Latest YouTube Video
Saturday, April 8, 2017
Anonymous helpers lend a hand
from Google Alert - anonymous http://ift.tt/2oc9Wbw
via IFTTT
You are Welcome at Alcoholics Anonymous
from Google Alert - anonymous http://ift.tt/2obD7va
via IFTTT
Ravens re-sign DB Lardarius Webb to 3-year deal - Adam Schefter; was previously released by team on March 10 (ESPN)
via IFTTT
Empathy on Stage: The Collector and Anonymous in Dublin
from Google Alert - anonymous http://ift.tt/2ns6Qmo
via IFTTT
Shadow Brokers Group Releases More Stolen NSA Hacking Tools & Exploits
from The Hacker News http://ift.tt/2penx18
via IFTTT
Menuet con variazioni
from Google Alert - anonymous http://ift.tt/2o9dZ8e
via IFTTT
Zeta Oph: Runaway Star
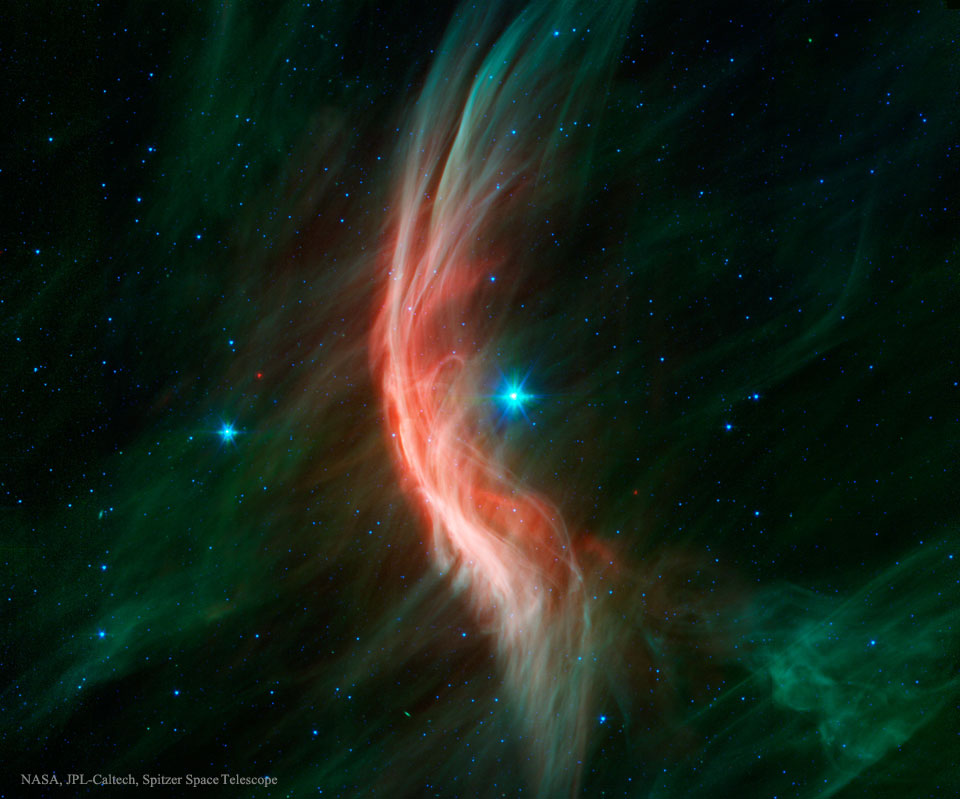
Friday, April 7, 2017
Easy anonymous button
from Google Alert - anonymous http://ift.tt/2oO6kis
via IFTTT
WATCH: O'Reilly Ad Factor; Anonymous Account; Fake Front Page
from Google Alert - anonymous http://ift.tt/2nUmt2t
via IFTTT
Orioles acquire RP Miguel Castro from Rockies for player to be named later or cash; 22 ER over 32.1 career IP (ESPN)
via IFTTT
I have a new follower on Twitter
Cailen Melville
Chess player . I also help defeat cybercriminals. ⚜
San Francisco, CA
https://t.co/iTIk0LkrcB
Following: 2332 - Followers: 4702
April 07, 2017 at 06:04PM via Twitter http://twitter.com/cailenmelville
I have a new follower on Twitter
InfoSec HotSpot
The Latest Regarding Cyber Security https://t.co/CHaVgpsrLO infosechotspot@gmail.com
USA
https://t.co/W48XPTvIt1
Following: 51320 - Followers: 101202
April 07, 2017 at 05:24PM via Twitter http://twitter.com/InfoSecHotSpot
Ravens' 1996 draft class that included Ray Lewis and Jonathan Ogden rated as 10th best in NFL history by ESPN analytics (ESPN)
via IFTTT
WikiLeaks Reveals CIA's Grasshopper Windows Hacking Framework
from The Hacker News http://ift.tt/2ogXr0u
via IFTTT
Ravens expected to re-sign CB Lardarius Webb barring any final complications with deal - Jamison Hensley (ESPN)
via IFTTT
ISS Daily Summary Report – 4/06/2017
from ISS On-Orbit Status Report http://ift.tt/2oMf3BT
via IFTTT
I have a new follower on Twitter
Iqbal Azad
I am the creator of the record label @ThinkersRave. A music channel like @Vevo @billboard @bbcmusic where we post new #musicvideo every friday.
