Latest YouTube Video
Saturday, December 24, 2016
Anonymous
from Google Alert - anonymous http://ift.tt/2iqkp2Z
via IFTTT
I have a new follower on Twitter
Viptela
Viptela provides Enterprise Grade SD-WAN technology that allows global companies to build cloud-aware, secure and policy-driven WAN infrastructure.
San Jose, CA
http://t.co/WOkqjt1XPh
Following: 6077 - Followers: 5590
December 24, 2016 at 07:28PM via Twitter http://twitter.com/Viptela
Not working with anonymous post
from Google Alert - anonymous http://ift.tt/2ipQuYS
via IFTTT
Cheaters Anonymous Lacey Silks $3.99
from Google Alert - anonymous http://ift.tt/2i5UzAH
via IFTTT
Hackers threaten to take down Xbox Live and PSN on Christmas Day
from The Hacker News http://ift.tt/2hB71Fg
via IFTTT
Once Upon a Solstice Eve
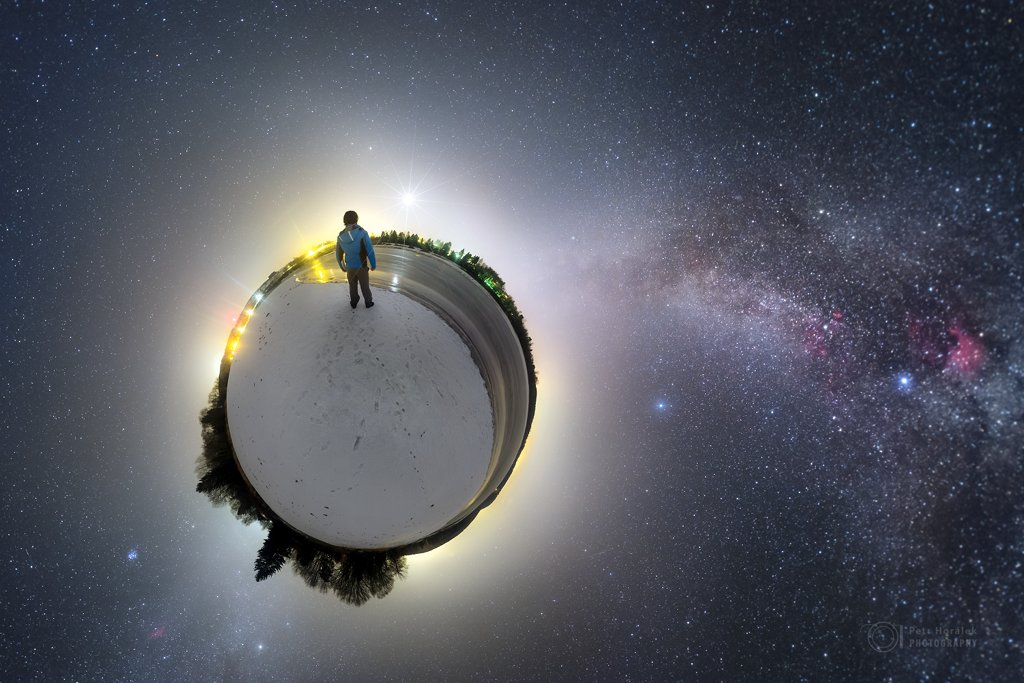
Friday, December 23, 2016
Ravens: WR Steve Smith Sr. (thigh) listed as questionable for Sunday's game vs. Steelers; CB Jimmy Smith (ankle) out (ESPN)
via IFTTT
Anonymous Mask Icon
from Google Alert - anonymous http://ift.tt/2i2WS7s
via IFTTT
[FD] [RT-SA-2016-001] Padding Oracle in Apache mod_session_crypto
Source: Gmail -> IFTTT-> Blogger
BIX Certificates: Cryptographic Tokens for Anonymous Transactions Based on Certificates Public ...
from Google Alert - anonymous http://ift.tt/2i90Zwg
via IFTTT
Anonymous donor puts $10000 check in Salvation Army kettle
from Google Alert - anonymous http://ift.tt/2h89RjI
via IFTTT
An Airplane Glory

Moon Phase and Libration, 2017
from NASA's Scientific Visualization Studio: Most Recent Items http://ift.tt/2i7Csrj
via IFTTT
Moon Phase and Libration, 2017 South Up
from NASA's Scientific Visualization Studio: Most Recent Items http://ift.tt/2i117jZ
via IFTTT
Thursday, December 22, 2016
I have a new follower on Twitter
Jeannie Hill
http://t.co/XwnXKkxlh4
Following: 508 - Followers: 766
December 22, 2016 at 11:08PM via Twitter http://twitter.com/essentialskill
Causal Effect Identification in Acyclic Directed Mixed Graphs and Gated Models. (arXiv:1612.07512v1 [stat.ML])
We introduce a new family of graphical models that consists of graphs with possibly directed, undirected and bidirected edges but without directed cycles. We show that these models are suitable for representing causal models with additive error terms. We provide a set of sufficient graphical criteria for the identification of arbitrary causal effects when the new models contain directed and undirected edges but no bidirected edge. We also provide a necessary and sufficient graphical criterion for the identification of the causal effect of a single variable on the rest of the variables. Moreover, we develop an exact algorithm for learning the new models from observational and interventional data via answer set programming. Finally, we introduce gated models for causal effect identification, a new family of graphical models that exploits context specific independences to identify additional causal effects.
from cs.AI updates on arXiv.org http://ift.tt/2i0hVaM
via IFTTT
Non-Deterministic Policy Improvement Stabilizes Approximated Reinforcement Learning. (arXiv:1612.07548v1 [cs.AI])
This paper investigates a type of instability that is linked to the greedy policy improvement in approximated reinforcement learning. We show empirically that non-deterministic policy improvement can stabilize methods like LSPI by controlling the improvements' stochasticity. Additionally we show that a suitable representation of the value function also stabilizes the solution to some degree. The presented approach is simple and should also be easily transferable to more sophisticated algorithms like deep reinforcement learning.
from cs.AI updates on arXiv.org http://ift.tt/2i7biAP
via IFTTT
The SP Theory of Intelligence as a Foundation for the Development of a General, Human-Level Thinking Machine. (arXiv:1612.07555v1 [cs.AI])
This paper summarises how the "SP theory of intelligence" and its realisation in the "SP computer model" simplifies and integrates concepts across artificial intelligence and related areas, and thus provides a promising foundation for the development of a general, human-level thinking machine, in accordance with the main goal of research in artificial general intelligence.
