Latest YouTube Video
Saturday, September 10, 2016
Ocean City, MD's surf is at least 5.16ft high
Ocean City, MD Summary
At 4:00 AM, surf min of 3.94ft. At 10:00 AM, surf min of 4.13ft. At 4:00 PM, surf min of 5.16ft. At 10:00 PM, surf min of 4.88ft.
Surf maximum: 5.56ft (1.7m)
Surf minimum: 5.16ft (1.57m)
Tide height: 1.15ft (0.35m)
Wind direction: SE
Wind speed: 7.72 KTS
from Surfline http://ift.tt/1kVmigH
via IFTTT
biblatex-anonymous
from Google Alert - anonymous http://ift.tt/2cf6dDj
via IFTTT
SKR Anonymous
from Google Alert - anonymous http://ift.tt/2cNxnWP
via IFTTT
Acid Anonymous 8
from Google Alert - anonymous http://ift.tt/2cf1jqW
via IFTTT
[FD] Persistent Cross-Site Scripting in Woocommerce WordPress plugin
Source: Gmail -> IFTTT-> Blogger
[FD] Authorization bypass in InfiniteWP Admin Panel
Source: Gmail -> IFTTT-> Blogger
[FD] Command injection in InfiniteWP Admin Panel
Source: Gmail -> IFTTT-> Blogger
[FD] Reflected Cross-Site Scripting vulnerability in MailPoet Newsletters plugin
Source: Gmail -> IFTTT-> Blogger
manchester utd vs manchester city Live Streaming
from Google Alert - anonymous http://ift.tt/2c80xA5
via IFTTT
Friday, September 9, 2016
PIL filed in Court to Ban ‘Pokémon Go’ in India for Hurting Religious Sentiments
from The Hacker News http://ift.tt/2ch7TLU
via IFTTT
I have a new follower on Twitter
Leonard Kim
Personal Branding Expert | Speaker → https://t.co/cnOinGn1BG ❤️✒️⛳️⌚️✈️⛱⛵️⛰⛺️⛴⛷⚾️ https://t.co/LumHkC2mFx Available for interviews: Hello@LeonardKim.com
Los Angeles
https://t.co/JIXNz6TkE4
Following: 52344 - Followers: 109162
September 09, 2016 at 02:24PM via Twitter http://twitter.com/MrLeonardKim
My Driverless Car's Cool But It Sure Pulls Over For Anonymous Sex A Lot
from Google Alert - anonymous http://ift.tt/2ccS4q2
via IFTTT
Anonymous Infamous
from Google Alert - anonymous http://ift.tt/2cjmg5Y
via IFTTT
ISS Daily Summary Report – 09/08/2016
from ISS On-Orbit Status Report http://ift.tt/2ccqHMJ
via IFTTT
Oh, It's On Sale! USB Kill to Destroy any Computer within Seconds
from The Hacker News http://ift.tt/2cySuHO
via IFTTT
Google Chrome to Label Sensitive HTTP Pages as "Not Secure"
from The Hacker News http://ift.tt/2cpQDI6
via IFTTT
Premier Li lays wreath at Laos' anonymous martyr's monument
from Google Alert - anonymous http://ift.tt/2bYjXpK
via IFTTT
Your Story, anonymous
from Google Alert - anonymous http://ift.tt/2cn1cge
via IFTTT
Mars in the Clouds
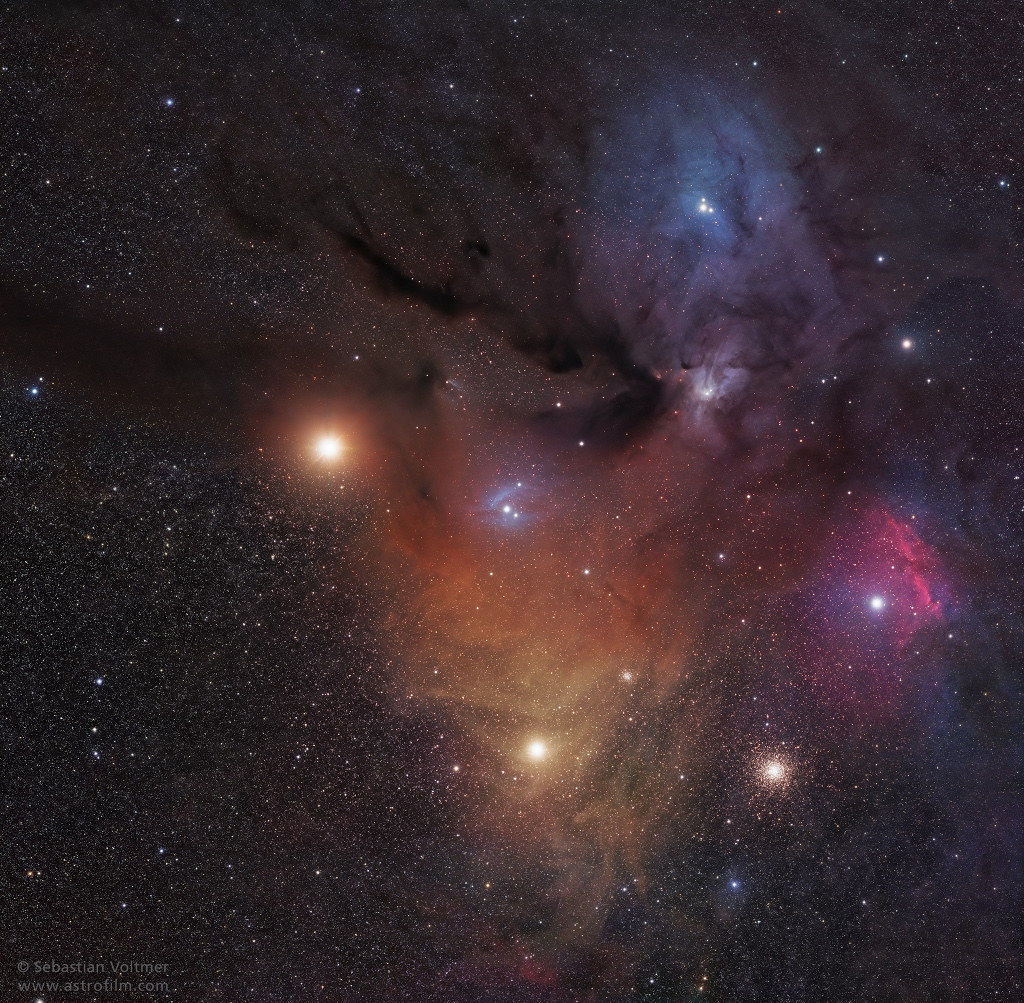
Thursday, September 8, 2016
I have a new follower on Twitter
TOP Step Consulting
TOP Step Consulting improves business efficiency and productivity for Professional Services business operations.