Manhattan, NY
https://t.co/pPb3IlO5Fc
Following: 1578 - Followers: 927
April 07, 2017 at 02:39AM via Twitter http://twitter.com/TheIqbalAzad
Castle Eye View
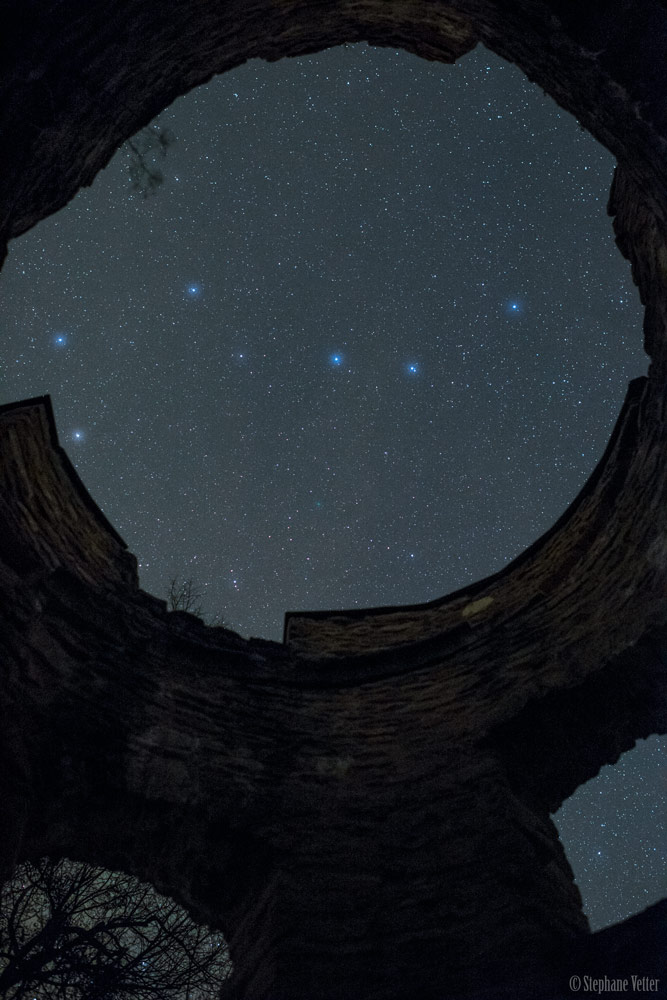
2017 Jupiter Opposition
from NASA's Scientific Visualization Studio: Most Recent Items http://ift.tt/2niXMAw
via IFTTT
Thursday, April 6, 2017
U.S. Trade Group Hacked by Chinese Hackers ahead of Trump-Xi Trade Summit
from The Hacker News http://ift.tt/2nhlyfU
via IFTTT
[FD] CSRF/stored XSS in WordPress Firewall 2 allows unauthenticated attackers to do almost anything an admin can (WordPress plugin)
Source: Gmail -> IFTTT-> Blogger
ISS Daily Summary Report – 4/05/2017
from ISS On-Orbit Status Report http://ift.tt/2nOjOqQ
via IFTTT
Microsoft Finally Reveals What Data Windows 10 Collects From Your PC
from The Hacker News http://ift.tt/2ocX4ns
via IFTTT
Wednesday, April 5, 2017
Orioles Video: Chris Davis crushes solo shot to left-center an inning after Adam Jones hit two-run blast in 3-1 win over Blue Jays (ESPN)
via IFTTT
Blog owner not liable for anonymous defamatory online comments
from Google Alert - anonymous http://ift.tt/2nFzUCm
via IFTTT
TIL 038 : The Bible And Alcoholics Anonymous (feat. Mark Shaw)
from Google Alert - anonymous http://ift.tt/2ncX5bR
via IFTTT
Police: Anonymous tip leads to arrest in Scranton hit-and-run
from Google Alert - anonymous http://ift.tt/2nFMscP
via IFTTT
ALCOHOLICS ANONYMOUS 208-235-1444 AL-ANON 208-232-2692
from Google Alert - anonymous http://ift.tt/2oECop0
via IFTTT
No More Ransom — 15 New Ransomware Decryption Tools Available for Free
from The Hacker News http://ift.tt/2nKNUM1
via IFTTT
Dueling 2-Rd Mock Draft: Mel Kiper gives Ravens two UCLA prospects, pass-rusher Takkarist McKinley at No. 16, CB Fabian Moreau at No. 47 (ESPN)
via IFTTT
Ravens GM Ozzie Newsome says \"high probability\" team adds free agent before draft; C Nick Mangold currently visiting (ESPN)
via IFTTT
Ravens hosting former Clemson WR Mike Williams and ex-Washington WR John Ross for pre-draft visits Wednesday (ESPN)
via IFTTT
ISS Daily Summary Report – 4/04/2017
from ISS On-Orbit Status Report http://ift.tt/2oIm04a
via IFTTT
I have a new follower on Twitter
TrooMobile
TrooMobile : We build mobile solutions! https://t.co/1EnkfkSxu7 https://t.co/AxD8uHwWgU 949.281.4970 hi@troomobile.com
Orange County, CA
http://troomobile.com
Following: 2407 - Followers: 2700
April 05, 2017 at 06:40AM via Twitter http://twitter.com/troomobile
Millions Of Smartphones Using Broadcom Wi-Fi Chip Can Be Hacked Over-the-Air
from The Hacker News http://ift.tt/2nDoH55
via IFTTT
+~ Free Download The Book of Anonymous Quotes sites for free books for kindle ID
from Google Alert - anonymous http://ift.tt/2o23YLQ
via IFTTT
Tim Berners-Lee, Inventor of the Web, Wins $1 Million Turing Award 2016
from The Hacker News http://ift.tt/2oZ8LLv
via IFTTT
NFL Free Agency: C Nick Mangold visiting with Ravens on Tuesday and Wednesday - Jamison Hensley (ESPN)
via IFTTT
Tuesday, April 4, 2017
I have a new follower on Twitter
