Latest YouTube Video
Saturday, July 2, 2016
Anonymous Egg Donors Needed. Donors set their own compensations!
from Google Alert - anonymous http://ift.tt/29etvJu
via IFTTT
Why Anonymous Keeps Hacking
from Google Alert - anonymous http://ift.tt/29kKwoq
via IFTTT
This Android Hacking Group is making $500,000 per day
from The Hacker News http://ift.tt/29BuqD0
via IFTTT
New Meditation Meeting
from Google Alert - anonymous http://ift.tt/29ljPAg
via IFTTT
Juno Approaching Jupiter
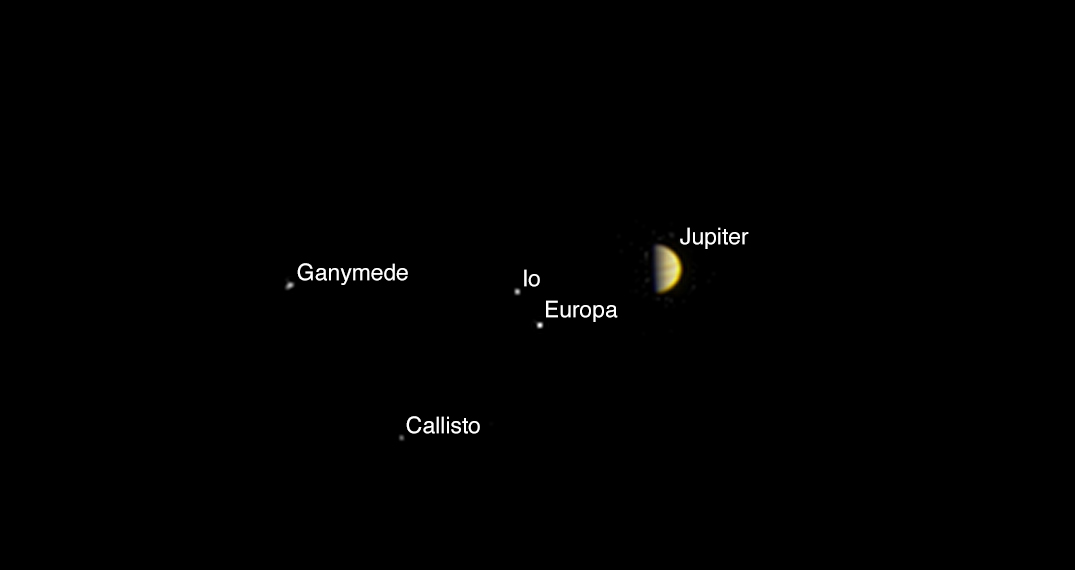
Friday, July 1, 2016
ISS Daily Summary Report – 07/01/16
from ISS On-Orbit Status Report http://ift.tt/29kTm5H
via IFTTT
Allow anonymous users to authenticate
from Google Alert - anonymous http://ift.tt/299HU8C
via IFTTT
Ravens: TE Darren Waller suspended 4 games for violating the NFL's substance abuse policy; 2 catches in 6 games in 2015 (ESPN)
via IFTTT
Access Denied for anonymous users
from Google Alert - anonymous http://ift.tt/296s8Kz
via IFTTT
Some anonymous players always use computers
from Google Alert - anonymous http://ift.tt/29cQwfq
via IFTTT
ISS Daily Summary Report – 06/30/16
from ISS On-Orbit Status Report http://ift.tt/29bhic6
via IFTTT
How to Crack Android Full Disk Encryption on Qualcomm Devices
from The Hacker News http://ift.tt/29ahhSW
via IFTTT
Apple Patents Technology to remotely disable your iPhone Camera at Concerts
from The Hacker News http://ift.tt/29gy6wn
via IFTTT
anonymous-sums
from Google Alert - anonymous http://ift.tt/295ejfk
via IFTTT
Google finally announces Android N's name and It's not Nutella
from The Hacker News http://ift.tt/29ag150
via IFTTT
MLB: Hyun Soo Kim homers in the 7th inning for Orioles' 56th home run of month, passing 1996 Athletics for most in June (ESPN)
via IFTTT
The New World Atlas of Artificial Sky Brightness
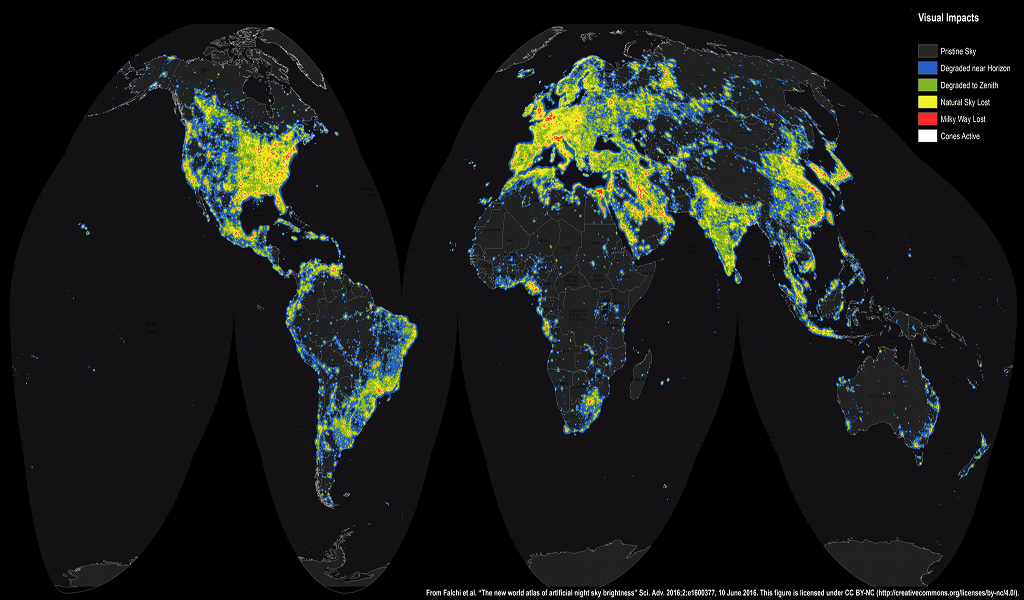
Thursday, June 30, 2016
Orioles: P T.J. McFarland (left knee) placed on the 15-day disabled list; P Vance Worley (groin) activated from the DL (ESPN)
via IFTTT
Anonymous should be able to edit their user profile
from Google Alert - anonymous http://ift.tt/296j0WT
via IFTTT
Swift: Compiled Inference for Probabilistic Programming Languages. (arXiv:1606.09242v1 [cs.AI])
A probabilistic program defines a probability measure over its semantic structures. One common goal of probabilistic programming languages (PPLs) is to compute posterior probabilities for arbitrary models and queries, given observed evidence, using a generic inference engine. Most PPL inference engines---even the compiled ones---incur significant runtime interpretation overhead, especially for contingent and open-universe models. This paper describes Swift, a compiler for the BLOG PPL. Swift-generated code incorporates optimizations that eliminate interpretation overhead, maintain dynamic dependencies efficiently, and handle memory management for possible worlds of varying sizes. Experiments comparing Swift with other PPL engines on a variety of inference problems demonstrate speedups ranging from 12x to 326x.