Your Office
http://t.co/v0bQkV4EHL
Following: 2538 - Followers: 3037
September 08, 2016 at 11:45PM via Twitter http://twitter.com/TOPStepTweets
Ask Anonymous
from Google Alert - anonymous http://ift.tt/2cGyzqU
via IFTTT
Random Shuffling and Resets for the Non-stationary Stochastic Bandit Problem. (arXiv:1609.02139v1 [cs.AI])
We consider a non-stationary formulation of the stochastic multi-armed bandit where the rewards are no longer assumed to be identically distributed. For the best-arm identification task, we introduce a version of Successive Elimination based on random shuffling of the $K$ arms. We prove that under a novel and mild assumption on the mean gap $\Delta$, this simple but powerful modification achieves the same guarantees in term of sample complexity and cumulative regret than its original version, but in a much wider class of problems, as it is not anymore constrained to stationary distributions. We also show that the original {\sc Successive Elimination} fails to have controlled regret in this more general scenario, thus showing the benefit of shuffling. We then remove our mild assumption and adapt the algorithm to the best-arm identification task with switching arms. We adapt the definition of the sample complexity for that case and prove that, against an optimal policy with $N-1$ switches of the optimal arm, this new algorithm achieves an expected sample complexity of $O(\Delta^{-2}\sqrt{NK\delta^{-1} \log(K \delta^{-1})})$, where $\delta$ is the probability of failure of the algorithm, and an expected cumulative regret of $O(\Delta^{-1}{\sqrt{NTK \log (TK)}})$ after $T$ time steps.
from cs.AI updates on arXiv.org http://ift.tt/2cxDGZW
via IFTTT
Fitted Learning: Models with Awareness of their Limits. (arXiv:1609.02226v1 [cs.AI])
Though deep learning has pushed the boundaries of classification forward, in recent years hints of the limits of standard classification have begun to emerge. Problems such as fooling, adding new classes over time, and the need to retrain learning models only for small changes to the original problem all point to a potential shortcoming in the classic classification regime, where a comprehensive a priori knowledge of the possible classes or concepts is critical. Without such knowledge, classifiers misjudge the limits of their knowledge and overgeneralization therefore becomes a serious obstacle to consistent performance. In response to these challenges, this paper extends the classic regime by reframing classification instead with the assumption that concepts present in the training set are only a sample of the hypothetical final set of concepts. To bring learning models into this new paradigm, a novel elaboration of standard architectures called the competitive overcomplete output layer (COOL) neural network is introduced. Experiments demonstrate the effectiveness of COOL by applying it to fooling, separable concept learning, one-class neural networks, and standard classification benchmarks. The results suggest that, unlike conventional classifiers, the amount of generalization in COOL networks can be tuned to match the problem.
from cs.AI updates on arXiv.org http://ift.tt/2cxD070
via IFTTT
Backpropagation of Hebbian plasticity for lifelong learning. (arXiv:1609.02228v1 [cs.NE])
Hebbian plasticity allows biological agents to learn from their lifetime experience, extending the fixed information provided by evolutionary search. Conversely, backpropagation methods can build high-performance fixed-weights networks, but are not currently equipped to design networks with Hebbian connections. Here we use backpropagation to train fully-differentiable plastic networks, such that backpropagation determines not only the baseline weights, but also the plasticity of each connection. To perform this backpropagation of Hebbian plasticity (BOHP), we derive error gradients for neural networks with Hebbian plastic connections. The equations for these gradients turn out to follow a simple, recursive form. We apply this method to train small networks for simple learning tasks inspired from classical conditioning. We show that, through Hebbian plasticity, the networks perform fast learning of unpredictable environmental features during their lifetime, successfully solving a task that fixed-weight feedforward networks cannot possibly solve. We conclude that backpropagation of Hebbian plasticity offers a powerful model for lifelong learning.
from cs.AI updates on arXiv.org http://ift.tt/2cxDnyC
via IFTTT
Latent Dependency Forest Models. (arXiv:1609.02236v1 [cs.AI])
Probabilistic modeling is one of the foundations of modern machine learning and artificial intelligence. In this paper, we propose a novel type of probabilistic models named latent dependency forest models (LDFMs). A LDFM models the dependencies between random variables with a forest structure that can change dynamically based on the variable values. It is therefore capable of modeling context-specific independence. We parameterize a LDFM using a first-order non-projective dependency grammar. Learning LDFMs from data can be formulated purely as a parameter learning problem, and hence the difficult problem of model structure learning is circumvented. Our experimental results show that LDFMs are competitive with existing probabilistic models.
from cs.AI updates on arXiv.org http://ift.tt/2cxDR7C
via IFTTT
Ashwin: Plug-and-Play System for Machine-Human Image Annotation. (arXiv:1609.02271v1 [cs.AI])
In this paper, we present an end-to-end machine-human image annotation system where each component can be attached in a plug-and-play fashion. These components include Feature Extraction, Machine Annotation, Task Sampling and Crowd Consensus.
from cs.AI updates on arXiv.org http://ift.tt/2coRUPE
via IFTTT
Ms. Pac-Man Versus Ghost Team CIG 2016 Competition. (arXiv:1609.02316v1 [cs.AI])
This paper introduces the revival of the popular Ms. Pac-Man Versus Ghost Team competition. We present an updated game engine with Partial Observability constraints, a new Multi-Agent Systems approach to developing Ghost agents and several sample controllers to ease the development of entries. A restricted communication protocol is provided for the Ghosts, providing a more challenging environment than before. The competition will debut at the IEEE Computational Intelligence and Games Conference 2016. Some preliminary results showing the effects of Partial Observability and the benefits of simple communication are also presented.
from cs.AI updates on arXiv.org http://ift.tt/2c9Dwsj
via IFTTT
Latest Datasets and Technologies Presented in the Workshop on Grasping and Manipulation Datasets. (arXiv:1609.02531v1 [cs.RO])
This paper reports the activities and outcomes in the Workshop on Grasping and Manipulation Datasets that was organized under the International Conference on Robotics and Automation (ICRA) 2016. The half day workshop was packed with nine invited talks, 12 interactive presentations, and one panel discussion with ten panelists. This paper summarizes all the talks and presentations and recaps what has been discussed in the panels session. This summary servers as a review of recent developments in data collection in grasping and manipulation. Many of the presentations describe ongoing efforts or explorations that could be achieved and fully available in a year or two. The panel discussion not only commented on the current approaches, but also indicates new directions and focuses. The workshop clearly displayed the importance of quality datasets in robotics and robotic grasping and manipulation field. Hopefully the workshop could motivate larger efforts to create big datasets that are comparable with big datasets in other communities such as computer vision.