Extreme Networks
The latest news and updates from Extreme Networks, delivering software-driven networking solutions that help IT departments deliver better business outcomes.
San Jose, California
http://t.co/XMoUXTF4IH
Following: 6369 - Followers: 11338
April 04, 2017 at 06:20PM via Twitter http://twitter.com/ExtremeNetworks
Anonymous Grammar Vigilante Corrects Mistakes On Signs
from Google Alert - anonymous http://ift.tt/2ozgFiB
via IFTTT
pills anonymous meets tuesday and thursday
from Google Alert - anonymous http://ift.tt/2oz2Z7d
via IFTTT
Anonymous Professor
from Google Alert - anonymous http://ift.tt/2nUjFmr
via IFTTT
St Petersburg shuts metro station after anonymous bomb threat
from Google Alert - anonymous http://ift.tt/2nZMvDO
via IFTTT
Ravens trade DT Timmy Jernigan and 2017 3rd-round pick (No. 99) to Eagles for 3rd-rounder (No. 74) this year (ESPN)
via IFTTT
Update Your Apple Devices to iOS 10.3.1 to Avoid Being Hacked Over Wi-Fi
from The Hacker News http://ift.tt/2n8pkbt
via IFTTT
Allow anonymous users to subscribe
from Google Alert - anonymous http://ift.tt/2oF5B08
via IFTTT
Google just discovered a dangerous Android Spyware that went undetected for 3 Years
from The Hacker News http://ift.tt/2nAD7Ts
via IFTTT
ISS Daily Summary Report – 4/03/2017
from ISS On-Orbit Status Report http://ift.tt/2oyspkX
via IFTTT
Hackers stole $800,000 from ATMs using Fileless Malware
from The Hacker News http://ift.tt/2nSwRbL
via IFTTT
Trail Blazer
from Google Alert - anonymous http://ift.tt/2nyGnPr
via IFTTT
Food Addicts in Recovery Anonymous(FA)
from Google Alert - anonymous http://ift.tt/2owmpcp
via IFTTT
Monday, April 3, 2017
The War Room – Episode 32 – Vandalholics Anonymous
from Google Alert - anonymous http://ift.tt/2nVboAu
via IFTTT
(\u25B6)Manny Machado dives down line for spectacular grab and still manages to throw out runner from knees (ESPN)
via IFTTT
\"A Trumbo jumbo!\" Orioles DH crushes walk-off HR in bottom of 11th for 3-2 Opening Day win over Blue Jays - #SCtop10 (ESPN)
via IFTTT
Load JS jQuery Libraries for Anonymous users
from Google Alert - anonymous http://ift.tt/2oCMyDI
via IFTTT
Los Angeles Alcoholics Anonymous
from Google Alert - anonymous http://ift.tt/2mQcdLr
via IFTTT
Beware! Views On New Feature Facebook Stories Feature Won't Be Anonymous
from Google Alert - anonymous http://ift.tt/2nUjGsl
via IFTTT
Android Beats Windows to Become World's Most Popular Operating System
from The Hacker News http://ift.tt/2ou5rvf
via IFTTT
Ravens schedule visit with Temple LB Haason Reddick - Jamison Hensley; possible first-round pick (ESPN)
via IFTTT
Buddy Montana for Ballers Anonymous
from Google Alert - anonymous http://ift.tt/2nTcI6P
via IFTTT
Facial landmarks with dlib, OpenCV, and Python
Last week we learned how to install and configure dlib on our system with Python bindings.
Today we are going to use dlib and OpenCV to detect facial landmarks in an image.
Facial landmarks are used to localize and represent salient regions of the face, such as:
- Eyes
- Eyebrows
