Latest YouTube Video
Saturday, September 30, 2017
Support admin payments on anonymous orders
from Google Alert - anonymous http://ift.tt/2xOGwXv
via IFTTT
12 Steps Companion
from Google Alert - anonymous http://ift.tt/2wqnSlC
via IFTTT
Anonymous anonymous
from Google Alert - anonymous http://ift.tt/2x4wgv6
via IFTTT
I have a new follower on Twitter
Fourth & Goal
Following: 2519 - Followers: 2617
September 30, 2017 at 06:01AM via Twitter http://twitter.com/FourthandGoal01
I have a new follower on Twitter
Robert Half MR
Robert Half Management Resources provides senior-level financial and business systems project professionals.
Menlo Park, CA
https://t.co/GmE2XD1RXP
Following: 3909 - Followers: 7291
September 30, 2017 at 06:01AM via Twitter http://twitter.com/RobertHalfMR
I have a new follower on Twitter
Marketing Show
https://t.co/h4tlby5GqL
Following: 1198 - Followers: 1363
September 30, 2017 at 06:01AM via Twitter http://twitter.com/CMMarketingShow
Transitional Master's
from Google Alert - anonymous http://ift.tt/2xFDbe0
via IFTTT
Anonymous - Culinary Consultant or Culinary Partner
from Google Alert - anonymous http://ift.tt/2x4aNhk
via IFTTT
Anonymous Source on the Texans
from Google Alert - anonymous http://ift.tt/2fEW9KS
via IFTTT
David Wright rips teammates for anonymous Collins bashing
from Google Alert - anonymous http://ift.tt/2xMQHvv
via IFTTT
Portrait of NGC 281
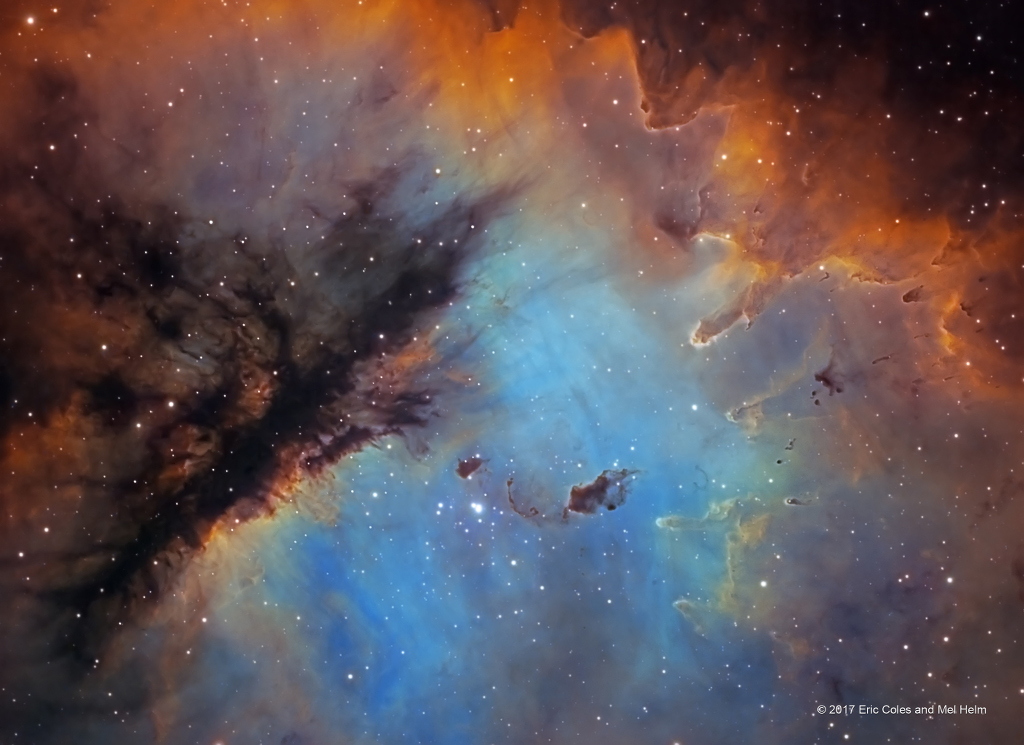
Friday, September 29, 2017
Procrastinators Anonymous Workshop
from Google Alert - anonymous http://ift.tt/2x1WnmN
via IFTTT
[InsideNothing] Harold's liked your post "[FD] DefenseCode Security Advisory: IBM DB2 Command Line Processor Buffer Overflow"
|
Source: Gmail -> IFTTT-> Blogger
thirty, forty, fifty thousand photocopies
from Google Alert - anonymous http://ift.tt/2x1AtzT
via IFTTT
Ravens: Cleaning up Joe Flacco's interceptions is key to getting offense on track - Jamison Hensley (ESPN)
via IFTTT
ISS Daily Summary Report – 9/28/2017
from ISS On-Orbit Status Report http://ift.tt/2xDDLsH
via IFTTT
[FD] OpenText Document Sciences xPression (formerly EMC Document Sciences xPression) - SQL Injection
Source: Gmail -> IFTTT-> Blogger
[FD] OpenText Document Sciences xPression (formerly EMC Document Sciences xPression) - Arbitrary File Read
Source: Gmail -> IFTTT-> Blogger
eduCBA Review: Software Testing by Anonymous Reviewer
from Google Alert - anonymous http://ift.tt/2fx4ZGL
via IFTTT
Millions of Up-to-Date Apple Macs Remain Vulnerable to EFI Firmware Hacks
from The Hacker News http://ift.tt/2ycwPDm
via IFTTT
Buy Hudson Blake Slim Straight In Anonymous Online
from Google Alert - anonymous http://ift.tt/2xQvxx5
via IFTTT
Ravens: Brandon Williams (leg) won't play Sunday vs. Steelers (ESPN)
via IFTTT
[FD] Trend Micro OfficeScan v11.0 and XG (12.0)* CURL (MITM) Remote Code Execution CVE-2017-14084
Source: Gmail -> IFTTT-> Blogger
[FD] Zoho Site24x7 for Android Didn’t Properly Validate SSL
Source: Gmail -> IFTTT-> Blogger
[FD] [CVE-2017-11322] UCOPIA Wireless Appliance < 5.1.8 Privileges Escalation
Source: Gmail -> IFTTT-> Blogger
[FD] [CVE-2017-11321] UCOPIA Wireless Appliance < 5.1.8 Restricted Shell Escape
Source: Gmail -> IFTTT-> Blogger
[FD] Zyxel P-2812HNU-F1 DSL router - command injection
Source: Gmail -> IFTTT-> Blogger
macOS for deep learning with Python, TensorFlow, and Keras
In today’s tutorial, I’ll demonstrate how you can configure your macOS system for deep learning using Python, TensorFlow, and Keras.