from cs.AI updates on arXiv.org http://ift.tt/2cmITb7
via IFTTT
Combining Monte-Carlo and Hyper-heuristic methods for the Multi-mode Resource-constrained Multi-project Scheduling Problem. (arXiv:1511.04387v2 [cs.DS] UPDATED)
Multi-mode resource and precedence-constrained project scheduling is a well-known challenging real-world optimisation problem. An important variant of the problem requires scheduling of activities for multiple projects considering availability of local and global resources while respecting a range of constraints. A critical aspect of the benchmarks addressed in this paper is that the primary objective is to minimise the sum of the project completion times, with the usual makespan minimisation as a secondary objective. We observe that this leads to an expected different overall structure of good solutions and discuss the effects this has on the algorithm design. This paper presents a carefully designed hybrid of Monte-Carlo tree search, novel neighbourhood moves, memetic algorithms, and hyper-heuristic methods. The implementation is also engineered to increase the speed with which iterations are performed, and to exploit the computing power of multicore machines. Empirical evaluation shows that the resulting information-sharing multi-component algorithm significantly outperforms other solvers on a set of "hidden" instances, i.e. instances not available at the algorithm design phase.
from cs.AI updates on arXiv.org http://ift.tt/1NyCIHb
via IFTTT
True Online Temporal-Difference Learning. (arXiv:1512.04087v2 [cs.AI] UPDATED)
The temporal-difference methods TD($\lambda$) and Sarsa($\lambda$) form a core part of modern reinforcement learning. Their appeal comes from their good performance, low computational cost, and their simple interpretation, given by their forward view. Recently, new versions of these methods were introduced, called true online TD($\lambda$) and true online Sarsa($\lambda$), respectively (van Seijen & Sutton, 2014). These new versions maintain an exact equivalence with the forward view at all times, whereas the traditional versions only approximate it for small step-sizes. We hypothesize that these true online methods not only have better theoretical properties, but also dominate the regular methods empirically. In this article, we put this hypothesis to the test by performing an extensive empirical comparison. Specifically, we compare the performance of true online TD($\lambda$)/Sarsa($\lambda$) with regular TD($\lambda$)/Sarsa($\lambda$) on random MRPs, a real-world myoelectric prosthetic arm, and a domain from the Arcade Learning Environment. We use linear function approximation with tabular, binary, and non-binary features. Our results suggest that the true online methods indeed dominate the regular methods. Across all domains/representations the learning speed of the true online methods are often better, but never worse than that of the regular methods. An additional advantage is that no choice between traces has to be made for the true online methods. Besides the empirical results, we provide an in-depth analysis of the theory behind true online temporal-difference learning. In addition, we show that new true online temporal-difference methods can be derived by making changes to the online forward view and then rewriting the update equations.
from cs.AI updates on arXiv.org http://ift.tt/1YbxOuA
via IFTTT
A Notation for Markov Decision Processes. (arXiv:1512.09075v2 [cs.AI] UPDATED)
This paper specifies a notation for Markov decision processes.
from cs.AI updates on arXiv.org http://ift.tt/1R9uxGn
via IFTTT
Deep Reinforcement Learning with a Combinatorial Action Space for Predicting Popular Reddit Threads. (arXiv:1606.03667v3 [cs.CL] UPDATED)
We introduce an online popularity prediction and tracking task as a benchmark task for reinforcement learning with a combinatorial, natural language action space. A specified number of discussion threads predicted to be popular are recommended, chosen from a fixed window of recent comments to track. Novel deep reinforcement learning architectures are studied for effective modeling of the value function associated with actions comprised of interdependent sub-actions. The proposed model, which represents dependence between sub-actions through a bi-directional LSTM, gives the best performance across different experimental configurations and domains, and it also generalizes well with varying numbers of recommendation requests.
from cs.AI updates on arXiv.org http://ift.tt/1UgLwrc
via IFTTT
Title Generation for User Generated Videos. (arXiv:1608.07068v2 [cs.CV] UPDATED)
A great video title describes the most salient event compactly and captures the viewer's attention. In contrast, video captioning tends to generate sentences that describe the video as a whole. Although generating a video title automatically is a very useful task, it is much less addressed than video captioning. We address video title generation for the first time by proposing two methods that extend state-of-the-art video captioners to this new task. First, we make video captioners highlight sensitive by priming them with a highlight detector. Our framework allows for jointly training a model for title generation and video highlight localization. Second, we induce high sentence diversity in video captioners, so that the generated titles are also diverse and catchy. This means that a large number of sentences might be required to learn the sentence structure of titles. Hence, we propose a novel sentence augmentation method to train a captioner with additional sentence-only examples that come without corresponding videos. We collected a large-scale Video Titles in the Wild (VTW) dataset of 18100 automatically crawled user-generated videos and titles. On VTW, our methods consistently improve title prediction accuracy, and achieve the best performance in both automatic and human evaluation. Finally, our sentence augmentation method also outperforms the baselines on the M-VAD dataset.
from cs.AI updates on arXiv.org http://ift.tt/2ccvuCG
via IFTTT
Error message when adding a reminder as anonymous user
from Google Alert - anonymous http://ift.tt/2bWkvHP
via IFTTT
[FD] AST-2016-006: Crash on ACK from unknown endpoint
Source: Gmail -> IFTTT-> Blogger
FBI Arrests Two Hackers Who Hacked US Spy Chief, FBI and CIA Director
from The Hacker News http://ift.tt/2cm8uRn
via IFTTT
[FD] CVE request - Samsumg Mobile Phone SVE-2016-6248: SystemUI Security issue
Source: Gmail -> IFTTT-> Blogger
[FD] CVE-2016-4264 Adobe ColdFusion <= 11 XXE Vulnerability
Source: Gmail -> IFTTT-> Blogger
[FD] cve request: Airmail URLScheme render and file:// xss vulnerability
Source: Gmail -> IFTTT-> Blogger
[FD] ELNet Energy & Electrical Power Meter - Mulitple Vulnerabilities
Source: Gmail -> IFTTT-> Blogger
[FD] Multiple vulnerabilities - Powerlogic/Schneider Electric IONXXXX series Smart Meters
Source: Gmail -> IFTTT-> Blogger
[FD] Persistent Cross-Site Scripting vulnerability in WordPress due to unsafe processing of file names
Source: Gmail -> IFTTT-> Blogger
I have a new follower on Twitter
Hacker Ventures
Team of hustlers and hackers. Increasing our productivity with https://t.co/DLEkgUUVw2 and innovating daily.