The key to this simplification and integration is the powerful concept of "multiple alignment", borrowed and adapted from bioinformatics. This concept has the potential to be the "double helix" of intelligence, with as much significance for human-level intelligence as has DNA for biological sciences.
Strengths of the SP system include: versatility in the representation of diverse kinds of knowledge; versatility in aspects of intelligence (including: strengths in unsupervised learning; the processing of natural language; pattern recognition at multiple levels of abstraction that is robust in the face of errors in data; several kinds of reasoning (including: one-step `deductive' reasoning; chains of reasoning; abductive reasoning; reasoning with probabilistic networks and trees; reasoning with 'rules'; nonmonotonic reasoning and reasoning with default values; Bayesian reasoning with 'explaining away'; and more); planning; problem solving; and more); seamless integration of diverse kinds of knowledge and diverse aspects of intelligence in any combination; and potential for application in several areas (including: helping to solve nine problems with big data; helping to develop human-level intelligence in autonomous robots; serving as a database with intelligence and with versatility in the representation and integration of several forms of knowledge; serving as a vehicle for medical knowledge and as an aid to medical diagnosis; and several more).
from cs.AI updates on arXiv.org http://ift.tt/2i0niHd
via IFTTT
Solving Set Optimization Problems by Cardinality Optimization via Weak Constraints with an Application to Argumentation. (arXiv:1612.07589v1 [cs.AI])
Optimization - minimization or maximization - in the lattice of subsets is a frequent operation in Artificial Intelligence tasks. Examples are subset-minimal model-based diagnosis, nonmonotonic reasoning by means of circumscription, or preferred extensions in abstract argumentation. Finding the optimum among many admissible solutions is often harder than finding admissible solutions with respect to both computational complexity and methodology. This paper addresses the former issue by means of an effective method for finding subset-optimal solutions. It is based on the relationship between cardinality-optimal and subset-optimal solutions, and the fact that many logic-based declarative programming systems provide constructs for finding cardinality-optimal solutions, for example maximum satisfiability (MaxSAT) or weak constraints in Answer Set Programming (ASP). Clearly each cardinality-optimal solution is also a subset-optimal one, and if the language also allows for the addition of particular restricting constructs (both MaxSAT and ASP do) then all subset-optimal solutions can be found by an iterative computation of cardinality-optimal solutions. As a showcase, the computation of preferred extensions of abstract argumentation frameworks using the proposed method is studied.
from cs.AI updates on arXiv.org http://ift.tt/2i77hfT
via IFTTT
Counting Answer Sets via Dynamic Programming. (arXiv:1612.07601v1 [cs.LO])
While the solution counting problem for propositional satisfiability (#SAT) has received renewed attention in recent years, this research trend has not affected other AI solving paradigms like answer set programming (ASP). Although ASP solvers are designed to enumerate all solutions, and counting can therefore be easily done, the involved materialization of all solutions is a clear bottleneck for the counting problem of ASP (#ASP). In this paper we propose dynamic programming-based #ASP algorithms that exploit the structure of the underlying (ground) ASP program. Experimental results for a prototype implementation show promise when compared to existing solvers.
from cs.AI updates on arXiv.org http://ift.tt/2i0t6jG
via IFTTT
Jointly Extracting Relations with Class Ties via Effective Deep Ranking. (arXiv:1612.07602v1 [cs.AI])
In distant supervised relation extraction, the connection between relations of one entity tuple, which we call class ties, is common. Exploiting this connection may be promising for relation extraction. However, this property is seldom considered by previous work. In this work, to leverage class ties, we propose to make joint relation extraction with a unified model that integrates convolutional neural network with a general pairwise ranking framework, in which two novel ranking loss functions are introduced. Besides, an effective method is proposed to relieve the impact of relation NR (not relation) for model training and test. Experimental results on a widely used dataset show that: (1) Our model is much more superior than the baselines, achieving state-of-the-art performance; (2) Leveraging class ties, joint extraction is indeed better than separated extraction; (3) Relieving the impact of NR will significantly boost our model performance; (4) Our model can primely deal with wrong labeling problem.
from cs.AI updates on arXiv.org http://ift.tt/2i7dI2c
via IFTTT
Difficulty Adjustable and Scalable Constrained Multi-objective Test Problem Toolkit. (arXiv:1612.07603v1 [cs.NE])
In order to better understand the advantages and disadvantages of a constrained multi-objective evolutionary algorithm (CMOEA), it is important to understand the nature of difficulty of a constrained multi-objective optimization problem (CMOP) that the CMOEA is going to deal with. In this paper, we first propose three primary types of difficulty to characterize the constraints in CMOPs, including feasibility-hardness, convergence-hardness and diversity-hardness. We then develop a general toolkit to construct difficulty adjustable CMOPs with three types of parameterized constraint functions according to the proposed three primary types of difficulty. In fact, combination of the three primary constraint functions with different parameters can lead to construct a large variety of CMOPs and CMaOPs, whose difficulty can be uniquely defined by a triplet with each of its parameter specifying the level of each primary difficulty type respectively. Based on this toolkit, we suggest fifteen difficulty adjustable CMOPs named DAC-MOP1-15 with different types and levels of difficulty. To study the effectiveness of DAC-MOP1-15, two popular CMOEAs - MOEA/D-CDP and NSGA-II-CDP are adopted to test their performances on them. Furthermore, this toolkit also has the ability to scale the number of objectives. Nine difficulty adjustable constrained many-objective optimization problems (DAC-MaOPs) named DAC-MaOP1-9 with the scalability to the number of objectives are also proposed using this toolkit. Two constrained many-objective evolutionary algorithms (CMaOEAs) - CNSGA-III and CMOEA/DD are applied to test their performances on three, five, seven and ten-objective DAC-MaOP1-9 with different difficulty levels and types.
from cs.AI updates on arXiv.org http://ift.tt/2i0sWsP
via IFTTT
Highway and Residual Networks learn Unrolled Iterative Estimation. (arXiv:1612.07771v1 [cs.NE])
The past year saw the introduction of new architectures such as Highway networks and Residual networks which, for the first time, enabled the training of feedforward networks with dozens to hundreds of layers using simple gradient descent. While depth of representation has been posited as a primary reason for their success, there are indications that these architectures defy a popular view of deep learning as a hierarchical computation of increasingly abstract features at each layer.