This tutorial is the final part of a series on configuring your development environment for deep learning. I created these tutorials to accompany my new book, Deep Learning for Computer Vision with Python; however, you can use these instructions to configure your system regardless if you bought my book or not.
In case you’re on the wrong page (or you don’t have macOS), take a look at the other deep learning development environment tutorials in this series:
- Your deep learning + Python Ubuntu virtual machine
- Pre-configured Amazon AWS deep learning AMI with Python
- Configuring Ubuntu for deep learning with Python (CPU only)
- Setting up Ubuntu 16.04 + CUDA + GPU for deep learning with Python
- macOS for deep learning with Python, TensorFlow, and Keras (this post)
To learn how to configure macOS for deep learning and computer vision with Python, just keep reading.
macOS for deep learning with Python, TensorFlow, and Keras
As you get acclimated in the deep learning domain, you’ll want to perform many experiments to hone your skills and even to solve real-world problems.
You’ll find that for experiments in the most chapters inside the Starter Bundle and half the chapters in the Practitioner Bundle can be executed on your CPU. Readers of the ImageNet Bundle will need a GPU machine in order to perform the more advanced experiments.
I definitely don’t recommend churning through large datasets and deep neural networks on your laptop, but like I said, for small experiments it is just fine.
Today, I’ll walk you through the steps to configure your Mac for deep learning.
First, we’ll install Xcode and Homebrew (a package manager). From there we will create a virtual environment called
dl4cvand install OpenCV, TensorFlow, and Keras into the environment.
Let’s get started.
Step #1: Install Xcode
For starters, you’ll need to get Xcode from the Apple App Store and install it. Don’t worry, it is 100% free.
From there, open a terminal and execute the following command to accept the developer license:
$ sudo xcodebuild -license
The next step is to install Apple command line tools:
$ sudo xcode-select --install
Step #2: Install Homebrew
Homebrew (also known as Brew), is a package manager for macOS. You may already have it on your system, but if you don’t you will want to perform the actions in this section.
First we’ll install Homebrew by copying and pasting the entire command into your terminal:
$ /usr/bin/ruby -e "$(curl -fsSL http://ift.tt/YQTuQh)"
Next we’ll update our package definitions:
$ brew update
Followed by updating your
~/.bash_profileusing the
nanoterminal editor (any other editor should do the trick as well):
$ nano ~/.bash_profile
Add the following lines to the file:
# Homebrew export PATH=/usr/local/bin:$PATH
Next, simply reload your
~/.bash_profile(this happens automatically when a new terminal is opened):
$ source ~/.bash_profile
Now that Brew is ready to go, let’s get Python 3 installed.
Step #3: Install Homebrew Python 3 for macOS
This step is actually very easy, but I want to clear up some possible confusion first.
macOS comes with Python installed; however we will be installing a non-system Python using Brew. While you could use your system Python, it is actually strongly discouraged. Therefore, don’t skip this step — it is very important to your successful install.
To install Python 3 with Homebrew, simply execute this command:
$ brew install python3
Before continuing you’ll want to verify that your Python 3 installation is Homebrew’s rather than the macOS system’s:
$ which python3 /usr/local/bin/python3 $ which pip3 /usr/local/bin/pip3
Ensure that you see “
local” in each path. If you don’t see this output, then you aren’t using Homebrew’s install of Python 3.
Figure 4: Executing which python3 and which pip3 to ensure that you are using the Homebrew version of each rather than the system version.
Assuming your Python 3 install worked, let’s continue on to Step #4.
Step #4: Create your Python virtual environment
As I’ve stated in other install guides on this site, virtual environments are definitely the way to go when working with Python, enabling you to accommodate different versions in sandboxed environments.
In other words, there is less of a chance that you’ll do something that is a pain in the ass to fix. If you mess up an environment, you can simply delete the environment and rebuild it.
Let’s install virtualenv and virtualenvwrapper via
pip:
$ pip3 install virtualenv virtualenvwrapper
From there, we’ll update our
~/.bash_profileagain:
$ nano ~/.bash_profile
Where we’ll add the following lines to the file:
# virtualenv and virtualenvwrapper export VIRTUALENVWRAPPER_PYTHON=/usr/local/bin/python3 source /usr/local/bin/virtualenvwrapper.sh
Figure 5: The bottom of our ~/.bash_profile should contain these lines for virtualenv and virtualenvwrapper.