https://t.co/DLEkgUUVw2
Following: 10952 - Followers: 15935
September 08, 2016 at 12:36PM via Twitter http://twitter.com/HackerVentures
Anonymous Survey but Possible Prize
from Google Alert - anonymous http://ift.tt/2c1chBI
via IFTTT
anonymous event creation does not work
from Google Alert - anonymous http://ift.tt/2cw7giM
via IFTTT
Map not visible for anonymous users when using AdvAgg js compression
from Google Alert - anonymous http://ift.tt/2cvXBIO
via IFTTT
I have a new follower on Twitter
Erik Fisher
Social Media Manager @SMExaminer | Productivity Podcaster @ https://t.co/cKnTdmuVKn | Follow me for News & How-to's on Social Media, Productivity & Podcasting
Indiana & The Interwebs
https://t.co/cKnTdmuVKn
Following: 23401 - Followers: 40284
September 08, 2016 at 06:50AM via Twitter http://twitter.com/ErikJFisher
Anonymous Has a New Message for the World
from Google Alert - anonymous http://ift.tt/2cE5a0M
via IFTTT
[FD] PHPHolidays CMS v3.00.50 - Cross Site Scripting Web Vulnerability
Source: Gmail -> IFTTT-> Blogger
[FD] Picosmos Shows v1.6.0 - Stack Buffer Overflow Vulnerability
Source: Gmail -> IFTTT-> Blogger
Tip-offs Anonymous and Trends
from Google Alert - anonymous http://ift.tt/2bVWYvn
via IFTTT
Warning: Mokes Cross-Platform Malware Can Hack Windows, Linux and OS X Systems
from The Hacker News http://ift.tt/2c8Y7Me
via IFTTT
Eclipse to Sunset
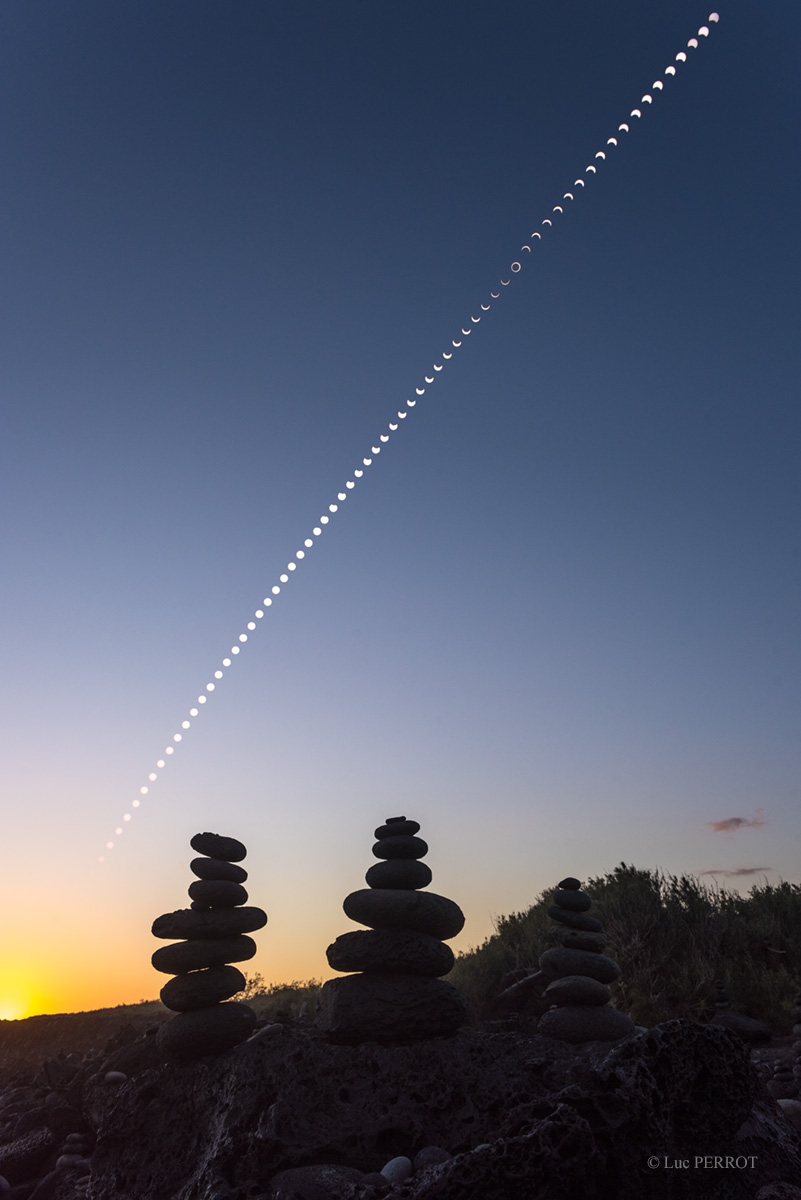
Wednesday, September 7, 2016
I have a new follower on Twitter
RushMillerFoundation
We put Blind/Visually Impaired (BVI) Kids butts on tandem bikes Dig hearing about BVI Tech - Cool BVI Orgs/Individuals - Crowdfunding - Social Entrepreneurism
Pueblo, Colorado USA
http://t.co/CIONv07BXN
Following: 15255 - Followers: 17102
September 07, 2016 at 10:34PM via Twitter http://twitter.com/RushMillerFound
Automation of Pedestrian Tracking in a Crowded Situation. (arXiv:1609.01710v1 [cs.CV])
Studies on microscopic pedestrian requires large amounts of trajectory data from real-world pedestrian crowds. Such data collection, if done manually, needs tremendous effort and is very time consuming. Though many studies have asserted the possibility of automating this task using video cameras, we found that only a few have demonstrated good performance in very crowded situations or from a top-angled view scene. This paper deals with tracking pedestrian crowd under heavy occlusions from an angular scene. Our automated tracking system consists of two modules that perform sequentially. The first module detects moving objects as blobs. The second module is a tracking system. We employ probability distribution from the detection of each pedestrian and use Bayesian update to track the next position. The result of such tracking is a database of pedestrian trajectories over time and space. With certain prior information, we showed that the system can track a large number of people under occlusion and clutter scene.