DONATE to arXiv: One hundred percent of your contribution will fund improvements and new initiatives to benefit arXiv's global scientific community. Please join the Simons Foundation and our generous member organizations and research labs in supporting arXiv. https://goo.gl/QIgRpr
from cs.AI updates on arXiv.org http://ift.tt/29a7xa5
via IFTTT
Compression of Neural Machine Translation Models via Pruning. (arXiv:1606.09274v1 [cs.AI])
Neural Machine Translation (NMT), like many other deep learning domains, typically suffers from over-parameterization, resulting in large storage sizes. This paper examines three simple magnitude-based pruning schemes to compress NMT models, namely class-blind, class-uniform, and class-distribution, which differ in terms of how pruning thresholds are computed for the different classes of weights in the NMT architecture. We demonstrate the efficacy of weight pruning as a compression technique for a state-of-the-art NMT system. We show that an NMT model with over 200 million parameters can be pruned by 40% with very little performance loss as measured on the WMT'14 English-German translation task. This sheds light on the distribution of redundancy in the NMT architecture. Our main result is that with retraining, we can recover and even surpass the original performance with an 80%-pruned model.
DONATE to arXiv: One hundred percent of your contribution will fund improvements and new initiatives to benefit arXiv's global scientific community. Please join the Simons Foundation and our generous member organizations and research labs in supporting arXiv. https://goo.gl/QIgRpr
from cs.AI updates on arXiv.org http://ift.tt/29hkhzv
via IFTTT
How Many Folders Do You Really Need?. (arXiv:1606.09296v1 [cs.AI])
Email classification is still a mostly manual task. Consequently, most Web mail users never define a single folder. Recently however, automatic classification offering the same categories to all users has started to appear in some Web mail clients, such as AOL or Gmail. We adopt this approach, rather than previous (unsuccessful) personalized approaches because of the change in the nature of consumer email traffic, which is now dominated by (non-spam) machine-generated email. We propose here a novel approach for (1) automatically distinguishing between personal and machine-generated email and (2) classifying messages into latent categories, without requiring users to have defined any folder. We report how we have discovered that a set of 6 "latent" categories (one for human- and the others for machine-generated messages) can explain a significant portion of email traffic. We describe in details the steps involved in building a Web-scale email categorization system, from the collection of ground-truth labels, the selection of features to the training of models. Experimental evaluation was performed on more than 500 billion messages received during a period of six months by users of Yahoo mail service, who elected to be part of such research studies. Our system achieved precision and recall rates close to 90% and the latent categories we discovered were shown to cover 70% of both email traffic and email search queries. We believe that these results pave the way for a change of approach in the Web mail industry, and could support the invention of new large-scale email discovery paradigms that had not been possible before.
DONATE to arXiv: One hundred percent of your contribution will fund improvements and new initiatives to benefit arXiv's global scientific community. Please join the Simons Foundation and our generous member organizations and research labs in supporting arXiv. https://goo.gl/QIgRpr
from cs.AI updates on arXiv.org http://ift.tt/29a7ZoC
via IFTTT
Learning Crosslingual Word Embeddings without Bilingual Corpora. (arXiv:1606.09403v1 [cs.CL])
Crosslingual word embeddings represent lexical items from different languages in the same vector space, enabling transfer of NLP tools. However, previous attempts had expensive resource requirements, difficulty incorporating monolingual data or were unable to handle polysemy. We address these drawbacks in our method which takes advantage of a high coverage dictionary in an EM style training algorithm over monolingual corpora in two languages. Our model achieves state-of-the-art performance on bilingual lexicon induction task exceeding models using large bilingual corpora, and competitive results on the monolingual word similarity and cross-lingual document classification task.
DONATE to arXiv: One hundred percent of your contribution will fund improvements and new initiatives to benefit arXiv's global scientific community. Please join the Simons Foundation and our generous member organizations and research labs in supporting arXiv. https://goo.gl/QIgRpr
from cs.AI updates on arXiv.org http://ift.tt/29hkYbZ
via IFTTT
Clique-Width and Directed Width Measures for Answer-Set Programming. (arXiv:1606.09449v1 [cs.AI])
Disjunctive Answer Set Programming (ASP) is a powerful declarative programming paradigm whose main decision problems are located on the second level of the polynomial hierarchy. Identifying tractable fragments and developing efficient algorithms for such fragments are thus important objectives in order to complement the sophisticated ASP systems available to date. Hard problems can become tractable if some problem parameter is bounded by a fixed constant; such problems are then called fixed-parameter tractable (FPT). While several FPT results for ASP exist, parameters that relate to directed or signed graphs representing the program at hand have been neglected so far. In this paper, we first give some negative observations showing that directed width measures on the dependency graph of a program do not lead to FPT results. We then consider the graph parameter of signed clique-width and present a novel dynamic programming algorithm that is FPT w.r.t. this parameter. Clique-width is more general than the well-known treewidth, and, to the best of our knowledge, ours is the first FPT algorithm for bounded clique-width for reasoning problems beyond SAT.
DONATE to arXiv: One hundred percent of your contribution will fund improvements and new initiatives to benefit arXiv's global scientific community. Please join the Simons Foundation and our generous member organizations and research labs in supporting arXiv. https://goo.gl/QIgRpr
from cs.AI updates on arXiv.org http://ift.tt/29a7f35
via IFTTT
Probabilistic Reasoning in the Description Logic ALCP with the Principle of Maximum Entropy (Full Version). (arXiv:1606.09521v1 [cs.AI])
A central question for knowledge representation is how to encode and handle uncertain knowledge adequately. We introduce the probabilistic description logic ALCP that is designed for representing context-dependent knowledge, where the actual context taking place is uncertain. ALCP allows the expression of logical dependencies on the domain and probabilistic dependencies on the possible contexts. In order to draw probabilistic conclusions, we employ the principle of maximum entropy. We provide reasoning algorithms for this logic, and show that it satisfies several desirable properties of probabilistic logics.