Followed by reloading the file:
$ source ~/.bash_profile
Creating the ‘dl4cv’ environment
The
dl4cvenvironment will house all of our software for performing experiments associated with my book. You can easily name the environment whatever you want, but from here on we’ll be referring to it as
dl4cv.
To create the dl4cv environment with Python 3 simply enter the following command:
$ mkvirtualenv dl4cv -p python3
After Python 3 and supporting scripts are installed into the new environment, you should actually be inside the environment. This is denoted by a ‘
(dl4cv)‘ at the beginning of your bash prompt as shown in the figure below:
Figure 6: The (dl4cv) in the bash prompt signifies that we are working inside the dl4cv virtual environment. If you don’t see this, then execute workon dl4cv to activate the environment.
If you do not see the modified bash prompt then you can enter the following command at any time to enter the environment at any time:
$ workon dl4cv
The only Python dependency required by OpenCV is NumPy, which we can install below:
$ pip install numpy
That’s it as far as creating a virtual environment and installing NumPy. Let’s continue to Step #5.
Step #5: Install OpenCV prerequisites using Homebrew
The following tools need to be installed for compilation, image I/O, and optimization:
$ brew install cmake pkg-config wget $ brew install jpeg libpng libtiff openexr $ brew install eigen tbb
After those packages are installed we’re ready to install OpenCV.
Step #6: Compile and Install OpenCV
First, let’s download the source code:
$ cd ~ $ wget -O opencv.zip http://ift.tt/2x4vwWB $ wget -O opencv_contrib.zip http://ift.tt/2xIPYcC
Then unpack the archives:
$ unzip opencv.zip $ unzip opencv_contrib.zip
Followed by configuring the build with CMake (it is very important that you copy the CMake command exactly as it appears here, taking care to copy and past the entire command; I would suggest clicking the “<=>” button in the toolbar below to expand the entire command):
$ cd ~/opencv-3.3.0/ $ mkdir build $ cd build $ cmake -D CMAKE_BUILD_TYPE=RELEASE \ -D CMAKE_INSTALL_PREFIX=/usr/local \ -D OPENCV_EXTRA_MODULES_PATH=~/opencv_contrib-3.3.0/modules \ -D PYTHON3_LIBRARY=`python -c 'import subprocess ; import sys ; s = subprocess.check_output("python-config --configdir", shell=True).decode("utf-8").strip() ; (M, m) = sys.version_info[:2] ; print("{}/libpython{}.{}.dylib".format(s, M, m))'` \ -D PYTHON3_INCLUDE_DIR=`python -c 'import distutils.sysconfig as s; print(s.get_python_inc())'` \ -D PYTHON3_EXECUTABLE=$VIRTUAL_ENV/bin/python \ -D BUILD_opencv_python2=OFF \ -D BUILD_opencv_python3=ON \ -D INSTALL_PYTHON_EXAMPLES=ON \ -D INSTALL_C_EXAMPLES=OFF \ -D BUILD_EXAMPLES=ON ..
Note: For the above CMake command, I spent considerable time creating, testing, and refactoring it. I’m confident that it will save you time and frustration if you use it exactly as it appears. Make sure you click the “<=>” button in the toolbar of the code block above to expand the code block. This will enable you to copy and paste the entire command.
Your output should be similar to the screenshot below which ensures that the correct Python 3 binary/library and NumPy version are utilized:
Figure 7: The OpenCV CMake output that ensures the correct Python 3 and NumPy will be used for compiling.
Then we’re ready to perform the compilation compile OpenCV:
$ make -j4
Note: The number ‘4’ above specifies that we have 4 cores/processors for compiling. If you have a different number of processors you can update the
-jswitch. For only one core/processor simply just use the
makecommand (from the build directory enter
make cleanprior to retrying if your build failed or got stuck).
From there you can install OpenCV:
$ sudo make install
After installing it is necessary to sym-link the
cv2.sofile into the
dl4cvvirtual environment:
$ cd ~/.virtualenvs/dl4cv/lib/python3.6/site-packages/ $ ln -s /usr/local/lib/python3.6/site-packages/cv2.cpython-36m-darwin.so cv2.so $ cd ~
Finally, we can test out the install:
$ python >>> import cv2 >>> cv2.__version__ '3.3.0'
Figure 8: OpenCV 3.3 has been installed and linked as is shown by the successful import and display of the version.
If your output properly shows the version of OpenCV that you installed, then you’re ready to go on to Step #7 where we will install the Keras deep learning library.
Step #7: Install Keras
Before beginning this step, ensure you have activated the
dl4cvvirtualenv. If you aren’t in the environment, simply execute:
$ workon dl4cv
Then, using
pip, install the required Python computer vision, image processing, and machine learning libraries:
$ pip install scipy matplotlib pillow $ pip install imutils h5py requests progressbar2 $ pip install scikit-learn scikit-image
Next, install TensorFlow:
$ pip install tensorflow
Followed by keras:
$ pip install keras
To verify that Keras is installed properly we can import it and check for errors:
$ python >>> import keras Using TensorFlow backend. >>>
Keras should be imported with no errors, while stating that TensorFlow is being utilized as the backend.
At this point, you can familiarize yourself with the
~/.keras/keras.jsonfile:
{ "image_data_format": "channels_last", "backend": "tensorflow", "epsilon": 1e-07, "floatx": "float32" }
Ensure that the
image_data_formatis set to
channels_lastand that the
backendis set to
tensorflow.
Congratulations! You’re now ready to go. If you didn’t open up a beer or coffee during the installation process, now is the time. It’s also the time to find a comfortable spot to read Deep Learning for Computer Vision with Python.