from cs.AI updates on arXiv.org http://ift.tt/2cHdFvK
via IFTTT
A modular architecture for transparent computation in Recurrent Neural Networks. (arXiv:1609.01926v1 [cs.NE])
Computation is classically studied in terms of automata, formal languages and algorithms; yet, the relation between neural dynamics and symbolic representations and operations is still unclear in traditional eliminative connectionism. Therefore, we suggest a unique perspective on this central issue, to which we would like to refer as to transparent connectionism, by proposing accounts of how symbolic computation can be implemented in neural substrates. In this study we first introduce a new model of dynamics on a symbolic space, the versatile shift, showing that it supports the real-time simulation of a range of automata. We then show that the Goedelization of versatile shifts defines nonlinear dynamical automata, dynamical systems evolving on a vectorial space. Finally, we present a mapping between nonlinear dynamical automata and recurrent artificial neural networks. The mapping defines an architecture characterized by its granular modularity, where data, symbolic operations and their control are not only distinguishable in activation space, but also spatially localizable in the network itself, while maintaining a distributed encoding of symbolic representations. The resulting networks simulate automata in real-time and are programmed directly, in absence of network training. To discuss the unique characteristics of the architecture and their consequences, we present two examples: i) the design of a Central Pattern Generator from a finite-state locomotive controller, and ii) the creation of a network simulating a system of interactive automata that supports the parsing of garden-path sentences as investigated in psycholinguistics experiments.
from cs.AI updates on arXiv.org http://ift.tt/2c691Ut
via IFTTT
Unifying task specification in reinforcement learning. (arXiv:1609.01995v1 [cs.AI])
Reinforcement learning tasks are typically specified as Markov decision processes. This formalism has been highly successful, though specifications often couple the dynamics of the environment and the learning objective. This lack of modularity can complicate generalization of the task specification, as well as obfuscate connections between different task settings, such as episodic and continuing. In this work, we introduce the RL task formalism, that provides a unification through simple constructs including a generalization to transition-based discounting. Through a series of examples, we demonstrate the generality and utility of this formalism. Finally, we extend standard learning constructs, including Bellman operators, and extend some seminal theoretical results, including approximation errors bounds. Overall, we provide a well-understood and sound formalism on which to build theoretical results and simplify algorithm use and development.
from cs.AI updates on arXiv.org http://ift.tt/2c8rGgQ
via IFTTT
Non-Evolutionary Superintelligences Do Nothing, Eventually. (arXiv:1609.02009v1 [cs.AI])
There is overwhelming evidence that human intelligence is a product of Darwinian evolution. Investigating the consequences of self-modification, and more precisely, the consequences of utility function self-modification, leads to the stronger claim that not only human, but any form of intelligence is ultimately only possible within evolutionary processes. Human-designed artificial intelligences can only remain stable until they discover how to manipulate their own utility function. By definition, a human designer cannot prevent a superhuman intelligence from modifying itself, even if protection mechanisms against this action are put in place. Without evolutionary pressure, sufficiently advanced artificial intelligences become inert by simplifying their own utility function. Within evolutionary processes, the implicit utility function is always reducible to persistence, and the control of superhuman intelligences embedded in evolutionary processes is not possible. Mechanisms against utility function self-modification are ultimately futile. Instead, scientific effort toward the mitigation of existential risks from the development of superintelligences should be in two directions: understanding consciousness, and the complex dynamics of evolutionary systems.
from cs.AI updates on arXiv.org http://ift.tt/2cHdroz
via IFTTT
Equilibrium Graphs. (arXiv:1609.02010v1 [cs.AI])
In this paper we present an extension of Peirce's existential graphs to provide a diagrammatic representation of expressions in Quantified Equilibrium Logic (QEL). Using this formalisation, logical connectives are replaced by encircled regions (circles and squares) and quantified variables are represented as "identity" lines. Although the expressive power is equivalent to that of QEL, the new representation can be useful for illustrative or educational purposes.
from cs.AI updates on arXiv.org http://ift.tt/2c8qb2f
via IFTTT
Deep Markov Random Field for Image Modeling. (arXiv:1609.02036v1 [cs.CV])
Markov Random Fields (MRFs), a formulation widely used in generative image modeling, have long been plagued by the lack of expressive power. This issue is primarily due to the fact that conventional MRFs formulations tend to use simplistic factors to capture local patterns. In this paper, we move beyond such limitations, and propose a novel MRF model that uses fully-connected neurons to express the complex interactions among pixels. Through theoretical analysis, we reveal an inherent connection between this model and recurrent neural networks, and thereon derive an approximated feed-forward network that couples multiple RNNs along opposite directions. This formulation combines the expressive power of deep neural networks and the cyclic dependency structure of MRF in a unified model, bringing the modeling capability to a new level. The feed-forward approximation also allows it to be efficiently learned from data. Experimental results on a variety of low-level vision tasks show notable improvement over state-of-the-arts.
from cs.AI updates on arXiv.org http://ift.tt/2cHbvMM
via IFTTT
Feasibility of Post-Editing Speech Transcriptions with a Mismatched Crowd. (arXiv:1609.02043v1 [cs.AI])
Manual correction of speech transcription can involve a selection from plausible transcriptions. Recent work has shown the feasibility of employing a mismatched crowd for speech transcription. However, it is yet to be established whether a mismatched worker has sufficiently fine-granular speech perception to choose among the phonetically proximate options that are likely to be generated from the trellis of an ASRU. Hence, we consider five languages, Arabic, German, Hindi, Russian and Spanish. For each we generate synthetic, phonetically proximate, options which emulate post-editing scenarios of varying difficulty. We consistently observe non-trivial crowd ability to choose among fine-granular options.
from cs.AI updates on arXiv.org http://ift.tt/2c69Hcj
via IFTTT
UberNet: Training a `Universal' Convolutional Neural Network for Low-, Mid-, and High-Level Vision using Diverse Datasets and Limited Memory. (arXiv:1609.02132v1 [cs.CV])
In this work we introduce a convolutional neural network (CNN) that jointly handles low-, mid-, and high-level vision tasks in a unified architecture that is trained end-to-end. Such a universal network can act like a `swiss knife' for vision tasks; we call this architecture an UberNet to indicate its overarching nature.
We address two main technical challenges that emerge when broadening up the range of tasks handled by a single CNN: (i) training a deep architecture while relying on diverse training sets and (ii) training many (potentially unlimited) tasks with a limited memory budget. Properly addressing these two problems allows us to train accurate predictors for a host of tasks, without compromising accuracy.