DONATE to arXiv: One hundred percent of your contribution will fund improvements and new initiatives to benefit arXiv's global scientific community. Please join the Simons Foundation and our generous member organizations and research labs in supporting arXiv. https://goo.gl/QIgRpr
from cs.AI updates on arXiv.org http://ift.tt/29hkLWe
via IFTTT
Ordering as privileged information. (arXiv:1606.09577v1 [cs.AI])
We propose to accelerate the rate of convergence of the pattern recognition task by directly minimizing the variance diameters of certain hypothesis spaces, which are critical quantities in fast-convergence results.We show that the variance diameters can be controlled by dividing hypothesis spaces into metric balls based on a new order metric. This order metric can be minimized as an ordinal regression problem, leading to a LUPI (Learning Using Privileged Information) application where we take the privileged information as some desired ordering, and construct a faster-converging hypothesis space by empirically restricting some larger hypothesis space according to that ordering. We give a risk analysis of the approach. We discuss the difficulties with model selection and give an innovative technique for selecting multiple model parameters. Finally, we provide some data experiments.
DONATE to arXiv: One hundred percent of your contribution will fund improvements and new initiatives to benefit arXiv's global scientific community. Please join the Simons Foundation and our generous member organizations and research labs in supporting arXiv. https://goo.gl/QIgRpr
from cs.AI updates on arXiv.org http://ift.tt/29a7mvj
via IFTTT
Performance Based Evaluation of Various Machine Learning Classification Techniques for Chronic Kidney Disease Diagnosis. (arXiv:1606.09581v1 [cs.LG])
Areas where Artificial Intelligence (AI) & related fields are finding their applications are increasing day by day, moving from core areas of computer science they are finding their applications in various other domains.In recent times Machine Learning i.e. a sub-domain of AI has been widely used in order to assist medical experts and doctors in the prediction, diagnosis and prognosis of various diseases and other medical disorders. In this manuscript the authors applied various machine learning algorithms to a problem in the domain of medical diagnosis and analyzed their efficiency in predicting the results. The problem selected for the study is the diagnosis of the Chronic Kidney Disease.The dataset used for the study consists of 400 instances and 24 attributes. The authors evaluated 12 classification techniques by applying them to the Chronic Kidney Disease data. In order to calculate efficiency, results of the prediction by candidate methods were compared with the actual medical results of the subject.The various metrics used for performance evaluation are predictive accuracy, precision, sensitivity and specificity. The results indicate that decision-tree performed best with nearly the accuracy of 98.6%, sensitivity of 0.9720, precision of 1 and specificity of 1.
DONATE to arXiv: One hundred percent of your contribution will fund improvements and new initiatives to benefit arXiv's global scientific community. Please join the Simons Foundation and our generous member organizations and research labs in supporting arXiv. https://goo.gl/QIgRpr
from cs.AI updates on arXiv.org http://ift.tt/29hkh2t
via IFTTT
Contextual Symmetries in Probabilistic Graphical Models. (arXiv:1606.09594v1 [cs.AI])
An important approach for efficient inference in probabilistic graphical models exploits symmetries among objects in the domain. Symmetric variables (states) are collapsed into meta-variables (meta-states) and inference algorithms are run over the lifted graphical model instead of the flat one. Our paper extends existing definitions of symmetry by introducing the novel notion of contextual symmetry. Two states that are not globally symmetric, can be contextually symmetric under some specific assignment to a subset of variables, referred to as the context variables. Contextual symmetry subsumes previous symmetry definitions and can rep resent a large class of symmetries not representable earlier. We show how to compute contextual symmetries by reducing it to the problem of graph isomorphism. We extend previous work on exploiting symmetries in the MCMC framework to the case of contextual symmetries. Our experiments on several domains of interest demonstrate that exploiting contextual symmetries can result in significant computational gains.
DONATE to arXiv: One hundred percent of your contribution will fund improvements and new initiatives to benefit arXiv's global scientific community. Please join the Simons Foundation and our generous member organizations and research labs in supporting arXiv. https://goo.gl/QIgRpr
from cs.AI updates on arXiv.org http://ift.tt/29a6Yx3
via IFTTT
A Permutation-based Model for Crowd Labeling: Optimal Estimation and Robustness. (arXiv:1606.09632v1 [cs.LG])
The aggregation and denoising of crowd labeled data is a task that has gained increased significance with the advent of crowdsourcing platforms and massive datasets. In this paper, we propose a permutation-based model for crowd labeled data that is a significant generalization of the common Dawid-Skene model, and introduce a new error metric by which to compare different estimators. Working in a high-dimensional non-asymptotic framework that allows both the number of workers and tasks to scale, we derive optimal rates of convergence for the permutation-based model. We show that the permutation-based model offers significant robustness in estimation due to its richness, while surprisingly incurring only a small additional statistical penalty as compared to the Dawid-Skene model. Finally, we propose a computationally-efficient method, called the OBI-WAN estimator, that is uniformly optimal over a class intermediate between the permutation-based and the Dawid-Skene models, and is uniformly consistent over the entire permutation-based model class. In contrast, the guarantees for estimators available in prior literature are sub-optimal over the original Dawid-Skene model.
DONATE to arXiv: One hundred percent of your contribution will fund improvements and new initiatives to benefit arXiv's global scientific community. Please join the Simons Foundation and our generous member organizations and research labs in supporting arXiv. https://goo.gl/QIgRpr
from cs.AI updates on arXiv.org http://ift.tt/29hkVNl
via IFTTT
Lifted Region-Based Belief Propagation. (arXiv:1606.09637v1 [cs.AI])
Due to the intractable nature of exact lifted inference, research has recently focused on the discovery of accurate and efficient approximate inference algorithms in Statistical Relational Models (SRMs), such as Lifted First-Order Belief Propagation. FOBP simulates propositional factor graph belief propagation without constructing the ground factor graph by identifying and lifting over redundant message computations. In this work, we propose a generalization of FOBP called Lifted Generalized Belief Propagation, in which both the region structure and the message structure can be lifted. This approach allows more of the inference to be performed intra-region (in the exact inference step of BP), thereby allowing simulation of propagation on a graph structure with larger region scopes and fewer edges, while still maintaining tractability. We demonstrate that the resulting algorithm converges in fewer iterations to more accurate results on a variety of SRMs.
DONATE to arXiv: One hundred percent of your contribution will fund improvements and new initiatives to benefit arXiv's global scientific community. Please join the Simons Foundation and our generous member organizations and research labs in supporting arXiv. https://goo.gl/QIgRpr
from cs.AI updates on arXiv.org http://ift.tt/29a7mvn
via IFTTT
Networked Intelligence: Towards Autonomous Cyber Physical Systems. (arXiv:1606.04087v4 [cs.AI] UPDATED)
The development of intelligent systems requires synthesis of progress made both in commerce and academia. In this report you find an overview of relevant research fields and industrially applicable technologies for building very large scale cyber physical systems. A concept architecture is used to illustrate how existing pieces may fit together, and the maturity of the subsystems is estimated.
The goal is to structure the developments and the challenge of machine intelligence for Consumer and Industrial Internet technologists, cyber physical systems researchers and people interested in the convergence of data & Internet of Things. It can be used for planning developments of intelligent systems.