Summary
In today’s post, we configured our macOS box for computer vision and deep learning. The main pieces of software included Python 3, OpenCV, TensorFlow, and Keras accompanied by dependencies and installation/compilation tools.
As you can see, utilizing Homebrew, pip, and virtualenv + virtualenvwrapper made this install rather easy. I spent quite a bit of time creating and testing the CMake command which should work easily on your computer. Be sure to give it a try.
If you encountered any problems along the way, leave a comment in the form below.
If you would like to put your newly configured macOS deep learning environment to good use, I would highly suggest you take a look at my new book, Deep Learning for Computer Vision with Python.
Regardless if you’re new to deep learning or already a seasoned practitioner, the book has content to help you reach deep learning mastery — take a look here.
The post macOS for deep learning with Python, TensorFlow, and Keras appeared first on PyImageSearch.
from PyImageSearch http://ift.tt/2xLkwMM
via IFTTT
Puppis A Supernova Remnant
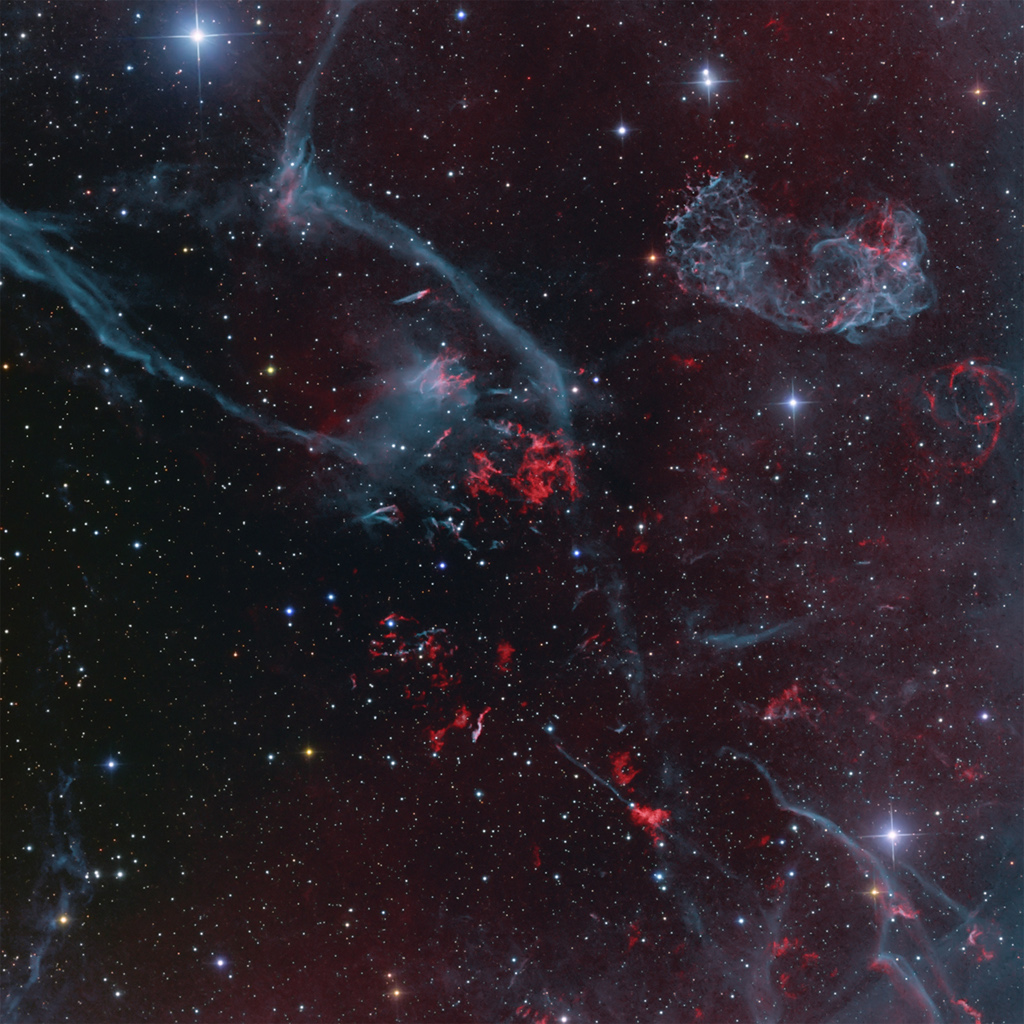
Amazon's Whole Foods Market Suffers Credit Card Breach In Some Stores
from The Hacker News http://ift.tt/2xFSxN8
via IFTTT
Thursday, September 28, 2017
Super Bowl XLVII hero Jacoby Jones will retire as a member of Ravens Friday (ESPN)
via IFTTT
Hackers Exploiting Microsoft Servers to Mine Monero - Makes $63,000 In 3 Months
from The Hacker News http://ift.tt/2fUqOR3
via IFTTT
Ravens defense refuses to fall for Ben Roethlisberger's Jedi mind tricks - Jamison Hensley (ESPN)
via IFTTT
ISS Daily Summary Report – 9/27/2017
from ISS On-Orbit Status Report http://ift.tt/2xIw0Ry
via IFTTT
Ravens increase security around Ray Lewis statue outside M&T Bank Stadium (ESPN)
via IFTTT
Dark-Web Drug Dealer Arrested After He Travelled US for World Beard Championships
from The Hacker News http://ift.tt/2wlTnNy
via IFTTT
Bank of Greece Denies Anonymous' Hacking Claim
from Google Alert - anonymous http://ift.tt/2xApuNa
via IFTTT
2-Year-Old Linux Kernel Issue Resurfaces As High-Risk Flaw
from The Hacker News http://ift.tt/2xMUIAD
via IFTTT
Anonymous user cc98b6
from Google Alert - anonymous http://ift.tt/2xDPO6X
via IFTTT
Stampers Anonymous® Tim Holtz Layered Stencil-Poinsettia
from Google Alert - anonymous http://ift.tt/2wY0Amy
via IFTTT
Anonymous Post doesn't generate thumbnails
from Google Alert - anonymous http://ift.tt/2wl5F8I
via IFTTT
Wednesday, September 27, 2017
Anonymous user e8aeba
from Google Alert - anonymous http://ift.tt/2xGS3I1
via IFTTT
Ravens: Eric Weddle takes playful jab at winless Chargers - Jamison Hensley (ESPN)
via IFTTT
Rude/Offensive Map Chats and Comments from Anonymous Users
from Google Alert - anonymous http://ift.tt/2hwSTBv
via IFTTT
Setting up Ubuntu 16.04 + CUDA + GPU for deep learning with Python
Welcome back! This is the fourth post in the deep learning development environment configuration series which accompany my new book, Deep Learning for Computer Vision with Python.