Through these advances we train in an end-to-end manner a CNN that simultaneously addresses (a) boundary detection (b) normal estimation (c) saliency estimation (d) semantic segmentation (e) human part segmentation (f) semantic boundary detection, (g) region proposal generation and object detection. We obtain competitive performance while jointly addressing all of these tasks in 0.7 seconds per frame on a single GPU. A demonstration of this system can be found at this http URL
from cs.AI updates on arXiv.org http://ift.tt/2c8pZjv
via IFTTT
Truth Serums for Massively Crowdsourced Evaluation Tasks. (arXiv:1507.07045v2 [cs.GT] UPDATED)
A major challenge in crowdsourcing evaluation tasks like labeling objects, grading assignments in online courses, etc., is that of eliciting truthful responses from agents in the absence of verifiability. In this paper, we propose new reward mechanisms for such settings that, unlike many previously studied mechanisms, impose minimal assumptions on the structure and knowledge of the underlying generating model, can account for heterogeneity in the agents' abilities, require no extraneous elicitation from them, and furthermore allow their beliefs to be (almost) arbitrary. These mechanisms have the simple and intuitive structure of an output agreement mechanism: an agent gets a reward if her evaluation matches that of her peer, but unlike the classic output agreement mechanism, this reward is not the same across evaluations, but is inversely proportional to an appropriately defined popularity index of each evaluation. The popularity indices are computed by leveraging the existence of a large number of similar tasks, which is a typical characteristic of these settings. Experiments performed on MTurk workers demonstrate higher efficacy (with a $p$-value of $0.02$) of these mechanisms in inducing truthful behavior compared to the state of the art.
from cs.AI updates on arXiv.org http://ift.tt/1fBCcOR
via IFTTT
Feature Based Task Recommendation in Crowdsourcing with Implicit Observations. (arXiv:1602.03291v2 [cs.AI] UPDATED)
Existing research in crowdsourcing has investigated how to recommend tasks to workers based on which task the workers have already completed, referred to as {\em implicit feedback}. We, on the other hand, investigate the task recommendation problem, where we leverage both implicit feedback and explicit features of the task. We assume that we are given a set of workers, a set of tasks, interactions (such as the number of times a worker has completed a particular task), and the presence of explicit features of each task (such as, task location). We intend to recommend tasks to the workers by exploiting the implicit interactions, and the presence or absence of explicit features in the tasks. We formalize the problem as an optimization problem, propose two alternative problem formulations and respective solutions that exploit implicit feedback, explicit features, as well as similarity between the tasks. We compare the efficacy of our proposed solutions against multiple state-of-the-art techniques using two large scale real world datasets.
from cs.AI updates on arXiv.org http://ift.tt/1KcDjDf
via IFTTT
A Repeated Signal Difference for Recognising Patterns. (arXiv:1604.05170v3 [cs.NE] UPDATED)
This paper describes a new mechanism that might help with defining pattern sequences, by the fact that it can produce an upper bound on the ensemble value that can persistently oscillate with the actual values produced from each pattern. With every firing event, a node also receives an on/off feedback switch. If the node fires, then it sends a feedback result depending on the input signal strength. If the input signal is positive or larger, it can store an 'on' switch feedback for the next iteration. If the signal is negative or smaller, it can store an 'off' switch feedback for the next iteration. If the node does not fire, then it does not affect the current feedback situation and receives the switch command produced by the last active pattern event for the same neuron. The upper bound therefore also represents the largest or most enclosing pattern set and the lower value is for the actual set of firing patterns. If the pattern sequence repeats, it will oscillate between the two values, allowing them to be recognised and measured more easily, over time. Tests show that changing the sequence ordering produces different value sets, which can also be measured.
from cs.AI updates on arXiv.org http://ift.tt/23UuRhj
via IFTTT
Anonymous scout dishes on Dallas Cowboys' most overrated, underrated players
from Google Alert - anonymous http://ift.tt/2cq3qIV
via IFTTT
Orioles Podcast: Manager Buck Showalter tells Baseball Tonight "we enjoy making people scratch their heads" with success (ESPN)
via IFTTT
Anonymous Voter Registration: Domestic Violence Victims
from Google Alert - anonymous http://ift.tt/2c5DYrE
via IFTTT
Orioles: 1B Chris Davis out of Wednesday's starting lineup vs. Rays due to sore left hand; hitting .229, 35 HR in 2016 (ESPN)
via IFTTT
Ravens: Elvis Dumervil (foot) says he won't be able to play in season opener vs. Bills after a minor setback in recovery (ESPN)
via IFTTT
Anonymous user a688ef
from Google Alert - anonymous http://ift.tt/2bYeZrD
via IFTTT
ISS Daily Summary Report – 09/06/2016
from ISS On-Orbit Status Report http://ift.tt/2ccQEif
via IFTTT
Here’s How to Hack Windows/Mac OS X Login Password (When Locked)
from The Hacker News http://ift.tt/2chb4rT
via IFTTT
Warning! Just an Image Can Hack Your Android Phone — Patch Now
from The Hacker News http://ift.tt/2bSsdCK
via IFTTT
Uncaught reflect-metadata shim is required when using class decorators
from Google Alert - anonymous http://ift.tt/2cnM4wf
via IFTTT
The Whirlpool Galaxy and Beyond
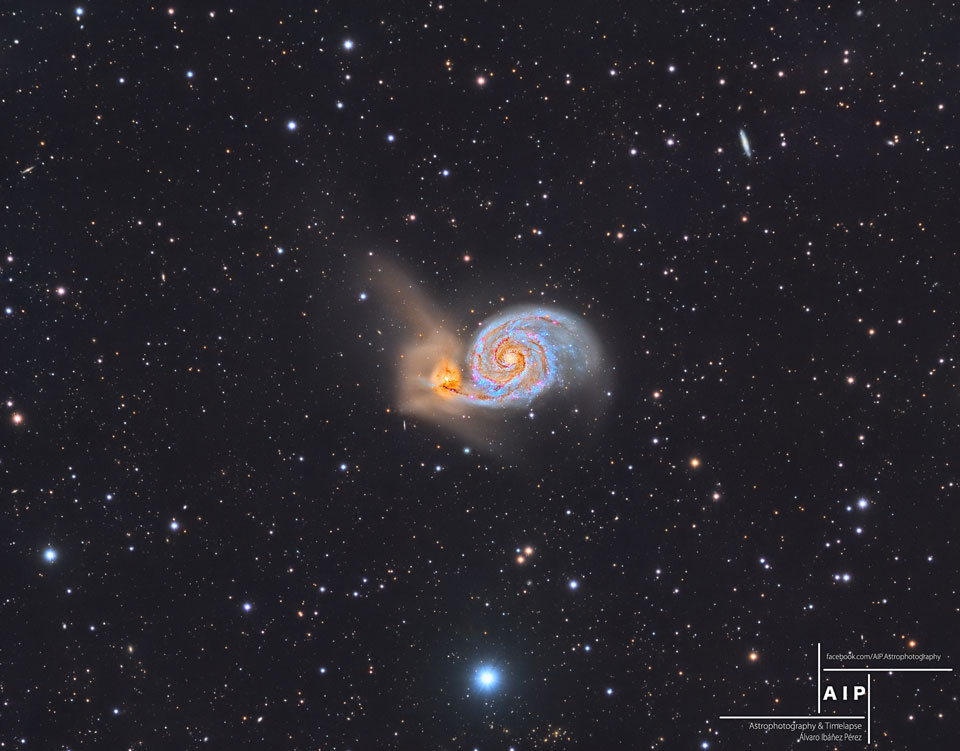
Tuesday, September 6, 2016
Orioles Video: Manny Machado's 34th HR of the season is a towering grand slam in the 4th inning of 11-2 win at Rays (ESPN)
via IFTTT
Check out our slate of Daily Kos-endorsed candidates
from Google Alert - anonymous http://ift.tt/2cEQoe9
via IFTTT
Deviant Learning Algorithm: Learning Sparse Mismatch Representations through Time and Space. (arXiv:1609.01459v1 [cs.AI])
Predictive coding (PDC) has recently attracted attention in the neuroscience and computing community as a candidate unifying paradigm for neuronal studies and artificial neural network implementations particularly targeted at unsupervised learning systems. The Mismatch Negativity (MMN) has also recently been studied in relation to PC and found to be a useful ingredient in neural predictive coding systems. Backed by the behavior of living organisms, such networks are particularly useful in forming spatio-temporal transitions and invariant representations of the input world. However, most neural systems still do not account for large number of synapses even though this has been shown by a few machine learning researchers as an effective and very important component of any neural system if such a system is to behave properly. Our major point here is that PDC systems with the MMN effect in addition to a large number of synapses can greatly improve any neural learning system's performance and ability to make decisions in the machine world. In this paper, we propose a novel bio-mimetic computational intelligence algorithm -- the Deviant Learning Algorithm, inspired by these key ideas and functional properties of recent brain-cognitive discoveries and theories. We also show by numerical experiments guided by theoretical insights, how our invented bio-mimetic algorithm can achieve competitive predictions with even with very small problem specific data.