DONATE to arXiv: One hundred percent of your contribution will fund improvements and new initiatives to benefit arXiv's global scientific community. Please join the Simons Foundation and our generous member organizations and research labs in supporting arXiv. https://goo.gl/QIgRpr
from cs.AI updates on arXiv.org http://ift.tt/1PZbsJd
via IFTTT
I have a new follower on Twitter
Jordi Ollier-Howard
#LGBT #GayLewisham #Gay #Chemsex #Recovering #CMA #NA Instagram: CatfordLad / KIK: JordiGOH / Facebook: JordiOH
Lewisham, London
https://t.co/GPEsUFQTkk
Following: 2043 - Followers: 529
June 30, 2016 at 06:27PM via Twitter http://twitter.com/JordiOH
Orioles need 3 HRs to tie record for most in any month (58), have gone deep on June 30 in 22 straight years - Eddie Matz (ESPN)
via IFTTT
Anonymous Buyer Snaps Up Trump-Maples Engagement Ring
from Google Alert - anonymous http://ift.tt/296tcPH
via IFTTT
Oculus CEO's Twitter gets Hacked; Hacker declares himself new CEO
from The Hacker News http://ift.tt/297XtT5
via IFTTT
Function auto post doesn't work with your Anonymous Post
from Google Alert - anonymous http://ift.tt/2950KN4
via IFTTT
ISS Daily Summary Report – 06/29/16
from ISS On-Orbit Status Report http://ift.tt/29dmWIN
via IFTTT
Check 'My Activity' Dashboard to know how much Google knows about you
from The Hacker News http://ift.tt/294ghNz
via IFTTT
From Alpha to Omega in Crete
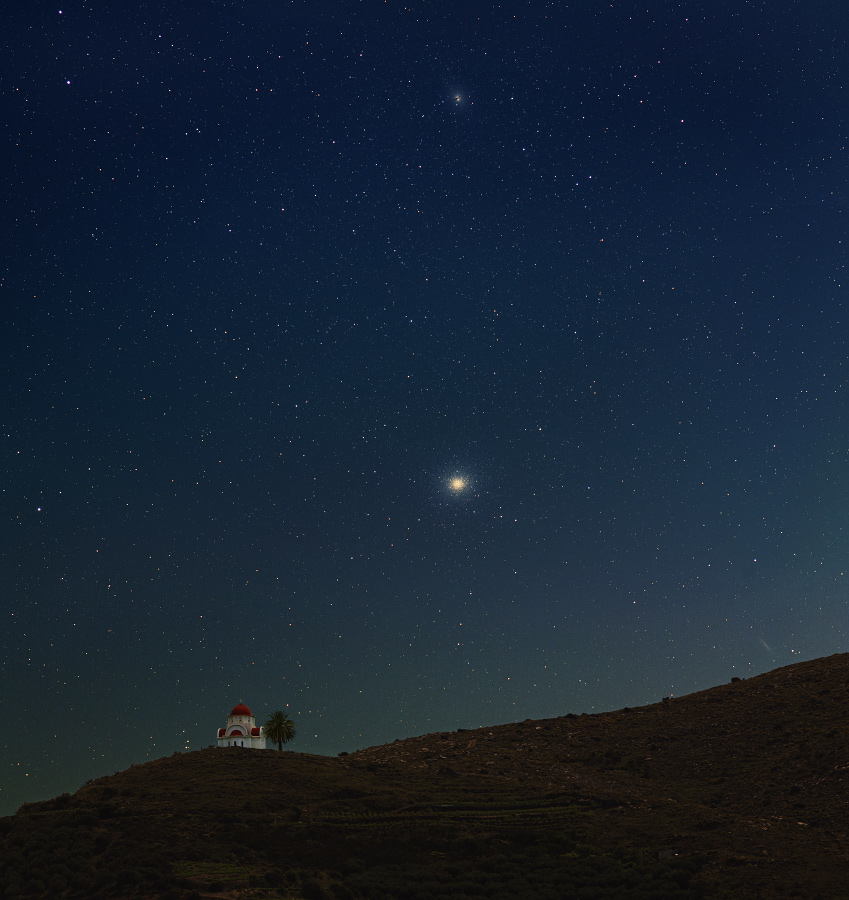
Wednesday, June 29, 2016
I have a new follower on Twitter
George Shiber
Alumnus: @Columbia University and @GC_CUNY: #Philosophy #Mathematics #Logic. Probing SuperString/M-Theory. Veni Vidi Vici. Breathing #Science and #Philosophy
New York, USA
https://t.co/7uMWhZFUZS
Following: 15317 - Followers: 16149
June 29, 2016 at 11:05PM via Twitter http://twitter.com/GeorgeShiber
Anonymous Viewer
from Google Alert - anonymous http://ift.tt/29r4h9X
via IFTTT
Technical Report: Towards a Universal Code Formatter through Machine Learning. (arXiv:1606.08866v1 [cs.PL])
There are many declarative frameworks that allow us to implement code formatters relatively easily for any specific language, but constructing them is cumbersome. The first problem is that "everybody" wants to format their code differently, leading to either many formatter variants or a ridiculous number of configuration options. Second, the size of each implementation scales with a language's grammar size, leading to hundreds of rules.
In this paper, we solve the formatter construction problem using a novel approach, one that automatically derives formatters for any given language without intervention from a language expert. We introduce a code formatter called CodeBuff that uses machine learning to abstract formatting rules from a representative corpus, using a carefully designed feature set. Our experiments on Java, SQL, and ANTLR grammars show that CodeBuff is efficient, has excellent accuracy, and is grammar invariant for a given language. It also generalizes to a 4th language tested during manuscript preparation.
DONATE to arXiv: One hundred percent of your contribution will fund improvements and new initiatives to benefit arXiv's global scientific community. Please join the Simons Foundation and our generous member organizations and research labs in supporting arXiv. https://goo.gl/QIgRpr
from cs.AI updates on arXiv.org http://ift.tt/291ux99
via IFTTT
On the Semantic Relationship between Probabilistic Soft Logic and Markov Logic. (arXiv:1606.08896v1 [cs.AI])
Markov Logic Networks (MLN) and Probabilistic Soft Logic (PSL) are widely applied formalisms in Statistical Relational Learning, an emerging area in Artificial Intelligence that is concerned with combining logical and statistical AI. Despite their resemblance, the relationship has not been formally stated. In this paper, we describe the precise semantic relationship between them from a logical perspective. This is facilitated by first extending fuzzy logic to allow weights, which can be also viewed as a generalization of PSL, and then relate that generalization to MLN. We observe that the relationship between PSL and MLN is analogous to the known relationship between fuzzy logic and Boolean logic, and furthermore the weight scheme of PSL is essentially a generalization of the weight scheme of MLN for the many-valued setting.
