < 0.5MB by model processing (also called “weight pruning” and “sparsifying a model”).
In the remainder of this tutorial I’ll be demonstrating how SqueezeNet can classify images in approximately half the time of GoogLeNet, making it a reasonable choice when applying deep learning on your Raspberry Pi.
Interested in learning more about SqueezeNet?
Figure 2: Deep Learning for Computer Vision with Python book
If you’re interested in learning more about SqueezeNet, I would encourage you to take a look at my new book, Deep Learning for Computer Vision with Python.
Inside the ImageNet Bundle, I:
- Explain the inner workings of the SqueezeNet architecture.
- Demonstrate how to implement SqueezeNet by hand.
- Train SqueezeNet from scratch on the challenging ImageNetd ataset and replicate the original results by Iandola et al.
Go ahead and take a look — I think you’ll agree with me when I say that this is the most complete deep learning + computer vision education you can find online.
Running a deep neural network on the Raspberry Pi
The source code from this blog post is heavily based on my previous post, Deep learning with OpenCV.
I’ll still review the code in its entirety here; however, I would like to refer you over to the previous post for a complete and exhaustive review.
To get started, create a new file named
pi_deep_learning.py, and insert the following source code:
# import the necessary packages import numpy as np import argparse import time import cv2
Lines 2-5 simply import our required packages.
From there, we need to parse our command line arguments:
# construct the argument parse and parse the arguments ap = argparse.ArgumentParser() ap.add_argument("-i", "--image", required=True, help="path to input image") ap.add_argument("-p", "--prototxt", required=True, help="path to Caffe 'deploy' prototxt file") ap.add_argument("-m", "--model", required=True, help="path to Caffe pre-trained model") ap.add_argument("-l", "--labels", required=True, help="path to ImageNet labels (i.e., syn-sets)") args = vars(ap.parse_args())
As is shown on Lines 9-16 we have four required command line arguments:
-
--image
: The path to the input image. -
--prototxt
: The path to a Caffe prototxt file which is essentially a plaintext configuration file following a JSON-like structure. I cover the anatomy of Caffe projects in my PyImageSearch Gurus course. -
--model
: The path to a pre-trained Caffe model. As stated above, you’ll want to train your model on hardware which packs much more punch than the Raspberry Pi — we can, however, leverage a small, pre-existing model on the Pi. -
--labels
: The path to class labels, in this case ImageNet “syn-sets” labels.
Next, we’ll load the class labels and input image from disk:
# load the class labels from disk rows = open(args["labels"]).read().strip().split("\n") classes = [r[r.find(" ") + 1:].split(",")[0] for r in rows] # load the input image from disk image = cv2.imread(args["image"])
Go ahead and open
synset_words.txtfound in the “Downloads” section of this post. You’ll see on each line/row there is an ID and class labels associated with it (separated by commas).
Lines 20 and 21 simply read in the labels file line-by-line (
rows) and extract the first relevant class label. The result is a
classeslist containing our class labels.
Then, we utilize OpenCV to load the image on Line 24.
Now we’ll make use of OpenCV 3.3’s Deep Neural Network (DNN) module to convert the
imageto a
blobas well as to load the model from disk:
# our CNN requires fixed spatial dimensions for our input image(s) # so we need to ensure it is resized to 224x224 pixels while # performing mean subtraction (104, 117, 123) to normalize the input; # after executing this command our "blob" now has the shape: # (1, 3, 224, 224) blob = cv2.dnn.blobFromImage(image, 1, (224, 224), (104, 117, 123)) # load our serialized model from disk print("[INFO] loading model...") net = cv2.dnn.readNetFromCaffe(args["prototxt"], args["model"])
Be sure to make note of the comment preceding our call to
cv2.dnn.blobFromImageon Line 31 above.
Common choices for width and height image dimensions inputted to Convolutional Neural Networks include 32 × 32, 64 × 64, 224 × 224, 227 × 227, 256 × 256, and 299 × 299. In our case we are pre-processing (normalizing) the image to dimensions of 224 x 224 (which are the image dimensions SqueezeNet was trained on) and performing a scaling technique known as mean subtraction. I discuss the importance of these steps in my book.