from cs.AI updates on arXiv.org http://ift.tt/2cqVki6
via IFTTT
Q-Learning with Basic Emotions. (arXiv:1609.01468v1 [cs.AI])
Q-learning is a simple and powerful tool in solving dynamic problems where environments are unknown. It uses a balance of exploration and exploitation to find an optimal solution to the problem. In this paper, we propose using four basic emotions: joy, sadness, fear, and anger to influence a Qlearning agent. Simulations show that the proposed affective agent requires lesser number of steps to find the optimal path. We found when affective agent finds the optimal path, the ratio between exploration to exploitation gradually decreases, indicating lower total step count in the long run
from cs.AI updates on arXiv.org http://ift.tt/2czjsje
via IFTTT
OpenTripPlanner, OpenStreetMap, General Transit Feed Specification: Tools for Disaster Relief and Recovery. (arXiv:1609.01472v1 [cs.CY])
Open Trip Planner was identified as the most promising open source multi-modal trip planning software. Open Street Map, which provides mapping data to Open Trip Planner, is one of the most well-known open source international repository of geographic data. General Transit Feed Specification, which provides transportation data to Open Trip Planner, has been the standard for describing transit systems and platform for numerous applications. Together, when used to implement an instance of Open Trip Planner, these software has been helping in traffic decongestion all over the world by assisting commuters to shift from using private transportation modes to public ones. Their potential however goes beyond providing multi-modal public transportation routes. This paper aims to first discuss the researchers' experience in implementing a public transportation route planner for the purpose of traffic decongestion.The researchers would examine the prospective of using the system for disaster preparedness and recovery and concrete ways on how to realize them.
from cs.AI updates on arXiv.org http://ift.tt/2cilJla
via IFTTT
Multi Exit Configuration of Mesoscopic Pedestrian Simulation. (arXiv:1609.01475v1 [cs.MA])
A mesoscopic approach to modeling pedestrian simulation with multiple exits is proposed in this paper. A floor field based on Qlearning Algorithm is used. Attractiveness of exits to pedestrian typically is based on shortest path. However, several factors may influence pedestrian choice of exits. Scenarios with multiple exits are presented and effect of Q-learning rewards system on navigation is investigated
from cs.AI updates on arXiv.org http://ift.tt/2cqVZQs
via IFTTT
Axiomatizing Category Theory in Free Logic. (arXiv:1609.01493v1 [cs.LO])
Starting from a generalization of the standard axioms for a monoid we present a stepwise development of various, mutually equivalent foundational axiom systems for category theory. Our axiom sets have been formalized in the Isabelle/HOL interactive proof assistant, and this formalization utilizes a semantically correct embedding of free logic in classical higher-order logic. The modeling and formal analysis of our axiom sets has been significantly supported by series of experiments with automated reasoning tools integrated with Isabelle/HOL. We also address the relation of our axiom systems to alternative proposals from the literature, including an axiom set proposed by Freyd and Scedrov for which we reveal a technical flaw: either all operations, e.g. morphism composition, are total or their axiom system is inconsistent. The repair for this problem is quite straightforward, however.
from cs.AI updates on arXiv.org http://ift.tt/2cilbLW
via IFTTT
Lie Access Neural Turing Machine. (arXiv:1602.08671v3 [cs.NE] UPDATED)
Following the recent trend in explicit neural memory structures, we present a new design of an external memory, wherein memories are stored in an Euclidean key space $\mathbb R^n$. An LSTM controller performs read and write via specialized read and write heads. It can move a head by either providing a new address in the key space (aka random access) or moving from its previous position via a Lie group action (aka Lie access). In this way, the "L" and "R" instructions of a traditional Turing Machine are generalized to arbitrary elements of a fixed Lie group action. For this reason, we name this new model the Lie Access Neural Turing Machine, or LANTM.