In this report, we argue that this view is incomplete and does not adequately explain several recent findings. We propose an alternative viewpoint based on unrolled iterative estimation---a group of successive layers iteratively refine their estimates of the same features instead of computing an entirely new representation. We demonstrate that this viewpoint directly leads to the construction of Highway and Residual networks. Finally we provide preliminary experiments to discuss the similarities and differences between the two architectures.
from cs.AI updates on arXiv.org http://ift.tt/2i7d4Sz
via IFTTT
Facial Expression Recognition Using a Hybrid CNN-SIFT Aggregator. (arXiv:1608.02833v4 [cs.CV] UPDATED)
Recognizing facial expression has remained a challenging task in computer vision. Deriving an effective facial expression recognition is an important step for successful human-computer interaction systems. This paper describes a novel approach towards facial expression recognition task. It is motivated by the success of Convolutional Neural Networks (CNN) on face recognition problems. Unlike other works, we focus on getting good accuracy results while requiring only a small sample data to train the model by merging the CNN and SIFT features. The proposed classification model is an aggregation of multiple deep convolutional neural networks and a hybrid CNN-SIFT classifiers. The goal of using SIFT features is to increase the performance on small data as SIFT does not require large training data to generate useful features. The model has been tested on FER-2013, CK+ and SFEW 2.0 datasets. The results showed how CNN-SIFT feature improve the accuracy when added as a voting member in an ensemble classifier. It generates state-of-art results on FER-2013 and CK+ datasets, where it achieved 73.58% on FER-2013 and 99.35% on CK+.
from cs.AI updates on arXiv.org http://ift.tt/2aTFFqv
via IFTTT
Commonsense Knowledge Enhanced Embeddings for Solving Pronoun Disambiguation Problems in Winograd Schema Challenge. (arXiv:1611.04146v2 [cs.AI] UPDATED)
In this paper, we propose commonsense knowledge enhanced embeddings (KEE) for solving the Pronoun Disambiguation Problems (PDP). The PDP task we investigate in this paper is a complex coreference resolution task which requires the utilization of commonsense knowledge. This task is a standard first round test set in the 2016 Winograd Schema Challenge. In this task, traditional linguistic features that are useful for coreference resolution, e.g. context and gender information, are no longer effective anymore. Therefore, the KEE models are proposed to provide a general framework to make use of commonsense knowledge for solving the PDP problems. Since the PDP task doesn't have training data, the KEE models would be used during the unsupervised feature extraction process. To evaluate the effectiveness of the KEE models, we propose to incorporate various commonsense knowledge bases, including ConceptNet, WordNet, and CauseCom, into the KEE training process. We achieved the best performance by applying the proposed methods to the 2016 Winograd Schema Challenge. In addition, experiments conducted on the standard PDP task indicate that, the proposed KEE models could solve the PDP problems by achieving 66.7% accuracy, which is a new state-of-the-art performance.
from cs.AI updates on arXiv.org http://ift.tt/2fSLYAl
via IFTTT
NewsQA: A Machine Comprehension Dataset. (arXiv:1611.09830v2 [cs.CL] UPDATED)
We present NewsQA, a challenging machine comprehension dataset of over 100,000 question-answer pairs. Crowdworkers supply questions and answers based on a set of over 10,000 news articles from CNN, with answers consisting in spans of text from the corresponding articles. We collect this dataset through a four-stage process designed to solicit exploratory questions that require reasoning. A thorough analysis confirms that NewsQA demands abilities beyond simple word matching and recognizing entailment. We measure human performance on the dataset and compare it to several strong neural models. The performance gap between humans and machines (25.3% F1) indicates that significant progress can be made on NewsQA through future research. The dataset is freely available at http://ift.tt/2gDWBrf.
from cs.AI updates on arXiv.org http://ift.tt/2g3UxFf
via IFTTT
Famously Anonymous: Tor Social Networks
from Google Alert - anonymous http://ift.tt/2h7CJsl
via IFTTT
ARES: Adaptive Receding-Horizon Synthesis of Optimal Plans. (arXiv:1612.07059v1 [cs.AI])
We introduce ARES, an efficient approximation algorithm for generating optimal plans (action sequences) that take an initial state of a Markov Decision Process (MDP) to a state whose cost is below a specified (convergence) threshold. ARES uses Particle Swarm Optimization, with adaptive sizing for both the receding horizon and the particle swarm. Inspired by Importance Splitting, the length of the horizon and the number of particles are chosen such that at least one particle reaches a next-level state, that is, a state where the cost decreases by a required delta from the previous-level state. The level relation on states and the plans constructed by ARES implicitly define a Lyapunov function and an optimal policy, respectively, both of which could be explicitly generated by applying ARES to all states of the MDP, up to some topological equivalence relation. We also assess the effectiveness of ARES by statistically evaluating its rate of success in generating optimal plans. The ARES algorithm resulted from our desire to clarify if flying in V-formation is a flocking policy that optimizes energy conservation, clear view, and velocity alignment. That is, we were interested to see if one could find optimal plans that bring a flock from an arbitrary initial state to a state exhibiting a single connected V-formation. For flocks with 7 birds, ARES is able to generate a plan that leads to a V-formation in 95% of the 8,000 random initial configurations within 63 seconds, on average. ARES can also be easily customized into a model-predictive controller (MPC) with an adaptive receding horizon and statistical guarantees of convergence. To the best of our knowledge, our adaptive-sizing approach is the first to provide convergence guarantees in receding-horizon techniques.
from cs.AI updates on arXiv.org http://ift.tt/2i2HOnz
via IFTTT
Anonymous proxy ip address
from Google Alert - anonymous http://ift.tt/2hhxkEm
via IFTTT
Climate scientists swing back, launch anonymous hotline for gov't employees to report Trump ...