DONATE to arXiv: One hundred percent of your contribution will fund improvements and new initiatives to benefit arXiv's global scientific community. Please join the Simons Foundation and our generous member organizations and research labs in supporting arXiv. https://goo.gl/QIgRpr
from cs.AI updates on arXiv.org http://ift.tt/29qYW2w
via IFTTT
Exploring high-level Perspectives on Self-Configuration Capabilities of Systems. (arXiv:1606.08906v1 [cs.AI])
Optimization of product performance repetitively introduces the need to make products adaptive in a more general sense. This more general idea is often captured under the term 'self-configuration'. Despite the importance of such capability, research work on this feature appears isolated by technical domains. It is not easy to tell quickly whether the approaches chosen in different technological domains introduce new ideas or whether the differences just reflect domain idiosyncrasies. For the sake of easy identification of key differences between systems with self-configuring capabilities, I will explore higher level concepts for understanding self-configuration, such as the {\Omega}-units, in order to provide theoretical instruments for connecting different areas of technology and research.
DONATE to arXiv: One hundred percent of your contribution will fund improvements and new initiatives to benefit arXiv's global scientific community. Please join the Simons Foundation and our generous member organizations and research labs in supporting arXiv. https://goo.gl/QIgRpr
from cs.AI updates on arXiv.org http://ift.tt/295tQ23
via IFTTT
subgraph2vec: Learning Distributed Representations of Rooted Sub-graphs from Large Graphs. (arXiv:1606.08928v1 [cs.LG])
In this paper, we present subgraph2vec, a novel approach for learning latent representations of rooted subgraphs from large graphs inspired by recent advancements in Deep Learning and Graph Kernels. These latent representations encode semantic substructure dependencies in a continuous vector space, which is easily exploited by statistical models for tasks such as graph classification, clustering, link prediction and community detection. subgraph2vec leverages on local information obtained from neighbourhoods of nodes to learn their latent representations in an unsupervised fashion. We demonstrate that subgraph vectors learnt by our approach could be used in conjunction with classifiers such as CNNs, SVMs and relational data clustering algorithms to achieve significantly superior accuracies. Also, we show that the subgraph vectors could be used for building a deep learning variant of Weisfeiler-Lehman graph kernel. Our experiments on several benchmark and large-scale real-world datasets reveal that subgraph2vec achieves significant improvements in accuracies over existing graph kernels on both supervised and unsupervised learning tasks. Specifically, on two realworld program analysis tasks, namely, code clone and malware detection, subgraph2vec outperforms state-of-the-art kernels by more than 17% and 4%, respectively.
DONATE to arXiv: One hundred percent of your contribution will fund improvements and new initiatives to benefit arXiv's global scientific community. Please join the Simons Foundation and our generous member organizations and research labs in supporting arXiv. https://goo.gl/QIgRpr
from cs.AI updates on arXiv.org http://ift.tt/2998ktw
via IFTTT
Greedy, Joint Syntactic-Semantic Parsing with Stack LSTMs. (arXiv:1606.08954v1 [cs.CL])
We present a transition-based parser that jointly produces syntactic and semantic dependencies. It learns a representation of the entire algorithm state, using stack long short-term memories. Our greedy inference algorithm has linear time, including feature extraction. On the CoNLL 2008--9 English shared tasks, we obtain the best published parsing performance among models that jointly learn syntax and semantics.
DONATE to arXiv: One hundred percent of your contribution will fund improvements and new initiatives to benefit arXiv's global scientific community. Please join the Simons Foundation and our generous member organizations and research labs in supporting arXiv. https://goo.gl/QIgRpr
from cs.AI updates on arXiv.org http://ift.tt/29qZ3Ls
via IFTTT
Evaluation and selection of Medical Tourism sites: A rough AHP based MABAC approach. (arXiv:1606.08962v1 [cs.AI])
In this paper, a novel multiple criteria decision making (MCDM) methodology is presented for assessing and prioritizing medical tourism destinations in uncertain environment. A systematic evaluation and assessment method is proposed by integrating rough number based AHP (Analytic Hierarchy Process) and rough number based MABAC (Multi-Attributive Border Approximation area Comparison). Rough number is used to aggregate individual judgments and preferences to deal with vagueness in decision making due to limited data. Rough AHP analyzes the relative importance of criteria based on their preferences given by experts. Rough MABAC evaluates the alternative sites based on the criteria weights. The proposed methodology is explained through a case study considering different cities for healthcare service in India. The validity of the obtained ranking for the given decision making problem is established by testing criteria proposed by Wang and Triantaphyllou (2008) along with further analysis and discussion.
DONATE to arXiv: One hundred percent of your contribution will fund improvements and new initiatives to benefit arXiv's global scientific community. Please join the Simons Foundation and our generous member organizations and research labs in supporting arXiv. https://goo.gl/QIgRpr
from cs.AI updates on arXiv.org http://ift.tt/295tWGF
via IFTTT
Non-linear Label Ranking for Large-scale Prediction of Long-Term User Interests. (arXiv:1606.08963v1 [cs.AI])
We consider the problem of personalization of online services from the viewpoint of ad targeting, where we seek to find the best ad categories to be shown to each user, resulting in improved user experience and increased advertisers' revenue. We propose to address this problem as a task of ranking the ad categories depending on a user's preference, and introduce a novel label ranking approach capable of efficiently learning non-linear, highly accurate models in large-scale settings. Experiments on a real-world advertising data set with more than 3.2 million users show that the proposed algorithm outperforms the existing solutions in terms of both rank loss and top-K retrieval performance, strongly suggesting the benefit of using the proposed model on large-scale ranking problems.
DONATE to arXiv: One hundred percent of your contribution will fund improvements and new initiatives to benefit arXiv's global scientific community. Please join the Simons Foundation and our generous member organizations and research labs in supporting arXiv. https://goo.gl/QIgRpr
from cs.AI updates on arXiv.org http://ift.tt/291u2Ms
via IFTTT
Credibilistic TOPSIS Model for Evaluation and Selection of Municipal Solid Waste Disposal Methods. (arXiv:1606.08965v1 [cs.AI])
Municipal solid waste management (MSWM) is a challenging issue of urban development in developing countries. Each country having different socio-economic-environmental background, might not accept a particular disposal method as the optimal choice. Selection of suitable disposal method in MSWM, under vague and imprecise information can be considered as multi criteria decision making problem (MCDM). In the present paper, TOPSIS (Technique for Order Preference by Similarity to Ideal Solution) methodology is extended based on credibility theory for evaluating the performances of MSW disposal methods under some criteria fixed by experts. The proposed model helps decision makers to choose a preferable alternative for their municipal area. A sensitivity analysis by our proposed model confirms this fact.