We tested two different configurations of LANTM against an LSTM baseline in several basic experiments. We found the right configuration of LANTM to outperform the baseline in all of our experiments. In particular, we trained LANTM on addition of $k$-digit numbers for $2 \le k \le 16$, but it was able to generalize almost perfectly to $17 \le k \le 32$, all with the number of parameters 2 orders of magnitude below the LSTM baseline.
from cs.AI updates on arXiv.org http://ift.tt/1XWzjsL
via IFTTT
2016 Preview: Ravens have better than a 65% chance to win 5 games on schedule and a 39.1% chance to make the playoffs (ESPN)
via IFTTT
ISS Daily Summary Report – 09/05/2016
from ISS On-Orbit Status Report http://ift.tt/2c11lCF
via IFTTT
Incorrect autofix of anonymous function body in Drupal.WhiteSpace.ScopeClosingBrace
from Google Alert - anonymous http://ift.tt/2bQGGUO
via IFTTT
ISS Daily Summary Report – 09/02/2016
from ISS On-Orbit Status Report http://ift.tt/2c9xUjJ
via IFTTT
[FD] SEC Consult SA-20160906-0 :: Private key for browser-trusted certificate embedded in multiple Aruba Networks / Alcatel-Lucent products
Source: Gmail -> IFTTT-> Blogger
Russia's Largest Portal HACKED; Nearly 100 Million Plaintext Passwords Leaked
from The Hacker News http://ift.tt/2bPvIdj
via IFTTT
Spiral Meteor through the Heart Nebula
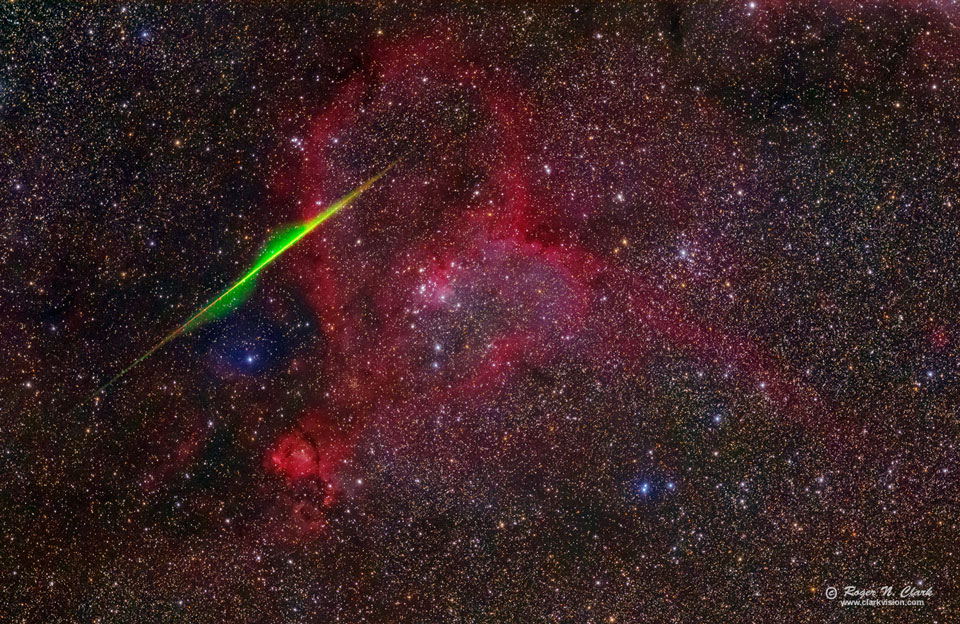
Monday, September 5, 2016
I have a new follower on Twitter
Exonar
Organise your Organisation's Information
Silicon Canal, Newbury
https://t.co/WuvRwoE62d
Following: 1014 - Followers: 531
September 05, 2016 at 11:54PM via Twitter http://twitter.com/exonar
A MIP Backend for the IDP System. (arXiv:1609.00759v1 [cs.AI])
The IDP knowledge base system currently uses MiniSAT(ID) as its backend Constraint Programming (CP) solver. A few similar systems have used a Mixed Integer Programming (MIP) solver as backend. However, so far little is known about when the MIP solver is preferable. This paper explores this question. It describes the use of CPLEX as a backend for IDP and reports on experiments comparing both backends.
from cs.AI updates on arXiv.org http://ift.tt/2bZwKp3
via IFTTT
An Online Universal Classifier for Binary, Multi-class and Multi-label Classification. (arXiv:1609.00843v1 [cs.LG])
Classification involves the learning of the mapping function that associates input samples to corresponding target label. There are two major categories of classification problems: Single-label classification and Multi-label classification. Traditional binary and multi-class classifications are sub-categories of single-label classification. Several classifiers are developed for binary, multi-class and multi-label classification problems, but there are no classifiers available in the literature capable of performing all three types of classification. In this paper, a novel online universal classifier capable of performing all the three types of classification is proposed. Being a high speed online classifier, the proposed technique can be applied to streaming data applications. The performance of the developed classifier is evaluated using datasets from binary, multi-class and multi-label problems. The results obtained are compared with state-of-the-art techniques from each of the classification types.
from cs.AI updates on arXiv.org http://ift.tt/2casp5R
via IFTTT
High Dimensional Human Guided Machine Learning. (arXiv:1609.00904v1 [cs.AI])
Have you ever looked at a machine learning classification model and thought, I could have made that? Well, that is what we test in this project, comparing XGBoost trained on human engineered features to training directly on data. The human engineered features do not outperform XGBoost trained di- rectly on the data, but they are comparable. This project con- tributes a novel method for utilizing human created classifi- cation models on high dimensional datasets.
from cs.AI updates on arXiv.org http://ift.tt/2bZwnuO
via IFTTT
Spectral learning of dynamic systems from nonequilibrium data. (arXiv:1609.00932v1 [cs.LG])
Observable operator models (OOMs) and related models are one of the most important and powerful tools for modeling and analyzing stochastic systems. They can exactly describe dynamics of finite-rank systems, and be efficiently learned from data by moment based algorithms. Almost all OOM learning algorithms are developed based on the assumption of equilibrium data which is very difficult to guarantee in real life, especially for complex processes with large time scales. In this paper, we derive a nonequilibrium learning algorithm for OOMs, which dismisses this assumption and can effectively extract the equilibrium dynamics of a system from nonequilibrium observation data. In addition, we propose binless OOMs for the application of nonequilibrium learning to continuous-valued systems. In comparison with the other OOMs with continuous observations, binless OOMs can achieve consistent estimation from nonequilibrium data with only linear computational complexity.
from cs.AI updates on arXiv.org http://ift.tt/2carJgV
via IFTTT
Multi-Label Classification Method Based on Extreme Learning Machines. (arXiv:1608.08435v1 [cs.LG] CROSS LISTED)
In this paper, an Extreme Learning Machine (ELM) based technique for Multi-label classification problems is proposed and discussed. In multi-label classification, each of the input data samples belongs to one or more than one class labels. The traditional binary and multi-class classification problems are the subset of the multi-label problem with the number of labels corresponding to each sample limited to one. The proposed ELM based multi-label classification technique is evaluated with six different benchmark multi-label datasets from different domains such as multimedia, text and biology. A detailed comparison of the results is made by comparing the proposed method with the results from nine state of the arts techniques for five different evaluation metrics. The nine methods are chosen from different categories of multi-label methods. The comparative results shows that the proposed Extreme Learning Machine based multi-label classification technique is a better alternative than the existing state of the art methods for multi-label problems.
from cs.AI updates on arXiv.org http://ift.tt/2bUdcDe
via IFTTT