Today, we will configure Ubuntu + NVIDIA GPU + CUDA with everything you need to be successful when training your own deep learning networks on your GPU.
Links to related tutorials can be found here:
- Your deep learning + Python Ubuntu virtual machine
- Pre-configured Amazon AWS deep learning AMI with Python
- Configuring Ubuntu for deep learning with Python (for a CPU only environment)
- Setting up Ubuntu 16.04 + CUDA + GPU for deep learning with Python (this post)
- Configuring macOS for deep learning with Python (releasing on Friday)
If you have an NVIDIA CUDA compatible GPU, you can use this tutorial to configure your deep learning development to train and execute neural networks on your optimized GPU hardware.
Let’s go ahead and get started!
Setting up Ubuntu 16.04 + CUDA + GPU for deep learning with Python
If you’ve reached this point, you are likely serious about deep learning and want to train your neural networks with a GPU.
Graphics Processing Units are great at deep learning for their parallel processing architecture — in fact, these days there are many GPUs built specicically for deep learning — they are put to use outside the domain of computer gaming.
NVIDIA is the market leader in deep learning hardware, and quite frankly the primary option I recommend if you are getting in this space. It is worth getting familiar with their lineup of products (hardware and software) so you know what you’re paying for if you’re using an instance in the cloud or building a machine yourself. Be sure to check out this developer page.
It is common to share high end GPU machines at universities and companies. Alternatively, you may build one, buy one (as I did), or rent one in the cloud (as I still do today).
If you are just doing a couple experiments then using a cloud service provider such as Amazon, Google, or FloydHub for a time-based usage charge is the way to go.
Longer term if you are working on deep learning experiments daily, then it would be wise to have one on hand for cost savings purposes (assuming you’re willing to keep the hardware and software updated regularly).
Note: For those utilizing AWS’s EC2, I recommend you select the p2.xlarge, p2.8xlarge, or p2.16xlarge machines for compatibility with these instructions (depending on your use case scenario and budget). The older instances, g2.2xlarge and g2.8xlarge are not compatible with the version of CUDA and cuDNN in this tutorial. I also recommend that you have about 32GB of space on your OS drive/partition. 16GB didn’t cut it for me on my EC2 instance.
It is important to point out that you don’t need access to an expensive GPU machine to get started with Deep Learning. Most modern laptop CPUs will do just fine with the small experiments presented in the early chapters in my book. As I say, “fundamentals before funds” — meaning, get acclimated with modern deep learning fundamentals and concepts before you bite off more than you can chew with expensive hardware and cloud bills. My book will allow you to do just that.
How hard is it to configure Ubuntu with GPU support for deep learning?
You’ll soon find out below that configuring a GPU machine isn’t a cakewalk. In fact there are quite a few steps and potential for things to go sour. That’s why I have built a custom Amazon Machine Instance (AMI) pre-configured and pre-installed for the community to accompany my book.
I detailed how to get it loaded into your AWS account and how to boot it up in this previous post.
Using the AMI is by far the fastest way to get started with deep learning on a GPU. Even if you do have a GPU, it’s worth experimenting in the Amazon EC2 cloud so you can tear down an instance (if you make a mistake) and then immediately boot up a new, fresh one.
Configuring an environment on your own is directly related to your:
- Experience with Linux
- Attention to detail
- Patience.
First, you must be very comfortable with the command line.
Many of the steps below have commands that you can simply copy and paste into your terminal; however it is important that you read the output, note any errors, try to resolve them prior to moving on to the next step.
You must pay particular attention to the order of the instructions in this tutorial, and furthermore pay attention to the commands themselves.
I actually do recommend copying and pasting to make sure you don’t mess up a command (in one case below backticks versus quotes could get you stuck).
If you’re up there for the challenge, then I’ll be right there with you getting your environment ready. In fact I encourage you to leave comments so that the PyImageSearch community can offer you assistance. Before you leave a comment be sure to review the post and comments to make sure you didn’t leave a step out.
Without further ado, let’s get our hands dirty and walk through the configuration steps.
Step #1: Install Ubuntu system dependencies
I’m assuming that you are SSH’d into or working directly on your GPU machine at this point.