We then load the network from disk on Line 35 by utilizing our
prototxtand
modelfile path references.
In case you missed it above, it is worth noting here that we are loading a pre-trained model. The training step has already been performed on a more powerful machine and is outside the scope of this blog post (but covered in detail in both PyImageSearch Gurus and Deep Learning for Computer Vision with Python).
Now we’re ready to pass the image through the network and look at the predictions:
# set the blob as input to the network and perform a forward-pass to # obtain our output classification net.setInput(blob) start = time.time() preds = net.forward() end = time.time() print("[INFO] classification took {:.5} seconds".format(end - start)) # sort the indexes of the probabilities in descending order (higher # probabilitiy first) and grab the top-5 predictions preds = preds.reshape((1, len(classes))) idxs = np.argsort(preds[0])[::-1][:5]
To classify the query
blob, we pass it forward through the network (Lines 39-42) and print out the amount of time it took to classify the input image (Line 43).
We can then sort the probabilities from highest to lowest (Line 47) while grabbing the top five
predictions(Line 48).
The remaining lines (1) draw the highest predicted class label and corresponding probability on the image, (2) print the top five results and probabilities to the terminal, and (3) display the image to the screen:
# loop over the top-5 predictions and display them for (i, idx) in enumerate(idxs): # draw the top prediction on the input image if i == 0: text = "Label: {}, {:.2f}%".format(classes[idx], preds[0][idx] * 100) cv2.putText(image, text, (5, 25), cv2.FONT_HERSHEY_SIMPLEX, 0.7, (0, 0, 255), 2) # display the predicted label + associated probability to the # console print("[INFO] {}. label: {}, probability: {:.5}".format(i + 1, classes[idx], preds[0][idx])) # display the output image cv2.imshow("Image", image) cv2.waitKey(0)
We draw the top prediction and probability on the top of the image (Lines 53-57) and display the top-5 predictions + probabilities on the terminal (Lines 61 and 62).
Finally, we display the output image on the screen (Lines 65 and 66). If you are using SSH to connect with your Raspberry Pi this will only work if you supply the
-Xflag for X11 forwarding when SSH’ing into your Pi.
To see the results of applying deep learning on the Raspberry Pi using OpenCV and Python, proceed to the next section.
Raspberry Pi and deep learning results
We’ll be benchmarking our Raspberry Pi for deep learning against two pre-trained deep neural networks:
- GoogLeNet
- SqueezeNet
As we’ll see, SqueezeNet is much smaller than GoogLeNet (5MB vs. 25MB, respectively) and will enable us to classify images substantially faster on the Raspberry Pi.
To run pre-trained Convolutional Neural Networks on the Raspberry Pi use the “Downloads” section of this blog post to download the source code + pre-trained neural networks + example images.
From there, let’s first benchmark GoogLeNet against this input image:
Figure 3: A “barbershop” is correctly classified by both GoogLeNet and Squeezenet using deep learning and OpenCV.
As we can see from the output, GoogLeNet correctly classified the image as “barbershop” in 1.7 seconds:
$ python pi_deep_learning.py --prototxt models/bvlc_googlenet.prototxt \ --model models/bvlc_googlenet.caffemodel --labels synset_words.txt \ --image images/barbershop.png [INFO] loading model... [INFO] classification took 1.7304 seconds [INFO] 1. label: barbershop, probability: 0.70508 [INFO] 2. label: barber chair, probability: 0.29491 [INFO] 3. label: restaurant, probability: 2.9732e-06 [INFO] 4. label: desk, probability: 2.06e-06 [INFO] 5. label: rocking chair, probability: 1.7565e-06
Let’s give SqueezeNet a try:
$ python pi_deep_learning.py --prototxt models/squeezenet_v1.0.prototxt \ --model models/squeezenet_v1.0.caffemodel --labels synset_words.txt \ --image images/barbershop.png [INFO] loading model... [INFO] classification took 0.92073 seconds [INFO] 1. label: barbershop, probability: 0.80578 [INFO] 2. label: barber chair, probability: 0.15124 [INFO] 3. label: half track, probability: 0.0052873 [INFO] 4. label: restaurant, probability: 0.0040124 [INFO] 5. label: desktop computer, probability: 0.0033352
SqueezeNet also correctly classified the image as “barbershop”…
…but in only 0.9 seconds!