- Nose
- Mouth
- Jawline
Facial landmarks have been successfully applied to face alignment, head pose estimation, face swapping, blink detection and much more.
In today’s blog post we’ll be focusing on the basics of facial landmarks, including:
- Exactly what facial landmarks are and how they work.
- How to detect and extract facial landmarks from an image using dlib, OpenCV, and Python.
In the next blog post in this series we’ll take a deeper dive into facial landmarks and learn how to extract specific facial regions based on these facial landmarks.
To learn more about facial landmarks, just keep reading.
Looking for the source code to this post?
Jump right to the downloads section.
Facial landmarks with dlib, OpenCV, and Python
The first part of this blog post will discuss facial landmarks and why they are used in computer vision applications.
From there, I’ll demonstrate how to detect and extract facial landmarks using dlib, OpenCV, and Python.
Finally, we’ll look at some results of applying facial landmark detection to images.
What are facial landmarks?
Figure 1: Facial landmarks are used to label and identify key facial attributes in an image (source).
Detecting facial landmarks is a subset of the shape prediction problem. Given an input image (and normally an ROI that specifies the object of interest), a shape predictor attempts to localize key points of interest along the shape.
In the context of facial landmarks, our goal is detect important facial structures on the face using shape prediction methods.
Detecting facial landmarks is therefore a two step process:
- Step #1: Localize the face in the image.
- Step #2: Detect the key facial structures on the face ROI.
Face detection (Step #1) can be achieved in a number of ways.
We could use OpenCV’s built-in Haar cascades.
We might apply a pre-trained HOG + Linear SVM object detector specifically for the task of face detection.
Or we might even use deep learning-based algorithms for face localization.
In either case, the actual algorithm used to detect the face in the image doesn’t matter. Instead, what’s important is that through some method we obtain the face bounding box (i.e., the (x, y)-coordinates of the face in the image).
Given the face region we can then apply Step #2: detecting key facial structures in the face region.
There are a variety of facial landmark detectors, but all methods essentially try to localize and label the following facial regions:
- Mouth
- Right eyebrow
- Left eyebrow
- Right eye
- Left eye
- Nose
- Jaw
The facial landmark detector included in the dlib library is an implementation of the One Millisecond Face Alignment with an Ensemble of Regression Trees paper by Kazemi and Sullivan (2014).
This method starts by using:
- A training set of labeled facial landmarks on an image. These images are manually labeled, specifying specific (x, y)-coordinates of regions surrounding each facial structure.
- Priors, of more specifically, the probability on distance between pairs of input pixels.