DONATE to arXiv: One hundred percent of your contribution will fund improvements and new initiatives to benefit arXiv's global scientific community. Please join the Simons Foundation and our generous member organizations and research labs in supporting arXiv. https://goo.gl/QIgRpr
from cs.AI updates on arXiv.org http://ift.tt/29qZ425
via IFTTT
Algebraic foundations for qualitative calculi and networks. (arXiv:1606.09140v1 [cs.AI])
A qualitative representation $\phi$ is like an ordinary representation of a relation algebra, but instead of requiring $(a; b)^\phi = a^\phi | b^\phi$, as we do for ordinary representations, we only require that $c^\phi\supseteq a^\phi | b^\phi \iff c\geq a ; b$, for each $c$ in the algebra. A constraint network is qualitatively satisfiable if its nodes can be mapped to elements of a qualitative representation, preserving the constraints. If a constraint network is satisfiable then it is clearly qualitatively satisfiable, but the converse can fail. However, for a wide range of relation algebras including the point algebra, the Allen Interval Algebra, RCC8 and many others, a network is satisfiable if and only if it is qualitatively satisfiable.
Unlike ordinary composition, the weak composition arising from qualitative representations need not be associative, so we can generalise by considering network satisfaction problems over non-associative algebras. We prove that computationally, qualitative representations have many advantages over ordinary representations: whereas many finite relation algebras have only infinite representations, every finite qualitatively representable algebra has a finite qualitative representation; the representability problem for (the atom structures of) finite non-associative algebras is NP-complete; the network satisfaction problem over a finite qualitatively representable algebra is always in NP; the validity of equations over qualitative representations is co-NP-complete. On the other hand we prove that there is no finite axiomatisation of the class of qualitatively representable algebras.
DONATE to arXiv: One hundred percent of your contribution will fund improvements and new initiatives to benefit arXiv's global scientific community. Please join the Simons Foundation and our generous member organizations and research labs in supporting arXiv. https://goo.gl/QIgRpr
from cs.AI updates on arXiv.org http://ift.tt/295tYyw
via IFTTT
Tractability and Decompositions of Global Cost Functions. (arXiv:1502.02414v2 [cs.AI] UPDATED)
Enforcing local consistencies in cost function networks is performed by applying so-called Equivalent Preserving Transformations (EPTs) to the cost functions. As EPTs transform the cost functions, they may break the property that was making local consistency enforcement tractable on a global cost function. A global cost function is called tractable projection-safe when applying an EPT to it is tractable and does not break the tractability property. In this paper, we prove that depending on the size r of the smallest scopes used for performing EPTs, the tractability of global cost functions can be preserved (r = 0) or destroyed (r > 1). When r = 1, the answer is indefinite. We show that on a large family of cost functions, EPTs can be computed via dynamic programming-based algorithms, leading to tractable projection-safety. We also show that when a global cost function can be decomposed into a Berge acyclic network of bounded arity cost functions, soft local consistencies such as soft Directed or Virtual Arc Consistency can directly emulate dynamic programming. These different approaches to decomposable cost functions are then embedded in a solver for extensive experiments that confirm the feasibility and efficiency of our proposal.
DONATE to arXiv: One hundred percent of your contribution will fund improvements and new initiatives to benefit arXiv's global scientific community. Please join the Simons Foundation and our generous member organizations and research labs in supporting arXiv. https://goo.gl/QIgRpr
from cs.AI updates on arXiv.org http://ift.tt/1CNwGly
via IFTTT
A Single-Pass Classifier for Categorical Data. (arXiv:1503.02521v4 [cs.AI] UPDATED)
This paper describes a new method for classifying a dataset that partitions elements into their categories. It has relations with neural networks but a slightly different structure, requiring only a single pass through the classifier to generate the weight sets. A grid-like structure is required as part of a novel idea of converting a 1-D row of real values into a 2-D structure of value bands. Each cell in any band then stores a distinct set of weights, to represent its own importance and its relation to each output category. During classification, all of the output weight lists can be retrieved and summed to produce a probability for what the correct output category is. The bands possibly work like hidden layers of neurons, but they are variable specific, making the process orthogonal. The construction process can be a single update process without iterations, making it potentially much faster. It can also be compared with k-NN and may be practical for partial or competitive updating.
DONATE to arXiv: One hundred percent of your contribution will fund improvements and new initiatives to benefit arXiv's global scientific community. Please join the Simons Foundation and our generous member organizations and research labs in supporting arXiv. https://goo.gl/QIgRpr
from cs.AI updates on arXiv.org http://ift.tt/1ENtzts
via IFTTT
Mapping Heritability of Large-Scale Brain Networks with a Billion Connections {\em via} Persistent Homology. (arXiv:1509.04771v2 [cs.AI] UPDATED)
In many human brain network studies, we do not have sufficient number (n) of images relative to the number (p) of voxels due to the prohibitively expensive cost of scanning enough subjects. Thus, brain network models usually suffer the small-n large-p problem. Such a problem is often remedied by sparse network models, which are usually solved numerically by optimizing L1-penalties. Unfortunately, due to the computational bottleneck associated with optimizing L1-penalties, it is not practical to apply such methods to construct large-scale brain networks at the voxel-level. In this paper, we propose a new scalable sparse network model using cross-correlations that bypass the computational bottleneck. Our model can build sparse brain networks at the voxel level with p > 25000. Instead of using a single sparse parameter that may not be optimal in other studies and datasets, the computational speed gain enables us to analyze the collection of networks at every possible sparse parameter in a coherent mathematical framework via persistent homology. The method is subsequently applied in determining the extent of heritability on a functional brain network at the voxel-level for the first time using twin fMRI.
DONATE to arXiv: One hundred percent of your contribution will fund improvements and new initiatives to benefit arXiv's global scientific community. Please join the Simons Foundation and our generous member organizations and research labs in supporting arXiv. https://goo.gl/QIgRpr
from cs.AI updates on arXiv.org http://ift.tt/1Fhseht
via IFTTT
Consciousness is Pattern Recognition. (arXiv:1605.03009v2 [cs.AI] UPDATED)
This is a proof of the strong AI hypothesis, i.e. that machines can be conscious. It is a phenomenological proof that pattern-recognition and subjective consciousness are the same activity in different terms. Therefore, it proves that essential subjective processes of consciousness are computable, and identifies significant traits and requirements of a conscious system. Since Husserl, many philosophers have accepted that consciousness consists of memories of logical connections between an ego and external objects. These connections are called "intentions." Pattern recognition systems are achievable technical artifacts. The proof links this respected introspective philosophical theory of consciousness with technical art. The proof therefore endorses the strong AI hypothesis and may therefore also enable a theoretically-grounded form of artificial intelligence called a "synthetic intentionality," able to synthesize, generalize, select and repeat intentions. If the pattern recognition is reflexive, able to operate on the set of intentions, and flexible, with several methods of synthesizing intentions, an SI may be a particularly strong form of AI. Similarities and possible applications to several AI paradigms are discussed. The article then addresses some problems: The proof's limitations, reflexive cognition, Searles' Chinese room, and how an SI could "understand" "meanings" and "be creative."