from Google Alert - anonymous http://ift.tt/2hXLKpX
via IFTTT
Anonymous donor pays off lunch balances at Maple Grove High
from Google Alert - anonymous http://ift.tt/2igy8tc
via IFTTT
Anonymous function versus File in matlabfunction
from Google Alert - anonymous http://ift.tt/2hXoj00
via IFTTT
Anonymous Hacks Thailand Navy Site to Protest Censorship
from Google Alert - anonymous http://ift.tt/2i5V7Un
via IFTTT
Russia Wants Apple to Unlock iPhone belonging to Killer of Russian Ambassador
from The Hacker News http://ift.tt/2hvszmz
via IFTTT
Raspberry Pi launches PIXEL OS for Mac and Windows PCs
from The Hacker News http://ift.tt/2hgDpAN
via IFTTT
ISS Daily Summary Report – 12/21/2016
from ISS On-Orbit Status Report http://ift.tt/2hWKIuF
via IFTTT
Wired, “Anonymous' Barrett Brown Is Free—and Ready to Pick New Fights”
from Google Alert - anonymous http://ift.tt/2hKJ6Wy
via IFTTT
NIST Calls Development of Quantum-Proof Encryption Algorithms
from The Hacker News http://ift.tt/2h4JuLC
via IFTTT
'Anonymous Billionaire' in the Spotlight After 1000% Rally
from Google Alert - anonymous http://ift.tt/2hK8uvy
via IFTTT
Wednesday, December 21, 2016
App combats online bullying by sending anonymous compliments
from Google Alert - anonymous http://ift.tt/2i36s7D
via IFTTT
I have a new follower on Twitter
Kelly Williams
https://t.co/4JW9jxB4Ng
Cornelius, NC, United States
https://t.co/RARdZ64e8K
Following: 1343 - Followers: 1033
December 21, 2016 at 09:20PM via Twitter http://twitter.com/DaintyDots
AIVAT: A New Variance Reduction Technique for Agent Evaluation in Imperfect Information Games. (arXiv:1612.06915v1 [cs.AI])
Evaluating agent performance when outcomes are stochastic and agents use randomized strategies can be challenging when there is limited data available. The variance of sampled outcomes may make the simple approach of Monte Carlo sampling inadequate. This is the case for agents playing heads-up no-limit Texas hold'em poker, where man-machine competitions have involved multiple days of consistent play and still not resulted in statistically significant conclusions even when the winner's margin is substantial. In this paper, we introduce AIVAT, a low variance, provably unbiased value assessment tool that uses an arbitrary heuristic estimate of state value, as well as the explicit strategy of a subset of the agents. Unlike existing techniques which reduce the variance from chance events, or only consider game ending actions, AIVAT reduces the variance both from choices by nature and by players with a known strategy. The resulting estimator in no-limit poker can reduce the number of hands needed to draw statistical conclusions by more than a factor of 10.
from cs.AI updates on arXiv.org http://ift.tt/2hVduvH
via IFTTT
Stochastic Runtime Analysis of a Cross Entropy Algorithm for Traveling Salesman Problems. (arXiv:1612.06962v1 [cs.DS])
This article analyzes the stochastic runtime of a Cross-Entropy Algorithm on two classes of traveling salesman problems. The algorithm shares main features of the famous Max-Min Ant System with iteration-best reinforcement.
For simple instances that have a $\{1,n\}$-valued distance function and a unique optimal solution, we prove a stochastic runtime of $O(n^{6+\epsilon})$ with the vertex-based random solution generation, and a stochastic runtime of $O(n^{3+\epsilon}\ln n)$ with the edge-based random solution generation for an arbitrary $\epsilon\in (0,1)$. These runtimes are very close to the known expected runtime for variants of Max-Min Ant System with best-so-far reinforcement. They are obtained for the stronger notion of stochastic runtime, which means that an optimal solution is obtained in that time with an overwhelming probability, i.e., a probability tending exponentially fast to one with growing problem size.
We also inspect more complex instances with $n$ vertices positioned on an $m\times m$ grid. When the $n$ vertices span a convex polygon, we obtain a stochastic runtime of $O(n^{3}m^{5+\epsilon})$ with the vertex-based random solution generation, and a stochastic runtime of $O(n^{2}m^{5+\epsilon})$ for the edge-based random solution generation. When there are $k = O(1)$ many vertices inside a convex polygon spanned by the other $n-k$ vertices, we obtain a stochastic runtime of $O(n^{4}m^{5+\epsilon}+n^{6k-1}m^{\epsilon})$ with the vertex-based random solution generation, and a stochastic runtime of $O(n^{3}m^{5+\epsilon}+n^{3k}m^{\epsilon})$ with the edge-based random solution generation. These runtimes are better than the expected runtime for the so-called $(\mu\!+\!\lambda)$ EA reported in a recent article, and again obtained for the stronger notion of stochastic runtime.
from cs.AI updates on arXiv.org http://ift.tt/2hJ5eAE
via IFTTT
Disjunctive Boolean Kernels for Collaborative Filtering in Top-N Recommendation. (arXiv:1612.07025v1 [cs.IR])
In many personalized recommendation problems available data consists only of positive interactions (implicit feedback) between users and items. This problem is also known as One-Class Collaborative Filtering (OC-CF). Linear models usually achieves state-of-the-art performances on OC-CF problems and many efforts have been devoted to build more expressive and complex representations able to improve the recommendations but with no much success. Recent analysis shows that collaborative filtering (CF) datasets have peculiar characteristics such as high sparsity and a long tailed distribution of the ratings. In this paper we propose a boolean kernel, called Disjunctive Kernel, which is less expressive than the linear one but it is able to alleviate the sparsity issue in CF contexts. The embedding of this kernel is composed by all the combinations of a certain degree $d$ of the input variables, and these combined features are semantically interpreted as disjunctions of the input variables. Experiments on several CF datasets show the effectiveness and the efficiency of the proposed kernel.
from cs.AI updates on arXiv.org http://ift.tt/2hVbiEk
via IFTTT
Deep-learning in Mobile Robotics - from Perception to Control Systems: A Survey on Why and Why not. (arXiv:1612.07139v1 [cs.RO])
Deep-learning has dramatically changed the world overnight. It greatly boosted the development of visual perception, object detection, and speech recognition, etc. That was attributed to the multiple convolutional processing layers for abstraction of learning representations from massive data. The advantages of deep convolutional structures in data processing motivated the applications of artificial intelligence methods in robotic problems, especially perception and control system, the two typical and challenging problems in robotics. This paper presents a survey of the deep-learning research landscape in mobile robotics. We start with introducing the definition and development of deep-learning in related fields, especially the essential distinctions between image processing and robotic tasks. We described and discussed several typical applications and related works in this domain, followed by the benefits from deep-learning, and related existing frameworks. Besides, operation in the complex dynamic environment is regarded as a critical bottleneck for mobile robots, such as that for autonomous driving. We thus further emphasize the recent achievement on how deep-learning contributes to navigation and control systems for mobile robots. At the end, we discuss the open challenges and research frontiers.