Given this training data, an ensemble of regression trees are trained to estimate the facial landmark positions directly from the pixel intensities themselves (i.e., no “feature extraction” is taking place).
The end result is a facial landmark detector that can be used to detect facial landmarks in real-time with high quality predictions.
For more information and details on this specific technique, be sure to read the paper by Kazemi and Sullivan linked to above, along with the official dlib announcement.
Understanding dlib’s facial landmark detector
The pre-trained facial landmark detector inside the dlib library is used to estimate the location of 68 (x, y)-coordinates that map to facial structures on the face.
The indexes of the 68 coordinates can be visualized on the image below:
Figure 2: Visualizing the 68 facial landmark coordinates from the iBUG 300-W dataset (higher resolution).
These annotations are part of the 68 point iBUG 300-W dataset which the dlib facial landmark predictor was trained on.
It’s important to note that other flavors of facial landmark detectors exist, including the 194 point model that can be trained on the HELEN dataset.
Regardless of which dataset is used, the same dlib framework can be leveraged to train a shape predictor on the input training data — this is useful if you would like to train facial landmark detectors or custom shape predictors of your own.
In the remaining of this blog post I’ll demonstrate how to detect these facial landmarks in images.
Future blog posts in this series will use these facial landmarks to extract specific regions of the face, apply face alignment, and even build a blink detection system.
Detecting facial landmarks with dlib, OpenCV, and Python
In order to prepare for this series of blog posts on facial landmarks, I’ve added a few convenience functions to my imutils library, specifically inside face_utils.py.
We’ll be reviewing two of these functions inside
face_utils.pynow and the remaining ones next week.
The first utility function is
rect_to_bb, short for “rectangle to bounding box”:
# import the necessary packages from collections import OrderedDict import numpy as np import cv2 # define a dictionary that maps the indexes of the facial # landmarks to specific face regions FACIAL_LANDMARKS_IDXS = OrderedDict([ ("mouth", (48, 68)), ("right_eyebrow", (17, 22)), ("left_eyebrow", (22, 27)), ("right_eye", (36, 42)), ("left_eye", (42, 48)), ("nose", (27, 35)), ("jaw", (0, 17)) ]) def rect_to_bb(rect): # take a bounding predicted by dlib and convert it # to the format (x, y, w, h) as we would normally do # with OpenCV x = rect.left() y = rect.top() w = rect.right() - x h = rect.bottom() - y # return a tuple of (x, y, w, h) return (x, y, w, h)
This function accepts a single argument,
rect, which is assumed to be a bounding box rectangle produced by a dlib detector (i.e., the face detector).
The
rectobject includes the (x, y)-coordinates of the detection.
However, in OpenCV, we normally think of a bounding box in terms of “(x, y, width, height)” so as a matter of convenience, the
rect_to_bbfunction takes this
rectobject and transforms it into a 4-tuple of coordinates.
Again, this is simply a matter of conveinence and taste.
Secondly, we have the
shape_to_npfunction:
# import the necessary packages from collections import OrderedDict import numpy as np import cv2 # define a dictionary that maps the indexes of the facial # landmarks to specific face regions FACIAL_LANDMARKS_IDXS = OrderedDict([ ("mouth", (48, 68)), ("right_eyebrow", (17, 22)), ("left_eyebrow", (22, 27)), ("right_eye", (36, 42)), ("left_eye", (42, 48)), ("nose", (27, 35)), ("jaw", (0, 17)) ]) def rect_to_bb(rect): # take a bounding predicted by dlib and convert it # to the format (x, y, w, h) as we would normally do # with OpenCV x = rect.left() y = rect.top() w = rect.right() - x h = rect.bottom() - y # return a tuple of (x, y, w, h) return (x, y, w, h) def shape_to_np(shape, dtype="int"): # initialize the list of (x, y)-coordinates coords = np.zeros((68, 2), dtype=dtype) # loop over the 68 facial landmarks and convert them # to a 2-tuple of (x, y)-coordinates for i in range(0, 68): coords[i] = (shape.part(i).x, shape.part(i).y) # return the list of (x, y)-coordinates return coords
The dlib face landmark detector will return a
shapeobject containing the 68 (x, y)-coordinates of the facial landmark regions.
Using the
shape_to_npfunction, we cam convert this object to a NumPy array, allowing it to “play nicer” with our Python code.
Given these two helper functions, we are now ready to detect facial landmarks in images.