First, let’s get our Ubuntu OS up to date:
$ sudo apt-get update $ sudo apt-get upgrade
Then, let’s install some necessary development tools, image/video I/O, GUI operations and various other packages:
$ sudo apt-get install build-essential cmake git unzip pkg-config $ sudo apt-get install libjpeg-dev libtiff5-dev libjasper-dev libpng12-dev $ sudo apt-get install libavcodec-dev libavformat-dev libswscale-dev libv4l-dev $ sudo apt-get install libxvidcore-dev libx264-dev $ sudo apt-get install libgtk-3-dev $ sudo apt-get install libhdf5-serial-dev graphviz $ sudo apt-get install libopenblas-dev libatlas-base-dev gfortran $ sudo apt-get install python-tk python3-tk python-imaging-tk
Next, let’s install both Python 2.7 and Python 3 header files so that we can compile OpenCV with Python bindings:
$ sudo apt-get install python2.7-dev python3-dev
We also need to prepare our system to swap out the default drivers with NVIDIA CUDA drivers:
$ sudo apt-get install linux-image-generic linux-image-extra-virtual $ sudo apt-get install linux-source linux-headers-generic
That’s it for Step #1, so let’s continue on.
Step #2: Install CUDA Toolkit
The CUDA Toolkit installation step requires attention to detail for it to go smoothly.
First disable the Nouveau kernel driver by creating a new file:
$ sudo nano /etc/modprobe.d/blacklist-nouveau.conf
Feel free to use your favorite terminal text editor such as
vimor
emacsinstead of
nano.
Add the following lines and then save and exit:
blacklist nouveau blacklist lbm-nouveau options nouveau modeset=0 alias nouveau off alias lbm-nouveau off
Your session should look like the following (if you are using nano):
Next let’s update the initial RAM filesystem and reboot the machine:
$ echo options nouveau modeset=0 | sudo tee -a /etc/modprobe.d/nouveau-kms.conf $ sudo update-initramfs -u $ sudo reboot
You will lose your SSH connection at the reboot step, so wait patiently and then reconnect before moving on.
You will want to download the CUDA Toolkit v8.0 via the NVIDIA CUDA Toolkit website:
Once you’re on the download page, select
Linux => x86_64 => Ubuntu => 16.04 => runfile (local).
Here is a screenshot of the download page:
From there, download the
.runfile which should have the filename
cuda_8.0.61_375.26_linux.runor similar. To do this, simply right-click to copy the download link and use
wgeton your remote GPU box:
wget http://ift.tt/2mtsbcZ
Note: You will need to click the “<=>” button in the code block toolbar above to expand the code block. This will enable you to copy the full URL to the
.runfile.
From there, unpack the
.runfile:
$ chmod +x cuda_8.0.61_375.26_linux-run $ mkdir installers $ sudo ./cuda_8.0.61_375.26_linux-run -extract=`pwd`/installers
The last step in the block above can take 30-60 seconds depending on the speed of your machine.
Now it is time to install the NVIDIA kernel driver:
$ cd installers $ sudo ./NVIDIA-Linux-x86_64-375.26.run
During this process, accept the license and follow prompts on the screen.
From there, add the NVIDIA loadable kernel module (LKM) to the Linux kernel:
$ modprobe nvidia
Install the CUDA Toolkit and examples:
$ sudo ./cuda-linux64-rel-8.0.61-21551265.run $ sudo ./cuda-samples-linux-8.0.61-21551265.run
Again, accepting the licenses and following the default prompts. You may have to press ‘space’ to scroll through the license agreement and then enter “accept” as I’ve done int the image above. When it asks you for installation paths, just press
<enter>to accept the defaults.
Now that the NVIDIA CUDA driver and tools are installed, you need to update your
~/.bashrcfile to include CUDA Toolkit (I suggest using terminal text editors such as
vim,
emacs, or
nano):
# NVIDIA CUDA Toolkit export PATH=/usr/local/cuda-8.0/bin:$PATH export LD_LIBRARY_PATH=/usr/local/cuda-8.0/lib64/
Now, reload your
~/.bashrc(
source ~/.bashrc) and then test the CUDA Toolkit installation by compiling the
deviceQueryexample program and running it:
$ source ~/.bashrc $ cd /usr/local/cuda-8.0/samples/1_Utilities/deviceQuery $ sudo make $ ./deviceQuery deviceQuery, CUDA Driver = CUDART, CUDA Driver Version = 8.0, CUDA Runtime Version = 8.0, NumDevs = 1, Device0 = Tesla K80 Result = PASS
Note: Calling
sourceon
~/.bashrconly has to be done once for our current shell session. Anytime we open up a new terminal, the contents of
~/.bashrcwill be automatically executed (including our updates).
At this point if you have a
Result = PASS, then congratulations because you are ready to move on to the next step.
If you do not see this result, I suggest you repeat Step #2 and examine the output of each and every command carefully to ensure there wasn’t an error during the install.
Step #3: Install cuDNN (CUDA Deep Learning Neural Network library)
For this step, you will need to Create a free account with NVIDIA and download cuDNN.
For this tutorial I used cuDNN v6.0 for Linux which is what TensorFlow requires.
Due to NVIDIA’s required authentication to access the download, you may not be able to use
wgeton your remote machine for the download.
Instead, download the file to your local machine and then (on your local machine) use
scp(Secure Copy) while replacing
<username>and
<password>with appropriate values to update the file to your remote instance (again, assuming you’re accessing your machine via SSH):
scp -i EC2KeyPair.pem ~/Downloads/cudnn-8.0-linux-x64-v6.0.tgz \ username@your_ip_address:~
Next, untar the file and then copy the resulting files into
lib64and
includerespectively, using the
-Pswitch to preserve sym-links:
$ cd ~ $ tar -zxf cudnn-8.0-linux-x64-v6.0.tgz $ cd cuda $ sudo cp -P lib64/* /usr/local/cuda/lib64/ $ sudo cp -P include/* /usr/local/cuda/include/ $ cd ~
That’s it for Step #3 — there isn’t much that can go wrong here, so you should be ready to proceed.