DONATE to arXiv: One hundred percent of your contribution will fund improvements and new initiatives to benefit arXiv's global scientific community. Please join the Simons Foundation and our generous member organizations and research labs in supporting arXiv. https://goo.gl/QIgRpr
from cs.AI updates on arXiv.org http://ift.tt/24M4BJZ
via IFTTT
Orioles Video: Mark Trumbo crushes 2-run shot into second deck in 12-6 win over Padres; MLB-best 23rd HR of season (ESPN)
via IFTTT
MLB: Mark Trumbo's 4th-inning HR was Orioles' 55th in June, tying mark set by 1996 A's; most HR for any month is 58 (ESPN)
via IFTTT
"Pretty Little Liars" recap (7.20): So long, anonymous white guys!
from Google Alert - anonymous http://ift.tt/296bDA2
via IFTTT
docker/docker
from Google Alert - anonymous http://ift.tt/295qP4m
via IFTTT
Global Terrorism Database Leaked! Reveals 2.2 Million Suspected Terrorists
from The Hacker News http://ift.tt/299m8pE
via IFTTT
ISS Daily Summary Report – 06/28/16
from ISS On-Orbit Status Report http://ift.tt/29amc78
via IFTTT
China Orders Apple to Monitor App Store Users and Track their Identities
from The Hacker News http://ift.tt/293aNmP
via IFTTT
STOP Sharing that Facebook Privacy and Permission Notice, It's a HOAX
from The Hacker News http://ift.tt/291WtKn
via IFTTT
Orioles Video: Chris Davis parks the ball over the center-field wall in the 6th inning of 11-7 win over the Padres (ESPN)
via IFTTT
Tuesday, June 28, 2016
Towards Verified Artificial Intelligence. (arXiv:1606.08514v1 [cs.AI])
Verified artificial intelligence (AI) is the goal of designing AI-based systems that are provably correct with respect to mathematically-specified requirements. This paper considers Verified AI from a formal methods perspective. We describe five challenges for achieving Verified AI, and five corresponding principles for addressing these challenges.
DONATE to arXiv: One hundred percent of your contribution will fund improvements and new initiatives to benefit arXiv's global scientific community. Please join the Simons Foundation and our generous member organizations and research labs in supporting arXiv. https://goo.gl/QIgRpr
from cs.AI updates on arXiv.org http://ift.tt/293kiU2
via IFTTT
A Learning Algorithm for Relational Logistic Regression: Preliminary Results. (arXiv:1606.08531v1 [cs.AI])
Relational logistic regression (RLR) is a representation of conditional probability in terms of weighted formulae for modelling multi-relational data. In this paper, we develop a learning algorithm for RLR models. Learning an RLR model from data consists of two steps: 1- learning the set of formulae to be used in the model (a.k.a. structure learning) and learning the weight of each formula (a.k.a. parameter learning). For structure learning, we deploy Schmidt and Murphy's hierarchical assumption: first we learn a model with simple formulae, then more complex formulae are added iteratively only if all their sub-formulae have proven effective in previous learned models. For parameter learning, we convert the problem into a non-relational learning problem and use an off-the-shelf logistic regression learning algorithm from Weka, an open-source machine learning tool, to learn the weights. We also indicate how hidden features about the individuals can be incorporated into RLR to boost the learning performance. We compare our learning algorithm to other structure and parameter learning algorithms in the literature, and compare the performance of RLR models to standard logistic regression and RDN-Boost on a modified version of the MovieLens data-set.
DONATE to arXiv: One hundred percent of your contribution will fund improvements and new initiatives to benefit arXiv's global scientific community. Please join the Simons Foundation and our generous member organizations and research labs in supporting arXiv. https://goo.gl/QIgRpr
from cs.AI updates on arXiv.org http://ift.tt/294qSfs
via IFTTT
A Local Density-Based Approach for Local Outlier Detection. (arXiv:1606.08538v1 [cs.AI])
This paper presents a simple but effective density-based outlier detection approach with the local kernel density estimation (KDE). A Relative Density-based Outlier Score (RDOS) is introduced to measure the local outlierness of objects, in which the density distribution at the location of an object is estimated with a local KDE method based on extended nearest neighbors of the object. Instead of using only $k$ nearest neighbors, we further consider reverse nearest neighbors and shared nearest neighbors of an object for density distribution estimation. Some theoretical properties of the proposed RDOS including its expected value and false alarm probability are derived. A comprehensive experimental study on both synthetic and real-life data sets demonstrates that our approach is more effective than state-of-the-art outlier detection methods.
DONATE to arXiv: One hundred percent of your contribution will fund improvements and new initiatives to benefit arXiv's global scientific community. Please join the Simons Foundation and our generous member organizations and research labs in supporting arXiv. https://goo.gl/QIgRpr
from cs.AI updates on arXiv.org http://ift.tt/293kouE
via IFTTT
"Show me the cup": Reference with Continuous Representations. (arXiv:1606.08777v1 [cs.CL])
One of the most basic functions of language is to refer to objects in a shared scene. Modeling reference with continuous representations is challenging because it requires individuation, i.e., tracking and distinguishing an arbitrary number of referents. We introduce a neural network model that, given a definite description and a set of objects represented by natural images, points to the intended object if the expression has a unique referent, or indicates a failure, if it does not. The model, directly trained on reference acts, is competitive with a pipeline manually engineered to perform the same task, both when referents are purely visual, and when they are characterized by a combination of visual and linguistic properties.
DONATE to arXiv: One hundred percent of your contribution will fund improvements and new initiatives to benefit arXiv's global scientific community. Please join the Simons Foundation and our generous member organizations and research labs in supporting arXiv. https://goo.gl/QIgRpr
from cs.AI updates on arXiv.org http://ift.tt/297sbuK
via IFTTT
Adaptive Training of Random Mapping for Data Quantization. (arXiv:1606.08808v1 [cs.LG])
Data quantization learns encoding results of data with certain requirements, and provides a broad perspective of many real-world applications to data handling. Nevertheless, the results of encoder is usually limited to multivariate inputs with the random mapping, and side information of binary codes are hardly to mostly depict the original data patterns as possible. In the literature, cosine based random quantization has attracted much attentions due to its intrinsic bounded results. Nevertheless, it usually suffers from the uncertain outputs, and information of original data fails to be fully preserved in the reduced codes. In this work, a novel binary embedding method, termed adaptive training quantization (ATQ), is proposed to learn the ideal transform of random encoder, where the limitation of cosine random mapping is tackled. As an adaptive learning idea, the reduced mapping is adaptively calculated with idea of data group, while the bias of random transform is to be improved to hold most matching information. Experimental results show that the proposed method is able to obtain outstanding performance compared with other random quantization methods.