As we can see, SqueezeNet is significantly faster than GoogLeNet — which is extremely important since we are applying deep learning to the resource constrained Raspberry Pi.
Let’s try another example with SqueezeNet:
$ python pi_deep_learning.py --prototxt models/squeezenet_v1.0.prototxt \ --model models/squeezenet_v1.0.caffemodel --labels synset_words.txt \ --image images/cobra.png [INFO] loading model... [INFO] classification took 0.91687 seconds [INFO] 1. label: Indian cobra, probability: 0.47972 [INFO] 2. label: leatherback turtle, probability: 0.16858 [INFO] 3. label: water snake, probability: 0.10558 [INFO] 4. label: common iguana, probability: 0.059227 [INFO] 5. label: sea snake, probability: 0.046393
Figure 4: SqueezeNet correctly classifies an image of a cobra using deep learning and OpenCV on the Raspberry Pi.
However, while SqueezeNet is significantly faster, it’s less accurate than GoogLeNet:
$ python pi_deep_learning.py --prototxt models/squeezenet_v1.0.prototxt \ --model models/squeezenet_v1.0.caffemodel --labels synset_words.txt \ --image images/jellyfish.png [INFO] loading model... [INFO] classification took 0.92117 seconds [INFO] 1. label: bubble, probability: 0.59491 [INFO] 2. label: jellyfish, probability: 0.23758 [INFO] 3. label: Petri dish, probability: 0.13345 [INFO] 4. label: lemon, probability: 0.012629 [INFO] 5. label: dough, probability: 0.0025394
Here we see the top prediction by SqueezeNet is “bubble”. While the image may appear to have bubble-like characteristics, the image is actually of a “jellyfish” (which is the #2 prediction from SqueezeNet).
GoogLeNet on the other hand correctly reports “jellyfish” as the #1 prediction (with the sacrifice of processing time):
$ python pi_deep_learning.py --prototxt models/bvlc_googlenet.prototxt \ --model models/bvlc_googlenet.caffemodel --labels synset_words.txt \ --image images/jellyfish.png [INFO] loading model... [INFO] classification took 1.7824 seconds [INFO] 1. label: jellyfish, probability: 0.53186 [INFO] 2. label: bubble, probability: 0.33562 [INFO] 3. label: tray, probability: 0.050089 [INFO] 4. label: shower cap, probability: 0.022811 [INFO] 5. label: Petri dish, probability: 0.013176
Summary
Today, we learned how to apply deep learning on the Raspberry Pi using Python and OpenCV.
In general, you should:
- Never use your Raspberry Pi to train a neural network.
- Only use your Raspberry Pi to deploy a pre-trained deep learning network.
The Raspberry Pi does not have enough memory or CPU power to train these types of deep, complex neural networks from scratch.
In fact, the Raspberry Pi barely has enough processing power to run them — as we’ll find out in next week’s blog post you’ll struggle to get a reasonable frames per second for video processing applications.
If you’re interested in embedded deep learning on low cost hardware, I’d consider looking at optimized devices such as NVIDIA’s Jetson TX1 and TX2. These boards are designed to execute neural networks on the GPU and provide real-time (or as close to real-time as possible) classification speed.
In next week’s blog post, I’ll be discussing how to optimize OpenCV on the Raspberry Pi to obtain performance gains by upwards of 100% for object detection using deep learning.
To be notified when this blog post is published, just enter your email address in the form below!
Downloads:
The post Deep learning on the Raspberry Pi with OpenCV appeared first on PyImageSearch.
from PyImageSearch http://ift.tt/2xadVbi
via IFTTT