Step #4: Create your Python virtual environment
In this section we will get a Python virtual environment configured on your system.
Installing pip
The first step is to install
pip, a Python package manager:
$ wget http://ift.tt/1mn7OFn $ sudo python get-pip.py $ sudo python3 get-pip.py
Installing virtualenv and virtualenvwrapper
Using
pip, we can install any package in the Python Package Index quite easily including virtualenv and virtualenvwrapper. As you know, I’m a fan of Python virtual environments and I encourage you to use them for deep learning as well.
In case you have multiple projects on your machine, using virtual environments will allow you to isolate them and install different versions of packages. In short, using both
virtualenvand
virtualenvwrapperallow you to solve the “Project X depends on version 1.x, but Project Y needs 4.x dilemma.
The folks over at RealPython may be able to convince you if I haven’t, so give this excellent blog post on RealPython a read.
Again, let me reiterate that it’s standard practice in the Python community to be leveraging virtual environments of some sort, so I suggest you do the same:
$ sudo pip install virtualenv virtualenvwrapper $ sudo rm -rf ~/.cache/pip get-pip.py
Once we have
virtualenvand
virtualenvwrapperinstalled, we need to update our
~/.bashrcfile to include the following lines at the bottom of the file:
# virtualenv and virtualenvwrapper export WORKON_HOME=$HOME/.virtualenvs export VIRTUALENVWRAPPER_PYTHON=/usr/bin/python3 source /usr/local/bin/virtualenvwrapper.sh
After editing our
~/.bashrcfile, we need to reload the changes:
$ source ~/.bashrc
Now that we have installed
virtualenvand
virtualenvwrapper, the next step is to actually create the Python virtual environment — we do this using the
mkvirtualenvcommand.
Creating the dl4cv virtual environment
In past install tutorials, I’ve presented the choice of Python 2.7 or Python 3. At this point in the Python 3 development cycle, I consider it stable and the right choice. You may elect to use Python 2.7 if you have specific compatibility requirements, but for the purposes of my book we will use Python 3.
With that said, for the following command, ensure you set the
-pflag to
python3.
$ mkvirtualenv dl4cv -p python3
You can name this virtual environment whatever you like (and create as many Python virtual environments as you want), but for the time being, I would suggest sticking with the
dl4cvname as that is what I’ll be using throughout the rest of this tutorial.
Verifying that you are in the “dl4cv” virtual environment
If you ever reboot your Ubuntu system; log out and log back in; or open up a new terminal, you’ll need to use the
workoncommand to re-access your
dl4cvvirtual environment. An example of the
workoncommand follows:
$ workon dl4cv
To validate that you are in the
dl4cvvirtual environment, simply examine your command line — if you see the text
(dl4cv)preceding your prompt, then you are in the
dl4cvvirtual environment:
Otherwise if you do not see the
dl4cvtext, then you are not in the
dl4cvvirtual environment:
Installing NumPy
The final step before we compile OpenCV is to install NumPy, a Python package used for numerical processing. To install NumPy, ensure you are in the
dl4cvvirtual environment (otherwise NumPy will be installed into the system version of Python rather than the
dl4cvenvironment).
From there execute the following command:
$ pip install numpy
Once NumPy is installed in your virtual environment, we can move on to compile and install OpenCV.
Step #5: Compile and Install OpenCV
First you’ll need to download opencv and opencv_contrib into your home directory. For this install guide, we’ll be using OpenCV 3.3:
$ cd ~ $ wget -O opencv.zip http://ift.tt/2x4vwWB $ wget -O opencv_contrib.zip http://ift.tt/2xIPYcC
Then, unzip both files:
$ unzip opencv.zip $ unzip opencv_contrib.zip
Running CMake
In this step we create a build directory and then run CMake:
$ cd ~/opencv-3.3.0/ $ mkdir build $ cd build $ cmake -D CMAKE_BUILD_TYPE=RELEASE \ -D CMAKE_INSTALL_PREFIX=/usr/local \ -D WITH_CUDA=OFF \ -D INSTALL_PYTHON_EXAMPLES=ON \ -D OPENCV_EXTRA_MODULES_PATH=~/opencv_contrib-3.3.0/modules \ -D BUILD_EXAMPLES=ON ..
Note: I turned CUDA off as it can lead to compile errors on some machines. The CUDA optimizations would internally be used for C++ functions so it doesn’t make much of a difference with Python + OpenCV. Again, the primary use of CUDA in this blog post is to optimize our deep learning libraries, not OpenCV itself.
For CMake, it is important that your flags match mine for compatibility. Also, make sure that your
opencv_contribversion is the exact same as the
opencvversion you downloaded (in this case version
3.3.0).
Before we move on to the actual compilation step, make sure you examine the output of CMake.
Start by scrolling to the section titled
Python 3.
Make sure that your Python 3 section looks like the figure below:
Figure 6: Verifying that CMake has properly set up the compile to use the correct Python 3 Interpreter and version of NumPy. Both Python 3 and NumPy should be pulled from the dl4cv virtual environment.
Ensure that the Interpreter points to our
python3.5binary located in the
dl4cvvirtual environment while
numpypoints to our NumPy install.
In either case if you do not see the
dl4cvvirtual environment in these variables’ paths, then it’s almost certainly because you are NOT in the
dl4cvvirtual environment prior to running CMake!
If this is the case, access the
dl4cvvirtual environment using
workon dl4cvand re-run the command outlined above.