Open up a new file, name it
facial_landmarks.py, and insert the following code:
# import the necessary packages from imutils import face_utils import numpy as np import argparse import imutils import dlib import cv2 # construct the argument parser and parse the arguments ap = argparse.ArgumentParser() ap.add_argument("-p", "--shape-predictor", required=True, help="path to facial landmark predictor") ap.add_argument("-i", "--image", required=True, help="path to input image") args = vars(ap.parse_args())
Lines 2-7 import our required Python packages.
We’ll be using the
face_utilssubmodule of
imutilsto access our helper functions detailed above.
We’ll then import
dlib. If you don’t already have dlib installed on your system, please follow the instructions in my previous blog post to get your system properly configured.
Lines 10-15 parse our command line arguments:
-
--shape-predictor
: This is the path to dlib’s pre-trained facial landmark detector. You can download the detector model here or you can use the “Downloads” section of this post to grab the code + example images + pre-trained detector as well. -
--image
: The path to the input image that we want to detect facial landmarks on.
Now that our imports and command line arguments are taken care of, let’s initialize dlib’s face detector and facial landmark predictor:
# import the necessary packages from imutils import face_utils import numpy as np import argparse import imutils import dlib import cv2 # construct the argument parser and parse the arguments ap = argparse.ArgumentParser() ap.add_argument("-p", "--shape-predictor", required=True, help="path to facial landmark predictor") ap.add_argument("-i", "--image", required=True, help="path to input image") args = vars(ap.parse_args()) # initialize dlib's face detector (HOG-based) and then create # the facial landmark predictor detector = dlib.get_frontal_face_detector() predictor = dlib.shape_predictor(args["shape_predictor"])
Line 19 initializes dlib’s pre-trained face detector based on a modification to the standard Histogram of Oriented Gradients + Linear SVM method for object detection.
Line 20 then loads the facial landmark predictor using the path to the supplied
--shape-predictor.
But before we can actually detect facial landmarks, we first need to detect the face in our input image:
# import the necessary packages from imutils import face_utils import numpy as np import argparse import imutils import dlib import cv2 # construct the argument parser and parse the arguments ap = argparse.ArgumentParser() ap.add_argument("-p", "--shape-predictor", required=True, help="path to facial landmark predictor") ap.add_argument("-i", "--image", required=True, help="path to input image") args = vars(ap.parse_args()) # initialize dlib's face detector (HOG-based) and then create # the facial landmark predictor detector = dlib.get_frontal_face_detector() predictor = dlib.shape_predictor(args["shape_predictor"]) # load the input image, resize it, and convert it to grayscale image = cv2.imread(args["image"]) image = imutils.resize(image, width=500) gray = cv2.cvtColor(image, cv2.COLOR_BGR2GRAY) # detect faces in the grayscale image rects = detector(gray, 1)
Line 23 loads our input image from disk via OpenCV, then pre-processes the image by resizing to have a width of 500 pixels and converting it to grayscale (Lines 24 and 25).
Line 28 handles detecting the bounding box of faces in our image.
The first parameter to the
detectoris our grayscale image (although this method can work with color images as well).
The second parameter is the number of image pyramid layers to apply when upscaling the image prior to applying the detector (this it the equivalent of computing cv2.pyrUp N number of times on the image).
The benefit of increasing the resolution of the input image prior to face detection is that it may allow us to detect more faces in the image — the downside is that the larger the input image, the more computaitonally expensive the detection process is.
Given the (x, y)-coordinates of the faces in the image, we can now apply facial landmark detection to each of the face regions:
# import the necessary packages from imutils import face_utils import numpy as np import argparse import imutils import dlib import cv2 # construct the argument parser and parse the arguments ap = argparse.ArgumentParser() ap.add_argument("-p", "--shape-predictor", required=True, help="path to facial landmark predictor") ap.add_argument("-i", "--image", required=True, help="path to input image") args = vars(ap.parse_args()) # initialize dlib's face detector (HOG-based) and then create # the facial landmark predictor detector = dlib.get_frontal_face_detector() predictor = dlib.shape_predictor(args["shape_predictor"]) # load the input image, resize it, and convert it to grayscale image = cv2.imread(args["image"]) image = imutils.resize(image, width=500) gray = cv2.cvtColor(image, cv2.COLOR_BGR2GRAY) # detect faces in the grayscale image rects = detector(gray, 1) # loop over the face detections for (i, rect) in enumerate(rects): # determine the facial landmarks for the face region, then # convert the facial landmark (x, y)-coordinates to a NumPy # array shape = predictor(gray, rect) shape = face_utils.shape_to_np(shape) # convert dlib's rectangle to a OpenCV-style bounding box # [i.e., (x, y, w, h)], then draw the face bounding box (x, y, w, h) = face_utils.rect_to_bb(rect) cv2.rectangle(image, (x, y), (x + w, y + h), (0, 255, 0), 2) # show the face number cv2.putText(image, "Face #{}".format(i + 1), (x - 10, y - 10), cv2.FONT_HERSHEY_SIMPLEX, 0.5, (0, 255, 0), 2) # loop over the (x, y)-coordinates for the facial landmarks # and draw them on the image for (x, y) in shape: cv2.circle(image, (x, y), 1, (0, 0, 255), -1) # show the output image with the face detections + facial landmarks cv2.imshow("Output", image) cv2.waitKey(0)
We start looping over each of the face detections on Line 31.