DONATE to arXiv: One hundred percent of your contribution will fund improvements and new initiatives to benefit arXiv's global scientific community. Please join the Simons Foundation and our generous member organizations and research labs in supporting arXiv. https://goo.gl/QIgRpr
from cs.AI updates on arXiv.org http://ift.tt/29mz1sQ
via IFTTT
Active Ranking from Pairwise Comparisons and the Futility of Parametric Assumptions. (arXiv:1606.08842v1 [cs.LG])
We consider sequential or active ranking of a set of n items based on noisy pairwise comparisons. Items are ranked according to the probability that a given item beats a randomly chosen item, and ranking refers to partitioning the items into sets of pre-specified sizes according to their scores. This notion of ranking includes as special cases the identification of the top-k items and the total ordering of the items. We first analyze a sequential ranking algorithm that counts the number of comparisons won, and uses these counts to decide whether to stop, or to compare another pair of items, chosen based on confidence intervals specified by the data collected up to that point. We prove that this algorithm succeeds in recovering the ranking using a number of comparisons that is optimal up to logarithmic factors. This guarantee does not require any structural properties of the underlying pairwise probability matrix, unlike a significant body of past work on pairwise ranking based on parametric models such as the Thurstone or Bradley-Terry-Luce models. It has been a long-standing open question as to whether or not imposing these parametric assumptions allow for improved ranking algorithms. Our second contribution settles this issue in the context of the problem of active ranking from pairwise comparisons: by means of tight lower bounds, we prove that perhaps surprisingly, these popular parametric modeling choices offer little statistical advantage.
DONATE to arXiv: One hundred percent of your contribution will fund improvements and new initiatives to benefit arXiv's global scientific community. Please join the Simons Foundation and our generous member organizations and research labs in supporting arXiv. https://goo.gl/QIgRpr
from cs.AI updates on arXiv.org http://ift.tt/29aiJau
via IFTTT
Deep Reinforcement Learning from Self-Play in Imperfect-Information Games. (arXiv:1603.01121v2 [cs.LG] UPDATED)
Many real-world applications can be described as large-scale games of imperfect information. To deal with these challenging domains, prior work has focused on computing Nash equilibria in a handcrafted abstraction of the domain. In this paper we introduce the first scalable end-to-end approach to learning approximate Nash equilibria without prior domain knowledge. Our method combines fictitious self-play with deep reinforcement learning. When applied to Leduc poker, Neural Fictitious Self-Play (NFSP) approached a Nash equilibrium, whereas common reinforcement learning methods diverged. In Limit Texas Holdem, a poker game of real-world scale, NFSP learnt a strategy that approached the performance of state-of-the-art, superhuman algorithms based on significant domain expertise.
DONATE to arXiv: One hundred percent of your contribution will fund improvements and new initiatives to benefit arXiv's global scientific community. Please join the Simons Foundation and our generous member organizations and research labs in supporting arXiv. https://goo.gl/QIgRpr
from cs.AI updates on arXiv.org http://ift.tt/1QWDK7A
via IFTTT
Landmark-based Plan Recognition. (arXiv:1604.01277v2 [cs.AI] UPDATED)
Recognition of goals and plans using incomplete evidence from action execution can be done efficiently by using planning techniques. In many applications it is important to recognize goals and plans not only accurately, but also quickly. In this paper, we develop a heuristic approach for recognizing plans based on planning techniques that rely on ordering constraints to filter candidate goals from observations. These ordering constraints are called landmarks in the planning literature, which are facts or actions that cannot be avoided to achieve a goal. We show the applicability of planning landmarks in two settings: first, we use it directly to develop a heuristic-based plan recognition approach; second, we refine an existing planning-based plan recognition approach by pre-filtering its candidate goals. Our empirical evaluation shows that our approach is not only substantially more accurate than the state-of-the-art in all available datasets, it is also an order of magnitude faster.
DONATE to arXiv: One hundred percent of your contribution will fund improvements and new initiatives to benefit arXiv's global scientific community. Please join the Simons Foundation and our generous member organizations and research labs in supporting arXiv. https://goo.gl/QIgRpr
from cs.AI updates on arXiv.org http://ift.tt/1SOnFhC
via IFTTT
Teaching natural language to computers. (arXiv:1604.08781v2 [cs.CL] UPDATED)
"Natural Language," whether spoken and attended to by humans, or processed and generated by computers, requires networked structures that reflect creative processes in semantic, syntactic, phonetic, linguistic, social, emotional, and cultural modules. Being able to produce novel and useful behavior following repeated practice gets to the root of both artificial intelligence and human language. This paper investigates the modalities involved in language-like applications that computers -- and programmers -- engage with, and aims to fine tune the questions we ask to better account for context, self-awareness, and embodiment.
DONATE to arXiv: One hundred percent of your contribution will fund improvements and new initiatives to benefit arXiv's global scientific community. Please join the Simons Foundation and our generous member organizations and research labs in supporting arXiv. https://goo.gl/QIgRpr
from cs.AI updates on arXiv.org http://ift.tt/1O8ysPZ
via IFTTT
The Transport Consortium starts testing the Public Transport Card with anonymous passes
from Google Alert - anonymous http://ift.tt/291bBfD
via IFTTT
Orioles: P T.J. McFarland recalled from Triple-A Tuesday; appeared in 23 innings over 14 games with Baltimore in 2016 (ESPN)
via IFTTT
IoT Botnet — 25,000 CCTV Cameras Hacked to launch DDoS Attack
from The Hacker News http://ift.tt/290Y1ZG
via IFTTT
[FD] KL-001-2016-002 : Ubiquiti Administration Portal CSRF to Remote Command Execution
Source: Gmail -> IFTTT-> Blogger
One Less Gun
from Google Alert - anonymous http://ift.tt/291u3kK
via IFTTT
[FD] [KIS-2016-10] Concrete5 <= 5.7.3.1 (Application::dispatch) Local File Inclusion Vulnerability
Source: Gmail -> IFTTT-> Blogger
[FD] [KIS-2016-08] Concrete5 <= 5.7.3.1 Multiple Cross-Site Request Forgeries Vulnerabilities
Source: Gmail -> IFTTT-> Blogger
Zero-Day Warning! Ransomware targets Microsoft Office 365 Users
from The Hacker News http://ift.tt/29173D1
via IFTTT
ISS Daily Summary Report – 06/27/16
from ISS On-Orbit Status Report http://ift.tt/28XGlsV
via IFTTT
[FD] Iranian Weblog Services v3.3 CMS - Multiple Web Vulnerabilities
Source: Gmail -> IFTTT-> Blogger