from cs.AI updates on arXiv.org http://ift.tt/2hJeWmI
via IFTTT
Understanding Error Correction and its Role as Part of the Communication Channel in Environments composed of Self-Integrating Systems. (arXiv:1612.07294v1 [cs.AI])
The raise of complexity of technical systems also raises knowledge required to set them up and to maintain them. The cost to evolve such systems can be prohibitive. In the field of Autonomic Computing, technical systems should therefore have various self-healing capabilities allowing system owners to provide only partial, potentially inconsistent updates of the system. The self-healing or self-integrating system shall find out the remaining changes to communications and functionalities in order to accommodate change and yet still restore function. This issue becomes even more interesting in context of Internet of Things and Industrial Internet where previously unexpected device combinations can be assembled in order to provide a surprising new function. In order to pursue higher levels of self-integration capabilities I propose to think of self-integration as sophisticated error correcting communications. Therefore, this paper discusses an extended scope of error correction with the purpose to emphasize error correction's role as an integrated element of bi-directional communication channels in self-integrating, autonomic communication scenarios.
from cs.AI updates on arXiv.org http://ift.tt/2hVbLGN
via IFTTT
Yum-me: A Personalized Nutrient-based Meal Recommender System. (arXiv:1605.07722v2 [cs.HC] UPDATED)
Nutrient-based meal recommendations have the potential to help individuals prevent or manage conditions such as diabetes and obesity. However, learning people's food preferences and making recommendations that simultaneously appeal to their palate and satisfy nutritional expectations are challenging. Existing approaches either only learn high-level preferences or require a prolonged learning period. We propose Yum-me, a personalized nutrient-based meal recommender system designed to meet individuals' nutritional expectations, dietary restrictions, and fine-grained food preferences. Yum-me enables a simple and accurate food preference profiling procedure via a visual quiz-based user interface, and projects the learned profile into the domain of nutritionally appropriate food options to find ones that will appeal to the user. We present the design and implementation of Yum-me, and further describe and evaluate two innovative components: 1) An open source state-of-the-art food image analysis model, named FoodDist. We demonstrate FoodDist's superior performance through careful benchmarking, and discuss its applicability across a wide array of dietary applications. 2) A novel online learning framework that learns food preference from itemwise and pairwise image comparisons. We evaluate the framework in a field study of 227 anonymous users and demonstrate that it outperforms other baselines by a significant margin. Finally, we conduct an end-to-end validation of the feasibility and effectiveness of Yum-me through a 60-person user study, in which Yum-me improves the recommendation acceptance rate by 42.63%.
from cs.AI updates on arXiv.org http://ift.tt/1TFeBMP
via IFTTT
Revisiting Causality Inference in Memory-less Transition Networks. (arXiv:1608.02658v3 [stat.ML] UPDATED)
Several methods exist to infer causal networks from massive volumes of observational data. However, almost all existing methods require a considerable length of time series data to capture cause and effect relationships. In contrast, memory-less transition networks or Markov Chain data, which refers to one-step transitions to and from an event, have not been explored for causality inference even though such data is widely available. We find that causal network can be inferred from characteristics of four unique distribution zones around each event. We call this Composition of Transitions and show that cause, effect, and random events exhibit different behavior in their compositions. We applied machine learning models to learn these different behaviors and to infer causality. We name this new method Causality Inference using Composition of Transitions (CICT). To evaluate CICT, we used an administrative inpatient healthcare dataset to set up a network of patients transitions between different diagnoses. We show that CICT is highly accurate in inferring whether the transition between a pair of events is causal or random and performs well in identifying the direction of causality in a bi-directional association.