Compiling OpenCV
Now we are now ready to compile OpenCV :
$ make -j4
Note: If you run into compilation errors, you may run the command
make cleanand then just compile without the flag:
make. You can adjust the number of processor cores you use the compile OpenCV via the
-jswitch (in the example above, I’m compiling OpenCV with four cores).
From there, all you need to do is to install OpenCV 3.3:
$ sudo make install $ sudo ldconfig $ cd ~
You can also delete your
opencvand
opencv_contribdirectories to free up space on your system; however, I highly recommend that you wait until the end of this tutorial and ensured OpenCV has been correctly installed before you delete these files (otherwise you’ll have to download them again).
Symbolic linking OpenCV to your virtual environment
To sym-link our OpenCV bindings into the
dl4cvvirtual environment, issue the following commands
$ cd ~/.virtualenvs/dl4cv/lib/python3.5/site-packages/ $ ln -s /usr/local/lib/python3.5/site-packages/cv2.cpython-35m-x86_64-linux-gnu.so cv2.so $ cd ~
Note: Make sure you click “<=>” button in the toolbar above to expand the code block. From there, ensure you copy and paste the
lncommand correctly, otherwise you’ll create an invalid sym-link and Python will not be able to find your OpenCV bindings.
Your
.sofile may be some variant of what is shown above, so be sure to use the appropriate file.
Testing your OpenCV 3.3 install
Now that we’ve got OpenCV 3.3 installed and linked, let’s do a quick sanity test to see if things work:
$ python >>> import cv2 >>> cv2.__version__ '3.3.0'
Make sure you are in the
dl4cvvirtual environment before firing up Python. You can accomplish this by running
workon dl4cv.
When you print the OpenCV version in your Python shell it should match the version of OpenCV that you installed (in our case OpenCV
3.3.0).
When your compilation is 100% complete you should see output that looks similar to the following:
That’s it — assuming you didn’t have an import error, then you’re ready to go on to Step #6 where we will install Keras.
Step #6: Install Keras
For this step, make sure that you are in the
dl4cvenvironment by issuing the
workon dl4cvcommand.
From there we can install some required computer vision, image processing, and machine learning libraries:
$ pip install scipy matplotlib pillow $ pip install imutils h5py requests progressbar2 $ pip install scikit-learn scikit-image
Next, install Tensorflow (GPU version):
$ pip install tensorflow-gpu
You can verify that TensorFlow has been installed by importing it in your Python shell:
$ python >>> import tensorflow >>>
Now we’re ready to install Keras:
$ pip install keras
Again, you can verify Keras has been installed via your Python shell:
$ python >>> import keras Using TensorFlow backend. >>>
You should see that Keras has been imported with no errors and the TensorFlow backend is being used.
Before you move on to Step #7, take a second to familiarize yourself with the
~/.keras/keras.jsonfile:
{ "image_data_format": "channels_last", "backend": "tensorflow", "epsilon": 1e-07, "floatx": "float32" }
Ensure that
image_data_formatis set to
channels_lastand
backendis
tensorflow.
Congratulations! You are now ready to begin your Deep learning for Computer Vision with Python journey (Starter Bundle and Practitioner Bundle readers can safely skip Step #7).
Step #7 Install mxnet (ImageNet Bundle only)
This step is only required for readers who purchased a copy of the ImageNet Bundle of Deep Learning for Computer Vision with Python. You may also choose to use these instructions if you want to configure mxnet on your system.
Either way, let’s first clone the mxnet repository and checkout branch
0.11.0:
$ cd ~ $ git clone --recursive http://ift.tt/2wjU0qU mxnet --branch 0.11.0
We can them compile mxnet:
$ cd mxnet $ make -j4 USE_OPENCV=1 USE_BLAS=openblas USE_CUDA=1 USE_CUDA_PATH=/usr/local/cuda USE_CUDNN=1
Followed by sym-linking to our dl4cv environment.
$ cd ~/.virtualenvs/dl4cv/lib/python3.5/site-packages/ $ ln -s ~/mxnet/python/mxnet mxnet $ cd ~
Finally, you may fire up Python in your environment to test that the installation was successful:
$ python >>> import mxnet >>>
Note: Do not delete the
mxnetdirectory in your home folder. Not only do our Python bindings live there, but we also need the files in
~/mxnet/binwhen creating serialized image datasets.
Cheers! You are done and deserve a cold beer while you read Deep Learning for Computer Vision with Python (ImageNet bundle).
Note: To avoid significant cloud expenses (or power bills if your box is beneath your desk), I’d recommend that you power off your machine until you’re ready to use it.
Summary
Today we learned how to set up an Ubuntu + CUDA + GPU machine with the tools needed to be successful when training your own deep learning networks.
If you encountered any issues along the way, I highly encourage you to check that you didn’t skip any steps. If you are still stuck, please leave a comment below.
I want to reiterate that you don’t need a fancy, expensive GPU machine to get started on your deep learning for computer vision journey. Your CPU can handle the introductory examples in the book. To help you get started, I have provided an install tutorial here for Ubuntu CPU users. If you prefer the easy, pre-configured route, my book comes with a VirtualBox virtual machine ready to go.
I hope this tutorial helps you on your deep learning journey!
If you want to study deep learning in-depth, be sure to take a look at my new book, Deep Learning for Computer Vision with Python.
To be notified when future blog posts and tutorials are published on the PyImageSearch blog, be sure to enter your email address in the form below!
The post Setting up Ubuntu 16.04 + CUDA + GPU for deep learning with Python appeared first on PyImageSearch.
from PyImageSearch http://ift.tt/2fpUYec
via IFTTT