For each of the face detections, we apply facial landmark detection on Line 35, giving us the 68 (x, y)-coordinates that map to the specific facial features in the image.
Line 36 then converts the dlib
shapeobject to a NumPy array with shape (68, 2).
Lines 40 and 41 draw the bounding box surrounding the detected face on the
imagewhile Lines 44 and 45 draw the index of the face.
Finally, Lines 49 and 50 loop over the detected facial landmarks and draw each of them individually.
Lines 53 and 54 simply display the output
imageto our screen.
Facial landmark visualizations
Before we test our facial landmark detector, make sure you have upgraded to the latest version of
imutilswhich includes the
face_utils.pyfile:
$ pip install --upgrade imutils
Note: If you are using Python virtual environments, make sure you upgrade the
imutilsinside the virtual environment.
From there, use the “Downloads” section of this guide to download the source code, example images, and pre-trained dlib facial landmark detector.
Once you’ve downloaded the .zip archive, unzip it, change directory to
facial-landmarks, and execute the following command:
$ python facial_landmarks.py --shape-predictor shape_predictor_68_face_landmarks.dat \ --image images/example_01.jpg
Figure 3: Applying facial landmark detection using dlib, OpenCV, and Python.
Notice how the bounding box of my face is drawn in green while each of the individual facial landmarks are drawn in red.
The same is true for this second example image:
$ python facial_landmarks.py --shape-predictor shape_predictor_68_face_landmarks.dat \ --image images/example_02.jpg
Figure 4: Facial landmarks with dlib.
Here we can clearly see that the red circles map to specific facial features, including my jawline, mouth, nose, eyes, and eyebrows.
Let’s take a look at one final example, this time with multiple people in the image:
$ python facial_landmarks.py --shape-predictor shape_predictor_68_face_landmarks.dat \ --image images/example_03.jpg
Figure 5: Detecting facial landmarks for multiple people in an image.
For both people in the image (myself and Trisha, my fiancée), our faces are not only detected but also annotated via facial landmarks as well.
Summary
In today’s blog post we learned what facial landmarks are and how to detect them using dlib, OpenCV, and Python.
Detecting facial landmarks in an image is a two step process:
- First we must localize a face(s) in an image. This can be accomplished using a number of different techniques, but normally involve either Haar cascades or HOG + Linear SVM detectors (but any approach that produces a bounding box around the face will suffice).
- Apply the shape predictor, specifically a facial landmark detector, to obtain the (x, y)-coordinates of the face regions in the face ROI.
Given these facial landmarks we can apply a number of computer vision techniques, including:
- Face part extraction (i.e., nose, eyes, mouth, jawline, etc.)
- Facial alignment
- Head pose estimation
- Face swapping
- Blink detection
- …and much more!
In next week’s blog post I’ll be demonstrating how to access each of the face parts individually and extract the eyes, eyebrows, nose, mouth, and jawline features simply by using a bit of NumPy array slicing magic.
To be notified when this next blog post goes live, be sure to enter your email address in the form below!