from cs.AI updates on arXiv.org http://ift.tt/2aQpZXE
via IFTTT
Anonymous Donor Gives Mass. Church $100k Winning Lottery Ticket
from Google Alert - anonymous http://ift.tt/2iheXLC
via IFTTT
Drafts for anonymous users
from Google Alert - anonymous http://ift.tt/2hbCXSQ
via IFTTT
Eric Weddle and Dennis Pitta among Ravens' snubs for 2017 Pro Bowl - Jamison Hensley (ESPN)
via IFTTT
Sharpless 308: Star Bubble
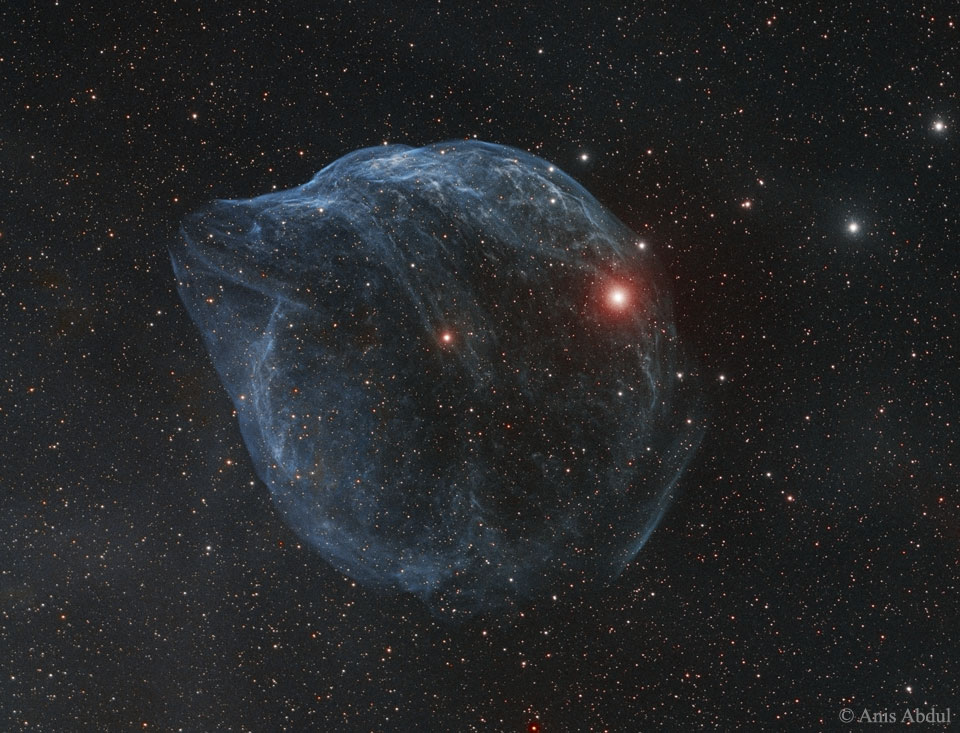
Ravens Image: WR Steve Smith Jr. wears elf costume to his Wednesday media session; wore a Yoda outfit a few weeks ago (ESPN)
via IFTTT
Ravens: CB Jimmy Smith (sprained ankle) not practicing Wednesday; missed 1 game after sustaining injury in Week 14 (ESPN)
via IFTTT
Search results not coming for anonymous users
from Google Alert - anonymous http://ift.tt/2hceyy7
via IFTTT
ISS Daily Summary Report – 12/20/2016
from ISS On-Orbit Status Report http://ift.tt/2i0vbcE
via IFTTT
Advice- Anonymous and Free
from Google Alert - anonymous http://ift.tt/2hce4Yz
via IFTTT
Google 'Android Things' — An Operating System for the Internet of Things
from The Hacker News http://ift.tt/2h0QrNR
via IFTTT
Hackers Suspected of Causing Second Power Outage in Ukraine
from The Hacker News http://ift.tt/2h8Z2Bx
via IFTTT
Sharpless 308: Star Bubble
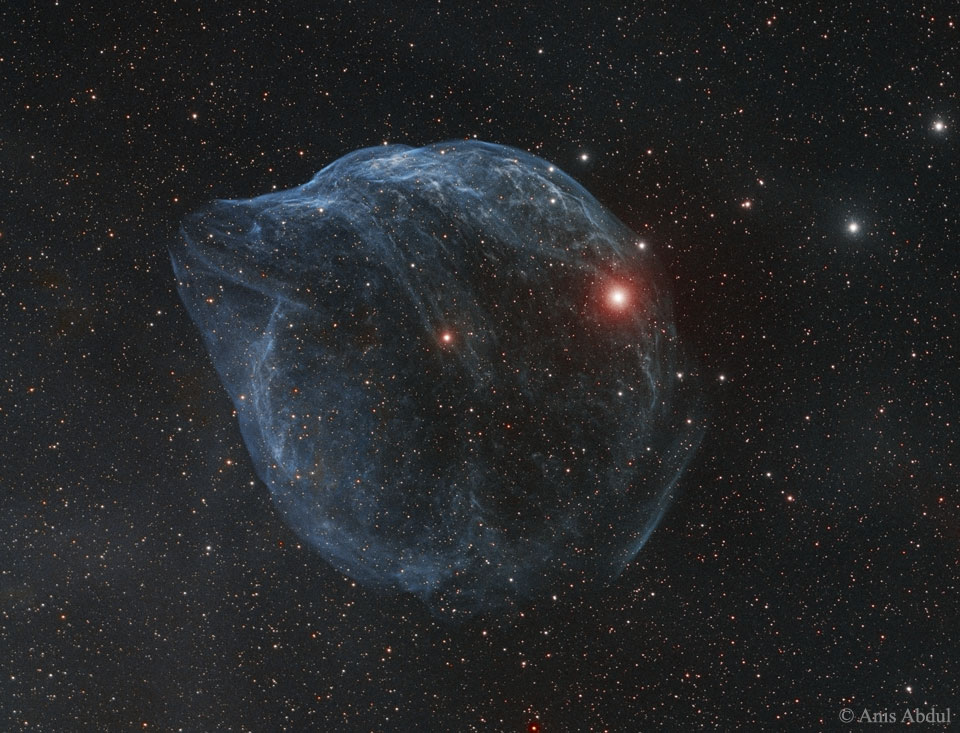
Tuesday, December 20, 2016
I have a new follower on Twitter
Vasayo byZodiac
Ground Floor Opportunity NEW Disruptive Nanotechnology Micro Nutritionals Delivery System.
Cheyenne, WY
https://t.co/z0hvTDe0Xk
Following: 626 - Followers: 518
December 20, 2016 at 11:55PM via Twitter http://twitter.com/VasayoZodiac
Anonymous Donor Gives Church $100k Winning Lottery Ticket
from Google Alert - anonymous http://ift.tt/2hYllbo
via IFTTT
I have a new follower on Twitter
Tutan Ramon
Film izlemek, müzik dinlemek, bir de seninle sohbet etmek
Following: 16 - Followers: 22
December 20, 2016 at 09:20PM via Twitter http://twitter.com/TutanR
I have a new follower on Twitter
Gerçekliğin Efendisi
Master of Reality
Following: 22 - Followers: 10
December 20, 2016 at 09:20PM via Twitter http://twitter.com/gercekefendi
Ravens: G Marshal Yanda (7th selection), K Justin Tucker (3rd selection) among four players named to 2017 Pro Bowl (ESPN)
via IFTTT
Computational Complexity of Testing Proportional Justified Representation. (arXiv:1612.06476v1 [cs.GT])
We consider a committee voting setting in which each voter approves of a subset of candidates and based on the approvals, a target number of candidates are selected. Aziz et al. (2015) proposed two representation axioms called justified representation and extended justified representation. Whereas the former can be tested as well as achieved in polynomial time, the latter property is coNP-complete to test and no polynomial-time algorithm is known to achieve it. Interestingly, S{\'a}nchez-Fern{\'a}ndez et~al. (2016) proposed an intermediate property called proportional justified representation that admits a polynomial-time algorithm to achieve. The complexity of testing proportional justified representation has remained an open problem. In this paper, we settle the complexity by proving that testing proportional justified representation is coNP-complete. We complement the complexity result by showing that the problem admits efficient algorithms if any of the following parameters are bounded: (1) number of voters (2) number of candidates (3) maximum number of candidates approved by a voter (4) maximum number of voters approving a given candidate.
from cs.AI updates on arXiv.org http://ift.tt/2gZWpym
via IFTTT
Parallelized Tensor Train Learning For Polynomial Pattern Classification. (arXiv:1612.06505v1 [cs.LG])
In pattern classification, polynomial classifiers are well-studied methods as they are capable of generating complex decision surfaces. Unfortunately, the use of multivariate polynomials is limited to support vector machine kernels, as polynomials quickly become impractical for high-dimensional problems. In this paper, we effectively overcome the curse of dimensionality by employing the tensor train format to represent a polynomial classifier. Based on the structure of tensor trains, two learning algorithms are proposed which involve solving different optimization problems of low computational complexity. Furthermore, we show how both regularization to prevent overfitting and parallelization, which enables the use of large training sets, are incorporated into these methods. Both the efficiency and efficacy of our tensor-based polynomial classifier are then demonstrated on the two popular datasets USPS and MNIST.
from cs.AI updates on arXiv.org http://ift.tt/2h00tPe
via IFTTT
Neuro-symbolic EDA-based Optimisation using ILP-enhanced DBNs. (arXiv:1612.06528v1 [cs.AI])
We investigate solving discrete optimisation problems using the estimation of distribution (EDA) approach via a novel combination of deep belief networks(DBN) and inductive logic programming (ILP).While DBNs are used to learn the structure of successively better feasible solutions,ILP enables the incorporation of domain-based background knowledge related to the goodness of solutions.Recent work showed that ILP could be an effective way to use domain knowledge in an EDA scenario.However,in a purely ILP-based EDA,sampling successive populations is either inefficient or not straightforward.In our Neuro-symbolic EDA,an ILP engine is used to construct a model for good solutions using domain-based background knowledge.These rules are introduced as Boolean features in the last hidden layer of DBNs used for EDA-based optimization.This incorporation of logical ILP features requires some changes while training and sampling from DBNs: (a)our DBNs need to be trained with data for units at the input layer as well as some units in an otherwise hidden layer, and (b)we would like the samples generated to be drawn from instances entailed by the logical model.We demonstrate the viability of our approach on instances of two optimisation problems: predicting optimal depth-of-win for the KRK endgame,and jobshop scheduling.Our results are promising: (i)On each iteration of distribution estimation,samples obtained with an ILP-assisted DBN have a substantially greater proportion of good solutions than samples generated using a DBN without ILP features, and (ii)On termination of distribution estimation,samples obtained using an ILP-assisted DBN contain more near-optimal samples than samples from a DBN without ILP features.These results suggest that the use of ILP-constructed theories could be useful for incorporating complex domain-knowledge into deep models for estimation of distribution based procedures.
from cs.AI updates on arXiv.org http://ift.tt/2gZRuxx
via IFTTT
A Latent-class Model for Estimating Product-choice Probabilities from Clickstream Data. (arXiv:1612.06589v1 [cs.AI])
This paper analyzes customer product-choice behavior based on the recency and frequency of each customer's page views on e-commerce sites. Recently, we devised an optimization model for estimating product-choice probabilities that satisfy monotonicity, convexity, and concavity constraints with respect to recency and frequency. This shape-restricted model delivered high predictive performance even when there were few training samples. However, typical e-commerce sites deal in many different varieties of products, so the predictive performance of the model can be further improved by integration of such product heterogeneity. For this purpose, we develop a novel latent-class shape-restricted model for estimating product-choice probabilities for each latent class of products. We also give a tailored expectation-maximization algorithm for parameter estimation. Computational results demonstrate that higher predictive performance is achieved with our latent-class model than with the previous shape-restricted model and common latent-class logistic regression.
from cs.AI updates on arXiv.org http://ift.tt/2gZYV7H
via IFTTT
Action-Driven Object Detection with Top-Down Visual Attentions. (arXiv:1612.06704v1 [cs.CV])
A dominant paradigm for deep learning based object detection relies on a "bottom-up" approach using "passive" scoring of class agnostic proposals. These approaches are efficient but lack of holistic analysis of scene-level context. In this paper, we present an "action-driven" detection mechanism using our "top-down" visual attention model. We localize an object by taking sequential actions that the attention model provides. The attention model conditioned with an image region provides required actions to get closer toward a target object. An action at each time step is weak itself but an ensemble of the sequential actions makes a bounding-box accurately converge to a target object boundary. This attention model we call AttentionNet is composed of a convolutional neural network. During our whole detection procedure, we only utilize the actions from a single AttentionNet without any modules for object proposals nor post bounding-box regression. We evaluate our top-down detection mechanism over the PASCAL VOC series and ILSVRC CLS-LOC dataset, and achieve state-of-the-art performances compared to the major bottom-up detection methods. In particular, our detection mechanism shows a strong advantage in elaborate localization by outperforming Faster R-CNN with a margin of +7.1% over PASCAL VOC 2007 when we increase the IoU threshold for positive detection to 0.7.
from cs.AI updates on arXiv.org http://ift.tt/2hFzmx3
via IFTTT
Data Centroid Based Multi-Level Fuzzy Min-Max Neural Network. (arXiv:1608.05513v2 [cs.AI] UPDATED)
Recently, a multi-level fuzzy min max neural network (MLF) was proposed, which improves the classification accuracy by handling an overlapped region (area of confusion) with the help of a tree structure. In this brief, an extension of MLF is proposed which defines a new boundary region, where the previously proposed methods mark decisions with less confidence and hence misclassification is more frequent. A methodology to classify patterns more accurately is presented. Our work enhances the testing procedure by means of data centroids. We exhibit an illustrative example, clearly highlighting the advantage of our approach. Results on standard datasets are also presented to evidentially prove a consistent improvement in the classification rate.
from cs.AI updates on arXiv.org http://ift.tt/2b9qJIS
via IFTTT
Quantum Machine Learning without Measurements. (arXiv:1612.05535v1 [quant-ph] CROSS LISTED)
We propose a quantum machine learning algorithm for efficiently solving a class of problems encoded in quantum controlled unitary operations. The central physical mechanism of the protocol is the iteration of a quantum time-delayed equation that introduces feedback in the dynamics and eliminates the necessity of intermediate measurements. The performance of the quantum algorithm is analyzed by comparing the results obtained in numerical simulations with the outcome of classical machine learning methods for the same problem. The use of time-delayed equations enhances the toolbox of the field of quantum machine learning, which may enable unprecedented applications in quantum technologies.