Downloads:
The post Facial landmarks with dlib, OpenCV, and Python appeared first on PyImageSearch.
from PyImageSearch http://ift.tt/2o2iZhc
via IFTTT
Facebook Stories Brings The Anonymous Online Creeping To An End
from Google Alert - anonymous http://ift.tt/2o2eq6e
via IFTTT
Ravens plan to meet with Clemson's Mike Williams, as team desperately needs to add WR this offseason - Jamison Hensley (ESPN)
via IFTTT
Overeaters Anonymous Meeting
from Google Alert - anonymous http://ift.tt/2nAcvD5
via IFTTT
ISS Daily Summary Report – 3/31/2017
from ISS On-Orbit Status Report http://ift.tt/2nvOIU2
via IFTTT
Microsoft is Shutting Down CodePlex, Asks Devs To Move To GitHub
from The Hacker News http://ift.tt/2nzCSci
via IFTTT
Alcoholics Anonymous
from Google Alert - anonymous http://ift.tt/2o1ijbL
via IFTTT
Sunday, April 2, 2017
Elegit Dominus Virum de plebe (Anonymous)
from Google Alert - anonymous http://ift.tt/2nZywhA
via IFTTT
You got 30 anonymous offers on your home? Yeah, right
from Google Alert - anonymous http://ift.tt/2nwTvFC
via IFTTT
I have a new follower on Twitter
ElephantLivesMatter
🐘 Elephants are beautiful, gentle, and intelligent creatures. Sadly, they face extermination by ivory poachers. Please follow me.
Worldwide
https://t.co/eiI0SgxDDO
Following: 1488 - Followers: 589
April 02, 2017 at 08:48AM via Twitter http://twitter.com/ElephantBV_Care
2 hours ago
from Google Alert - anonymous http://ift.tt/2n0RvsM
via IFTTT
NGC 602 and Beyond
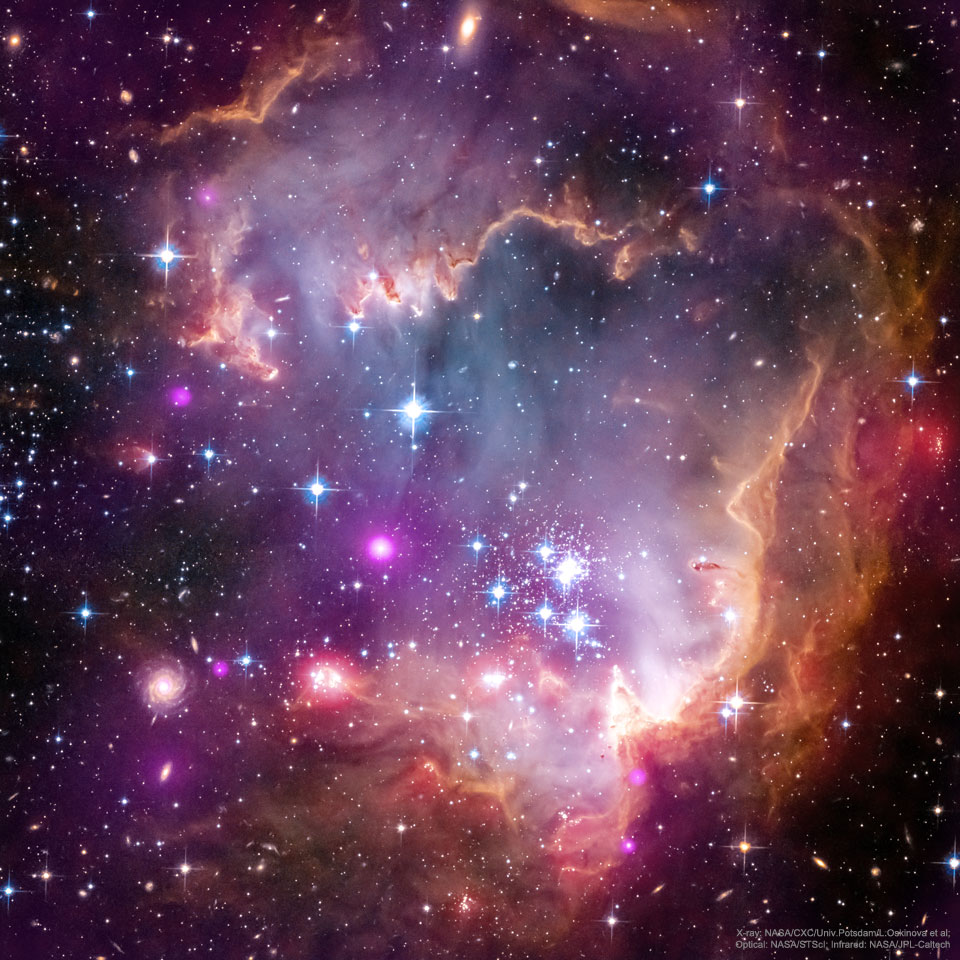