from cs.AI updates on arXiv.org http://ift.tt/2hgF9WP
via IFTTT
[FD] NEW VMSA-2016-0023 VMware ESXi updates address a cross-site scripting issue
Source: Gmail -> IFTTT-> Blogger
[FD] CVE-2014-1785: MSIE 11 MSHTML CSpliceTreeEngine::RemoveSplice use-after-free
Source: Gmail -> IFTTT-> Blogger
'MethBot' Ad Fraud Operators Making $5 Million Revenue Every Day
from The Hacker News http://ift.tt/2icj6Az
via IFTTT
Ravens (8-6) up 2 spots to No. 10 in the Week 16 NFL Power Rankings; next game Sunday at the Steelers (ESPN)
via IFTTT
Hacker Demonstrates How Easy In-flight Entertainment System Can Be Hacked
from The Hacker News http://ift.tt/2hE9LUZ
via IFTTT
Entityform anonymous requires this module
from Google Alert - anonymous http://ift.tt/2h66yNe
via IFTTT
ISS Daily Summary Report – 12/19/2016
from ISS On-Orbit Status Report http://ift.tt/2hVrdlt
via IFTTT
RansomFree Tool Detects Never-Seen-Before Ransomware Before It Encrypts Your Data
from The Hacker News http://ift.tt/2hUtIVh
via IFTTT
[FD] Hotlinking Vulnerability in Glype (All Versions)
Source: Gmail -> IFTTT-> Blogger
Anonymous Donor Gives Thousands For Animal Rescue's New Shelter
from Google Alert - anonymous http://ift.tt/2hCtDYu
via IFTTT
Monday, December 19, 2016
Greenwald: Anonymous leaks not evidence in Russian hacking
from Google Alert - anonymous http://ift.tt/2gVOHW8
via IFTTT
A User Simulator for Task-Completion Dialogues. (arXiv:1612.05688v1 [cs.LG])
Despite widespread interests in reinforcement-learning for task-oriented dialogue systems, several obstacles can frustrate research and development progress. First, reinforcement learners typically require interaction with the environment, so conventional dialogue corpora cannot be used directly. Second, each task presents specific challenges, requiring separate corpus of task-specific annotated data. Third, collecting and annotating human-machine or human-human conversations for task-oriented dialogues requires extensive domain knowledge. Because building an appropriate dataset can be both financially costly and time-consuming, one popular approach is to build a user simulator based upon a corpus of example dialogues. Then, one can train reinforcement learning agents in an online fashion as they interact with the simulator. Dialogue agents trained on these simulators can serve as an effective starting point. Once agents master the simulator, they may be deployed in a real environment to interact with humans, and continue to be trained online. To ease empirical algorithmic comparisons in dialogues, this paper introduces a new, publicly available simulation framework, where our simulator, designed for the movie-booking domain, leverages both rules and collected data. The simulator supports two tasks: movie ticket booking and movie seeking. Finally, we demonstrate several agents and detail the procedure to add and test your own agent in the proposed framework.
from cs.AI updates on arXiv.org http://ift.tt/2hOrIkh
via IFTTT
Optimal Target Assignment and Path Finding for Teams of Agents. (arXiv:1612.05693v1 [cs.AI])
We study the TAPF (combined target-assignment and path-finding) problem for teams of agents in known terrain, which generalizes both the anonymous and non-anonymous multi-agent path-finding problems. Each of the teams is given the same number of targets as there are agents in the team. Each agent has to move to exactly one target given to its team such that all targets are visited. The TAPF problem is to first assign agents to targets and then plan collision-free paths for the agents to their targets in a way such that the makespan is minimized. We present the CBM (Conflict-Based Min-Cost-Flow) algorithm, a hierarchical algorithm that solves TAPF instances optimally by combining ideas from anonymous and non-anonymous multi-agent path-finding algorithms. On the low level, CBM uses a min-cost max-flow algorithm on a time-expanded network to assign all agents in a single team to targets and plan their paths. On the high level, CBM uses conflict-based search to resolve collisions among agents in different teams. Theoretically, we prove that CBM is correct, complete and optimal. Experimentally, we show the scalability of CBM to TAPF instances with dozens of teams and hundreds of agents and adapt it to a simulated warehouse system.
from cs.AI updates on arXiv.org http://ift.tt/2hOocX4
via IFTTT
Quantum Reinforcement Learning. (arXiv:1612.05695v1 [quant-ph])
We investigate whether quantum annealers with select chip layouts can outperform classical computers in reinforcement learning tasks. We associate a transverse field Ising spin Hamiltonian with a layout of qubits similar to that of a deep Boltzmann machine (DBM) and use simulated quantum annealing (SQA) to numerically simulate quantum sampling from this system. We design a reinforcement learning algorithm in which the set of visible nodes representing the states and actions of an optimal policy are the first and last layers of the deep network. In absence of a transverse field, our simulations show that DBMs train more effectively than restricted Boltzmann machines (RBM) with the same number of weights. Since sampling from Boltzmann distributions of a DBM is not classically feasible, this is evidence of supremacy of a non-Turing sampling oracle. We then develop a framework for training the network as a quantum Boltzmann machine (QBM) in the presence of a significant transverse field for reinforcement learning. The latter method further improves the reinforcement learning method using DBMs.
from cs.AI updates on arXiv.org http://ift.tt/2hTnbdG
via IFTTT
Exploiting sparsity to build efficient kernel based collaborative filtering for top-N item recommendation. (arXiv:1612.05729v1 [cs.IR])
The increasing availability of implicit feedback datasets has raised the interest in developing effective collaborative filtering techniques able to deal asymmetrically with unambiguous positive feedback and ambiguous negative feedback. In this paper, we propose a principled kernel-based collaborative filtering method for top-N item recommendation with implicit feedback. We present an efficient implementation using the linear kernel, and we show how to generalize it to kernels of the dot product family preserving the efficiency. We also investigate on the elements which influence the sparsity of a standard cosine kernel. This analysis shows that the sparsity of the kernel strongly depends on the properties of the dataset, in particular on the long tail distribution. We compare our method with state-of-the-art algorithms achieving good results both in terms of efficiency and effectiveness.
from cs.AI updates on arXiv.org http://ift.tt/2hNwUlS
via IFTTT
Web-based Semantic Similarity for Emotion Recognition in Web Objects. (arXiv:1612.05734v1 [cs.AI])
In this project we propose a new approach for emotion recognition using web-based similarity (e.g. confidence, PMI and PMING). We aim to extract basic emotions from short sentences with emotional content (e.g. news titles, tweets, captions), performing a web-based quantitative evaluation of semantic proximity between each word of the analyzed sentence and each emotion of a psychological model (e.g. Plutchik, Ekman, Lovheim). The phases of the extraction include: text preprocessing (tokenization, stop words, filtering), search engine automated query, HTML parsing of results (i.e. scraping), estimation of semantic proximity, ranking of emotions according to proximity measures. The main idea is that, since it is possible to generalize semantic similarity under the assumption that similar concepts co-occur in documents indexed in search engines, therefore also emotions can be generalized in the same way, through tags or terms that express them in a particular language, ranking emotions. Training results are compared to human evaluation, then additional comparative tests on results are performed, both for the global ranking correlation (e.g. Kendall, Spearman, Pearson) both for the evaluation of the emotion linked to each single word. Different from sentiment analysis, our approach works at a deeper level of abstraction, aiming at recognizing specific emotions and not only the positive/negative sentiment, in order to predict emotions as semantic data.
from cs.AI updates on arXiv.org http://ift.tt/2i3gNne
via IFTTT
A Comment on Argumentation. (arXiv:1612.05756v1 [math.LO])
We use the theory of defaults and their meaning of [GS16] to develop (the outline of a) new theory of argumentation.
from cs.AI updates on arXiv.org http://ift.tt/2hOtphH
via IFTTT